How AI Agents Optimize Predictive Decision Pathways
-
Hello Insight
- 10 min read
Predictive Pathway Optimization is transforming how AI agents make decisions today. By enhancing their ability to analyze vast amounts of data, these agents can identify more efficient pathways to achieve desired outcomes. This advanced optimization not only saves time but also significantly improves accuracy in decision-making processes.
Understanding the principles behind this optimization is crucial for businesses that want to stay competitive. The journey towards effective predictive pathways involves collecting quality data and building robust models. As AI continues to evolve, grasping these concepts will empower organizations to harness the full potential of predictive decision-making.
Analyze & Evaluate Calls. At Scale.
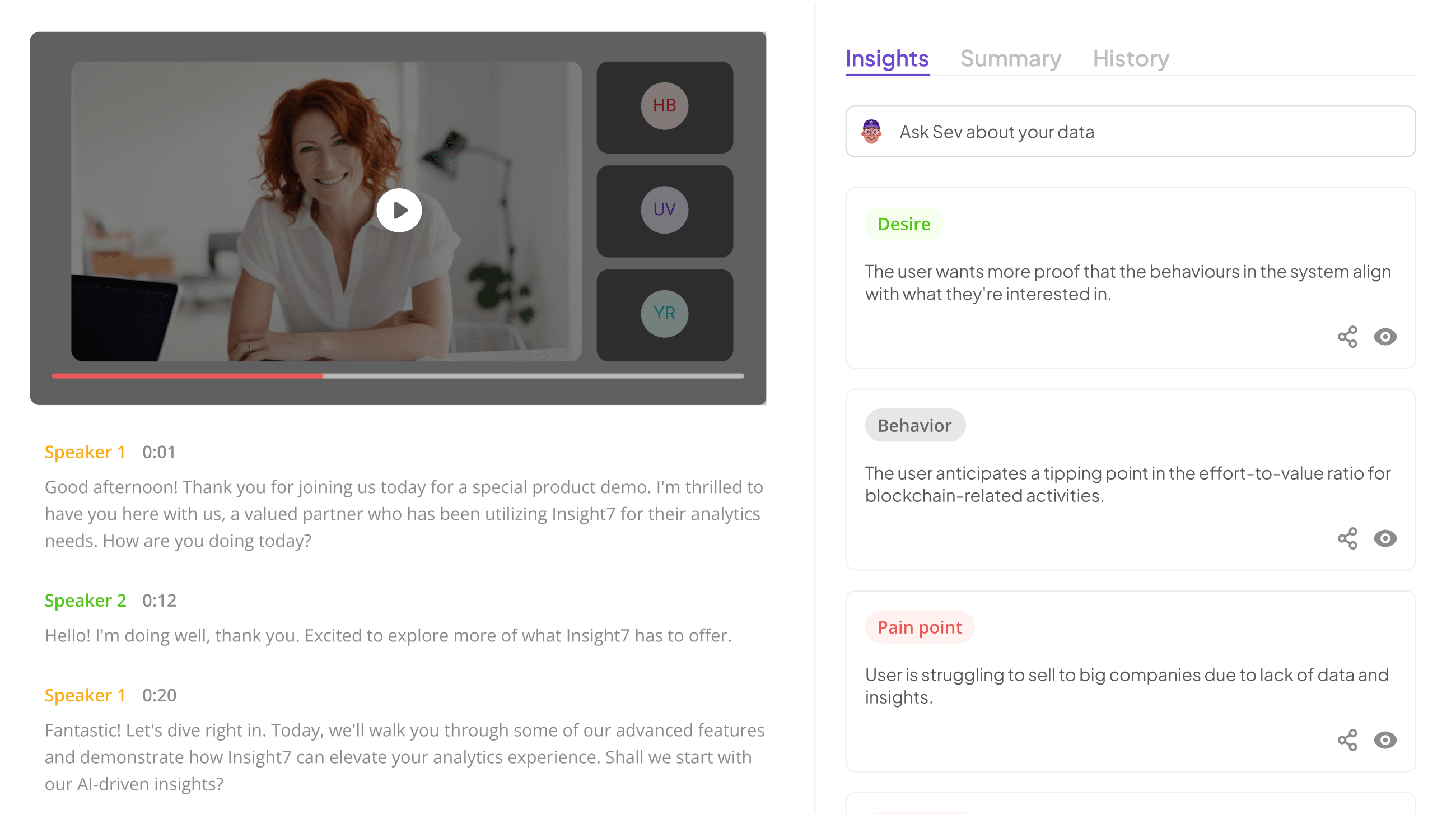
The Role of AI in Enhancing Predictive Pathway Optimization
Artificial Intelligence significantly enhances predictive pathway optimization by transforming how data is analyzed and decisions are made. By employing sophisticated algorithms, AI efficiently processes vast amounts of data, leading to more accurate forecasts and insights. This optimization begins with thorough data collection and preprocessing, which lays the groundwork for the effectiveness of subsequent modeling and simulations. Ensuring that the data is clean and relevant is essential, as quality data directly influences the outcomes of predictive models.
Moreover, AI agents excel in building and testing various predictive models, simulating different scenarios to evaluate potential outcomes. This capability allows organizations to consider multiple decision paths simultaneously, reducing the uncertainty associated with complex decision-making. As AI evolves, integrating these advanced tools becomes increasingly vital for enhancing predictive pathways, ultimately leading to better, data-driven decisions that can sharpen competitive advantages. By understanding the integral role of AI in this optimization process, organizations can harness its power to navigate uncertainties and make informed choices.
Key Principles for Effective Optimization
In the dynamic field of AI, effective optimization is crucial for successful predictive pathway optimization. This process hinges on several core principles that guide the development and implementation of AI agents. First, understanding the importance of quality data is essential. Data collection and preprocessing directly influence the performance of predictive models, as even the most advanced algorithms rely on accurate information to drive sound decisions.
Second, modeling and simulation play a pivotal role. Building robust predictive models requires not only skilled design but also continuous refinement based on outcomes. Simulation allows practitioners to test various scenarios, improving decision-making processes with actionable insights. By focusing on these key principles, organizations can enhance their predictive capabilities, leading to more informed decisions and a greater competitive edge. Staying adaptable and continuously reflecting on data-driven practices ensures effective optimization in every aspect of the predictive pathway.
- Data Collection and Preprocessing
Data collection and preprocessing are essential steps in harnessing AI for predictive pathway optimization. The accuracy of predictions greatly depends on the quality of the data gathered. High-quality data is vital for ensuring that AI models can identify patterns and insights effectively. This phase involves collecting diverse datasets from various sources, including customer feedback, transactional records, and other relevant fields. Careful attention to the data's provenance and completeness is crucial in minimizing errors that could derail decision-making processes.
Once the data is collected, preprocessing becomes the focal point. This entails cleaning, transforming, and organizing data into a usable format for analysis. Missing values must be addressed, noise eliminated, and relevant features extracted. Various techniques, such as normalization and data augmentation, contribute to optimizing the data for predictive models. By emphasizing high-quality data collection and thorough preprocessing, organizations can significantly enhance their predictive pathway optimization, leading to more informed and effective decision-making.
- The Importance of Quality Data
Quality data serves as the backbone of predictive pathway optimization. Without reliable and accurate information, AI agents struggle to generate meaningful insights. Effective decision-making relies on datasets that are current and representative of real-world scenarios. When AI systems are fed quality data, they can identify patterns and trends that support accurate predictions. This capacity enables organizations to make informed choices that drive success and enhance customer engagement.
Furthermore, the quality of data influences the efficiency of optimization processes. Poor data can lead to misguided analyses and ultimately flawed decision paths, jeopardizing outcomes. To ensure the effectiveness of AI-driven strategies, organizations must prioritize data accuracy, relevance, and consistency. Implementing robust data management techniques guarantees that the information used is not only sufficient but also serves as a powerful tool in refining and enhancing predictive models. By understanding the value of quality data, stakeholders can maximize the potential of predictive pathway optimization in their decision-making frameworks.
- Techniques for Efficient Data Management
Efficient data management is the backbone of predictive pathway optimization, providing the necessary structure for insightful analysis. One must begin with solid data collection strategies, ensuring that the information gathered is both relevant and accurate. Techniques such as automated data logging and integrated data sources streamline this process and reduce the potential for errors. Historical data visualization can further enhance understanding by revealing patterns and trends that inform future decisions.
Once the data is in place, preprocessing techniques become essential. Methods such as data cleaning, normalization, and transformation are critical for preparing datasets for analysis. Effective management also involves implementing robust storage solutions, ensuring quick access to data for real-time insights. In doing so, AI agents can harness this well-organized information, monitoring ongoing trends and fostering informed decisions that optimize predictive pathways in any scenario.
- Modeling and Simulation
In this section, we explore how modeling and simulation play crucial roles in predictive pathway optimization. Through robust predictive models, AI agents can analyze complex datasets to forecast potential outcomes effectively. These models leverage historical data to identify patterns and establish relationships, which inform decision-makers about likely scenarios and their implications.
Simulating outcomes enables organizations to visualize various pathways and assess potential risks and benefits. This empirical approach to decision-making allows for an iterative process where various strategies can be compared. Additionally, simulation helps to pinpoint high-reward options while minimizing risks associated with alternative pathways. By integrating modeling and simulation, AI agents enhance the ability to navigate uncertain environments and optimize decision-making processes for superior performance.
- Building Robust Predictive Models
Building robust predictive models involves a comprehensive approach to understanding data and its nuances. In essence, these models serve as the backbone of predictive pathway optimization, transforming raw data into actionable insights. A key aspect is ensuring data quality, as the effectiveness of predictive models hinges on reliable datasets. This requires diligent preprocessing, which includes cleaning and structuring data for maximum impact.
Furthermore, building predictive models involves employing advanced algorithms that can simulate various outcomes. By testing different scenarios, organizations can refine their decision-making processes, leading to more effective strategies. Through continuous iteration and validation, these models can adapt and incorporate new information, enhancing their accuracy over time. In this way, constructing robust predictive models not only supports immediate business needs but also lays the groundwork for long-term success through informed decision-making.
- Simulating Outcomes for Better Decision-Making
Effective decision-making often requires forecasting future outcomes based on available data. Simulating outcomes helps organizations visualize various scenarios and their implications. This approach enables better strategizing by presenting clear pathways and potential consequences of different decisions.
Predictive Pathway Optimization involves creating dynamic models that forecast various potential outcomes. These simulations utilize historical data and identify trends, allowing decision-makers to explore options visually. For example, an organization might assess the effects of introducing a new product by simulating customer responses, market conditions, and competitor actions. By understanding these dynamics, decision-makers can anticipate challenges and capitalize on opportunities, ultimately leading to more informed choices and improved business outcomes.
This simulation-based approach empowers organizations to navigate uncertainty and make proactive decisions. Integrating sophisticated AI tools in this process elevates the accuracy of these predictive analyses, ensuring that organizations remain agile and responsive in a rapidly changing environment.
[remaining content here…]Tools for Predictive Pathway Optimization in AI
In the realm of AI, tools for predictive pathway optimization serve as a foundation for effective decision-making. Utilizing these tools allows organizations to refine their processes and improve overall outcomes. They can analyze vast amounts of data, identify patterns, and accurately predict future scenarios. By enhancing the quality of insights derived from data, these tools facilitate better strategic planning and execution.
Key tools essential for predictive pathway optimization include Insight7, which offers robust data analytics capabilities for actionable insights. IBM Watson Studio stands out with its comprehensive modeling and simulation features, enabling detailed scenario analysis. Additionally, H2O.ai provides scalable solutions that adapt as data needs evolve, while DataRobot simplifies the model-building process. Lastly, Google Cloud AI Platform ensures a versatile approach to deployment with various practical applications. Each of these tools offers unique attributes that significantly contribute to optimizing predictive pathways in AI.
Essential Tools to Consider
When considering essential tools for Predictive Pathway Optimization, several key options stand out for their innovative capabilities. Tools like Insight7 and IBM Watson Studio offer comprehensive data analysis and modeling features that enhance decision-making. Insight7 focuses on user-centric insights, streamlining the process of capturing essential customer data. On the other hand, IBM Watson Studio provides an integrated workflow that facilitates smooth collaboration among data scientists and business analysts, which fosters effective predictive modeling.
Additionally, platforms like H2O.ai and DataRobot bring unique attributes to the table. H2O.ai excels in democratizing machine learning, allowing organizations to implement sophisticated models easily. DataRobot's automated machine learning capabilities enable rapid deployment of predictive models, yielding swift insights. Lastly, Google Cloud AI Platform supports scalability and accessibility, making it versatile for various applications. Using these tools strategically can significantly enhance your approach to Predictive Pathway Optimization, empowering more informed decision-making processes.
- Insight7
AI agents are revolutionizing the way we approach predictive pathway optimization. By effectively analyzing vast amounts of customer data, these agents facilitate timely decision-making that enhances strategic planning. Insights gleaned from customer interactions inform business actions, enabling organizations to stay ahead in a competitive landscape. The key lies in transforming raw customer signals into actionable choices, which requires sophisticated models and adequate data management.
In this context, focusing on the intricacies of AI-driven analysis is vital. Efficient data collection and preprocessing ensure that the input used for predictive modeling is of high quality. Simulating various outcomes based on these models allows businesses to visualize potential scenarios and refine strategies accordingly. Embracing these practices not only improves predictive accuracy but also ensures that insights drive meaningful actions, ultimately leading to enhanced performance in decision-making processes.
- Features and Benefits
AI agents optimize predictive pathways through a variety of features and benefits that significantly enhance decision-making processes. One of the core advantages lies in their ability to collect and preprocess vast amounts of data. This ensures the quality and relevance of insights, enabling organizations to make informed decisions swiftly. By utilizing advanced algorithms, AI agents can model scenarios accurately, simulating different outcomes for better predictive analysis.
Additionally, these agents facilitate collaboration among teams by providing easy access to real-time information. Their data visualization capabilities break down complex datasets, allowing stakeholders to understand trends and patterns effortlessly. This transparency not only boosts confidence in the decision-making process but also fosters an environment of continuous improvement, as teams can quickly adjust strategies based on insights derived from AI. Overall, predictive pathway optimization empowers organizations to navigate challenges effectively, ensuring they stay ahead in a competitive market.
- Use Cases
The application of predictive pathway optimization through AI agents is rapidly transforming various industries, enhancing their decision-making processes. One prominent use case involves quality assurance, where AI assesses customer interactions to evaluate compliance with established standards. This not only streamlines evaluations but also ensures that customer service representatives adhere to best practices.
Moreover, AI agents can synthesize large datasets from diverse channels, facilitating more informed decision-making. For example, by analyzing call transcripts, AI identifies key areas for improvement and provides granular insights on customer engagement. This use case exemplifies how predictive pathway optimization can lead to substantial enhancements in performance, ultimately improving customer satisfaction and operational efficiency. Organizations that harness these capabilities will be better positioned to adapt, respond to customer needs, and maintain a competitive edge in their respective markets.
- IBM Watson Studio
This platform serves as an accessible environment for predictive pathway optimization, enabling users to harness AI capabilities without the need for extensive training. Its user-friendly interface allows team members to generate reports, transcribe files, and identify customer experience pain points effortlessly. The design promotes democratization of insights, ensuring that anyone within an organization can contribute to data-driven decision-making.
Within this platform, users can organize various files, allowing for in-depth analysis at both the individual and project levels. The system automatically extracts valuable insights, highlighting key themes such as customer pain points and desires. By utilizing this analytical framework, businesses can better understand the factors impacting their predictive pathway optimization, leading to improved strategies for decision-making and enhanced overall performance. This approach fosters a culture of informed decision-making while streamlining processes, ultimately paving the way for more effective and efficient outcomes.
- Overview and Capabilities
Predictive Pathway Optimization is a transformative approach that harnesses the power of artificial intelligence to enhance decision-making processes. By employing advanced algorithms, AI agents analyze vast amounts of data, uncovering insights that drive strategic choices. This optimization enables organizations to pinpoint critical factors influencing outcomes, ultimately leading to more effective actions.
AI agents exhibit exceptional capabilities in both data processing and decision modeling. Through automated data collection and preprocessing, they ensure high-quality inputs, which are essential for building robust predictive models. Moreover, these agents simulate different scenarios, allowing businesses to visualize potential outcomes and refine their strategies. The seamless integration of these capabilities empowers organizations to respond proactively to dynamic market conditions, assertively navigating their predictive pathways. As AI continues to evolve, the potential for optimizing decision pathways grows, opening new avenues for innovation and success.
- Integration Workflow
An effective integration workflow is crucial in optimizing predictive pathways in AI systems. This process begins with aligning data sources and various applications for seamless information flow. By consolidating resources, businesses can reduce redundancy and enhance collaboration across teams. This unified approach not only streamlines operations but also accelerates the decision-making timeline, ultimately maximizing predictive pathway optimization.
Furthermore, effective integration involves careful modeling and simulation of outcomes. By utilizing advanced algorithms, businesses can generate insights from data and anticipate potential scenarios. This predictive modeling helps teams understand the implications of their choices, leading to improved strategies and outcomes. By focusing on integration workflows, organizations can harness the power of AI to develop more accurate and actionable insights, enhancing both operational efficiency and stakeholder satisfaction.
- H2O.ai
The integration of advanced algorithms in AI significantly enhances predictive pathway optimization, enabling organizations to make informed decisions based on reliable data insights. By utilizing innovative machine learning techniques, organizations can streamline their decision-making processes, thus improving efficiency. This powerful capability is increasingly becoming essential across various sectors, allowing businesses to forecast outcomes with remarkable accuracy.
One of the key features within this domain includes robust model-building capabilities that leverage diverse datasets. This facilitates the simulation of various scenarios, providing organizations with the foresight to explore different decision pathways. Ultimately, as businesses embrace these advancements in technology, the ability to optimize decision-making processes will only grow stronger, driving more effective strategies for addressing future challenges. Harnessing these technologies is vital for staying competitive and adapting to changing market dynamics.
💬 Questions about How AI Agents Optimize Predictive Decision Pathways?
Our team typically responds within minutes
- Key Features
AI agents are redefining how organizations approach Predictive Pathway Optimization. One key feature of these agents is their ability to efficiently collect and preprocess data. This function ensures that only high-quality data is used, which is vital for reliable predictions. By employing advanced techniques, AI simplifies data management, making it accessible to users at all levels of expertise.
Another noteworthy feature is the capability for robust modeling and simulation. AI agents can create predictive models that accurately forecast various outcomes based on historical data. Simulating these scenarios allows organizations to make informed decisions quickly, ultimately leading to enhanced operational efficiency. As AI technology continues to evolve, its potential to streamline predictive pathways and drive impactful decision-making will significantly benefit organizations striving for competitive advantage.
- Applications in Various Industries
AI agents are transforming various industries by enhancing predictive decision-making processes. In healthcare, predictive pathway optimization assists with patient outcome forecasting, enabling personalized treatment plans. This technology analyzes historical data and real-time inputs, thus reducing response times and making interventions more timely.
In finance, risk management benefits from AI's ability to predict market trends and customer behavior. By analyzing vast amounts of financial data, AI aids in identifying potential risks, optimizing investment pathways, and ultimately enhancing returns. Manufacturing industries also leverage predictive analytics to streamline production processes and ensure inventory management, effectively minimizing waste and maximizing efficiency.
Throughout sectors such as retail, transportation, and energy, predictive pathway optimization fosters a proactive rather than reactive approach. By integrating AI into decision-making frameworks, organizations can cultivate agility, drive innovation, and exceed customer expectations. Through continuous improvement and data-driven insights, the journey toward optimization is well underway across numerous fields.
- DataRobot
In the realm of Predictive Pathway Optimization, one crucial aspect is the utilization of advanced algorithms for improved predictions. These algorithms are designed to analyze vast amounts of data efficiently, revealing insights that can guide crucial business decisions. Selecting the right platform for this purpose can significantly enhance the optimization process.
The implementation of specific features on the chosen platform is essential for obtaining actionable insights. For instance, the platform must facilitate seamless data integration, allowing the analysis of various data types simultaneously. This capability enables users to examine patterns and relationships that inform strategic pathways, ensuring that decision-making is based on comprehensive and relevant information. Additionally, effective visualization tools allow stakeholders to easily interpret data, fostering a collaborative environment for decision-making. By focusing on these areas, organizations can improve their predictive decision pathways and ultimately achieve better outcomes.
- Unique Attributes
The unique attributes of AI agents in the realm of Predictive Pathway Optimization significantly enhance their capacity to make informed decisions. One key attribute involves data-driven insights that AI systems utilize to craft predictive models. These models not only analyze historical data but also simulate potential future scenarios, offering a comprehensive view of possible outcomes. As a result, organizations can make strategic choices rooted in empirical evidence, minimizing risks associated with uncertainty.
Another noteworthy feature is the adaptability of AI agents. They learn from past interactions and continuously refine their algorithms based on emerging data. This dynamic learning process allows these systems to optimize predictive pathways over time and respond to shifting variables in real time. Ultimately, this helps organizations navigate complex decision-making environments more effectively. By leveraging unique attributes, AI agents empower businesses to improve efficiency and enhance decision quality.
- Implementation and Results
The journey of implementation begins with a thorough grasp of data collection and preprocessing. This phase establishes a solid foundation essential for successful predictive pathway optimization. Ensuring data quality is paramount; poor data can lead to misleading insights. Techniques such as normalization and missing value treatment are vital for preparing data appropriately, creating a reliable dataset to fuel AI algorithms.
Following preprocessing, the modeling phase takes center stage. Robust predictive models offer simulations of various decision pathways, enriching insights and enhancing overall decision-making outcomes. Through iterative testing and refinement, AI agents learn from real-world applications, adjusting pathways in response to changing variables. The results from this implementation phase illustrate the substantial impact of AI on predictive pathway optimization, providing actionable insights that drive efficiencies and inform strategic decisions. This dual approach, combining data integrity with sophisticated modeling, exemplifies the transformative potential of AI in decision-making frameworks.
- Google Cloud AI Platform
The Google Cloud AI Platform offers a powerful environment for organizations seeking to enhance their predictive pathway optimization capabilities. Through its robust tooling and infrastructure, users can build and deploy machine learning models, streamlining the analysis of complex datasets. This platform supports various applications, enabling businesses to harness insights from their data effectively. With its extensive documentation and user-friendly interfaces, even those new to AI can effectively navigate the landscape.
By integrating various data sources, the platform empowers users to analyze trends and make informed decisions swiftly. Predictive pathway optimization becomes more accessible as organizations can simulate different scenarios, assessing potential outcomes before execution. The Google Cloud AI Platform's capabilities not only improve decision-making processes but also encourage collaboration across teams, ensuring that all stakeholders contribute to developing tailored solutions. This ensures that companies can optimize their operations efficiently while fostering innovation.
- Best Practices for Deployment
Successful deployment of AI agents for predictive pathway optimization hinges on a structured approach that combines strategic planning with real-time data management. Initially, clearly defining objectives ensures that the deployed systems are aligned with business goals, which can significantly enhance decision-making efficiency. Additionally, it is vital to invest in robust data preprocessing techniques. Ensuring high-quality data minimizes inaccuracies and provides a solid foundation for predictive modeling.
To maintain effective operations, continual monitoring of AI agent performance is essential. Leveraging metrics allows organizations to assess how well the predictive models function over time, ensuring they adapt to changing conditions. Furthermore, providing training for end-users can foster a deeper understanding of the optimization tools available, ensuring that team members can make informed decisions. Establishing these best practices promotes a seamless integration of AI agents into existing workflows, ultimately optimizing predictive pathways.
- A Broad Range of Uses
AI agents are transforming multiple sectors through predictive pathway optimization, enabling organizations to enhance their decision-making processes. The adaptability of these agents allows for tailored applications across various industries, from customer service to quality assurance. For instance, organizations can use AI technology to analyze call transcripts and evaluate the quality of interactions based on established criteria. This optimization not only improves customer engagement but also fosters better compliance with regulatory standards.
Additionally, predictive pathway optimization can streamline workflows and enhance efficiency. Companies in the healthcare sector are leveraging AI for predictive analytics to forecast patient outcomes effectively, which supports better resource allocation. On the other hand, the finance industry employs AI to predict market trends, significantly improving investment strategies. These examples illustrate how versatile AI agents can be across different domains, showcasing their value to diverse sectors aiming for innovation in decision-making.
Future Trends in Predictive Pathway Optimization
The future of Predictive Pathway Optimization is poised to undergo significant transformation, driven by technological advancements and changing market dynamics. One prominent trend is increased automation, where machine learning algorithms streamline decision-making processes, reducing human intervention and enhancing rapid response capabilities. However, this shift requires careful consideration of potential challenges, such as biases in automated systems or the need for human oversight.
Moreover, advancements in interpretability are set to enhance transparency in AI-driven models. As organizations seek to understand the decision-making processes behind predictive analytics, tools that improve the clarity of these models will become invaluable. Techniques such as explainable AI are being developed to provide insights into how and why certain pathways are chosen, fostering trust among users and stakeholders. Engaging with these trends will be crucial for organizations aiming to optimize their predictive decision pathways in the rapidly evolving landscape of AI technologies.
Emerging Technologies and Their Impact
In today's rapidly evolving technological landscape, emerging technologies profoundly affect predictive decision-making processes. Innovations like artificial intelligence and machine learning drive significant advancements in predictive pathway optimization. These technologies facilitate greater accuracy in forecasting outcomes, enabling organizations to enhance their strategic decision-making.
One of the key impacts of emerging technologies is increased automation. By employing machine learning algorithms, businesses can automate data analysis, reducing human error and increasing efficiency. This automation not only saves time but also allows for more complex analyses that were previously unattainable. Additionally, advancements in interpretability improve transparency, allowing stakeholders to understand the reasoning behind predictive models. Such progress enables organizations to make informed decisions with greater confidence, ultimately driving better outcomes and growth.
Understanding these advancements is crucial for optimizing predictive pathways in any sector, ensuring that enterprises remain competitive in a technology-driven world.
- Increased Automation
Increased automation plays a crucial role in optimizing predictive pathways, significantly transforming how data-driven decisions are made. As organizations embrace machine learning technologies, the manual elements of data analysis and decision-making processes are streamlined. Automation reduces human error and enhances the efficiency of processing vast datasets, allowing predictive models to generate insights rapidly and accurately.
Furthermore, automating these processes allows teams to focus on strategic planning rather than routine tasks. This shift not only increases productivity but also improves the precision of predictive pathway optimization, enabling more informed decisions based on real-time data. As the demand for faster and smarter decision-making grows, automation is becoming an essential component of predictive analytics. Incorporating automated systems ultimately leads to better alignment between data analysis and business objectives, driving overall success in various industries.
- The Role of Machine Learning in Automation
Machine learning plays a pivotal role in automation, driving efficiency and consistency while streamlining complex processes. By analyzing vast datasets, machine learning algorithms identify patterns and trends that human analysts might overlook. This enables organizations to automate decision-making processes and optimize predictive pathways, leading to improved outcomes.
The integration of machine learning in automation allows systems to learn from previous actions and results. This self-improvement cycle enhances accuracy over time, making eventual predictions more reliable. Specific applications include predictive maintenance, where algorithms forecast equipment failures, and customer experience optimizations, where AI anticipates individual preferences. As a result, businesses can make informed choices swiftly, thus fostering innovation and maintaining a competitive edge in their respective industries. Overall, the collaboration of automated systems and machine learning amplifies the potential of predictive pathway optimization, reshaping operational landscapes.
- Benefits and Potential Challenges
AI agents have the potential to significantly enhance predictive pathway optimization, offering numerous benefits. These intelligent systems analyze vast amounts of data quickly, identifying trends and insights that inform decision-making processes. By leveraging advanced algorithms, organizations can reduce uncertainty in their strategies, leading to more effective and timely decisions. Furthermore, AI enables continuous learning, allowing models to adapt and improve over time, ultimately providing deeper insights and fostering innovation.
However, the integration of AI in predictive decision pathways does come with challenges. One major concern is data quality, as biased or incomplete information can lead to flawed predictions. Additionally, organizations may face resistance from employees due to apprehension about job displacement or the complexity of new technologies. Ensuring transparency in AI operations and focusing on user training can help mitigate some of these challenges. Balancing these benefits and potential issues is essential for harnessing the full capabilities of AI in enhancing predictive pathways.
- Advancements in Interpretability
The field of AI has seen significant advancements in interpretability, enhancing how predictive pathway optimization is approached. Improved transparency in AI models allows stakeholders to understand the reasons behind model outputs, thereby facilitating trust in decision-making. This increased clarity becomes crucial when making strategic choices, as it enables users to align predictions with their goals effectively.
Several techniques and tools have emerged to support interpretability. For instance, model-agnostic methods offer insights across various predictive models, allowing for broader application. Additionally, visualization tools help articulate complex data outcomes clearly, turning abstract predictions into actionable insights. Methods such as feature importance and SHAP values are particularly effective in elucidating how input variables contribute to final predictions, fostering a deeper understanding of AI-driven decisions. These advancements are reshaping the landscape, empowering organizations to optimize their predictive pathways with confidence.
- Improving Transparency in AI Models
Transparency in AI models is a crucial aspect of predictive pathway optimization. As AI systems make increasingly complex decisions, users and stakeholders require a clear understanding of how these decisions are made. Enhancing transparency not only builds trust but also facilitates accountability in AI systems, enabling users to assess models' performance and decisions more effectively.
One way to improve transparency is by implementing interpretability tools. These tools can shed light on the inner workings of a model while providing insights into its decision-making processes. Another approach involves creating documentation that details the data sources, algorithms, and assumptions used in model development. This transparency allows users to discern the rationale behind a model’s predictions, ultimately leading to better-informed decisions and strategies. By fostering a culture of transparency, organizations can greatly enhance the effectiveness of predictive pathway optimization in their AI applications.
- Tools and Techniques for Better Understanding
To foster a better understanding of predictive pathway optimization, it's essential to utilize various tools and techniques. Embracing advanced analytics and artificial intelligence can significantly enhance decision-making processes. These methods allow organizations to identify trends and behaviors within vast datasets, ultimately leading to more informed choices.
Several key techniques are particularly effective in this pursuit. First, employing intuitive data visualization tools can simplify complex information, making it more accessible to stakeholders. Second, implementing real-time analytics enables teams to react swiftly to emerging insights, ensuring decisions are timely and relevant. Lastly, utilizing collaborative platforms enhances knowledge-sharing among team members, fostering a collective approach to problem-solving. By integrating these strategies, businesses can optimize predictive pathways and gain a competitive edge in their respective markets.
Conclusion: Maximizing Potential with Predictive Pathway Optimization
In today's rapidly evolving landscape, maximizing potential through Predictive Pathway Optimization becomes paramount for success. By harnessing AI agents, businesses can transform vast amounts of data into actionable insights, paving the way for informed decision-making. This optimization process not only streamlines workflows but also enhances the accuracy of predictions, enabling organizations to stay ahead of the competition.
Moreover, as technology advances, the continuous refinement of predictive models ensures that outcomes remain relevant and effective. As companies embrace these innovations, they unlock new opportunities to improve customer experiences and drive growth. Ultimately, the strategic application of Predictive Pathway Optimization empowers organizations to navigate challenges with confidence and agility, thereby realizing their fullest potential.
💬 Questions about How AI Agents Optimize Predictive Decision Pathways?
Our team typically responds within minutes