Google-Based Tools to Analyze Interview Data Automatically
-
Bella Williams
- 10 min read
Automated Interview Analysis is revolutionizing how organizations process qualitative data. As interview-driven insights become crucial, the challenge of manual data analysis can lead to inconsistencies and slow turnaround times. By utilizing automation, research teams can streamline their workflows, ensuring quicker access to meaningful insights while minimizing bias.
Incorporating technology into the analysis process can enhance both speed and accuracy. With tools that harness artificial intelligence, researchers can focus on extracting valuable findings rather than getting bogged down in tedious tasks. As this methodology evolves, it promises to reshape how businesses approach user feedback and market research, ultimately improving decision-making processes.
Extract insights from Customer & Employee Interviews. At Scale.
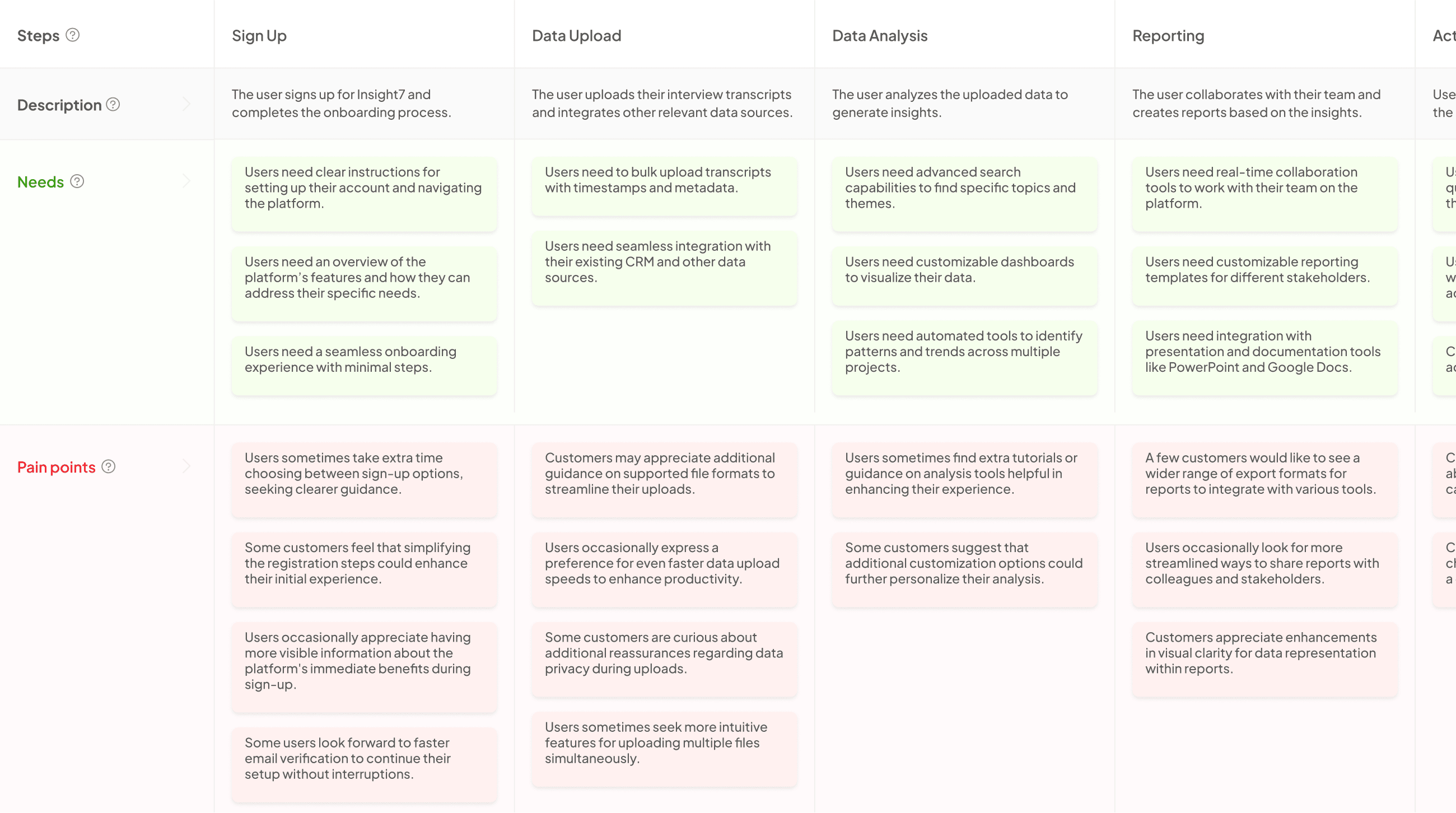
Tools for Automated Interview Analysis
Automated Interview Analysis streamlines the often tedious process of extracting insights from interviews. Analyzing interviews manually can consume significant time and lead to inconsistent results. Automation tools can efficiently process large volumes of data, providing rapid insights that enhance decision-making. Many organizations seek to reduce delays in reporting and improve data accuracy through these innovative tools.
Among the many available solutions, several Google-based tools stand out. The Google Cloud Natural Language API performs sentiment analysis, entity recognition, and more, enabling nuanced insights from qualitative data. Additionally, Google AutoML for natural language offers customizable models for analyzing interview transcripts. These tools can help ensure consistency and clarity in data interpretation, fostering improved collaboration among research teams. By implementing automated analysis, organizations can cultivate richer insights while freeing up valuable time for strategic initiatives.
Insight7: Leading the Way in Analysis
Automated Interview Analysis marks a pivotal advancement in converting raw interview data into meaningful insights. As companies increasingly rely on data-driven decision-making, the ability to analyze large volumes of interview transcripts quickly and accurately is invaluable. By incorporating modern technology, organizations can save time and resources while enhancing the quality of their analysis.
The journey towards effective Automated Interview Analysis can be encapsulated in a few key aspects. First, efficient data capture is crucial. Teams should focus on collecting clear, comprehensive transcripts to establish a strong foundation for further analysis. Second, employing Google-based tools can streamline the process significantly. These tools can quickly parse through extensive datasets and identify themes, sentiment, and relevant information without human bias. Lastly, integrating findings into actionable insights will enable teams to make informed decisions based on comprehensive analysis rather than instinct alone. Embracing automated solutions can enhance the workflow, leading to improved strategies and outcomes in various industries.
Google Cloud Natural Language API
The Google Cloud Natural Language API is a powerful asset for automated interview analysis. This tool allows users to analyze text to extract meaningful insights, uncover sentiments, and identify key entities. By processing interview transcripts or audio scripts, the API helps teams gain a deeper understanding of participantsโ responses and experiences through text analysis. Automated technology simplifies the evaluation of large volumes of qualitative data, significantly reducing manual effort.
Key features enhance its utility for interview analysis. First, sentiment analysis offers insights into the emotional tone of responses, providing context that numbers alone cannot convey. Second, entity recognition identifies people, places, and terminology relevant to the discussion, maintaining focus on what matters most. Lastly, the ability to categorize themes from responses ensures researchers can draw actionable conclusions quickly. By integrating this API into interview analysis workflows, teams can benefit from efficiency and accuracy while revealing nuances in participant feedback.
Google AutoML for Natural Language
Google AutoML for Natural Language offers a powerful solution for analyzing data from interviews automatically. This tool simplifies the process of extracting insights from conversational data, providing users with meaningful analysis while saving time. By utilizing machine learning algorithms, it can identify key themes, sentiments, and entities within the text, ensuring a comprehensive understanding of the information gathered.
When using Google AutoML, users can easily train models to fit their specific needs, allowing for tailored responses to unique interview contexts. This customization enables the extraction of relevant insights for various applications, whether in research, product development, or customer feedback. Moreover, the model evolves as new data is introduced, improving accuracy over time.
Incorporating automated interview analysis not only enhances efficiency but also supports user-driven decisions, leading to better outcomes in research and development efforts. This integration of technology into qualitative analysis transforms how organizations derive insights from interviews, ultimately facilitating more strategic decision-making.
Steps for Implementing Automated Interview Analysis
Implementing Automated Interview Analysis begins by collecting and preparing your data effectively. Initially, gather interview transcripts and ensure they are well-structured, as accurate data forms the backbone of any successful analysis. Proper formatting allows tools to identify essential themes and insights, thus making the analysis process smoother.
Next, move on to employing Google-based tools specifically designed for analyzing this data. Utilize platforms such as the Google Cloud Natural Language API to extract relevant keywords and sentiment analysis efficiently. Once the initial insights are drawn, you can utilize Google AutoML to refine your findings further, ensuring deeper insights are uncovered. By following these steps, your team will experience a notable reduction in manual analysis time, yielding quicker, more consistent, and unbiased insights from interview data. Embracing automation will ultimately enhance your research quality and empower your decision-making process.
Generate Journey maps, Mind maps, Bar charts and more from your data in Minutes
Step 1: Data Collection and Preparation
To initiate a successful process of automated interview analysis, the first step is data collection and preparation. This phase is critical as it lays the groundwork for accurate and effective analysis. Begin by organizing all the interview data youโve gathered, ensuring that itโs comprehensive and clearly labeled. This includes audio files, transcriptions, and any supplementary materials that may provide context.
Next, prepare the data for analysis. This involves using transcription tools to convert audio recordings into text format, which can be more easily manipulated. Many platforms offer native transcription services that support multiple languages and maintain high accuracy rates, which can save significant time. After transcription, review the data to correct any inaccuracies and maintain clarity. Once your data is organized and prepared, you can seamlessly transition to the next phase, enhancing the quality of insights derived from automated interview analysis.
Step 2: Leveraging Google-Based Tools for Analysis
In the realm of automated interview analysis, utilizing Google-based tools can significantly enhance efficiency and accuracy. Start by exploring the Google Cloud Natural Language API, which excels at extracting insights from text data. This tool offers sentiment analysis, allowing researchers to understand the emotional tone behind responses, providing invaluable context for analysis.
Next, consider Google AutoML for Natural Language. This platform enables users to create custom models tailored to specific data types, such as interview transcripts. By training these models on existing data, you can streamline the process of categorizing and drawing insights from responses. Finally, integrate mind mapping tools to visually represent themes and insights, making complex data more digestible. Emphasizing user-defined goals during this analysis will enhance the relevance of generated insights. By combining these Google tools thoughtfully, you pave the way for a more intuitive understanding of your interview data.
Conclusion: Enhancing Insights with Automated Interview Analysis
Automated Interview Analysis brings a transformative approach to understanding qualitative data. By utilizing advanced technologies, organizations can streamline the process of extracting meaningful insights from interviews. This not only saves time but also reduces the potential for bias and inconsistency that often arises in manual analysis. With quicker turnaround times, teams can respond to client needs more efficiently, ensuring that valuable insights are readily available.
Implementing these automated tools provides a dual advantage. First, they facilitate more accurate analysis, allowing multiple researchers to function cohesively. Second, they empower smaller teams to manage larger volumes of qualitative data without being overwhelmed. Embracing these solutions can significantly enhance the quality of insights derived from interviews, leading to informed decision-making and improved outcomes.
๐ฌ Need Help? Chat with Support
Our team typically responds within minutes