Generative AI Agents for Predictive Customer Feedback Analysis
-
Bella Williams
- 10 min read
AI-Driven Feedback Forecasting has emerged as a vital tool in understanding customer preferences and behaviors. In an era where customer feedback is abundant, synthesizing this data effectively can turn insights into strategic advantages. By harnessing advanced generative AI technologies, businesses can accurately predict trends and responses, shaping products and services that resonate with their audience.
As we delve into the mechanics of AI-Driven Feedback Forecasting, the emphasis will be on how it empowers companies to translate unstructured feedback into actionable insights. This introduction serves to inspire analyses that not only recognize customer sentiments but also anticipate future needs, ultimately driving innovation and enhancing customer satisfaction. Understanding these dynamics will be essential for leveraging technology in today's competitive marketplaces.
Analyze & Evaluate Calls. At Scale.
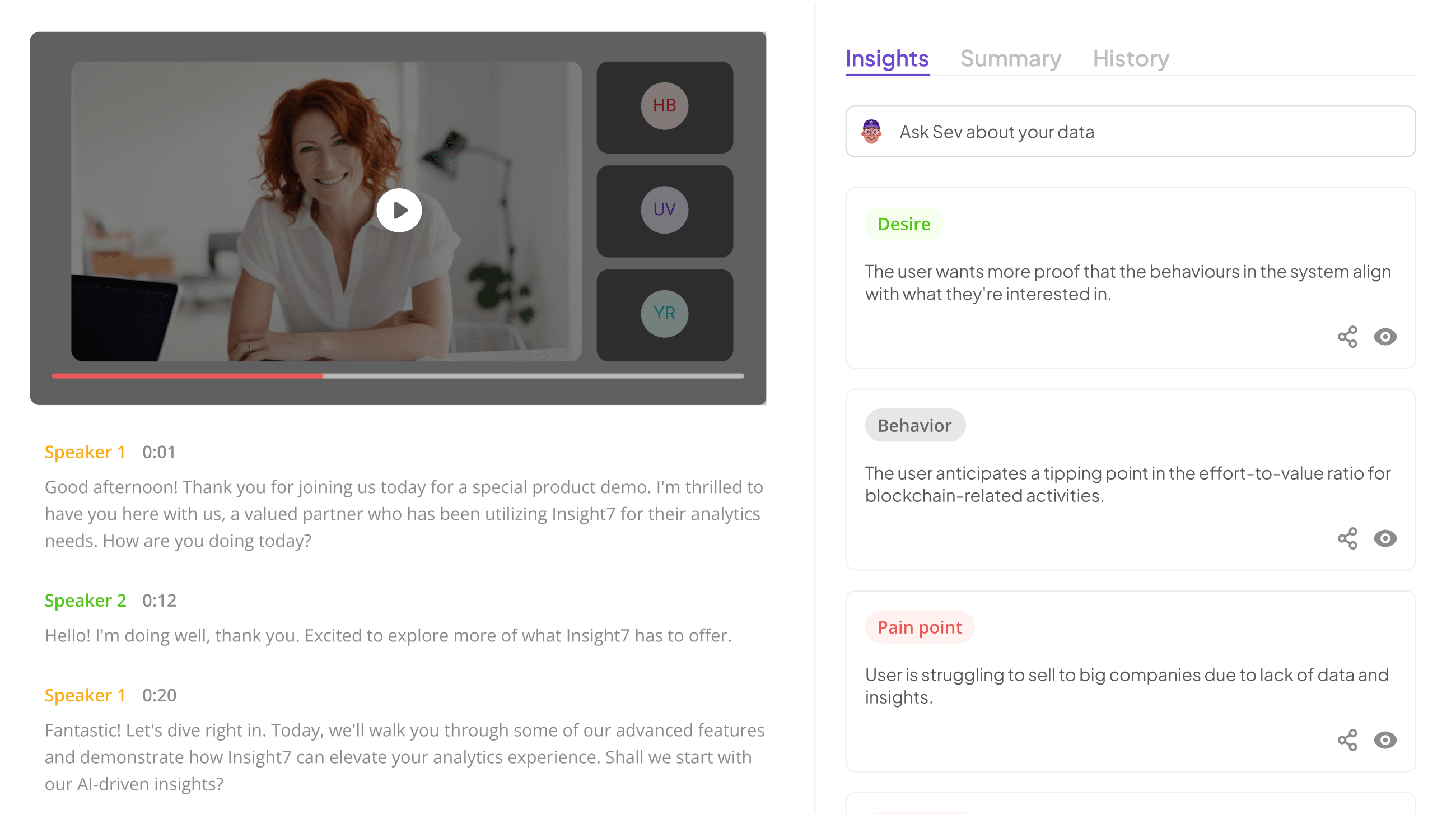
Understanding AI-Driven Feedback Forecasting
AI-Driven Feedback Forecasting represents a transformative approach in understanding customer sentiment and preferences. By harnessing advanced algorithms and machine learning, organizations can predict future customer reactions based on past feedback. This proactive method allows businesses to adjust their strategies dynamically, ensuring that they remain aligned with customer expectations.
The process begins with collecting comprehensive customer feedback data, which AI analyzes to identify patterns and trends. Generative AI agents enhance this functionality by delivering insights that can be linked to specific business metrics. As organizations increasingly adopt AI-Driven Feedback Forecasting, they can not only improve their customer engagement but also enhance their overall decision-making processes. By translating feedback into actionable strategies, companies foster trust and loyalty among their customer base, leading to sustained growth.
The Role of Generative AI Agents in Predictive Analysis
Generative AI agents play a significant role in predictive analysis, particularly in the realm of AI-driven feedback forecasting. These advanced systems process vast amounts of customer data, identifying patterns and trends that inform future strategies. By utilizing sophisticated algorithms, these agents enhance the accuracy of forecasts, allowing businesses to anticipate customer needs and preferences effectively.
To maximize the benefits of generative AI in predictive analysis, several key elements must be considered. First, specificity in data input is crucial; precise criteria lead to more reliable outcomes. Second, continuous evaluation of the AI's performance ensures it adapts to changing customer dynamics. Lastly, a collaborative approach, where teams refine AI insights together, builds a comprehensive understanding of the data landscape. This cohesive strategy not only improves decision-making but also drives innovative solutions tailored to customer feedback, ultimately enhancing business growth through effective AI-driven feedback forecasting.
How AI-Driven Feedback Forecasting Transforms Customer Insights
AI-Driven Feedback Forecasting is revolutionizing how businesses gather and interpret customer insights. By utilizing advanced algorithms and machine learning, organizations can process extensive feedback data more efficiently than traditional methods allow. This transformation enables businesses to unveil patterns and trends that were previously obscured, ensuring that valuable customer sentiments are captured and acted upon.
As competition intensifies, timely insights become critical to success. Companies equipped with AI-driven tools can swiftly identify emerging customer needs and adjust strategies accordingly. This proactive approach not only enhances customer experience but also fosters deeper engagement. By bridging the gap between customer feedback and actionable business strategies, AI-driven forecasting empowers organizations to stay ahead and make informed decisions based on real-time insights. Ultimately, it ensures that businesses are not just reacting to customer feedback but actively using it to shape their future.
Extract insights from interviews, calls, surveys and reviews for insights in minutes
Tools and Techniques for AI-Driven Feedback Forecasting
In the quest for improved customer understanding, tools and techniques play a vital role in AI-Driven Feedback Forecasting. These methods enable businesses to extract actionable insights from vast datasets, optimizing product development and enhancing customer experience. Integrating advanced AI tools allows organizations to efficiently analyze feedback, identify trends, and accurately predict future customer sentiments.
Several key tools enhance the capability of AI-Driven Feedback Forecasting. For instance, platforms like Insight7 and Qualtrics facilitate data collection and sentiment analysis. Additionally, IBM Watson and MonkeyLearn are instrumental in training generative models that generate insightful predictions. By leveraging these technologies, teams can implement a structured approach to forecasting, ensuring that their predictive analytics are based on reliable information. This structured method not only boosts accuracy but also refines the overall decision-making process based on customer feedback, ultimately driving business growth.
Key Tools for Predictive Customer Feedback Analysis
To effectively harness AI-Driven Feedback Forecasting, organizations must utilize several key tools in their predictive customer feedback analysis. These tools are designed to collect, analyze, and interpret a vast amount of customer feedback, transforming data into valuable insights. Tools such as Insight7 and MonkeyLearn empower businesses to effortlessly identify trends and patterns within customer responses. They allow teams to filter through positive and negative comments, enhancing the understanding of customer sentiment.
Furthermore, platforms like Qualtrics, IBM Watson, and Lexalytics facilitate more sophisticated analysis by utilizing machine learning algorithms. These technologies can help uncover nuances in customer feedback, providing a deeper understanding of user experiences. By adopting these tools, companies position themselves to predict future customer behaviors and preferences more accurately. Ultimately, the integration of these powerful resources not only streamlines the feedback analysis process but also enhances overall customer engagement strategies, enabling businesses to respond proactively to market needs.
- Insight7
In the realm of customer feedback, AI-Driven Feedback Forecasting plays a transformative role, reshaping how businesses extract insights from customer interactions. Generative AI agents analyze vast amounts of qualitative dataโlike interviews and surveysโproviding companies with a clearer understanding of customer sentiments and behaviors. The ability to process and interpret this information quickly empowers businesses to pivot strategies, enhancing both product offerings and customer experiences.
To implement effective AI-driven feedback strategies, organizations should consider three essential components: data collection, model training, and ongoing analysis. First, businesses need to gather high-quality data through well-structured interviews and surveys. Second, training generative AI models on this data is crucial for recognizing patterns and trends. Finally, continuous validation and refinement of predictions ensure that insights remain relevant and actionable. By mastering these components, companies will not only keep pace with customer needs but also outpace their competitors in the fast-evolving market landscape.
- MonkeyLearn
In the realm of predictive customer feedback analysis, one powerful tool stands out due to its versatility and ease of use. This platform empowers businesses to harness the potential of AI-driven feedback forecasting effectively. By taking advantage of advanced text analytics, it allows organizations to examine customer sentiments and opinions across various channels quickly. With its intuitive interface, teams can access insights that would otherwise require extensive manual analysis.
Moreover, this tool streamlines the feedback process, enabling organizations to respond more dynamically to customer needs. Implementing this technology facilitates the identification of trends and potential areas for improvement, reinforcing the value of customer relationships. As businesses incorporate AI-driven feedback forecasting into their strategies, they can make informed decisions, enhancing their overall customer experience. Adopting such tools is essential for companies looking to stay competitive in an ever-evolving market.
- Qualtrics
In the realm of predictive customer feedback analysis, effective tools play a crucial role in shaping strategies and enhancing decision-making. Qualtrics stands out as a comprehensive solution designed to facilitate AI-driven feedback forecasting. It enables organizations to seamlessly collect, analyze, and act on customer insights in real-time. This platform empowers businesses to transition from traditional survey methods to dynamic, AI-enabled approaches, thereby improving response accuracy and speed.
The functionality of Qualtrics extends beyond mere data gathering. It provides advanced analytics and reporting features, allowing teams to visualize trends and identify key areas for improvement. By integrating generative AI, it enhances the ability to predict customer sentiments and preferences. This capability is essential for businesses aiming to refine their offerings and align with market demands. Ultimately, utilizing Qualtrics in the context of AI-driven feedback forecasting can lead to more informed decisions and stronger customer relationships.
- IBM Watson
In the realm of AI-Driven Feedback Forecasting, advanced analytics is crucial for transforming customer insights into actionable strategies. Generative AI agents play a pivotal role by enabling businesses to interpret vast amounts of feedback effectively. These powerful tools can analyze sentiment and extract patterns from unstructured data, which enhances decision-making processes.
Utilizing sophisticated algorithms, businesses can identify trends in customer behavior and predict future feedback, allowing for timely adjustments to products or services. The strengths of AI-driven solutions include their capability to analyze large datasets efficiently and provide insights that may otherwise go unnoticed. By integrating these innovative technologies, organizations can enhance their understanding of customer sentiments and ultimately strengthen their market positioning.
With the right tools, companies can optimize their feedback loops and create more personalized customer experiences that drive loyalty and satisfaction.
๐ฌ Questions about Generative AI Agents for Predictive Customer Feedback Analysis?
Our team typically responds within minutes
- Lexalytics
In the landscape of predictive customer feedback analysis, specific tools stand out for their capabilities, including the one we are discussing now. This platform excels in providing user-friendly access to advanced analytics features. Its design encourages broad usability, allowing teams to explore insights without requiring specialized training. Users can easily input data, such as conversation transcripts, to uncover trends and pain points affecting customer experience.
Its core functionality is built around the extraction of meaningful insights from extensive datasets. Users can generate detailed reports while visually navigating through conversations and their related themes. By identifying customer behaviors and preferences, businesses can make informed decisions that enhance overall service. The platform's ability to analyze multiple calls simultaneously further amplifies the potential for AI-driven feedback forecasting, ensuring that organizations remain agile in responding to customer needs.
Implementing Forecasting Techniques: A Step-by-Step Guide
Implementing AI-Driven Feedback Forecasting involves a systematic approach to harnessing generative AI agents for predictive customer feedback analysis. Begin by identifying vital metrics you want to predict, such as customer satisfaction or product performance. Next, gather relevant data from various sources, ensuring it covers historical trends and current interactions to create a solid foundation.
Proceed by training your generative AI models on the collected data. Use algorithms that can recognize patterns and correlations within the feedback. Once the models are sufficiently trained, analyze and predict future feedback trends. Regularly validate these predictions against real-world outcomes to refine your models and enhance accuracy. This iterative process ensures that your forecasting improves over time, empowering your organization to make data-driven decisions that resonate with your customers.
Step 1: Data Collection and Preparation
Data Collection and Preparation is a critical initial step in AI-Driven Feedback Forecasting. During this phase, it is essential to gather diverse and relevant data sources that can illuminate customer sentiments and feedback. To achieve this, businesses should collect data from multiple channels, such as surveys, social media interactions, and customer service calls, ensuring a comprehensive view of customer opinions.
After gathering the data, the next task focuses on preparation. This involves cleaning the data to remove duplicates or irrelevant information, ensuring accuracy and reliability. Structured formats, such as CSV or JSON, can be utilized to facilitate seamless analysis. By thoroughly preparing the data, businesses can optimize the performance of AI models, leading to more accurate predictions and actionable insights in the future. Implementing these practices sets the foundation for effective feedback analysis and enhances decision-making capabilities.
Step 2: Training Generative AI Models
Training generative AI models is a foundational step in developing effective AI-driven feedback forecasting systems. This phase involves feeding the model with high-quality, diverse datasets to enable it to learn and recognize patterns in customer feedback. The specific characteristics of the data significantly influence the model's performance. Therefore, itโs crucial to ensure that the datasets are representative of various customer segments.
Once the data is curated, the model undergoes extensive training using machine learning techniques. This includes refining algorithms and adjusting parameters to enhance accuracy. Continuous evaluation and iteration are necessary, as the model must adapt to changing feedback trends. By monitoring performance, businesses can tailor the model to predict future customer sentiments effectively. Ultimately, well-trained generative AI models will empower organizations to gain richer insights, leading to more informed decision-making and significant growth.
Training generative AI models not only improves predictive capabilities but also helps in understanding customer needs on a deeper level. This commitment to excellence lays the groundwork for developing solutions that transform raw feedback into actionable insights for enhancing customer experience.
Step 3: Analyzing and Forecasting Feedback
Analyzing and forecasting feedback is a crucial step in maximizing customer insights. This phase often utilizes AI-driven techniques to mine invaluable data from customer interactions. By systematically assessing the patterns in feedback, organizations can forecast future trends, identify emerging customer needs, and address potential issues proactively.
To effectively conduct analyzing and forecasting feedback, consider the following key aspects:
Data Segmentation: Break down feedback into distinct categories, such as positive and negative comments. This helps in understanding customer sentiment across various touchpoints.
Trend Identification: Use AI algorithms to identify recurring themes and trends in the feedback. This can provide insights into evolving customer preferences and market dynamics.
Predictive Modeling: Employ machine learning models to predict potential future feedback scenarios based on historical data. This way, businesses can prepare for shifts in customer expectations.
Continuous Monitoring: Establish a routine for regularly updating the analysis to adapt to new insights. This is essential for staying relevant and responsive to customer needs.
Incorporating these strategies enhances AI-driven feedback forecasting, making it an essential component of customer analysis. By doing so, organizations can unlock actionable insights that ultimately lead to improved customer satisfaction and growth.
Step 4: Validating and Refining Predictions
In this crucial step, the focus shifts to validating and refining predictions generated through AI-driven feedback forecasting. It is imperative to ensure that the insights derived from customer feedback are not only accurate but also actionable. Start by reviewing the initial predictions against actual customer behaviors and sentiments. This review process helps identify any discrepancies, allowing for adjustments to be made to the model or data set.
Next, conduct further data analysis to refine the initial forecasts. This can include cross-referencing findings with multiple customer segments or geographic regions. By analyzing variations, businesses can tailor their strategies to better resonate with specific customer groups. Continual validation and refinement of predictions ultimately enhance the reliability of insights and strengthen decision-making processes. This iterative process ensures that AI-driven feedback forecasting remains a robust tool in understanding customer needs and driving business growth.
Conclusion: Harnessing AI-Driven Feedback Forecasting for Business Growth
To harness AI-Driven Feedback Forecasting for business growth, organizations must identify and integrate advanced analytical tools. By leveraging generative AI agents, businesses can delve into rich customer feedback data, allowing for insightful predictions and trend identification. This process enhances understanding of customer preferences, leading to more tailored products and services aligned with market needs.
Furthermore, effective implementation of AI-Driven Feedback Forecasting can streamline decision-making processes. By converting raw feedback into actionable insights, businesses position themselves for sustainable growth and improved customer satisfaction. As companies embrace this innovative approach, they unlock new opportunities for engaging with their target audience while fostering long-term loyalty and success.
๐ฌ Questions about Generative AI Agents for Predictive Customer Feedback Analysis?
Our team typically responds within minutes