Generate Abstract From Text Tools And Techniques
-
Hello Insight
- 10 min read
Text summarization methods play a crucial role in generating concise abstracts from extensive content. By streamlining information, these techniques enable readers to grasp essential ideas without navigating through lengthy materials. In various fields, the ability to condense information efficiently promotes better comprehension and knowledge transfer.
The primary methods of text summarization include extractive and abstractive approaches. Extractive summarization selects key phrases and sentences directly from the source material, effectively capturing the core concepts. Conversely, abstractive summarization takes a more creative route by generating new phrases that encapsulate the original text’s essence. Such diverse methodologies empower users to choose suitable techniques based on their specific needs and contexts.
Analyze & Evaluate Calls. At Scale.
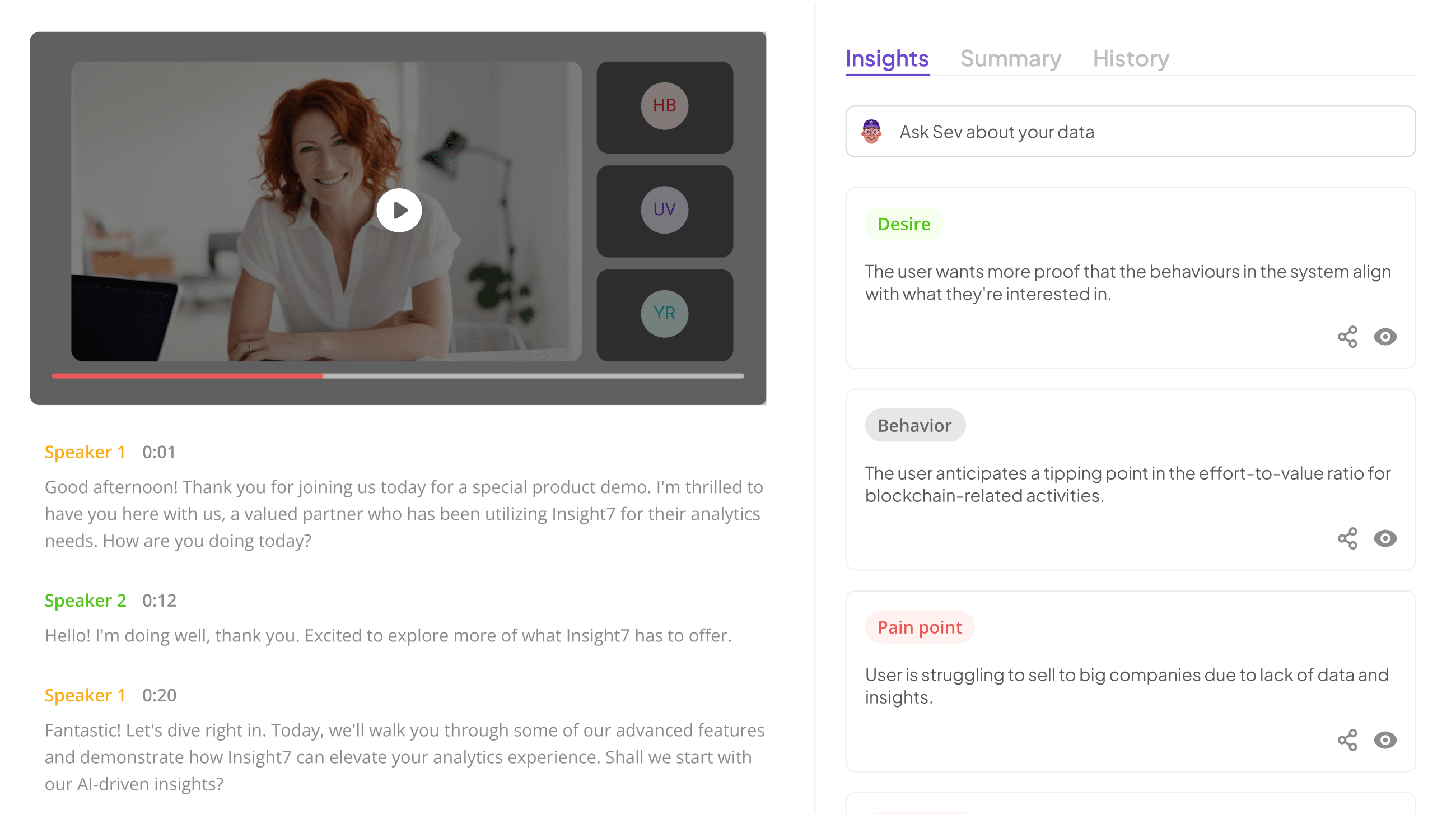
Understanding Text Summarization Methods
Understanding Text Summarization Methods is essential for anyone seeking to generate concise abstracts effectively. There are two primary types of methods within this field. Extractive summarization focuses on selecting sentences and phrases from the original text. This method preserves the original wording of the source material while distilling it to its essential points. Conversely, abstractive summarization generates new sentences that capture the core ideas, often resulting in a more fluid and coherent summary that may rephrase the original content significantly.
Each method offers distinct advantages depending on the context of use. Extractive summarization is particularly useful for maintaining the integrity of the source material, while abstractive summarization leverages creativity in phrasing, yielding a more reader-friendly output. Understanding the differences between these Text Summarization Methods can help users choose the best approach for their specific needs in generating high-quality abstracts.
Types of Text Summarization Methods
Text summarization methods can be categorized primarily into two main types, each serving distinct purposes. Extractive summarization involves selecting key phrases and sentences directly from the original text. This method retains the most relevant portions of the content while ensuring cohesion, making it easy for readers to grasp critical information without altering the original wording. It is particularly useful when speed and efficiency are paramount.
On the other hand, abstractive summarization generates new phrases that capture the essence of the original text. This technique requires a deeper understanding of the material and typically results in more concise and coherent summaries. By reformulating the content, it can present insights in a more engaging way. Both types of text summarization methods offer unique benefits depending on the context in which they are applied, enhancing the reader's experience and making information digestible.
- Extractive Summarization: Selecting key phrases and sentences from text.
Extractive summarization is a valuable method for identifying and selecting key phrases and sentences from text. This approach focuses on pulling out essential content without altering the original meaning. It thrives in scenarios where clarity and directness are paramount, as it presents information in a structured and coherent way. By utilizing algorithms that recognize context and importance, extractive summarization can effectively distill large volumes of text into concise summaries.
One primary method involves scoring sentences based on their significance within the overall text. Sentences that score above a certain threshold are then selected for inclusion in the summary. Additionally, techniques such as frequency analysis and semantic similarity can enhance the process, ensuring the chosen phrases accurately reflect the main ideas. By applying these methods, users can efficiently synthesize large texts into manageable segments, fostering better understanding and retention of important information.
- Abstractive Summarization: Generating new phrases to represent the content.
Abstractive summarization is an innovative approach to text summarization methods, focusing on generating new phrases that encapsulate the core ideas of the original content. Unlike extractive summarization, which simply selects existing phrases, abstractive summarization requires understanding the material deeply to create concise yet coherent representations. This technique can generate summaries that express the original thoughts in novel ways, providing fresh insights and enhancing readability.
To achieve effective abstractive summarization, several key techniques are employed. Firstly, preprocessing the text involves cleaning and tokenizing the content, setting the stage for meaningful analysis. Next, various summarization algorithms are applied, allowing for the exploration of both extractive and abstractive methods. Experimentation is crucial, as fine-tuning these algorithms helps refine the generated phrases, ensuring they accurately reflect the essence of the original text. By embracing these techniques, content creators can facilitate better comprehension and retention of information while navigating complex subjects.
Benefits of Using Text Summarization Methods
Text Summarization Methods offer a wide array of advantages, particularly in today’s fast-paced information age. One key benefit is time efficiency; they help users quickly grasp core ideas without sifting through lengthy documents. This flexibility is essential for professionals who rely on concise information to make informed decisions. By employing these methods, users can allocate more time to critical tasks rather than reading extensive texts.
Furthermore, these summarization techniques aid in managing information overload. In an era saturated with data, focusing on essential information becomes increasingly vital. Text Summarization Methods streamline this process, allowing users to concentrate on what truly matters. In addition, by highlighting significant themes and insights, these methods enhance understanding and retention. Ultimately, utilizing text summarization leads to a more productive and informed approach to information consumption.
- Time Efficiency: Reducing time spent on reading.
Reading can often consume significant time, especially in an era where information is readily available and plentiful. Text Summarization Methods provide efficient solutions to this problem, enabling individuals to grasp essential content quickly. By employing techniques such as extractive and abstractive summarization, readers can navigate vast volumes of text with ease, extracting only the most critical insights.
For instance, extractive summarization identifies and distills key sentences from a text, while abstractive summarization generates new phrases that encapsulate the original meaning. These methods can dramatically reduce the time spent sifting through pages of information, allowing readers to focus on what's truly important. Ultimately, utilizing these techniques promotes greater comprehension and retention of information, making the reading experience more productive. Implementing effective Text Summarization Methods not only enhances understanding but also fosters a more efficient approach to consuming written content.
- Information Overload Management: Focusing on essential information only.
Managing information overload involves honing in on essential data that drives decision-making and enhances productivity. In today's fast-paced world, individuals often find themselves inundated with information, which can lead to confusion and inefficiency. By implementing effective text summarization methods, one can distill vast amounts of data into comprehensible and actionable insights.
To tackle information overload, it is vital to prioritize key elements within the textual content. First, identify the core messages that align with your objectives. Next, utilize extractive summarization to pinpoint significant phrases and sentences without losing context. Finally, consider employing abstractive summarization, which allows for the generation of new phrases that convey the same essentials. These strategies not only streamline our reading process but also facilitate better comprehension, ultimately making knowledge acquisition more efficient.
Tools and Techniques for Generating Abstracts
Generating abstracts from extensive textual materials is crucial in various fields, enabling readers to grasp core ideas quickly. To facilitate this process, different tools and techniques come into play, employing diverse text summarization methods tailored to specific user needs. These methods can be categorized into two primary approaches: extractive and abstractive summarization. Extractive summarization focuses on selecting existing key phrases or sentences, while abstractive summarization generates new sentences to convey the original content's essence.
Several effective tools support users in creating concise abstracts. For instance, tools like AutoSummarizer and Resoomer allow for quick text condensing, catering to diverse summarization requirements. Additionally, employing systematic techniques is essential, starting with preprocessing the text by removing unnecessary elements and following up with the appropriate summarization algorithms. Each chosen method will maximize the effectiveness of the generated abstracts and enhance the user's ability to communicate complex information succinctly.
Extract insights from interviews, calls, surveys and reviews for insights in minutes
Top Tools for Abstraction
In the realm of text summarization, various tools stand out for their ability to distill complex information into concise abstracts. These platforms cater to different needs while enhancing understanding and productivity, making them essential for anyone needing efficient abstraction techniques. Notable tools include insight7, which allows users to efficiently analyze conversations and extract insights, ensuring users can focus on critical details without extensive training.
Another valuable tool is AutoSummarizer, which generates quick summaries with minimal user input. For those seeking flexibility, Resoomer offers both online and offline text summarization capabilities. Alternatively, Sumy supports various summarization techniques, appealing to diverse user preferences. Lastly, SMMRY excels at simplifying text, thereby allowing users to focus on the main ideas. By leveraging such tools, individuals can significantly enhance their capability to manage information overload through effective text summarization methods.
- insight7: A versatile tool for effective abstraction.
insight7 stands out as a versatile tool for effective abstraction, catering to various user needs. It streamlines the process of text summarization, allowing anyone from a novice to an expert to generate concise summaries effortlessly. Whether you need to condense lengthy reports or summarize user feedback, this platform simplifies complexity. Its user-friendly interface facilitates quick access to summaries derived from extensive datasets.
The tool supports multiple methods of text summarization, focusing on extractive and abstractive techniques. Users can pull key phrases directly from the source or generate new, coherent summaries that capture the essence of the text. With insight7, you can manage information overload efficiently, extracting meaningful insights that save time and enhance decision-making capabilities. The platform not only democratizes data but also empowers users to derive actionable insights without requiring extensive training or expertise, making it an essential resource in today’s fast-paced environment.
- AutoSummarizer: Provides quick summaries with minimal input.
AutoSummarizer is designed to enable users to generate quick summaries with minimal input efficiently. This tool operates under the principles of text summarization methods, primarily focusing on extracting key information while preserving the essence of the original content. Users simply input their text, and the AutoSummarizer swiftly identifies the most relevant points, allowing for effortless comprehension without lengthy reading sessions.
💬 Questions about Generate Abstract From Text Tools And Techniques?
Our team typically responds within minutes
The simplicity and effectiveness of the AutoSummarizer make it accessible for various users, regardless of their expertise level. This tool provides a practical solution for managing information overload and aids quick decision-making by offering concise summaries. Moreover, its ease of use democratizes data insights, empowering anyone in a professional setting to obtain valuable information quickly, thus enhancing overall productivity and focus on essential tasks. With AutoSummarizer, the challenge of sifting through extensive documents becomes a streamlined process, making vital insights readily available for effective communication and execution.
- Resoomer: Offers both online and offline text summarization.
Text summarization using tools like Resoomer enables users to condense large amounts of information efficiently. With its functionality for both online and offline summarization, it caters to a broad audience looking for flexible solutions. Users can easily input textual content and receive concise summaries that capture the essence of the original material.
The platform supports various text summarization methods, allowing users to select between extricating key information or generating new phrases. This adaptability is particularly beneficial in a fast-paced environment where managing vast amounts of information is crucial. In addition, Resoomer’s user-friendly interface ensures that individuals from different backgrounds, regardless of technical expertise, can utilize its summarization capabilities effectively. Ultimately, this tool stands out in the landscape of abstract generation, proving valuable for students, professionals, and anyone needing to distill complex texts into digestible formats.
- Sumy: Flexible tool supporting multiple summarization techniques.
Sumy is a highly flexible tool, designed to support various text summarization methods that cater to diverse user needs. It simplifies the complex process of generating abstracts, allowing users to choose between extractive and abstractive summarization techniques. By leveraging such options, individuals can extract essential information efficiently or create entirely new phrases that encapsulate the content meaningfully. This adaptability makes Sumy a valuable resource, especially in a surging information age where clarity and brevity are vital.
Through its user-friendly interface, Sumy democratizes the process of summarization. Whether you are analyzing a few documents or processing a large collection of texts, the tool ensures accessibility for all users, regardless of their technical background. With features that allow for effortless extraction of key insights and themes, Sumy transforms the way users engage with detailed text, ultimately supporting improved productivity and understanding.
- SMMRY: Simplifies text to help focus on main ideas.
SMMRY serves as a valuable tool for simplifying text and honing in on main ideas. With the rise of information overload, summarization methods have become essential for distilling content down to its essence. By using SMMRY, users can easily generate succinct abstracts that capture core concepts, thus saving time and enhancing comprehension. This tool also accommodates various types of content, whether it's articles, reports, or academic papers, and allows for customization based on user needs.
The process of simplification employed by SMMRY involves analyzing and extracting significant sentences while maintaining clarity and coherence. Users are empowered to quickly grasp the primary messages without wading through excessive details. As a result, SMMRY exemplifies effective text summarization methods by fostering efficiency and focus in diverse reading scenarios, thus aligning perfectly with today’s fast-paced information landscape.
Effective Techniques in Abstract Generation
To achieve effective abstract generation, various techniques are instrumental in streamlining text summarization methods. First, preprocessing the text is crucial. This step involves cleaning the data by removing stop words and tokenizing sentences to enhance clarity and focus. By preparing the text adequately, the summarization algorithms can operate more effectively, producing concise abstracts.
Once preprocessing is completed, the next technique involves applying appropriate summarization algorithms. Depending on the requirements, one can choose between extractive and abstractive methods. Extractive summarization extracts key phrases and sentences directly from the original text, while abstractive summarization generates new content to convey the same ideas. Testing different algorithms allows for better evaluation of their performance and adaptability to specific contexts. Employing these strategies ensures that abstracts contain relevant information, thus delivering value and clarity.
- Step 1: Preprocessing the Text
Preprocessing the text is a crucial step in the journey toward effective abstraction. It ensures that the selected content is clean and relevant for further analysis. This stage typically involves removing unnecessary or irrelevant data, such as stop words and punctuation. By eliminating these elements, the text becomes more focused, allowing for a clearer understanding of its essential components.
Another vital aspect of preprocessing is tokenization, which breaks down the text into smaller, manageable pieces known as tokens. This process helps in analyzing the text at a granular level, facilitating the identification of key phrases and topics. Overall, thorough preprocessing significantly enhances the performance and accuracy of subsequent Text Summarization Methods. Clean and organized data leads to better insights, enabling a more effective summarization process. Therefore, dedicating time to preprocessing ultimately pays off in the quest for efficient abstract generation.
- Removing stop words and irrelevant data.
In the process of generating abstracts, removing stop words and irrelevant data is crucial for effective text summarization methods. Stop words—common words like "and," "the," or "is"—add little meaning and often clutter the text. By eliminating them, we can focus on the critical content that drives the core message. This cleanup helps algorithms concentrate on more meaningful words, thereby enhancing the summarization quality.
Moreover, irrelevant data can hinder the clarity of the main ideas. It is essential to assess the text and identify portions that do not contribute to the primary narrative. This includes repetitive phrases, unnecessary adjectives, or tangential information. By filtering out these elements, the summarization process becomes more streamlined. This approach not only optimizes algorithm performance but also ensures that the final abstract conveys the essence of the original text succinctly.
- Tokenization of the text.
Tokenization is a fundamental step in the text summarization process, serving as the bridge between raw text and meaningful summaries. It involves breaking down the text into smaller, manageable units, typically words or sentences. This process not only provides a clear structure but also allows for the efficient analysis of content, which is crucial for meaningful text representation.
The effectiveness of tokenization directly impacts the quality of summarization techniques, as it aids in identifying key phrases and concepts. Furthermore, accurate tokenization enhances the subsequent phases of text summarization, whether that be extractive methods that pull existing sentences or abstractive methods that paraphrase content. Understanding proper tokenization methods ensures a smoother transition to summarization, revealing the essential points of the text while stripping away unnecessary clutter.
- Step 2: Applying Summarization Algorithms
In this crucial step of applying summarization algorithms, the focus shifts to selecting and implementing appropriate text summarization methods to achieve desired outcomes. Various summarization algorithms cater to different needs, which means understanding each type is essential. For instance, extractive methods emphasize capturing important sentences or phrases from the original text, while abstractive methods focus on generating new content that encapsulates the main ideas.
To effectively apply these algorithms, it’s important to start by assessing the specific requirements of your project. Consider the volume of data and the complexity of information when deciding between extractive or abstractive summarization. Testing both methods on sample data can help illustrate their strengths and weaknesses in different scenarios. Ideally, this testing phase leads to selecting the most effective approach that optimally reduces information overload while retaining the core insights. An iterative approach can refine outcomes and enhance overall comprehension from the summarized content.
- Selecting suitable algorithms based on needs.
Choosing the right algorithm for text summarization methods is crucial, as the selected approach significantly impacts the quality of the generated abstract. First, it's essential to understand your specific needs and objectives. For instance, if your goal is to condense the information while maintaining original phrasing, extractive summarization may be the best choice. This method picks key sentences and phrases directly from the text. Alternatively, if you desire a more innovative summary that captures the essence with rephrased content, consider employing abstractive summarization techniques.
When selecting algorithms, consider the complexity of the text and the desired output. Some algorithms excel in handling technical documents, while others may suit general content better. Conducting tests on both extractive and abstractive methods will help identify which technique aligns best with your project demands. Ultimately, understanding the nuances of these text summarization methods empowers you to create more effective and precise abstracts tailored to your needs.
- Testing extractive vs. abstractive methods.
Testing extractive and abstractive methods can significantly influence how well text summarization methods are applied. In extractive summarization, key phrases and sentences are selected directly from the source material. This approach maintains the original wording and structure, providing a straightforward representation of the text. On the other hand, abstractive summarization involves generating new phrases that capture the essence of the content. This method can lead to more coherent summaries but often requires advanced algorithms and deeper understanding of the content.
Ultimately, the choice between these two approaches depends on the specific needs of the task. For instance, if accuracy and fidelity to the original text are paramount, extractive methods may be preferable. Conversely, when a more nuanced understanding and interpretation are desired, abstractive methods shine. Understanding the strengths and weaknesses of both methods is crucial for choosing the most effective text summarization techniques for any given application.
Conclusion on Utilizing Text Summarization Methods
Utilizing text summarization methods can significantly enhance the process of generating concise abstracts from extensive textual data. These methods, whether extractive or abstractive, streamline the way information is distillated, making large sets of data more manageable and accessible. By applying effective summarization techniques, users are empowered to focus on core ideas, promoting better understanding and retention of key information.
Moreover, the advantages of these methods should not be overlooked. They not only reduce the time required for information digestion but also mitigate the challenges posed by information overload. Embracing text summarization methods can lead to more efficient workflows and improved productivity across various domains, making them essential for anyone looking to work smarter with content.
💬 Questions about Generate Abstract From Text Tools And Techniques?
Our team typically responds within minutes