Forecasting Churn Risk Using AI and Call Transcripts
-
Bella Williams
- 10 min read
Churn Prediction AI offers a transformative approach for businesses aiming to minimize customer attrition. By analyzing call transcripts, organizations can uncover valuable insights into customer sentiment, satisfaction, and potential churn indicators. These transcripts provide a rich resource for understanding customer concerns and expectations, allowing companies to respond proactively.
Incorporating Churn Prediction AI enables businesses to accurately forecast customer retention risks through detailed analysis of conversational data. By systematically extracting key themes and pain points from call transcripts, businesses can harness this intelligence to develop more effective retention strategies. Understanding customer needs and addressing them timely is crucial for sustaining customer loyalty and driving long-term success.
Analyze & Evaluate Calls. At Scale.
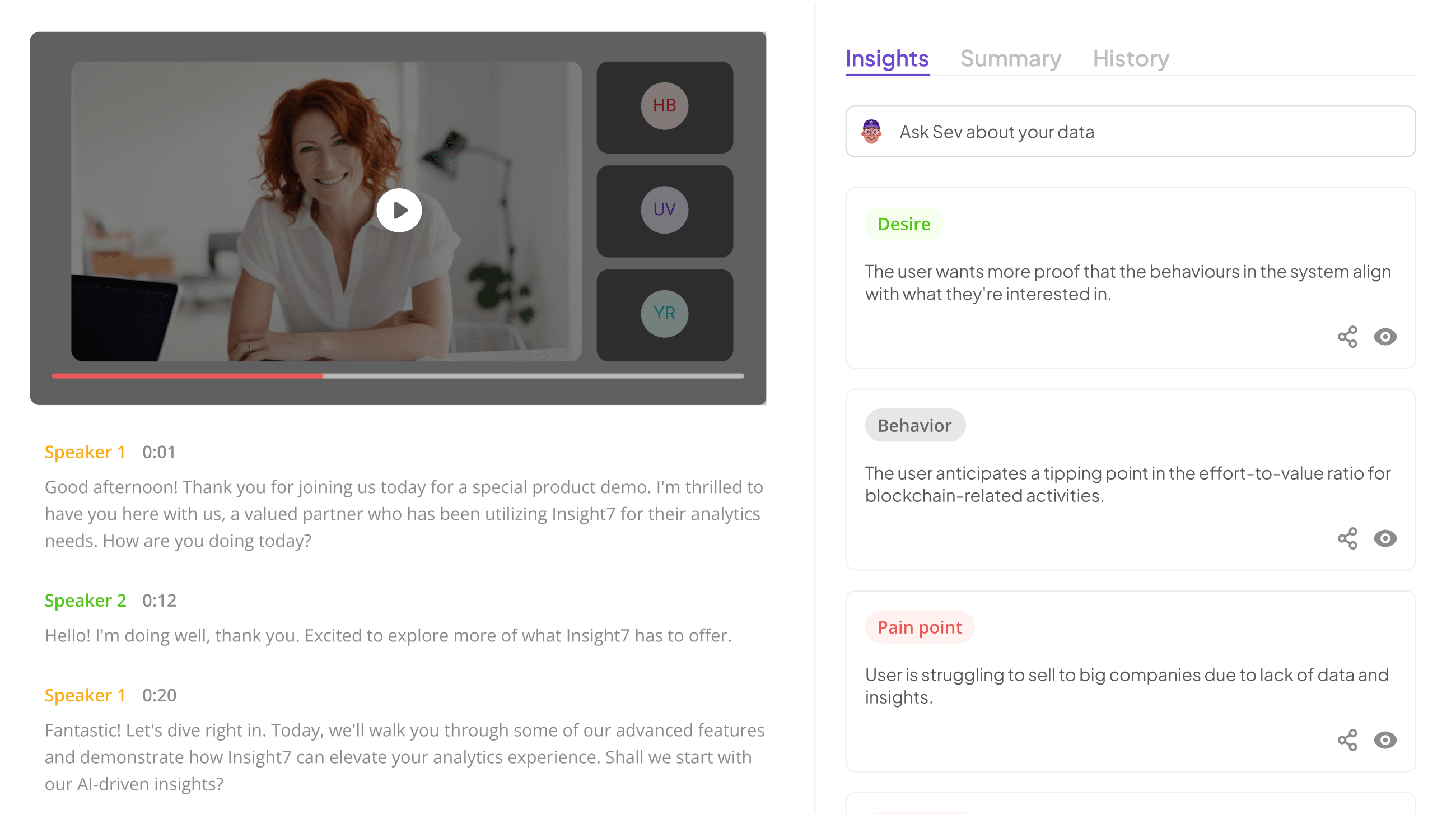
Understanding Churn Prediction AI: Key Concepts and Importance
Churn Prediction AI plays a pivotal role in understanding customer retention and identifying potential churn risks. By analyzing call transcripts, businesses can uncover insights hidden within customer conversations. This process helps in identifying patterns, such as frequent pain points mentioned by customers and overall sentiment towards the brand. Each call carries valuable data, and when aggregated, it helps create a comprehensive view of customer attitudes and behaviors.
The importance of Churn Prediction AI lies in its ability to forecast customer attrition before it occurs. Businesses equipped with these insights can proactively address issues, improve customer satisfaction, and tailor their services to meet evolving needs. This not only enhances customer loyalty but also optimizes resources, as companies can focus on targeted strategies that yield the highest return on investment. Embracing Churn Prediction AI can ultimately lead to a healthier, more profitable customer relationship.
The Role of AI in Churn Prediction
AI plays a crucial role in churn prediction, providing businesses with advanced tools to analyze customer interactions effectively. By processing and interpreting call transcripts, Churn Prediction AI can identify patterns and sentiments that might indicate a risk of churn. This technology uses natural language processing to gauge customer satisfaction and detect potential issues early, allowing for timely interventions.
Moreover, the deep learning capabilities of AI help organizations develop more accurate predictive models. These models not only highlight which customers are most likely to leave but also uncover the underlying reasons behind their dissatisfaction. This insight enables businesses to tailor their strategies effectively, focusing on retention efforts where they are needed most. As the landscape of customer engagement continues to evolve, embracing AI for churn prediction is becoming increasingly essential for sustained growth and customer loyalty.
Key Benefits of Using Churn Prediction AI in Business
Churn Prediction AI offers invaluable insights that empower businesses to enhance customer retention strategies effectively. By accurately analyzing customer interactions, companies can identify warning signs of dissatisfaction and disengagement. This proactive approach allows businesses to tailor their offerings based on real-time feedback, improving customer satisfaction. Additionally, leveraging AI in this context streamlines the analysis process, enabling teams to derive actionable insights from call transcripts without requiring extensive expertise.
One significant advantage is the ability to segment customers based on their behaviors and sentiment expressed during conversations. This segmentation facilitates personalized communication and customized solutions, ultimately strengthening customer loyalty. Furthermore, integrating Churn Prediction AI into existing systems can lead to more informed decision-making aligned with strategic goals. As a result, businesses not only reduce churn rates but also foster a culture of continuous improvement based on customer insights. Embracing this technology is essential for companies striving to sustain competitive advantage in todayโs dynamic market.
Extract insights from interviews, calls, surveys and reviews for insights in minutes
Implementing Churn Prediction AI with Call Transcripts
Implementing Churn Prediction AI with call transcripts begins with a systematic approach to understanding customer interactions. Initially, organizations should focus on collecting and preparing call transcripts. This involves obtaining raw audio recordings and converting them into text format for easier analysis. Capture all relevant conversations consistently to ensure comprehensive data collection.
Once transcripts are ready, the next step is leveraging AI tools to analyze the content. This analysis can identify sentiment shifts and key topics that may indicate dissatisfaction or potential churn. By extracting these insights, businesses can build predictive models that assess churn risk factors based on real customer feedback. Integration of these findings into operational strategies can proactively address issues before they escalate.
Top tools such as CallMiner and VoiceBase can facilitate these processes, making it simpler to derive actionable insights from call transcripts and enhance Churn Prediction AI capabilities.
Step-by-Step Process for Analyzing Call Transcripts
The process of analyzing call transcripts to predict churn can significantly enhance your business's understanding of customer behavior. Initially, gather a comprehensive dataset by transcribing all relevant call recordings. Utilize bulk transcription tools to convert audio files into text swiftly, ensuring that all customer interactions are captured accurately for analysis. This foundational step is crucial, as it sets the stage for deeper examination.
Next, apply AI-driven tools to examine the transcripts for sentiment and key themes. Focus on identifying pain points, satisfaction levels, and recurring questions or issues raised by customers. By categorizing this information, you can gain valuable insights into customer experiences and potential red flags. Finally, integrate your findings into predictive models aimed at forecasting churn risk. This detailed analysis not only informs retention strategies but also empowers you to address concerns proactively, improving overall customer satisfaction.
Step 1: Collecting and Preparing Call Transcripts
To initiate the process of churn prediction AI effectively, start by collecting and preparing call transcripts. Capturing valuable customer interactions is crucial in understanding their needs and concerns. Begin by obtaining audio recordings of customer calls, as these often provide rich insights into customer sentiment and behavior. Use transcription tools to convert these audio files into text, ensuring accuracy in reflecting the conversations.
Once you have your transcripts, organizing them is essential. Create a centralized library that allows easy access and management of transcripts. This structure will enable efficient analysis of conversations and help identify patterns or pain points indicative of potential churn risks. Analyzing these transcripts thoroughly will set a strong foundation for leveraging AI tools. This first step paves the way for deeper insights, ultimately enhancing your ability to predict customer churn accurately and improve retention strategies.
Step 2: Leveraging AI to Analyze Sentiment and Keywords
To effectively analyze sentiment and keywords in call transcripts, utilizing AI provides invaluable insights for churn prediction. This technology allows businesses to sift through vast amounts of conversation data and identify emotional undertones in customer interactions. By leveraging natural language processing, organizations can discern whether customer sentiments lean positive or negative.
AI-powered tools can extract critical keywords that reveal recurring concerns or praises. For instance, if multiple customers highlight a specific feature positively, it signals satisfaction, while frequent negative mentions could indicate potential churn triggers. Building a robust sentiment analysis framework helps prioritize actionable insights. Utilizing these findings allows businesses to enhance customer experiences and proactively address issues, ultimately reducing the risk of churn. Embracing AI for sentiment and keyword analysis positions companies to make data-driven decisions that foster long-term customer loyalty.
๐ฌ Questions about Forecasting Churn Risk Using AI and Call Transcripts?
Our team typically responds within minutes
Step 3: Integrating Findings into Predictive Models
In Step 3, integrating findings into predictive models is a crucial phase in utilizing Churn Prediction AI effectively. This stage involves translating insights derived from call transcripts into structured data that predictive algorithms can recognize and analyze. By identifying trends and common pain points, businesses can model potential churn scenarios more accurately.
To integrate these findings, consider a structured approach. First, categorize key insights into themes, such as identifying frequently mentioned issues or customer sentiment. Next, translate these themes into quantifiable metrics that predictive models can process. By doing this, organizations can enhance their ability to project churn risks and devise strategies to mitigate them.
Finally, itโs essential to continuously refine your models with incoming data, ensuring predictions remain relevant and actionable. This iterative process of integrating findings into predictive models ensures businesses stay ahead of churn risks and understand their customers better.
Top Tools for AI-Driven Churn Prediction in Call Transcripts
To effectively predict churn using call transcripts, organizations can utilize several advanced tools specifically designed for this purpose. The first tool, insight7, streamlines the entire process by enabling users to transcribe multiple call files simultaneously. Utilizing its intuitive interface, businesses can quickly derive insights from conversations by selecting specific templates that focus on customer pain points or sentiments.
Following on, MonkeyLearn employs machine learning to enhance text analysis, allowing companies to discern patterns in customer interactions. CallMiner offers comprehensive analysis of customer calls with features that track sentiment and speech patterns, making it easier to identify at-risk customers. Next, VoiceBase excels in voice recognition and serves up detailed analytics on call dynamics, promoting informed decisions. Lastly, Speechmatics provides robust transcription services with multilingual support, ensuring organizations can capture valuable insights across diverse customer bases. These tools collectively empower effective churn prediction through the analysis of call transcripts.
insight7
The application of churn prediction AI in analyzing call transcripts offers businesses a significant opportunity to enhance customer retention strategies. By employing advanced analytics, companies can identify patterns and signals in customer conversations that signal potential churn. Understanding these insights allows organizations to proactively address customer concerns and needs, fostering a stronger relationship with their clientele.
In this process, businesses should prioritize data collection and preparation, ensuring transcripts are accurate and relevant. Next, leveraging AI technologies enables teams to extract sentiment and keyword insights that correlate with customer satisfaction. Finally, integrating these findings into predictive models equips organizations with the foresight necessary to implement targeted interventions. This systematic approach not only reduces churn risk but also drives long-term customer loyalty, ultimately enhancing overall business performance.
MonkeyLearn
The platform in focus provides user-friendly tools designed for analyzing call transcripts, making it accessible to all team members. By democratizing insights, it allows anyone in the organization to extract valuable information without requiring specialized training. This approach simplifies the process of transcribing calls and gathering data to assess customer experiences. For instance, users can upload call files and generate reports effortlessly, which is particularly beneficial for business reviews.
The intuitive interface enables users to visualize conversations, pinpoint pain points, and identify customer desires easily. By analyzing multiple calls within projects, teams can compile data at scale, enhancing their understanding of customer behavior. These insights support effective churn risk mitigation strategies, ensuring businesses can proactively address issues that may lead to customer attrition. Ultimately, this platform empowers organizations to harness their call data for better decision-making and improved customer retention strategies.
CallMiner
CallMiner provides essential tools for understanding customer interactions through AI-powered analysis. By harnessing advanced algorithms, it scrutinizes call transcripts to identify patterns associated with customer churn. This process enables businesses to anticipate potential churn risks, allowing for timely interventions. Moreover, the platformโs ability to filter and assess vast volumes of calls simplifies the data analysis process significantly.
Utilizing Churn Prediction AI, organizations can prioritize calls based on compliance or sales indicators. By focusing on key phrases and sentiments expressed during calls, businesses can develop insights that guide training for staff. This proactive approach not only improves customer satisfaction but also enhances retention strategies. In today's competitive landscape, understanding when customers may be at risk of leaving is crucial, and advanced tools like this are vital in supporting those efforts.
VoiceBase
VoiceBase excels in transforming raw call data into actionable insights, making it an essential tool for businesses seeking to reduce churn risk. By utilizing advanced AI capabilities, it analyzes call transcripts with remarkable efficiency, allowing users to identify critical customer sentiments and trends. This process starts with bulk transcription, enabling users to upload multiple audio files simultaneously.
Once transcribed, businesses can easily visualize individual calls along with their insights, helping to pinpoint customer pain points and satisfaction levels. The platformโs ability to extract specific insights with just one click simplifies the analysis process tremendously. Users can filter through insights to better understand customer experiences, thus enabling proactive measures to mitigate churn. Such capabilities make VoiceBase a crucial ally in developing robust churn prediction strategies that drive business growth through enhanced customer loyalty.
Speechmatics
The integration of advanced technologies has made it increasingly possible to improve customer engagement and retention. Powerful AI tools can extract valuable insights from call transcripts, enhancing the ability to predict customer churn effectively. One of the prominent solutions in this arena focuses on transcribing and analyzing spoken words to derive actionable insights. This technology employs speech recognition algorithms to transform conversations into text format, enabling businesses to assess customer sentiment and identify potential pain points.
Understanding these insights is crucial for churn prediction AI. The ability to analyze transcripts allows organizations to identify trends and common issues faced by customers. This proactive approach not only aids in addressing immediate concerns but also facilitates strategic decision-making for long-term engagement. By harnessing the power of speech technology, companies can effectively mitigate churn risk and foster a more responsive customer experience.
Conclusion: Unlocking Business Potential with Churn Prediction AI and Call Transcripts
Integrating Churn Prediction AI with call transcripts is an essential step in transforming customer interaction into actionable insights. By analyzing recorded conversations, businesses can uncover underlying patterns and factors contributing to churn risk. This proactive approach enables companies to identify at-risk customers and craft tailored retention strategies, ultimately driving increased customer loyalty and satisfaction.
Moreover, the potential of Churn Prediction AI extends beyond merely understanding current trends. Utilizing this technology fosters a data-driven culture, enhancing decision-making processes across the organization. As businesses unlock the full potential of their customer interactions, they position themselves favorably within the market, ensuring sustainable growth and profitability.
๐ฌ Questions about Forecasting Churn Risk Using AI and Call Transcripts?
Our team typically responds within minutes