AI Research Ethics is becoming increasingly essential in the realm of decision-making, where algorithms and automated systems shape critical outcomes. As artificial intelligence continues to infiltrate research practices, the ethical implications of these technologies warrant careful examination. Researchers must grapple with questions of fairness, bias, and transparency, ensuring that their methodologies do not perpetuate existing inequalities.
In this section, we will explore the fundamental concepts of AI Research Ethics, emphasizing its importance in crafting ethical decision-making frameworks. By addressing the ethical challenges posed by AI, we can foster a more responsible approach to research that prioritizes integrity and accountability. Understanding the ethical landscape helps researchers navigate complex situations, ultimately contributing to more trustworthy outcomes in their work.
Analyze & Evaluate Calls. At Scale.
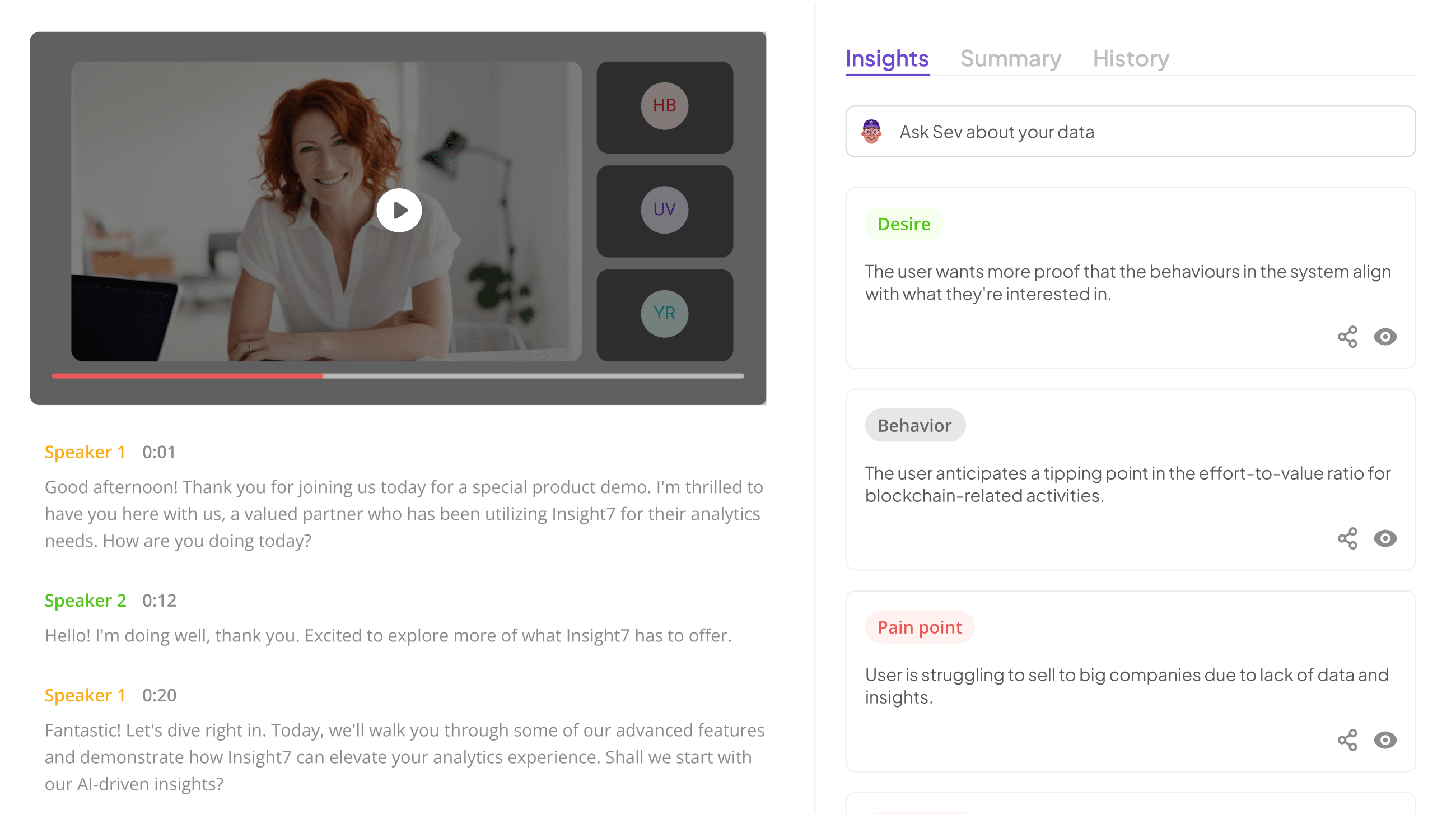
The Role of AI in Research Ethics
Artificial Intelligence (AI) profoundly influences ethical considerations in research, particularly concerning decision-making. In the context of AI Research Ethics, the technology must serve as a tool for enhancing, rather than undermining, ethical norms. Researchers are tasked with ensuring that AI systems maintain fairness and transparency, crucial aspects that hold significant implications for study outcomes.
To uphold these ethical standards, it is essential to address the potential biases that may arise from AI algorithms. Recognizing these biases allows researchers to implement measures that promote fairness and accountability. Furthermore, ongoing monitoring is vital to assess whether AI continues to align with ethical standards throughout its application. By integrating these practices into the research framework, the role of AI in research ethics can evolve to produce more reliable and ethical outcomes in decision-making.
Understanding AI Research Ethics: Key Concepts
AI Research Ethics plays a vital role in shaping responsible decision-making in research contexts. It encompasses a series of principles designed to guide how AI technologies should be used, ensuring that the outcomes are fair, transparent, and accountable. Understanding these key concepts is essential for researchers who seek to navigate the complex ethical landscape of AI applications.
Several foundational elements define AI Research Ethics. First, there is the significance of bias and fairness, which challenges researchers to consider the potential biases inherent in AI systems. Next is the need for transparency and accountability, emphasizing that researchers must clearly communicate how AI-driven decisions are made. Additionally, fostering an ethical culture within research teams is crucial, as it promotes awareness and adherence to ethical standards throughout the research process. By grasping these concepts, researchers can better align their work with ethical practices, ultimately improving trust and integrity in AI research outcomes.
- Definition of AI Research Ethics
AI Research Ethics involves a framework for understanding and addressing the moral implications of artificial intelligence in research. This concept emphasizes principles such as responsibility, transparency, and fairness that guide researchers in their decision-making processes. As AI tools shape our findings and influence outcomes, it becomes crucial to ensure that these technologies are employed ethically and responsibly.
One key aspect of AI Research Ethics is recognizing potential biases embedded in algorithms. Researchers must critically assess their data sources and application contexts to ensure fairness and avoid unjust representations. Additionally, maintaining transparency about data usage and algorithmic processes helps build trust between researchers and the communities they serve. Upholding these ethical standards is vital not only for credibility but also for advancing the integrity of research that leverages AI technologies in decision-making.
- Importance in decision-making
Effective decision-making is at the core of successful research outcomes, especially when integrating AI into the process. The importance of AI research ethics cannot be overstated in this context. Ethical considerations guide researchers in making decisions that are not only data-driven but also responsible and fair. By prioritizing ethics, researchers foster trust with participants and stakeholders, ensuring that all outcomes reflect a commitment to integrity.
Moreover, ethical frameworks assist in navigating complex scenarios where AI models might perpetuate biases or lack transparency. Engaging with AI research ethics means that decision-makers can address potential ethical pitfalls before they compromise the integrity of the research. Incorporating ethical practices into AI-informed decisions creates a robust foundation for innovation and accountability, which are essential in fostering a responsible research environment. Ultimately, making informed, ethical decisions enhances the credibility and impact of research in society.
Ethical Challenges in AI-Powered Decision Making
AI-powered decision-making presents significant ethical challenges that require careful consideration. One of the main concerns is bias and fairness. Algorithms can unintentionally perpetuate existing biases found in their training data, leading to unequal treatment of different groups. Addressing this issue involves acknowledging the potential for discriminatory outcomes and implementing measures to mitigate bias in AI systems.
Transparency and accountability are also critical ethical challenges. Stakeholders must understand the underlying processes of AI decision-making, which can often seem opaque. Ensuring that AI systems operate transparently allows for greater scrutiny and fosters trust in their outcomes. Furthermore, establishing accountability mechanisms guarantees that those responsible for AI implementations can be held answerable for their consequences. By focusing on these key areas, the ethical dilemmas in AI-powered decision-making can be effectively navigated, promoting integrity and fairness in research practices.
- Bias and Fairness
AI-powered decision-making processes can unintentionally perpetuate bias and inequality. Understanding bias and fairness is crucial in the realm of AI research ethics. Bias may arise from various sources, including the data used to train algorithms and the assumptions built into model design. Consequently, outcomes can reflect historical injustices or exclude certain demographics, leading to unfairness. This scenario not only compromises the integrity of research methodologies but also raises serious ethical questions regarding the impact on affected individuals.
To address these issues, it is imperative to establish mechanisms for identifying and mitigating bias. First, conducting comprehensive audits of datasets is vital to uncover any embedded prejudices. Second, employing fairness metrics can help gauge the equity of outcomes generated by AI systems. Lastly, fostering a culture of inclusivity in AI research development can significantly enhance the effectiveness of these solutions. Navigating these complexities requires ongoing assessment and commitment to fairness, pivotal components of AI research ethics in decision-making.
- Transparency and Accountability
Transparency and accountability are key components of ethical AI research. Researchers must ensure that the processes surrounding AI decision-making are open and comprehensible. This means clearly documenting how algorithms function and the data sources they utilize. Stakeholders should have access to this information to understand the AI's decision-making processes, enhancing trust and reducing skepticism.
Moreover, accountability involves recognizing the impact of AI decisions and being prepared to address any adverse outcomes. Establishing a clear framework for responsibility is essential. Researchers should identify who makes decisions based on AI outcomes and how they will respond to errors or biases. This holistic approach is crucial in aligning AI applications with societal values, fostering ethical standards in AI research, and creating an environment where stakeholders are informed and empowered.
Extract insights from interviews, calls, surveys and reviews for insights in minutes
Best Practices for Ethical Decision-Making with AI
Ethical decision-making in AI requires a deliberate approach to ensure responsible use. First, it's essential to identify potential ethical concerns that may arise during research processes. This involves recognizing factors such as bias, data privacy, and the implications of AI-generated outcomes. Clarifying these issues allows researchers to create a framework of ethical guidelines that can effectively guide decisions.
Next, developing comprehensive guidelines is crucial for navigating complex scenarios. These guidelines should address standards for transparency, accountability, and fairness in AI applications, ensuring research outcomes align with broader ethical principles. Finally, implementing monitoring and oversight procedures helps maintain these ethical standards. Regular assessments can identify areas for improvement and ensure adherence to established guidelines. By following these practices, researchers can uphold AI research ethics and promote responsible decision-making in their work.
Implementing AI Research Ethics: A Step-by-Step Guide
Implementing AI Research Ethics requires a structured approach to ensure ethical considerations are integrated into decision-making processes. The first step involves identifying potential ethical concerns that may arise from AI applications. This could include issues like bias, privacy violations, and the impact on marginalized communities. A thorough risk assessment helps visualize the ethical landscape and prioritize areas needing attention.
Next, developing ethical guidelines is crucial. These guidelines should outline organizational principles and standards, addressing transparency, inclusivity, and accountability. Engaging diverse stakeholders during this process ensures adherence to ethical norms and cultivates a culture of ethical awareness.
Lastly, establishing monitoring and oversight procedures is essential. Regular evaluations help track compliance with ethical standards and adjust practices as needed. This systematic approach not only promotes responsible AI use but also fosters trust and credibility in research outputs, making AI Research Ethics an integral part of decision-making.
Step 1: Identifying Ethical Concerns
In Step 1: Identifying Ethical Concerns, it is crucial to assess various ethical dilemmas that may arise when employing AI in research. The integration of AI in decision-making processes has the potential to improve efficiencies, but it also introduces challenges related to ethics. Several primary concerns include privacy, data security, and bias in data and algorithms. Understanding these issues is essential for fostering an ethical approach to AI Research Ethics.
To effectively identify ethical concerns, researchers should consider the following points:
Data Privacy: Ensuring sensitive information remains protected is paramount. Researchers must evaluate how data is collected, stored, and used.
Algorithmic Bias: AI systems can inadvertently reinforce societal biases. Detailed scrutiny of data sources and algorithmic outcomes is necessary to mitigate this risk.
💬 Ask About This Article
Have questions? Get instant answers about this article.
Powered by Insight7Informed Consent: Engaging participants without transparency about AI's role can lead to ethical breaches. Researchers should seek clear consent regarding the use of AI-driven analysis.
By addressing these ethical dimensions upfront, researchers can create a stronger foundation for responsible and trustworthy AI-driven decision-making.
Step 2: Developing Ethical Guidelines
Creating ethical guidelines is a critical step in establishing principles around AI research ethics. These guidelines help navigate the complex issues surrounding AI decision-making, ensuring that researchers uphold integrity and accountability throughout their work. To effectively develop these guidelines, it is essential to consider the perspectives of all stakeholders involved, including researchers, participants, and the broader community.
The process typically involves several key considerations. First, identifying potential ethical dilemmas related to bias, privacy, and data usage is crucial. Next, clear protocols should be established to address these concerns, ensuring transparency and fairness in AI applications. Finally, ongoing training and education should be implemented to foster a culture of ethical awareness among researchers and decision-makers. By building these ethical frameworks, organizations can better safeguard trust and enhance the value of their AI-driven research initiatives.
Step 3: Monitoring and Oversight Procedures
Monitoring and oversight procedures are crucial for ensuring ethical standards in AI research. This step focuses on continuous evaluation of AI systems used in decision-making processes, safeguarding against unethical practices. By implementing these procedures, organizations can identify and address issues such as data misuse or non-compliance with ethical guidelines. The goal is to create a robust framework that sustains AI research ethics throughout its lifecycle.
To effectively monitor AI applications, organizations should establish clear protocols. First, ensure regular audits of AI algorithms to identify any biases that may arise. Second, foster transparent communication, allowing stakeholders to report concerns without fear of retaliation. Third, incorporate user feedback mechanisms to gather insights on the ethical implications of AI use. Finally, provide training for staff on ethical conduct and data protection requirements. By addressing these aspects, monitoring can empower ethical decision-making, maintaining trust in AI systems.
Tools to Enhance Ethical AI Research Decision-Making
Integrating robust tools into ethical AI research decision-making is essential for maintaining integrity and accountability. Several applications can enhance AI research ethics, ensuring that diverse concerns, such as bias and transparency, are adequately addressed. For instance, tools like AI Fairness 360 provide comprehensive metrics to evaluate model fairness, helping researchers identify potential biases in data or algorithms. Similarly, IBM Watson OpenScale offers insights into AI model performance and accountability, enabling teams to monitor their systems continually.
Incorporating these tools facilitates a structured approach to ethical decision-making. By utilizing specialized software for assessing ethical compliance, researchers can systematically identify ethical concerns, develop robust guidelines, and implement effective monitoring practices. This proactive strategy ensures that AI applications in research uphold the principles of fairness and equity, fostering trust and promoting responsible innovation within the research community. Ultimately, these advanced tools empower researchers to navigate the complex landscape of AI ethics effectively.
- insight7
In today's data-driven world, the application of AI technology in research decision-making raises significant ethical concerns. insight7 aims to enhance understanding of these AI research ethics by providing a framework to analyze data responsibly. As organizations increasingly utilize AI to interpret vast amounts of data, the importance of ethical practices becomes paramount. Striking a balance between innovation and ethical integrity ensures that decisions are made in a fair and accountable manner.
To navigate the complexities of AI research ethics, several key challenges must be addressed. First, bias must be recognized and mitigated, as it can skew data analysis and lead to unjust outcomes. Second, transparency is critical; stakeholders should understand the tools used and the implications of AI-driven insights. Finally, establishing a culture of accountability within organizations helps create an environment where ethical considerations are prioritized. By understanding and implementing these facets of AI research ethics, organizations can forge a path toward responsible decision-making.
- Features and applications
AI tools have transformative features that significantly enhance research decision-making. These innovations allow researchers to automate data analysis, identify patterns, and gain insights quickly. One notable feature is natural language processing, which enables users to extract meaningful information from unstructured text, such as customer interviews or survey responses. This capability promotes ethical practices by minimizing cognitive bias and providing a more objective perspective when summarizing findings.
Applications of AI in research extend to various fields, including market research, healthcare, and social sciences. By employing predictive analytics, researchers can forecast trends and make informed decisions while adhering to ethical guidelines. Moreover, visual analytics tools can help researchers communicate their findings effectively, ensuring transparency in methodology and results. As AI continues to evolve, integrating ethical considerations in its deployment becomes essential, ensuring that advanced features are used responsibly for impactful research outcomes.
- Other AI Ethics Tools
In the evolving field of AI research ethics, several tools can assist researchers in navigating ethical dilemmas. These tools aim to address critical issues such as bias, fairness, and accountability. By utilizing these resources, researchers can ensure that their decision-making processes align with ethical standards. Being mindful of AI research ethics is essential, as it directly impacts the integrity of the findings and their implications in society.
Among the notable tools available, AI Fairness 360 stands out for its ability to detect and mitigate biases across datasets. DataRobot AI Ethics offers a platform for teams to develop ethical models seamlessly. Fairness Flow provides a framework for evaluating ethical considerations throughout the AI lifecycle. IBM Watson OpenScale enhances transparency by enabling continuous monitoring of AI systems. Each of these tools serves as a valuable asset in promoting ethical practices within AI-driven research, ensuring responsible decision-making.
- AI Fairness 360
AI Fairness 360 plays a pivotal role in addressing ethical challenges faced during AI research decision-making. This framework critically evaluates AI models for fairness, helping researchers identify and mitigate biases that could skew results. As organizations increasingly rely on AI technologies, understanding these ethical implications becomes paramount.
The AI Fairness 360 toolkit provides essential resources for assessing model bias, implementing fairness metrics, and fostering transparency in AI systems. By utilizing this toolkit, researchers can enhance their compliance with AI research ethics, ensuring that their decisions are not only data-driven but also socially responsible. For example, researchers can evaluate the fairness of their algorithms across various demographics, striving to eliminate unfair advantages and promote equitable outcomes. Ultimately, AI Fairness 360 empowers researchers to create models that uphold ethical standards, fostering trust and accountability in AI-driven decision-making processes.
- DataRobot AI Ethics
Ethical considerations are paramount when integrating AI into research decision-making. DataRobot AI Ethics serves as a framework for understanding the implications of using artificial intelligence responsibly and effectively. It encapsulates the principles governing AI use in research, focusing on the intricacies of AI Research Ethics. This framework guides stakeholders to navigate the complexities of ethical dilemmas and promote fairness and transparency, essential traits in the evolving AI landscape.
Key aspects include the commitment to minimizing bias and ensuring accountability in AI systems. Researchers must continuously assess how AI impacts diverse populations and consider the long-term consequences of their decisions. The frameworks employed by DataRobot and similar entities also underscore the necessity of engaging diverse teams in the development and deployment of AI technologies. Embracing these ethical principles not only enhances the credibility of research outcomes but also fosters public trust in AI-driven decision-making processes.
- Fairness Flow
Fairness Flow refers to the continuous evaluation and adjustment of AI systems to ensure equitable outcomes in research decision-making. This process is vital in addressing the ethical concerns associated with AI Research Ethics. As AI technologies are employed in various sectors, monitoring for biases throughout their operation becomes necessary. Fairness Flow emphasizes the need for ongoing scrutiny to safeguard against unintentional discrimination or inequity.
Establishing a Fairness Flow involves several key steps. First, it requires systematically identifying potential sources of bias that could skew results or affect decision outcomes. Next, the data and algorithms used in AI models should be assessed for fairness, ensuring they align with established ethical guidelines. Finally, consistent monitoring and updates to these systems are essential to maintain their fairness over time. By prioritizing Fairness Flow, organizations can foster trust and accountability in their AI-driven research processes, ultimately supporting better-informed decisions for all stakeholders involved.
- IBM Watson OpenScale
IBM Watson OpenScale offers a comprehensive approach to addressing AI Research Ethics, particularly in research decision-making. This platform enables organizations to monitor AI models for fairness, transparency, and accountability. By embedding these ethical principles into the AI lifecycle, it becomes easier to identify and mitigate biases that could affect research outcomes. This approach not only promotes ethical standards but also bolsters trust in AI-driven results.
Furthermore, OpenScale allows organizations to visualize how algorithms make decisions, fostering transparency in their methodologies. With features that assess and illustrate the ethical implications of AI, this platform supports researchers in aligning their practices with established ethical guidelines. By utilizing OpenScale, decision-makers can create a more ethical framework, ensuring that AI Research Ethics are prioritized in their research initiatives. This alignment can ultimately lead to more reliable and inclusive outcomes in decision-making processes.
Conclusion on AI Research Ethics in Decision Making
Ethical considerations in AI research decision-making are critical for fostering trust and integrity in various fields. Ultimately, AI Research Ethics serves as a guide to navigate the complex landscape of technology's potential impacts. It emphasizes the need for fairness, accountability, and transparency, ensuring that AI-driven decisions respect fundamental human rights and societal values.
As we conclude, it is vital to prioritize ethical frameworks throughout the decision-making process. By embedding ethics into AI applications, researchers can mitigate risks associated with bias and misinformation. Therefore, making informed choices aligned with AI Research Ethics will contribute significantly to responsible innovation, benefiting society as a whole.
Analyze & Evaluate Calls. At Scale.
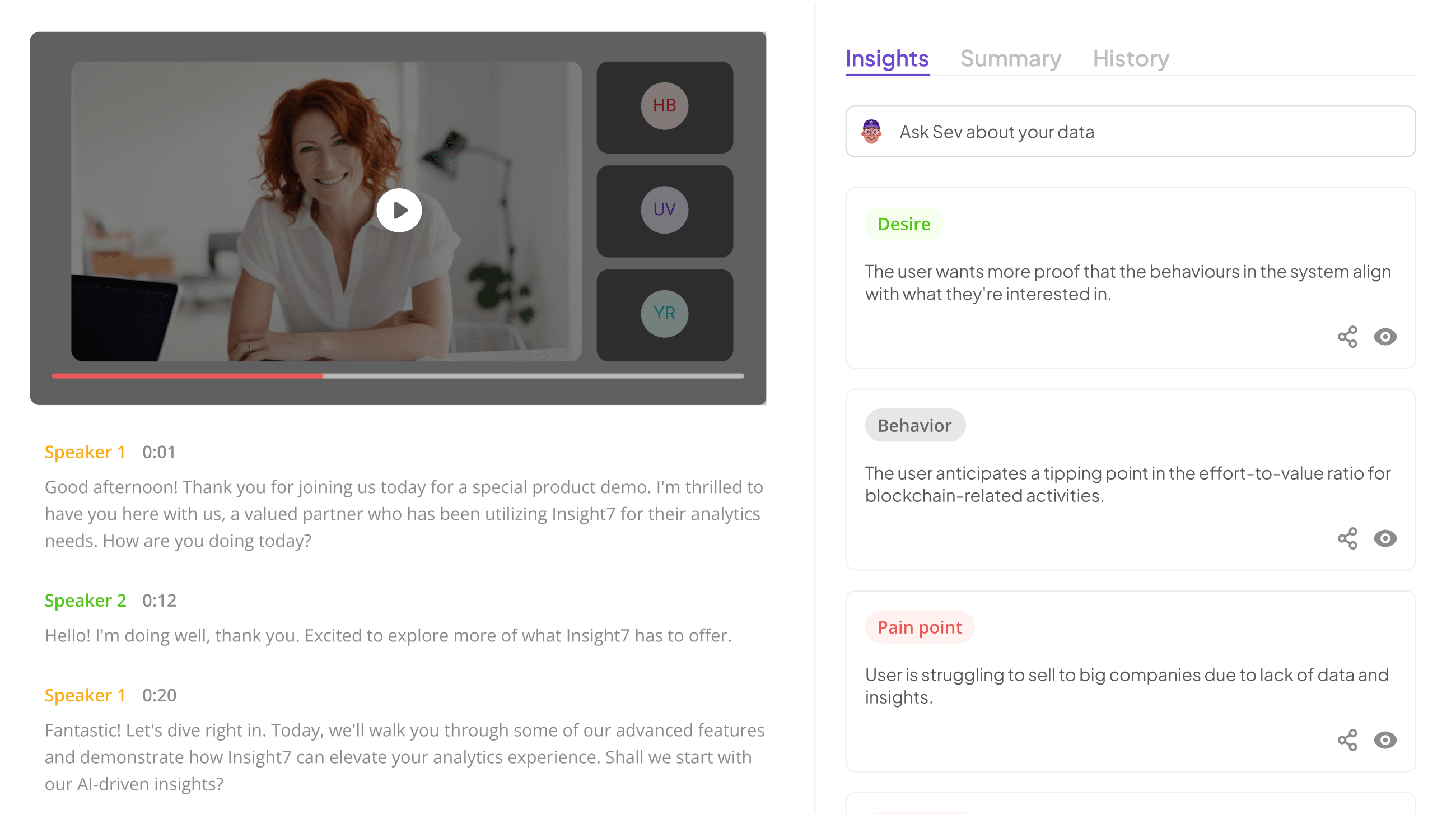
💬 Ask About This Article
Have questions? Get instant answers about this article.