Ethical AI in Qualitative Research: Avoiding Algorithmic Bias
-
Bella Williams
- 10 min read
In our increasingly data-driven world, the influence of Artificial Intelligence on qualitative research has grown exponentially. However, with this evolution comes the critical issue of algorithmic bias, which can distort findings and perpetuate inequalities. Understanding algorithmic bias mitigation is essential for researchers who aim to produce reliable and ethically sound results. As AI applications become more prevalent, it becomes imperative to address biases that may arise from flawed data or decision-making processes.
Qualitative research, rooted in human experience and subjectivity, demands a nuanced approach to algorithmic bias mitigation. This section will delve into the pressing need for researchers to identify potential biases within their methodologies and develop effective strategies to counter them. By examining the intersection of AI and qualitative practices, we can foster a space that prioritizes ethical standards and promotes fairness. It is through this understanding that researchers can enhance the integrity of AI-driven analyses, paving the way for more equitable outcomes in various fields.
Analyze & Evaluate Calls. At Scale.
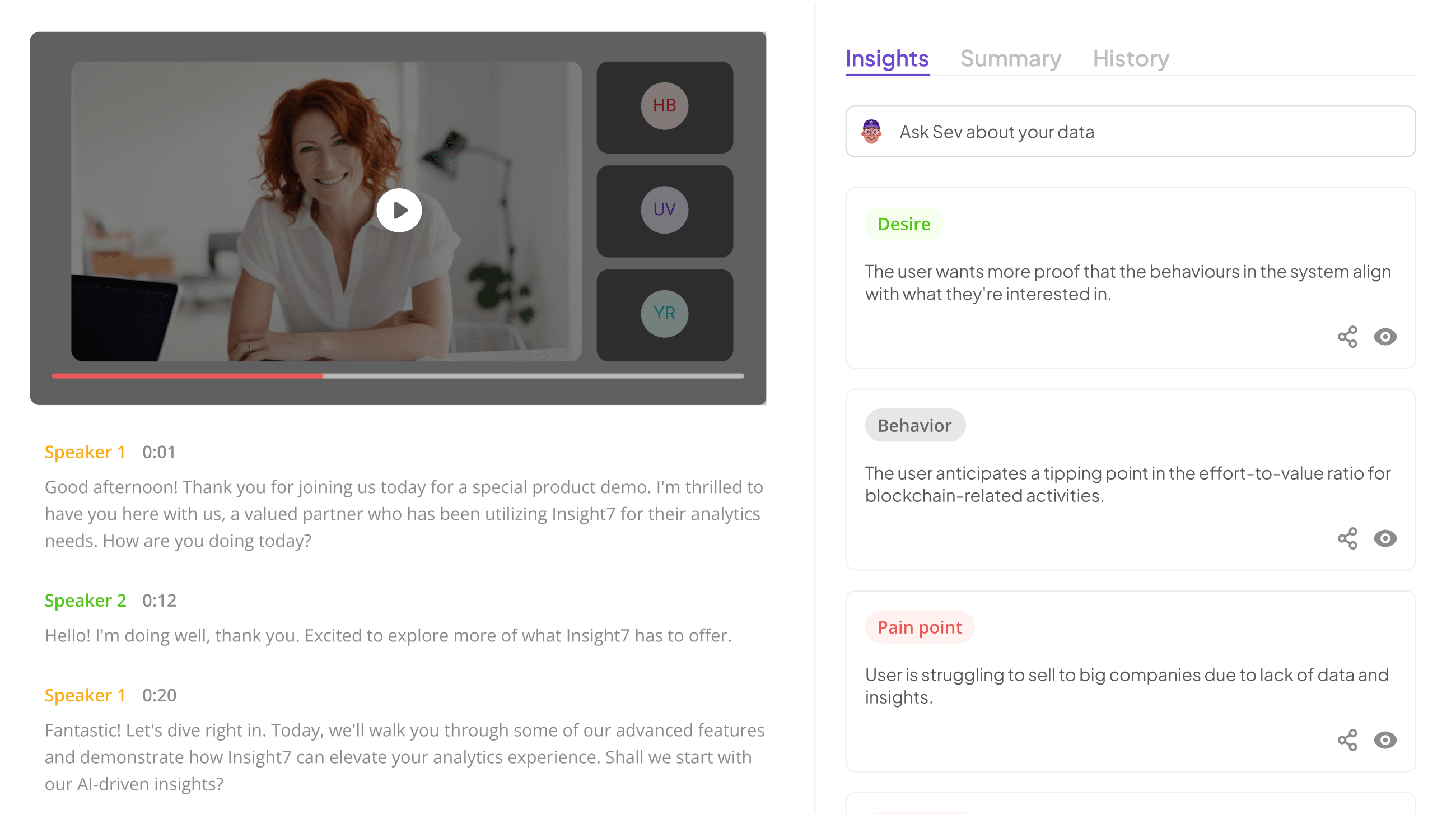
In the rapidly evolving field of Artificial Intelligence, ethical considerations are paramount. This blog explores the concept of Algorithmic Bias Mitigation, particularly within qualitative research, to ensure AI applications uphold integrity and fairness.
In the rapidly evolving field of Artificial Intelligence, ethical considerations are paramount. As researchers and developers harness the power of AI in qualitative research, the need for Algorithmic Bias Mitigation becomes increasingly critical. To maintain the integrity of AI applications, it is essential to recognize how biases can distort research outcomes and affect decision-making processes.
Addressing this challenge involves several key approaches. First, identifying sources of bias is crucial; researchers must analyze the training datasets and the algorithms used to ensure they are free from prejudices. Next, implementing fairness-enhancing interventions will help create more equitable AI models. By adopting these strategies, we can ensure that AI technologies not only function effectively but also uphold fairness and inclusivity across diverse communities. Ultimately, fostering an ethical AI landscape invites trust and promotes better outcomes in qualitative research, reinforcing the importance of responsible AI development.
The Role of AI in Qualitative Research
Artificial Intelligence plays a transformative role in qualitative research by significantly enhancing data analysis. Researchers can benefit from AI's ability to sift through vast amounts of unstructured data, identifying patterns and themes that human analysts might overlook. This capability can lead to richer insights, improving the overall quality of research findings.
However, the introduction of AI also raises concerns regarding algorithmic bias mitigation. If left unaddressed, biases in AI datasets can skew results and perpetuate existing societal disparities. Therefore, researchers must remain vigilant, ensuring that the AI systems they utilize are transparent, fair, and accountable. Implementing strategies that identify and rectify bias at various stages of the research process is essential to maintaining the integrity and reliability of qualitative insights. By incorporating these considerations, AI can become a valuable ally in achieving ethical research outcomes.
AI technologies, when integrated into qualitative research, can greatly enhance data analysis capabilities. However, its crucial to remain vigilant about potential biases that could compromise research outcomes.
AI technologies have the potential to revolutionize qualitative research by significantly enhancing data analysis capabilities. These tools offer unparalleled efficiency, allowing researchers to process vast amounts of qualitative data more thoughtfully and quickly. With advanced algorithms, AI can uncover patterns and insights that might otherwise go unnoticed, ultimately enriching the research process. However, as we leverage these technologies, it's imperative to recognize the importance of algorithmic bias mitigation.
When AI is employed without a critical eye, the risk of perpetuating existing biases is considerable. Bias can seep into AI models, influencing outcomes and leading to flawed interpretations of data. For qualitative research to remain ethical and valid, researchers must be proactive in identifying potential sources of bias within their AI systems. By implementing targeted strategies and fairness-enhancing interventions, they can safeguard the integrity of their findings and ensure a more equitable representation of diverse perspectives in their analyses.
Key Benefits of AI Integration
Integrating AI into qualitative research presents significant advantages, particularly in terms of efficiency and depth of analysis. Firstly, AI can process vast amounts of data quickly, enabling researchers to derive insights faster than traditional manual methods. This expedites decision-making processes and enhances the overall quality of research outcomes.
Moreover, AI tools can detect patterns and trends in data that may be challenging for human analysts to identify. This capability aids in making sense of complex data sets, ensuring that valuable insights are not overlooked. Additionally, the integration of AI supports a more diverse range of qualitative methods, such as sentiment analysis and thematic exploration, which can further enrich research findings.
However, as AI becomes more prevalent, careful attention to algorithmic bias mitigation is essential. Unaddressed biases in AI systems can distort research findings and perpetuate existing inequalities. Therefore, leveraging AI responsibly involves continuous scrutiny to ensure ethical standards are met.
AI tools can streamline data processing, leading to more efficient and comprehensive analysis.
AI tools significantly enhance the efficiency of data processing in qualitative research. By automating labor-intensive tasks, these technologies allow researchers to focus on more complex analyses that require human insight. The integration of AI streamlines the handling of large volumes of qualitative data, transforming it into structured formats for easier evaluation. This capability not only enhances speed but also improves the comprehensiveness of insights derived from the data.
However, it is critical to ensure that these tools are designed with algorithmic bias mitigation in mind. AI systems can unintentionally perpetuate existing biases if not carefully monitored and adjusted. By fostering a robust methodology for identifying and addressing potential biases within AI datasets, researchers can leverage these tools to deliver not only faster insights but also more equitable outcomes. Proper implementation of AI in qualitative research creates opportunities to unearth deeper insights while upholding ethical standards and integrity in research practices.
Potential Pitfalls without Bias Mitigation
In the absence of bias mitigation, researchers face significant pitfalls that can compromise the integrity of their findings. One primary risk is the reinforcement of existing societal biases, which leads to skewed data interpretations. For instance, if training datasets reflect historical inequalities, AI models may perpetuate those biases, skewing the research outcomes and further marginalizing underrepresented groups. Moreover, without bias mitigation, researchers may unknowingly rely on flawed assumptions that distort qualitative insights, ultimately affecting decision-making processes.
Another potential pitfall is the erosion of trust among participants and stakeholders. When biases infiltrate research, the credibility of the findings can come into question. This mistrust not only jeopardizes future research efforts but also undermines the ethical foundations of qualitative inquiry. By failing to address algorithmic bias, researchers risk misinforming policy decisions, which can have far-reaching consequences across various sectors. Therefore, proactive measures in algorithmic bias mitigation are essential to uphold the quality and fairness of qualitative research.
Failing to address biases can result in skewed data interpretations and reinforce societal biases.
Failing to address biases can create significant challenges in the field of qualitative research. When biases go unrecognized, data interpretations become skewed, leading to decisions grounded in invalid assumptions. For instance, algorithmic tools can reflect societal biases present in their training data, inadvertently perpetuating stereotypes. This misrepresentation marginalizes minority communities, posing ethical dilemmas that researchers must confront.
To mitigate algorithmic bias effectively, researchers should first identify potential sources of bias within the datasets. This might include evaluating the demographics represented in the training data or scrutinizing the algorithms used. Next, implementing fairness-enhancing interventions can be crucial. Techniques may involve adjusting datasets to ensure a more balanced representation or continuously monitoring model outputs to detect and address biases. By adopting these strategies, researchers can help ensure that data interpretations are more accurate and equitable, ultimately supporting the ethical advancement of AI in qualitative research.
Strategies for Effective Algorithmic Bias Mitigation
To mitigate algorithmic bias effectively, researchers must first identify the sources of bias that can infiltrate their models. This includes scrutinizing training datasets and understanding how certain data elements may inadvertently represent or reinforce existing prejudices. By conducting a rigorous examination of the underlying algorithms, researchers can pinpoint areas where bias is most likely to emerge.
Next, implementing fairness-enhancing interventions is crucial. This might involve adopting techniques such as algorithmic adjustments or utilizing fairness-aware algorithms designed specifically to counteract biases. Researchers can also focus on inclusive data practices, ensuring that diverse perspectives are represented in their datasets. By fostering a culture of awareness and continual evaluation of practices, researchers contribute to building ethical AI frameworks that prioritize equity and integrity in qualitative research. In sum, a proactive approach to algorithmic bias mitigation not only enhances research outcomes but also promotes trust in AI technologies.
Extract insights from interviews, calls, surveys and reviews for insights in minutes
To maintain ethical standards, researchers must implement strategies to mitigate algorithmic bias.
To uphold ethical standards in qualitative research, it is crucial for researchers to actively implement strategies that address algorithmic bias. These biases often stem from the data used to train AI models, which can reflect societal inequities and stereotypes. Therefore, the first strategy involves identifying sources of bias, requiring a meticulous review of datasets to understand the underlying narratives they may perpetuate.
Following identification, researchers should implement fairness-enhancing interventions. This could include techniques such as data augmentation, which balances representation, or model adjustments that promote equitable outcomes. Additional practices involve regular audits of AI outputs for unintentional bias and engaging diverse stakeholders in the research process. Through these concerted efforts, researchers not only improve the reliability of their findings but also foster trust and integrity in their work, ultimately contributing to the mission of ethical AI.
Step 1: Identifying Sources of Bias
Identifying sources of bias represents a foundational step in Algorithmic Bias Mitigation. By examining the origins of bias, researchers can gain insight into how it manifests and influences outcomes in AI-driven qualitative research. Bias may stem from various sources, including unrepresentative training datasets, flawed algorithms, and even subjective human judgments made during data collection and analysis. Recognizing these factors early on empowers researchers to mitigate their impacts effectively.
To navigate these complexities, consider the following key pointers:
Analyze Training Data: Scrutinize the datasets used for training AI models to ensure they are representative of the population being studied. Diversity in data can greatly reduce bias.
Examine Algorithm Design: Review the algorithms for potential biases in logic or processing that could skew results. Collaboration with data scientists can aid in detecting subtle biases.
Gather Diverse Perspectives: Involve a team of researchers with varied backgrounds throughout the research process. This diversity helps ensure that multiple viewpoints are considered.
💬 Questions about Ethical AI in Qualitative Research: Avoiding Algorithmic Bias?
Our team typically responds within minutes
By methodically identifying these sources, researchers will be better equipped to enhance the ethical standards necessary for impactful qualitative research.
Understanding where biases originate is critical for addressing them effectively. This involves a thorough examination of AI training datasets and algorithms.
Identifying the origins of biases in AI is essential for developing solutions that effectively address them. These biases usually stem from the datasets used in training algorithms, which can often reflect historical inequalities or societal prejudices. A careful examination of these datasets is critical to discern the specific areas where bias may enter the system. This investigation allows researchers to understand how underlying factors influence AI outcomes, a necessary step in the Algorithmic Bias Mitigation process.
Moreover, scrutinizing algorithms themselves is vital. Even well-intentioned algorithms can propagate biases, primarily when the underlying assumptions are flawed. By analyzing how data is processed, researchers can uncover flaws and implement fairness-enhancing interventions. This approach promotes an equitable framework in qualitative research, ultimately leading to unbiased AI outputs. The goal is to ensure that AI technologies enhance, rather than hinder, the pursuit of fair and reliable research insights.
Step 2: Implementing Fairness-Enhancing Interventions
To combat algorithmic bias effectively, researchers must proactively implement fairness-enhancing interventions. These interventions can take several forms, but they primarily focus on adjusting algorithms or their training data to ensure more equitable outputs. By incorporating techniques such as re-sampling, re-weighting, or utilizing adversarial training methods, researchers can actively address biases that may already exist in their datasets.
One effective approach involves auditing the datasets for representational fairness. Ensuring that the data reflects diverse demographics can significantly reduce bias. Additionally, employing fairness constraints during model training can help manage and mitigate biased outcomes. Encouraging transparent reporting and collaborative evaluations within research teams will also foster an environment of accountability, making it easier to identify and rectify sources of bias. By being vigilant and diligent in these efforts, researchers can ensure that their AI applications maintain ethical standards, ultimately leading to more trustworthy qualitative research.
Adopt techniques and interventions designed to promote fairness and reduce bias within AI models.
Adopting techniques and interventions designed to promote fairness and reduce bias within AI models is essential in ensuring that qualitative research produces equitable outcomes. Researchers can implement various strategies to address algorithmic bias effectively. One of the first steps is to establish guidelines that outline the specific criteria for fairness. By clearly defining what fairness means in the context of their work, researchers can measure and improve their AI models accordingly.
Additionally, data preprocessing techniques play a crucial role in promoting fairness. These methods involve scrutinizing datasets for imbalances that may introduce biases. Researchers should also consider algorithmic interventions, like fairness-enhancing modifications, which can adjust how AI systems make decisions based on their training data. Emphasizing continuous evaluation and feedback will help further refine the models. By adopting these techniques, researchers can better uphold ethical standards, significantly reducing the impact of algorithmic bias in their findings.
Tools for Effective Bias Mitigation in Qualitative Research
Effective bias mitigation in qualitative research involves utilizing various tools to ensure that algorithmic bias does not compromise the integrity of research outcomes. To achieve this, it is essential to harness tools that can identify, monitor, and address biases proactively. By leveraging these tools, researchers can create a more equitable research environment, ultimately enhancing the reliability of their findings.
Among the effective tools available for bias mitigation are those designed for pre-processing datasets, monitoring AI model outputs, and enabling collaborative bias assessments. For instance, some tools offer functionalities to balance datasets properly, while others specialize in real-time bias alert systems that notify researchers of potential issues in their AI models. Each of these tools contributes significantly to ethical AI practices in qualitative research, ensuring researchers maintain a critical focus on fairness and equity within their studies.
insight7
Ethical AI in qualitative research hinges on the complex, yet vital, task of Algorithmic Bias Mitigation. It begins with embracing the full scope of data generated from user interactions. By proactively addressing biases that emerge from data collection methods, researchers can create a more balanced understanding of customer insights. Recognizing the sources of these biases allows researchers to tailor their approaches and findings effectively.
To navigate the challenges posed by algorithmic bias, consider employing a series of strategic measures. First, conduct a comprehensive examination of your data sources. Next, implement fairness-enhancing interventions that focus on training datasets and algorithms. Lastly, utilize advanced tools designed to identify and rectify biases in AI models. By adhering to these steps, researchers can significantly improve the integrity and reliability of their qualitative research efforts, fostering a more ethical application of AI technologies.
As a leading tool, insight7 offers advanced capabilities for detecting and mitigating biases in AI-driven qualitative research.
The tool set designed to detect and mitigate biases in AI-driven qualitative research has significantly evolved. These advanced capabilities are essential for researchers aiming to uphold ethical standards in their work. By incorporating techniques that scrutinize data sources and algorithms, the system allows for the identification of potential algorithmic biases that can distort research findings. This proactive approach empowers researchers to refine their methodologies, ensuring that the insights gathered are both accurate and representative.
Moreover, the tool facilitates the implementation of fairness-enhancing interventions, which are vital in promoting equitable outcomes in research. By providing a structured framework for collaboration, researchers can engage in comprehensive bias assessment processes. This not only enhances the reliability of research outcomes but also fosters a culture of accountability in AI use. Overall, leveraging such advanced capabilities ultimately leads to more trustworthy qualitative research, contributing to improved decision-making in various sectors.
Other Tools
In the quest for ethical AI in qualitative research, various tools play a crucial role in mitigating algorithmic bias. These tools are designed to enhance data integrity and promote fairness in research findings. Some tools focus on preprocessing datasets, enabling researchers to balance data inputs and reduce inherent biases. This foundational step ensures that the analysis reflects a diverse set of perspectives and experiences.
Additionally, tools that monitor AI outputs for hidden biases are critical. They provide insights into how AI models may unintentionally reinforce stereotypes or preconceived notions. Collaboration tools facilitate feedback integration and bias assessment across teams, fostering a more transparent research environment. Lastly, real-time bias alert systems empower researchers to identify and address issues swiftly, nurturing a culture of continuous improvement. Together, these resources support a strategy for ethical engagement with AI, ensuring research outcomes are equitable and reliable.
- Tool A: Offers robust features to pre-process and balance datasets.
In the pursuit of ethical AI in qualitative research, Tool A plays a crucial role in Algorithmic Bias Mitigation. This tool offers robust features designed to pre-process and balance datasets, ensuring that data analysis is both equitable and reliable. By preparing datasets for analysis, Tool A assists researchers in identifying underlying biases before they skew research outcomes. This pre-processing step is essential, as it can illuminate discrepancies and areas of overrepresentation that may inadvertently perpetuate bias.
The balancing features of Tool A further enhance its efficacy by equalizing the representation of various demographic groups within dataset samples. This balancing act helps to prevent any single group from disproportionately influencing the results. As researchers utilize Tool A to refine their datasets, they fortify their commitment to integrity and fairness in AI applications, leading to more valid insights. Leveraging these capabilities promotes a holistic understanding of data while minimizing biases that can arise from unexamined sources.
- Tool B: Specializes in monitoring AI model outputs for hidden biases.
Artificial Intelligence (AI) models can produce unexpected outcomes, which may include subtle biases. Tool B focuses on monitoring these AI outputs, helping researchers identify hidden biases that could skew their findings. By continuously analyzing results, this tool ensures that any bias present does not influence research outcomes, preserving the integrity of qualitative inquiry.
Additionally, it operates by assessing patterns in responses and outputs, highlighting discrepancies that might indicate unfair treatment of certain groups or viewpoints. Understanding these biases is essential for effective algorithmic bias mitigation, as it empowers researchers to make informed decisions based on accurate and representative data. By integrating Tool B into the research process, qualitative analysts gain a powerful ally in their quest to achieve fairness and equity in AI applications, ultimately enhancing the trustworthiness of their findings.
- Tool C: Facilitates collaborative efforts in bias assessment and feedback integration.
Collaborative efforts in bias assessment and feedback integration are vital for ethical AI practices. Tool C serves as an essential component in this process, enabling researchers to engage multiple stakeholders in their projects. By fostering a collaborative environment, teams can draw on diverse perspectives and experiences, which aids in identifying potential sources of bias. This shared approach not only enhances the quality of data analysis but also promotes a culture of accountability and transparency.
Additionally, this tool facilitates continuous feedback integration. Regular feedback from various participants allows for a dynamic assessment of the methodologies employed, ensuring that biases are continuously monitored and addressed. This iterative process reinforces the commitment to algorithmic bias mitigation, as it encourages ongoing dialogue and adaptation of strategies to reduce bias effectively. With Tool C, teams can navigate the complexities of qualitative research with a clearer understanding of ethical implications and a stronger foundation for reliable insights.
- Tool D: Provides real-time bias alert systems to researchers.
Real-time bias alert systems serve as critical tools for researchers seeking to navigate the complexities of qualitative research. These systems actively monitor incoming data, flagging potential biases as they arise. This immediate feedback is crucial, allowing researchers to address issues before they influence research outcomes. As a result, researchers can develop a more nuanced understanding of the data, enhancing the integrity of their findings.
One of the main advantages of utilizing real-time bias alert systems is their ability to continuously adapt to changing variables in the data collection process. This ensures that researchers are always informed about the potential for algorithmic bias. Additionally, these systems foster a culture of accountability and transparency, as researchers become proactive in assessing their work. By implementing such proactive measures, organizations not only advance the conversation around algorithmic bias mitigation but also reinforce their commitment to ethical AI practices in qualitative research.
Conclusion: The Future of Algorithmic Bias Mitigation in AI Research
In the realm of artificial intelligence, the commitment to ethical practices will shape the future of algorithmic bias mitigation. As we advance, addressing biases in AI tools becomes increasingly vital. By prioritizing transparency and accountability, researchers can ensure that AI applications are designed to reflect diverse perspectives. This process requires a continuous cycle of monitoring, assessment, and adjustment, promoting fairness in AI-driven qualitative research.
Future efforts should focus on fostering collaboration among AI developers, researchers, and ethicists. This collective approach can enhance our understanding of bias origins and support the development of comprehensive mitigation strategies. As we move forward, embracing innovative solutions will be crucial for forging equitable pathways, ultimately ensuring technology uplifts all communities while mitigating the risk of inherent biases.
As AI continues to permeate qualitative research, ongoing commitment to ethical practice through algorithmic bias mitigation is essential. The future of AI research hinges on our ability to anticipate, identify, and address biases, ensuring technology serves all communities equitably.
As AI becomes an integral part of qualitative research, ethical practice must steer its development. Algorithmic bias mitigation emerges as a key concern, as it directly influences the fairness and reliability of research outcomes. Researchers must remain proactive in recognizing and addressing biases inherent in AI systems. This vigilance ensures that technology does not inadvertently perpetuate existing societal inequalities.
To foster equitable AI applications, researchers can implement several strategies. First, it is essential to identify sources of bias within training data and algorithms, allowing for a clearer understanding of where issues may arise. Next, implementing fairness-enhancing interventions can help reduce bias and promote more accurate, inclusive results. By committing to these practices, we can ensure that AI technology serves diverse communities, making it a powerful tool for advancing qualitative research in an ethical manner.
💬 Questions about Ethical AI in Qualitative Research: Avoiding Algorithmic Bias?
Our team typically responds within minutes