Enterprise-Ready Forecasting Solutions With Predictive AI Modeling
-
Bella Williams
- 10 min read
Predictive Enterprise Forecasting begins with recognizing how powerful data-driven insights can transform decision-making processes within organizations. As businesses face constant market fluctuations, leveraging predictive AI modeling equips enterprises to anticipate demand, assess risks, and optimize resources effectively. The integration of advanced algorithms allows companies to convert historical data into actionable forecasts, ensuring they stay ahead of their competitors.
Furthermore, embracing Predictive Enterprise Forecasting not only enhances operational efficiency but also promotes strategic planning. By utilizing robust forecasting solutions, enterprises can create tailored strategies that align with their unique objectives and customer needs. This introduction serves as a stepping stone to understanding the significance of predictive models in achieving sustainable growth and navigating the complexities of modern markets.
Analyze & Evaluate Calls. At Scale.
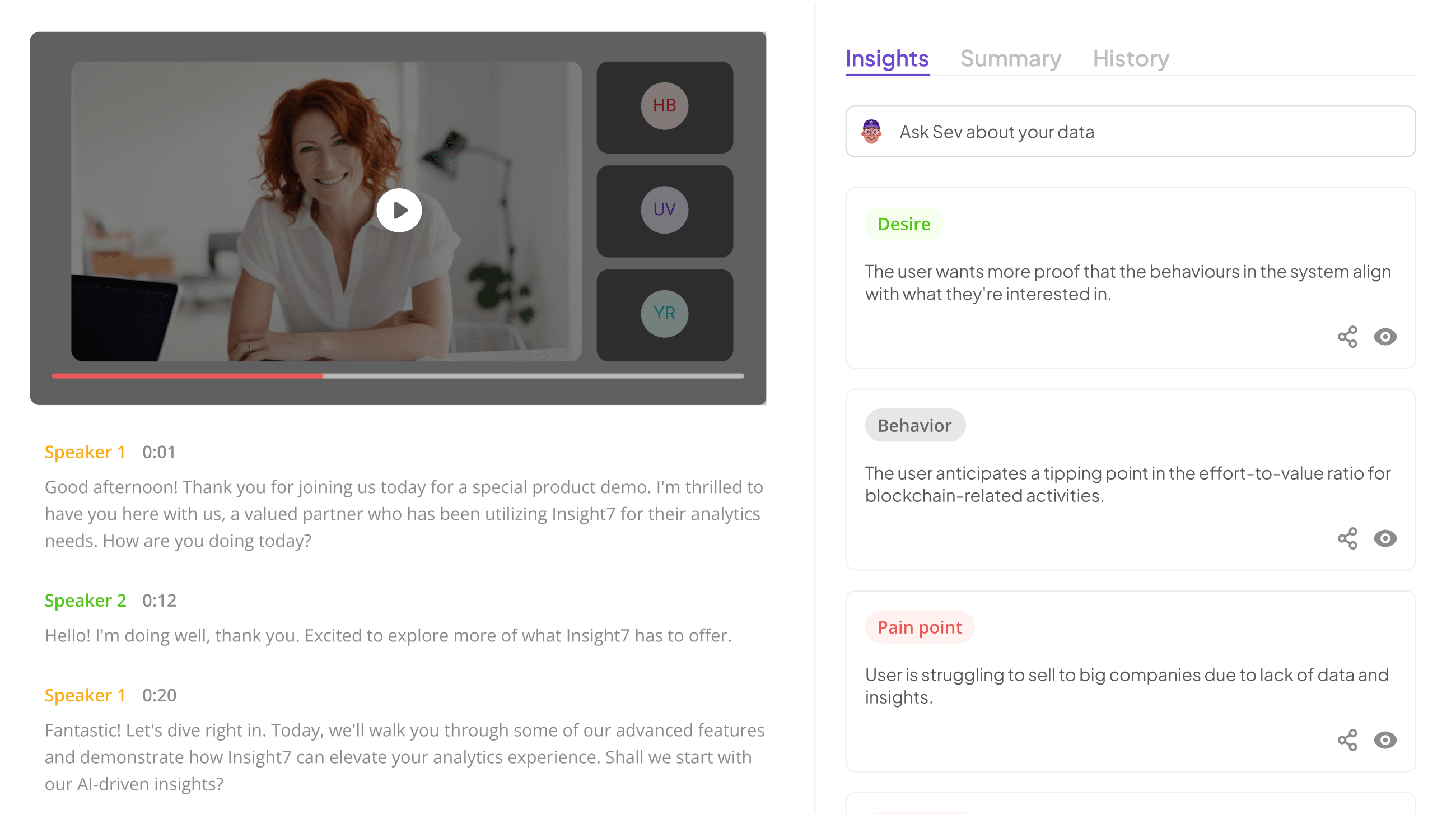
Understanding Predictive Enterprise Forecasting
Predictive Enterprise Forecasting refers to the application of advanced analytics and AI technologies to project future business outcomes based on historical data. By harnessing vast amounts of data, organizations can gain insights into trends, customer behaviors, and market dynamics. This method helps businesses make informed decisions, allowing them to allocate resources effectively and anticipate changes before they occur.
In understanding Predictive Enterprise Forecasting, it's essential to recognize that it encompasses a systematic approach that combines data collection, model development, and continuous refinement. Organizations start by analyzing historical data to identify patterns, followed by selecting appropriate predictive models tailored to their specific needs. Through ongoing validation and adjustment, these models become increasingly accurate over time, enabling businesses to thrive in competitive markets. Embracing this forecasting approach not only enhances operational efficiency but also positions enterprises to better respond to evolving demands and challenges.
The Importance of Predictive AI in Forecasting
Predictive AI plays a pivotal role in enhancing forecasting accuracy and efficiency within enterprises. By analyzing historical data and identifying patterns, it helps organizations anticipate future trends and prepare accordingly. This proactive approach goes beyond traditional methods, enabling businesses to make informed decisions based on predictive insights. Predictive enterprise forecasting transforms raw data into valuable insights, guiding strategies and resource allocation.
One key aspect of predictive AI is its ability to refine forecasts over time. As more data is collected, machine learning algorithms can adjust and improve predictions, resulting in greater accuracy. Additionally, it allows for real-time updates, meaning businesses can respond to market changes swiftly. This agility is vital in today’s fast-paced environment, ensuring that companies remain competitive and responsive. Understanding the importance of predictive AI in forecasting is essential for businesses aiming to optimize their decision-making processes and drive growth.
Key Benefits of Predictive Enterprise Forecasting
Predictive Enterprise Forecasting offers several crucial advantages that can transform how organizations plan for the future. Firstly, it enhances accuracy by analyzing large datasets, enabling businesses to make informed decisions based on real patterns rather than gut feelings. This statistical approach minimizes financial risk and optimizes resource allocation, leading to greater profitability.
Secondly, the speed of insights derived from advanced predictive models significantly improves agility. Companies can quickly respond to market shifts and customer demands, adapting strategies far more efficiently than traditional methods allow. Additionally, these forecasts promote better communication across departments, aligning teams toward common goals, thus streamlining processes and fostering collaboration.
In summary, embracing Predictive Enterprise Forecasting equips organizations with a competitive edge in an increasingly dynamic market. By improving accuracy and responsiveness, companies can unlock new growth opportunities and enhance overall decision-making processes.
Extract insights from interviews, calls, surveys and reviews for insights in minutes
Building Enterprise-Ready Forecasting Models with Predictive AI
To build enterprise-ready forecasting models with predictive AI, it is essential first to understand your data thoroughly. This step involves data collection, cleansing, and exploration to identify patterns and trends that the model can leverage. A deep understanding of data sets the foundation for accurate predictive enterprise forecasting and ensures that the model will address specific business needs and challenges effectively.
Next, selecting the right AI techniques becomes crucial. Depending on the complexity of your data and the forecasting requirements, various algorithms—from regression analysis to neural networks—might be used. After selecting the techniques, the model undergoes training and validation to ensure its robustness against real-world scenarios. By continuously monitoring model performance and making adjustments as necessary, organizations can harness predictive AI's power to drive strategic decisions and enhance operational efficiency.
Steps to Develop Effective Predictive Models
Developing effective predictive models is crucial for implementing robust predictive enterprise forecasting solutions. The process begins with understanding your data. This means not only collecting relevant datasets but also ensuring that they are clean and structured, enabling accurate analysis. Without a strong foundation in data preparation, the reliability of your forecasts may be compromised.
The next step involves selecting the right AI techniques aligned with your business goals. Different modeling techniques, such as regression, classification, or time-series analysis, should be considered based on the specific nature of your data and predictions. After selecting the appropriate methods, you’ll proceed to model training and validation. This phase involves using your datasets to train the model while also validating its performance through cross-validation techniques. Ultimately, these steps will help you generate reliable forecasts that can positively impact decision-making and business strategy.
- Step 1: Understanding Your Data
To embark on the journey of Predictive Enterprise Forecasting, understanding your data is paramount. Start by evaluating the quality, quantity, and structure of the data at your disposal. A comprehensive assessment will allow you to pinpoint any gaps or inconsistencies that could hinder the forecasting process. This foundational work establishes a framework for harnessing predictive AI effectively.
Next, categorize your data based on relevance and applicability. Consider the different types—historical sales records, customer feedback, or market trends—each of which provides unique insights crucial for accurate predictions. By organizing your data thoughtfully, you set the stage for powerful analyses that can drive decision-making and innovation. This process ensures that when you apply predictive modeling techniques, the outcomes are not only reliable but also actionable, enhancing your enterprise's forecasting capabilities substantially. Understanding your data lays the groundwork for sophisticated predictive strategies that align with organizational goals.
- Step 2: Selecting the Right AI Techniques
Selecting the right AI techniques is essential for effective predictive enterprise forecasting. Begin by recognizing your specific forecasting needs, and the attributes of your data. This clarity will help you choose AI methods that align with your goals. For example, if your data involves time series, consider using techniques like ARIMA or LSTM neural networks, which excel in capturing temporal patterns.
💬 Questions about Enterprise-Ready Forecasting Solutions With Predictive AI Modeling?
Our team typically responds within minutes
Next, evaluate various AI models based on their strengths and weaknesses. Techniques like decision trees and ensemble methods are valuable in understanding complex data interactions. It’s imperative to balance model complexity with interpretability to ensure insights are actionable. Remember, the goal is to create forecasting solutions that not only predict outcomes but also offer strategic insights for decision-making. By conducting a thoughtful analysis of your options, you can harness the power of AI to enhance your predictive capabilities effectively.
- Step 3: Model Training and Validation
During the Model Training and Validation phase, the focus shifts to refining the predictive AI model for reliable outcomes. This step involves training the model using historical data, enabling it to identify patterns and relationships that inform future forecasts. Proper training is crucial, as it ensures the model learns from the data accurately.
After training, validation follows to assess the model's performance. This is essential to establish how well the model generalizes to new, unseen data. Techniques such as cross-validation can help evaluate its predictive accuracy. Ensuring robust validation not only enhances the credibility of the predictive enterprise forecasting solution but also builds user trust in its results. By meticulously training and validating the model, organizations can ensure their forecasting capabilities are not only accurate but also enterprise-ready.
Tools for Enhancing Forecasting Accuracy
Improving forecasting accuracy is essential for effective decision-making in enterprises. Many organizations leverage various tools to enhance their predictive capabilities, ensuring data-driven strategies yield better outcomes. Predictive Enterprise Forecasting involves using sophisticated technologies to analyze historical data patterns, allowing businesses to make informed predictions about future trends.
Key tools for enhancing forecasting accuracy include advanced analytics software, machine learning algorithms, and data visualization platforms. Advanced analytics helps uncover insights within large datasets, which can inform strategic planning. Machine learning algorithms optimize models based on real-time data, continuously improving prediction accuracy over time. Data visualization platforms allow users to see trends and anomalies clearly, facilitating quicker and more effective decision-making. By integrating these tools, businesses equip themselves to anticipate market changes effectively, thus building a more resilient enterprise ready to adapt in a dynamic environment.
- Insight7: The Leading Forecasting Tool
In today’s dynamic business environment, Insight7 stands out as a premier solution in the realm of predictive enterprise forecasting. Organizations are inundated with vast amounts of customer data, making it essential to extract actionable insights rapidly. Traditional methods of data analysis fall short in this fast-paced landscape, resulting in inefficiencies and missed opportunities. Insight7 provides a user-friendly platform that facilitates the systematic analysis of data, allowing companies to make informed decisions swiftly.
The true power of Insight7 lies in its ability to transform cluttered and scattered data into coherent strategies. It streamlines the process of gathering customer insights from various sources, thus enabling businesses to convert these insights into concrete actions that drive results. By automating the analysis and interpretation of customer signals, organizations can enhance their competitive edge and foster better collaboration among teams. Adopting Insight7 is not just about keeping up with data; it's about leading the charge in predictive enterprise forecasting.
- Tool 2: A Comprehensive Overview
Effective predictive enterprise forecasting involves a multifaceted approach to data analysis and AI modeling. In this section, we will delve into essential aspects, emphasizing how businesses can harness AI-driven insights for strategic decision-making. Understanding the components of predictive forecasting tools enables enterprises to refine their projections for future trends, leading to improved operational efficiency and customer satisfaction.
Key aspects to consider include data comprehension, AI technique selection, and robust model training. First, having a thorough understanding of your data sets the foundation for effective forecasts. Second, selecting the right AI methodologies can profoundly impact the accuracy of predictions. Finally, diligent model training and validation ensure that the forecasting tools perform optimally in a dynamic market. By mastering these elements, organizations can leverage predictive enterprise forecasting to align their resources and strategies more effectively.
- Tool 3: Key Features and Capabilities
Predictive Enterprise Forecasting provides a suite of powerful features tailored for organizations aiming to enhance their predictive accuracy and operational efficiency. One of the standout capabilities is its user-friendly interface, which democratizes data access across departments. This eliminates the need for specialized training, enabling employees to leverage forecasting insights seamlessly. From generating meaningful reports to highlighting customer pain points, these tools transform raw data into actionable insights effortlessly.
Another significant feature is the robust analytics dashboard that visualizes performance metrics in real-time. By categorizing insights into clear segments, users can quickly identify trends, understand customer behavior, and make data-driven decisions. The integration of advanced AI modeling ensures that forecasts remain reliable and adaptive as patterns shift. Enhanced collaboration features also facilitate team projects, allowing users to collectively analyze multiple datasets and refine strategies based on comprehensive insights. These capabilities underline the effectiveness of Predictive Enterprise Forecasting as an essential weapon in achieving business goals.
- Tool 4: Why It’s Recommended for Enterprises
Predictive Enterprise Forecasting is highly recommended for enterprises due to its ability to transform data into actionable insights. First, it enhances decision-making by providing accurate predictions, enabling businesses to better plan their resources and strategies. This tool empowers organizations to anticipate market trends and customer behaviors, which can greatly improve competitive positioning.
Moreover, Predictive Enterprise Forecasting fosters a data-driven culture within companies. By incorporating AI, businesses can analyze vast amounts of data, uncover patterns, and generate forecasts that were previously unattainable. This leads to more informed planning and can drive operational efficiency. Lastly, utilizing such advanced forecasting tools can significantly reduce risks associated with unpredictability in the market, creating a solid foundation for growth and innovation in an ever-evolving business landscape.
Conclusion: The Future of Predictive Enterprise Forecasting
The future of predictive enterprise forecasting is promising, with advancements in AI technology paving the way for enhanced business intelligence. As organizations increasingly rely on data-driven insights, predictive analytics will become integral to decision-making processes. By leveraging historical data and real-time metrics, businesses can anticipate market trends and consumer behaviors more accurately.
Looking ahead, those adopting predictive enterprise forecasting solutions will find themselves better equipped to navigate the complexities of evolving markets. Enhanced capabilities in forecasting will not only streamline operations but also foster strategic innovation across industries. Embracing this technological advancement will empower enterprises to make informed decisions that drive sustainable growth and competitive advantage.
💬 Questions about Enterprise-Ready Forecasting Solutions With Predictive AI Modeling?
Our team typically responds within minutes