In today’s digital world, vast amounts of unstructured text data are generated every second, representing both challenges and opportunities for businesses. Search Engine Insights serve as a critical tool for transforming this data into knowledge that can guide decision-making and strategy development. ElasticSearch emerges as a powerful ally in this landscape, helping organizations unlock insights from their textual data effortlessly.
Understanding how ElasticSearch text analytics works requires a look at its sophisticated processes. By breaking down text into manageable components, it enables businesses to analyze large volumes of data seamlessly. Through this approach, firms can derive actionable insights that not only inform immediate actions but also shape long-term strategies in a competitive market. Embracing ElasticSearch empowers companies to tap into the potential of their data, ultimately driving growth and enhancing customer experiences.
Generate visualizations from your qualitative data. At Scale.
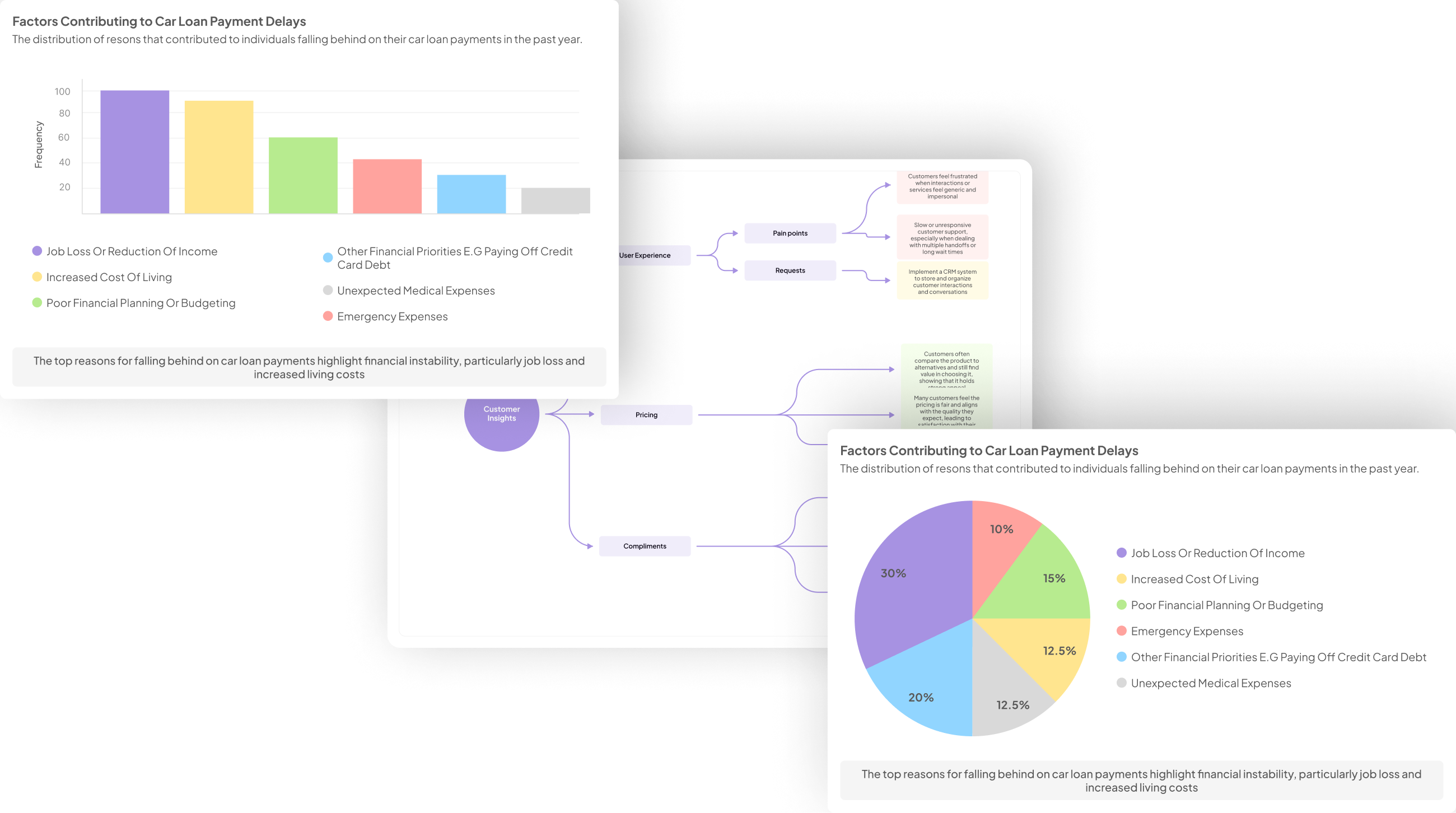
How ElasticSearch Processes Text for Search Engine Insights
ElasticSearch processes text for Search Engine Insights through an intricate series of steps that optimize data retrieval and relevance. Initially, it employs a method called tokenization, where the input text is broken down into smaller pieces, or tokens. This allows for a more granular analysis of the text, enabling the system to understand and categorize the content effectively. Each token is analyzed for its relevance, helping create a contextual framework that enhances the search experience.
Subsequently, ElasticSearch builds inverted indexes, which serve as a quick reference for matching queries with the stored data. This indexing method allows the engine to retrieve relevant documents swiftly, thus improving the overall search efficiency. By organizing the tokens in a way that maps them to their respective documents, ElasticSearch ensures that users receive accurate and timely insights, ultimately strengthening decision-making processes within organizations. Engaging in this systematic approach empowers businesses to extract meaningful trends and patterns from vast amounts of data.
The Role of Tokenization and Analysis
Tokenization and analysis play a crucial role in transforming raw text into actionable search engine insights. Tokenization, the process of breaking down text into individual terms or tokens, allows ElasticSearch to index and retrieve relevant data efficiently. By identifying key terms, it enhances a search engine’s ability to understand and interpret user queries, making information easily accessible.
Once tokenization occurs, analysis further examines these tokens to derive meaning. This involves filtering, stemming, and applying linguistic techniques to ensure accurate representation. Through this dual process, ElasticSearch creates refined indexes, significantly boosting search relevance. Understanding how tokenization and analysis work together can empower users to optimize their search strategies effectively, ensuring that they uncover valuable insights from the vast amounts of text data available.
Building Inverted Indexes for Efficient Searching
Inverted indexes are essential for optimizing search efficiency within ElasticSearch. By associating each word in a dataset with its corresponding document, these indexes enable fast retrieval of information. When a search query is made, the system quickly locates the relevant documents by referencing the inverted index, rather than scanning through all stored data. This process significantly reduces the time required for search operations, making it vital for effective text analytics.
To build an inverted index, follow these key steps: first, tokenize the text into individual terms. Next, create a mapping of these terms to their respective documents. Finally, organize the data in a way that facilitates rapid lookups. This indexing method enhances not only the speed but also the accuracy of search results. Adopting this approach allows businesses to gain critical insights, thereby transforming raw data into actionable intelligence for decision-making.
Generate Journey maps, Mind maps, Bar charts and more from your data in Minutes
Advanced Techniques in ElasticSearch Text Analytics
Incorporating advanced techniques in ElasticSearch text analytics significantly enhances the quality and depth of search engine insights. One pivotal approach is utilizing machine learning to process and analyze text data more effectively. By training models on historical data patterns, the system becomes adept at identifying trends and generating actionable insights. This intelligence aids in refining search results, making them more relevant to user queries.
Another essential technique involves implementing synonyms and autocomplete functionalities. This not only improves user experience but also broadens the spectrum of search results. When users input a query, the system can suggest variations or related terms, ensuring comprehensive coverage of relevant content. As a result, users receive a more enriched experience, driving engagement and satisfaction. Employing these advanced techniques significantly transforms the way text analytics operate within ElasticSearch, ultimately leading to more profound search engine insights.
Leveraging Machine Learning for Enhanced Search Engine Insights
Machine learning plays a crucial role in providing enhanced search engine insights by analyzing large volumes of data efficiently. By applying algorithms to text data, organizations can identify patterns, trends, and sentiments that would otherwise remain hidden. This capability allows for more nuanced search results tailored to user intentions, ultimately improving search relevance and user satisfaction.
Sentiment Analysis: Machine learning algorithms can be trained to evaluate user feedback, distinguishing between positive and negative sentiments. This insight helps guide product development and marketing strategies effectively.
Topic Modeling: By grouping similar content, machine learning aids in identifying the prevalent themes within a dataset. Understanding these topics assists in refining search queries and improving content discovery.
Predictive Analytics: Utilizing historical data, machine learning can forecast future trends and user behaviors. This information equips organizations with the ability to proactively adapt their strategies.
These techniques empower businesses to extract actionable insights, transforming raw data into valuable information for decision-making. Through machine learning, search engines can evolve, delivering deeper insights for users.
Implementing Synonyms and Autocomplete for Improved User Experience
Implementing synonyms and autocomplete functionality is essential for creating a seamless user experience in text analytics systems. When users search for a term, their assumptions about spelling or phrasing may vary. By incorporating synonyms, we enable the search engine to understand not just literal queries but also different expressions of the same intent. This translates to providing users with more meaningful and relevant results, significantly enhancing user satisfaction.
Autocomplete features streamline the search process by predicting user input as they type, reducing effort and time spent on queries. This functionality encourages exploration, as users can discover new relevant information they might not have originally sought. Together, these enhancements lead to increased engagement and retention, ultimately maximizing the potential of search engine insights through informed, user-centric search experiences.
Conclusion: Harnessing the Power of ElasticSearch for Optimal Search Engine Insights
ElasticSearch stands as a pivotal tool for businesses striving to extract valuable insights from their search engines. By harnessing its advanced features, organizations can transform their data into actionable intelligence. This capability enables users to delve deep into text analytics, thereby identifying patterns and extracting meaningful content that informs strategic decisions. With its user-friendly interface, even non-technical team members can contribute to uncovering these insights, fostering a culture of data-driven decision-making.
In conclusion, leveraging ElasticSearch empowers companies to enhance their understanding of user behavior and preferences. It enables businesses to not only optimize search functionality but also to gain a competitive edge in analysis and market research. Ultimately, harnessing the power of ElasticSearch for optimal search engine insights opens up new avenues for innovation and growth.