In the ever-evolving world of product development, understanding how features are perceived is essential. The introduction of innovative functionalities can sometimes lead to confusion for users, hindering their experience. Feature Confusion Detection becomes a critical process in identifying these points of friction during customer calls, allowing businesses to refine their offerings effectively.
Recognizing the signs of feature confusion in conversations is crucial for enhancing customer satisfaction. By analyzing customer support calls, companies can uncover recurring themes and misunderstandings related to specific features. This insight empowers product teams to address user concerns proactively, ensuring that every feature enhances rather than complicates the user experience.
Analyze qualitative data. At Scale.
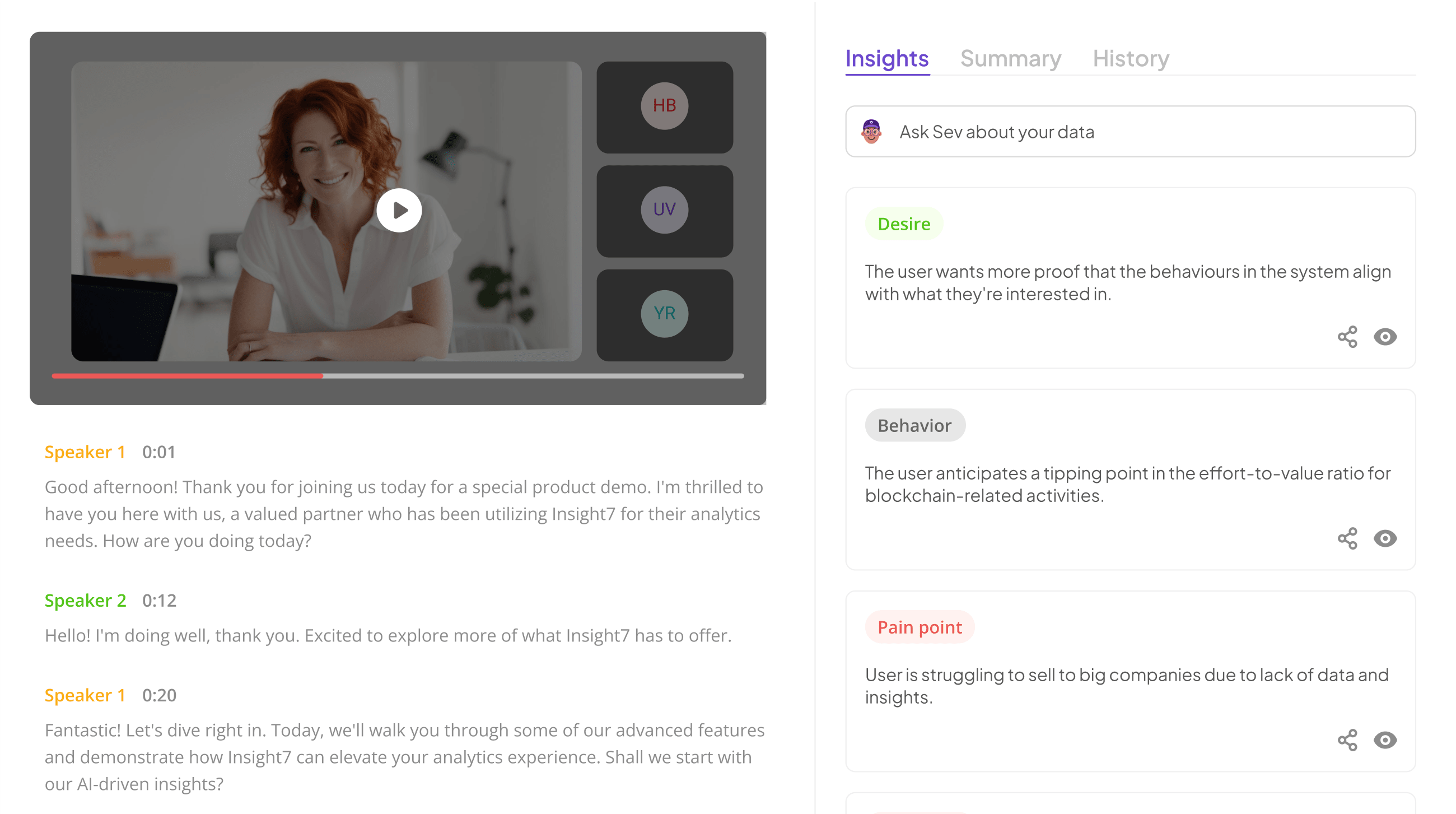
Identifying Feature Confusion in Customer Interactions
Identifying feature confusion in customer interactions is crucial for enhancing the user experience. Customers often struggle to understand complex products, leading to frustration and potential disengagement. By focusing on feature confusion detection, businesses can pinpoint where customers feel confused, unwittingly affecting their interaction with the product.
Common indicators of feature confusion include customers asking repetitive questions or displaying uncertainty about specific features. Listening to customer support calls can reveal significant patterns and moments of friction. Additionally, utilizing tools for data analysis can help in recognizing recurring themes in customer interactions. This allows organizations to address the root causes of confusion and streamline their offerings, ultimately improving overall satisfaction and loyalty. By refining communication around product features, businesses can transform confusion into clarity, paving the way for a more user-friendly experience.
The Role of Customer Support Calls in Feature Confusion Detection
Customer support calls play a critical role in feature confusion detection by providing direct insight into user experiences. When customers struggle to understand product features, their calls often reveal specific pain points and misunderstandings. These calls serve as rich data sources, allowing organizations to identify patterns of confusion that may not be apparent through surveys or usage data alone.
To effectively harness this resource, it's essential to analyze the content of customer interactions systematically. First, collect and transcribe the calls to create a searchable database of customer feedback. Next, employ algorithms designed for feature confusion detection to pinpoint recurring themes and issues. This process can reveal common indicators of confusion, highlighting areas where features may need clearer explanations or adjustments. By closely examining customer support calls, businesses can take proactive steps to enhance user experience and minimize friction.
Common Indicators of Feature Confusion in Conversations
In conversations, common indicators of feature confusion may surface, revealing that customers struggle with understanding product features. Listening for hesitations or repeated requests for clarification can highlight moments of confusion. When customers frequently respond with phrases like โI donโt quite get that" or "Can you explain this again?โ it signals a lack of clarity and potential friction in their interaction.
Another sign of feature confusion involves the customer's questions reflecting misunderstandings of the product's purpose. For instance, if users inquire about features that are already included, it implies they might be unaware of the productโs functionalities. Tracking these signs is crucial for effective feature confusion detection, as it can guide teams in refining product communication and enhancing user experience. Addressing these complexities early on can significantly reduce customer frustrations and improve satisfaction over time.
Extract insights from interviews, calls, surveys and reviews for insights in minutes
Analyzing Calls for Friction Points: A Step-by-Step Guide
To effectively analyze calls for friction points, begin by collecting and transcribing customer interactions. This foundational step ensures you have accurate records to dissect, allowing for a clearer understanding of feature confusion detection. Transcriptions can be enhanced through various tools, making it easier to pinpoint troublesome areas in product features that may perplex users.
Next, leverage feature confusion detection algorithms to identify patterns in the transcribed data. These algorithms can highlight recurring issues, customer sentiments, and specific phrases indicating confusion. Finally, thoroughly analyze the identified patterns to generate actionable insights. Recognize trends that consistently appear, such as specific features causing disputes or misunderstandings. This structured approach not only clarifies which areas need improvement but also enriches the overall user experience by resolving friction points effectively. By following these steps, you can systematically reduce customer confusion and reinforce product understanding.
Step 1: Collect and Transcribe Customer Calls
To effectively identify feature confusion among your customers, the first step involves collecting and transcribing customer calls. This essential task allows you to gather direct feedback regarding your product's features and how users interact with them. By capturing these conversations, you not only preserve valuable insights but also set the stage for deeper analysis of feature-related issues.
During this phase, ensure that you utilize reliable transcription tools to convert spoken dialogue into written format. This clarity will facilitate a thorough examination of user concerns and confusion surrounding specific features. As you transcribe calls, flag instances where customers express uncertainty; this data will be vital in pinpointing areas where confusion occurs. Collecting and transcribing customer calls is not just about accumulating information; itโs about understanding user experiences to address friction and enhance overall satisfaction in your productโs offerings.
Step 2: Implement Feature Confusion Detection Algorithms
Implementing Feature Confusion Detection Algorithms is critical for identifying friction points within product features. This step begins with the collection of data from customer interactions, particularly support calls. Advanced algorithms are employed to analyze conversational data, highlighting areas where customers express confusion or frustration. By applying these algorithms, organizations can pinpoint specific features leading to customer misunderstandings and adapt their messaging accordingly.
These algorithms typically function by examining patterns in linguistic cues, emotional tone, and contextual relevance in conversations. For instance, identifying repeated questions about certain features can reveal a lack of clarity. Additionally, integrating tools like Insight7 enhances the efficiency of this analysis, equipping businesses with insights to refine their offerings. Ultimately, the goal of implementing these algorithms is to streamline user experience, ensuring that product features are communicated clearly and effectively to the customer.
Step 3: Analyze Patterns and Trends in Confusion
Analyzing patterns and trends in confusion is a crucial step in addressing product feature misunderstanding. This analysis involves meticulously reviewing customer interactions to identify recurring themes that point to friction. Begin by examining customer support calls to determine how often confusion arises about specific features. Look for repeated phrases, questions, or expressions of frustration that can signal a lack of clarity.
Next, categorize these findings based on common traits. For instance, categorize confusion related to functionality versus confusion due to terminology. Understanding these distinctions can help pinpoint whether the issue stems from misunderstanding how a feature works or a lack of clear communication about it. By systematically analyzing these patterns, organizations can enhance their product education efforts and create more intuitive user experiences, leading to increased customer satisfaction.
In summary, focusing on analyzing trends allows for proactive measures to prevent confusion. As you iterate through this process, remain attentive to changes in customer feedback, as they can provide insights into evolving perceptions of product features.
Top Tools for Feature Confusion Detection
Effective tools for Feature Confusion Detection can help identify moments of misunderstanding during customer interactions. By using advanced analytics, businesses can trace back the points of confusion across product features in support calls. Primary tools include Insight7, Gong, and Chorus.ai, each designed to assist businesses in gaining clarity from customer conversations.
Insight7: This tool specializes in analyzing quantitative data and transcribing customer feedback, allowing you to uncover confusion patterns in real-time.
Gong: Known for its AI-driven analytics, Gong can evaluate the tone and sentiment of calls, helping to pinpoint areas where customers struggle to grasp product features.
Chorus.ai: Similar to Gong, this platform provides insights by recording calls and analyzing conversations for confusion-driving keywords and phrases.
CallRail: With its call tracking capabilities, CallRail enables teams to review conversations and make informed adjustments to product messaging.
Talkdesk: This cloud-based solution integrates various communication channels, allowing teams to monitor confusion indicators across multiple platforms seamlessly.
By employing these tools, organizations can proactively address confusion related to product features, ultimately improving overall customer satisfaction.
insight7
Detecting feature confusion during customer interactions is essential for enhancing user experience. By employing straightforward techniques, you can identify when customers struggle to comprehend or navigate product features. This confusion can stem from insufficient explanations or overly complex functionalities, highlighting the need for clarity in your communication.
To effectively implement Feature Confusion Detection, consider the following steps. First, collect and transcribe customer support calls to have a clear record of interactions. Next, utilize specialized algorithms that analyze these conversations for signs of confusion or misunderstanding. Analyzing these patterns allows teams to pinpoint specific features that generate friction, leading to targeted improvements in product design and communication strategies. By paying close attention to how customers interact with your services, you can create a user-friendly experience that addresses their needs and enhances satisfaction.
Gong
Monitoring customer interactions through calls reveals key insights into Feature Confusion Detection. Gong acts as an essential tool, providing an avenue to analyze recorded calls for recurring patterns of confusion surrounding product features. By transcribing conversations, teams can pinpoint areas where messaging fails, allowing for immediate adjustments.
In practice, Gong provides real-time analytics that highlight specific moments of misunderstanding. These insights empower product teams to refine their offerings based on customer feedback. Effectively navigating these complex interactions helps organizations uncover friction points, streamlining processes to enhance user experience. By prioritizing this analysis, companies can make informed adjustments, ultimately leading to a more straightforward and user-friendly product experience. Understanding where confusion occurs allows businesses to evolve their services in alignment with customer needs, reducing friction and enhancing satisfaction.
Chorus.ai
In the realm of identifying feature confusion during customer interactions, harnessing technology plays a significant role. As conversations unfold, utilizing advanced analysis tools can help detect distinct patterns of confusion among users. This is where effective communication becomes key; understanding how customers perceive product features allows teams to address friction points proactively.
With specialized algorithms, feature confusion detection can analyze recorded calls and highlight when customers express uncertainty or frustration. By transcribing these conversations, businesses can pinpoint specific features that may be causing misunderstandings. Such insights allow for targeted improvements in product communication, minimizing confusion and enhancing user satisfaction. Addressing these pain points ultimately aids in refining the customer experience, making it smoother and more enjoyable for all involved.
CallRail
Customer interactions often reveal critical insights into potential confusion around product features. CallRail serves as a powerful component in detecting feature confusion by recording and analyzing customer calls. By reviewing these conversations, product teams can pinpoint friction points that may stem from unclear features or documentation.
This tool enables businesses to sift through large volumes of call data efficiently. With CallRail, teams can identify patterns of confusion linked to specific features. Analysis of these conversations supports the objective of enhancing user experiences, ensuring features are both effective and user-friendly. Implementing this strategic approach to feature confusion detection fosters a clearer understanding between customers and products, ultimately driving better engagement and satisfaction.
Additionally, it's essential to consider other tools such as Gong and Chorus.ai to complement CallRail's capabilities. These tools further enhance the ability to analyze call data for insights, ensuring a comprehensive understanding of customer needs.
Talkdesk
Customer support calls are a pivotal resource for understanding user experience. During these interactions, it becomes crucial to detect feature confusion, which can lead to customer frustration. By leveraging call transcripts and analyzing customer dialogues, organizations can pinpoint moments of misunderstanding or miscommunication regarding product features. This not only sheds light on areas requiring improvement but also aids in adjusting messaging and user guidance.
The process of feature confusion detection involves identifying recurring patterns in customer calls. For instance, if multiple callers express confusion about a specific feature, it indicates a need for clearer documentation or tutorials. Additionally, employing tools such as Insight7 can enhance the analysis, allowing for a more structured evaluation of customer feedback. Through careful examination of these calls, companies can effectively reduce friction points, ultimately leading to a smoother user experience and increased customer satisfaction.
Conclusion: Streamlining Feature Confusion Detection to Improve User Experience
Improving user experience hinges on effectively streamlining Feature Confusion Detection within product interactions. By identifying specific areas where customers experience confusion, businesses can create a more intuitive environment. Focusing on these confusion points allows for targeted improvements, ultimately leading to a seamless user journey.
To achieve this, companies must employ robust methodologies for analyzing customer interactions, particularly via support calls. By combining advanced detection algorithms with thorough pattern analysis, organizations can pinpoint friction areas. This approach not only enhances clarity around features but also fosters user satisfaction through a more accessible and user-friendly experience.