Multilingual AI Performance models are becoming increasingly vital as businesses expand their reach across diverse linguistic markets. The need for efficient and effective language processing tools is more pressing than ever. In this context, these models not only enhance user experience but also drive engagement and satisfaction in multilingual settings.
With the ongoing globalization of markets, understanding how to optimize AI for various languages is essential. However, the journey involves navigating through various obstacles, such as data scarcity and algorithmic bias. This section lays the groundwork for exploring the fundamental aspects of multilingual AI performance models, equipping stakeholders with the knowledge needed to design robust, inclusive systems that meet diverse language needs.
Analyze qualitative data. At Scale.
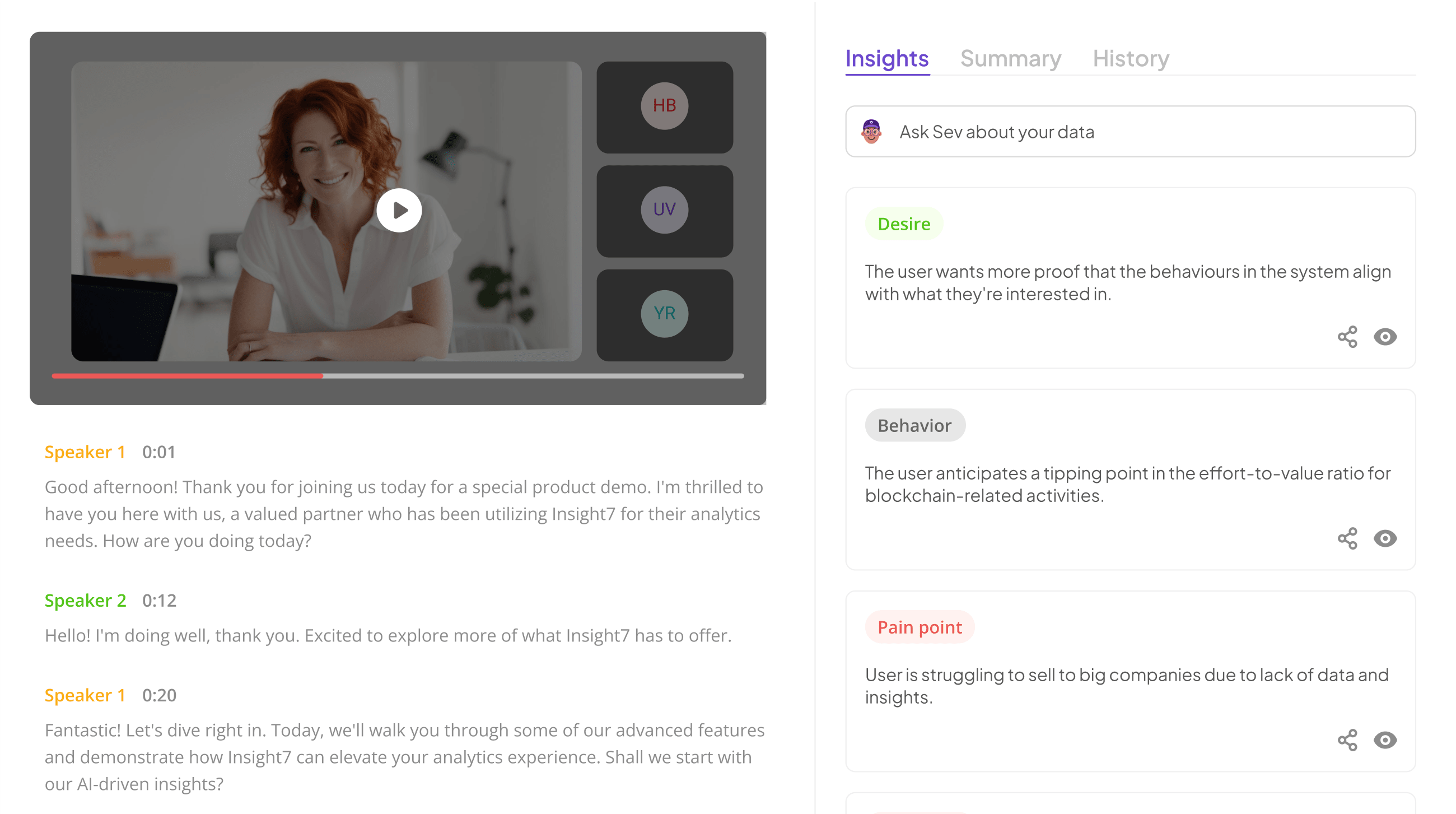
Understanding the Basics of Multilingual AI Performance
Multilingual AI Performance refers to the ability of artificial intelligence systems to understand and process multiple languages efficiently. This includes tasks such as translation, sentiment analysis, and speech recognition across diverse languages, ensuring seamless communication in a global context. A robust multilingual AI system should not only understand the syntax of different languages but also grasp cultural nuances, idioms, and context-specific interpretations.
Understanding how multilingual AI optimally functions requires addressing key aspects. First, different languages present unique challenges due to variations in structure and grammar. Second, ensuring consistent performance across languages depends on diverse training data that accurately represents these languages. Finally, evaluating the performance of multilingual models involves metrics that assess accuracy, fluency, and user satisfaction, making it essential to adopt a user-centric approach for continuous improvement. Mastering these principles is crucial for developing an effective AI model tailored to multilingual support.
What is Multilingual AI Performance?
Multilingual AI Performance refers to the ability of artificial intelligence systems to understand and process multiple languages effectively. This capability is crucial in an increasingly globalized world where diverse linguistic backgrounds present both opportunities and challenges. To succeed, these systems must not only translate text accurately but also comprehend cultural nuances and context, enhancing user experiences regardless of language.
In the realm of AI, performance is measured by accuracy, efficiency, and adaptability. It involves various methodologies, from natural language processing to machine learning techniques. A robust multilingual AI system should ensure that users receive seamless interactions despite language barriers. This includes interpreting intents, meaning, and context, thus providing responses that feel personalized and relevant. By focusing on these elements, organizations can design performance models that cater to audiences across linguistic divides, ultimately fostering better engagement and satisfaction.
Key Challenges in Designing Multilingual Models
Designing multilingual models presents several key challenges that can significantly impact Multilingual AI Performance. First, the diversity of languages requires comprehensive data that accurately represents linguistic nuances. Unlike monolingual systems, multilingual models must understand various dialects, colloquialisms, and syntactical structures. This complexity can hinder the modelโs ability to generate relevant and contextually appropriate responses.
Second, achieving high-quality translations is a formidable task. The intricacies of meaning in one language often do not have direct counterparts in another, leading to potential ambiguity or loss of context. Furthermore, there exists a need for cultural sensitivity in communication, which adds another layer to the design process. These challenges underscore the necessity for a robust framework that can integrate localized content while maintaining overall performance across multiple languages. Addressing these complexities is paramount for creating effective and reliable AI solutions that cater to a global audience.
Building an AI-Based Performance Model for Multilingual Support
Creating an effective AI-based performance model for multilingual support begins with understanding language requirements. When developing a system, itโs essential to identify which languages will be supported to meet user needs effectively. This ensures that the model can function accurately across diverse linguistic contexts. Next, selecting appropriate datasets plays a critical role in training the model. Data should reflect the nuances of each language involved, capturing regional dialects and cultural contexts.
Following this, developing language-independent algorithms enhances the system's ability to operate seamlessly across languages. These algorithms help ensure that the AI can produce reliable outputs, regardless of linguistic differences. Ultimately, these steps aim to elevate multilingual AI performance, creating a robust framework for interaction that accommodates various languages and dialects while ensuring optimal user experience. By focusing on these foundational aspects, organizations can better cater to their multilingual audiences, fostering broader engagement and satisfaction.
Extract insights from interviews, calls, surveys and reviews for insights in minutes
Step-by-Step Guide to Designing an Effective Model
Designing an effective model for multilingual AI performance begins with a clear understanding of your specific needs and challenges. Start by identifying the languages your application will support. This foundational step shapes the entire model, ensuring that you address the unique nuances of each language involved. Once your language requirements are set, the next crucial phase is selecting appropriate datasets. Rich, diverse datasets enhance the modelโs ability to understand and generate text across multiple languages, reflecting real-world usage.
Next, focus on developing language-independent algorithms. These algorithms facilitate seamless performance across various languages, minimizing potential biases that could arise from language-specific training. By following these steps, you can create an effective multilingual AI performance model that meets the diverse needs of your users while maintaining high quality and reliability. Each phase is interlinked, forming a comprehensive approach to support robust multilingual capabilities in your AI systems.
- Step 1: Identifying Language Requirements
To ensure optimal Multilingual AI Performance, identifying language requirements is a critical first step. Start by determining the primary languages your model must support based on user needs, regional presence, and market demand. Conducting a comprehensive analysis of your target audience will illuminate the specific languages that require focus.
Next, document dialects, regional variations, and cultural contexts associated with each target language. This is vital as subtle linguistic differences can impact user experience significantly. Additionally, engage with language experts or collaborate with native speakers to validate your findings. These actions create a robust foundation, aligning the AI model's capabilities with real-world user interactions, thereby enhancing overall effectiveness and reliability. Ensuring that language requirements are meticulously identified lays the groundwork for a successful multilingual support system that meets diverse user needs.
- Step 2: Selecting Appropriate Data Sets
Selecting appropriate data sets is crucial in building an effective AI-based performance model for multilingual support. Firstly, it's essential to consider the diversity of languages represented in your data. The selected data sets should encompass various dialects, regional variations, and cultural contexts to ensure the model's accuracy and reliability. A comprehensive collection of data helps your multilingual AI performance by capturing nuances in language use.
Secondly, itโs important to evaluate the quality and size of the data sets. High-quality, well-annotated data allows for better training outcomes. Aim for a mixture of structured and unstructured data to address different aspects of multilingual communication. Finally, keep in mind the ethical considerations surrounding data selection. Ensuring that your data set is free from bias and respects privacy will enhance the trustworthiness of your model. By carefully curating the right data sets, you lay the foundation for a multilingual AI that meets the needs of diverse user groups.
- Step 3: Developing Language-Independent Algorithms
Developing language-independent algorithms is a critical aspect of achieving multilingual AI performance. To design an effective performance model, it is essential to create algorithms that function seamlessly across various languages. These algorithms should focus on core functionalities instead of language-specific nuances, ensuring that the AI can perform optimally regardless of the userโs preferred language.
Key elements in this developmental phase include abstraction, data normalization, and modular design. Abstraction allows the system to handle language particularities without compromising functionality. Data normalization ensures consistent input across different languages, enabling more accurate processing. Finally, a modular design promotes flexibility and scalability, allowing for easier updates and enhancements. Incorporating these principles into your algorithms will greatly improve the multilingual capabilities of your AI model, making it more efficient and user-friendly across diverse audiences.
Tools and Technologies for Enhancing Multilingual AI Performance
To enhance multilingual AI performance, various tools and technologies play a crucial role in shaping effective solutions. These tools help bridge language gaps, improving AI's ability to understand and generate human-like responses across languages. Key technologies include natural language processing (NLP) frameworks, which allow AI systems to analyze and interpret human languages, enhancing contextual understanding. Additionally, translation services, such as Google Translate and Amazon Translate, offer dynamic multilingual capabilities that integrate seamlessly into applications.
Another essential technology is machine learning, which allows AI models to learn from multilingual datasets. This enables continuous improvement and adaptation to various linguistic nuances. Furthermore, utilizing cloud-based AI services can provide scalability and flexibility, ensuring performance remains optimal as demand increases. By implementing these tools and technologies, organizations can significantly enhance their multilingual AI performance, ultimately leading to better user experiences and broader accessibility across diverse languages.
- insight7: Overview and Capabilities
The insight7 section delves into the Overview and Capabilities of our platform, focusing on Multilingual AI Performance. Here, ease of use and accessibility stand out as core features. Users can interact with the platform without requiring specialized training. This democratization allows anyone in the organization to access valuable insights seamlessly.
Key capabilities include the ability to analyze diverse language datasets efficiently. Users can transcribe calls, visualize conversations, and social interactions effortlessly within the platform. Insight generation focuses on various dimensions such as pain points and customer desires, equipped with evidence supporting each insight. This structured approach not only aids in identifying friction points but also enhances understanding of customer experiences within multilingual contexts. Ultimately, this empowers teams to make informed decisions and elevate customer satisfaction effectively.
- Google Translate API: Key Features and Use Cases
The Google Translate API offers valuable features that contribute significantly to multilingual AI performance models. Its real-time translation capability supports over a hundred languages, making it a vital tool for businesses targeting diverse markets. The API provides seamless integration into applications, enabling users to communicate efficiently across language barriers. Additionally, with features like automatic language detection and extensive vocabulary support, it enhances the user experience by providing accurate translations in context.
In terms of use cases, organizations utilize the API for customer support, content localization, and data analysis. For instance, businesses can quickly translate user inquiries to improve support services, ensuring timely and accurate responses. Furthermore, developers harness the API for real-time translation in apps, enhancing user interaction. By incorporating these features into multilingual AI systems, businesses can optimize performance and create user-centric solutions that foster global engagement.
- Microsoft Azure Cognitive Services: Multilingual Support Features
Microsoft Azure Cognitive Services offers robust multilingual support features that enhance the capabilities of AI-based performance models. These services include advanced text analytics and language understanding, which enable businesses to engage effectively with diverse audiences. By incorporating such features, organizations can ensure superior multilingual AI performance, which is crucial for global outreach.
One of the standout aspects is its capability to identify and translate various languages seamlessly. This enables real-time communication and understanding, making it easier to manage multilingual content. Moreover, features like text translation and sentiment analysis help organizations gain insights into customer perceptions across different cultures. Overall, harnessing these multilingual support features leads to more effective interactions and improves user satisfaction, significantly benefiting any global initiative.
- IBM Watson Language Translator: Benefits and Implementation
The implementation of advanced translation technology offers substantial benefits for enhancing multilingual AI performance. A significant advantage lies in its ability to facilitate seamless communication across different languages. By integrating effective translation solutions, organizations can improve their global reach and customer experience. Additionally, such technology enables businesses to analyze and evaluate conversations in multiple languages, leading to better insights for quality assurance and compliance evaluations.
Furthermore, the implementation process often involves a few critical steps. First, assessing the specific language requirements is essential, helping to define the scope of the translation features needed. Next, selecting appropriate data sets ensures that the translation models are accurate and relevant. Finally, developing language-independent algorithms allows for consistency in performance across various languages, significantly enhancing overall AI capabilities. By focusing on these areas, organizations can create a robust multilingual environment that perfectly aligns with their business goals.
- Amazon Translate: Integration and Performance
Effective integration of multilingual AI tools into workflows can significantly enhance overall performance. When considering a performance model, it's crucial to recognize how a reliable translation service can streamline communication across diverse languages. This integration not only improves user experience but also allows businesses to engage international markets more effectively.
To ensure optimal performance of multilingual AI in translation, the following factors should be considered:
Ease of Integration: The translation tool should seamlessly integrate with existing systems, eliminating barriers for users.
Accuracy and Context Understanding: Effective models use advanced algorithms to ensure translations retain contextual meaning rather than just converting text verbatim.
Real-time Processing: Quick translation capabilities are vital, particularly for businesses that require immediate communication across various languages.
Scalability: A robust model should support an increasing number of languages and user requests without compromising performance.
By focusing on these key factors, businesses can elevate their multilingual AI performance, driving communication efficiency and fostering global growth.
Conclusion on Multilingual AI Performance Models
As we conclude our exploration of multilingual AI performance models, it's clear that these systems offer significant promise for enhancing communication across diverse languages. By effectively integrating multilingual AI performance into various applications, businesses can foster better engagement and inclusivity among users. This increased accessibility can lead to improved customer satisfaction and loyalty.
To maximize the potential of multilingual AI performance, it is crucial to focus on continuous optimization of algorithms and careful selection of training data. Investing in advanced technologies and methodologies ensures that these models remain accurate and effective. Ultimately, the evolution of multilingual AI performance will play a vital role in bridging linguistic divides and enriching user experiences globally.