Introduction to R&D Data Insights begins with the understanding that every piece of data holds the potential to drive innovation. In the research and development sector, insights gleaned from data analysis can revolutionize processes and product outcomes. These insights are not just byproducts; they serve as essential components for informed decision-making, enabling teams to push boundaries and achieve their goals.
Effective utilization of R&D Data Insights requires a strategic approach. Organizations must first recognize the vital role that data plays in their processes. By identifying key metrics, teams can ensure that they not only gather relevant information but also interpret it accurately. The transformation of complex data sets into actionable insights is crucial, as it guides teams toward making data-driven decisions that enhance productivity and innovation.
Generate visualizations from your qualitative data. At Scale.
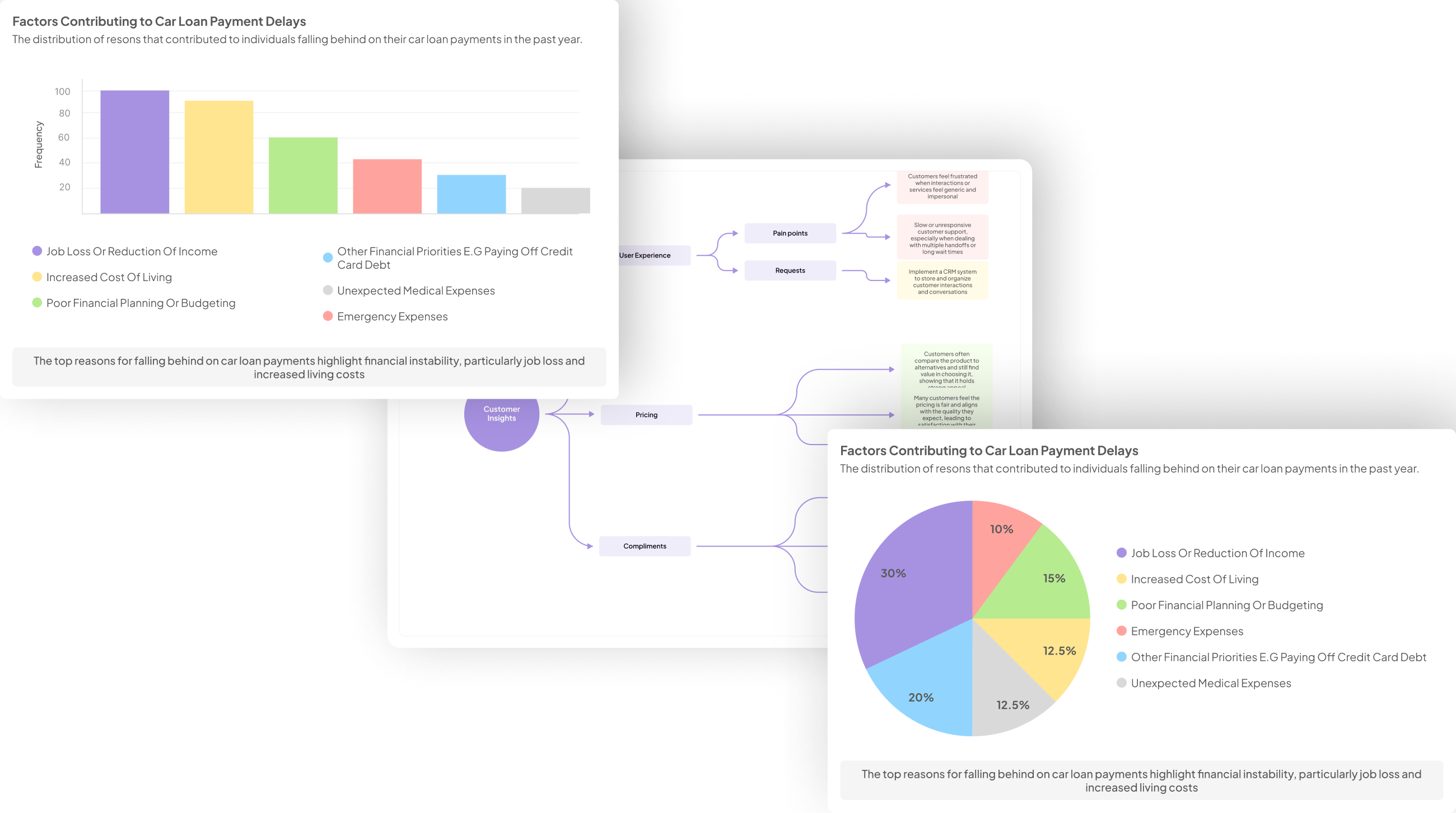
Leveraging R&D Data Insights in Development Processes
R&D Data Insights can significantly enhance development processes by uncovering key patterns and trends within data. Understanding these insights allows teams to make informed decisions that drive innovation. For instance, analyzing customer feedback can reveal preferences that inform product design and features, leading to a better alignment with market demands.
To effectively integrate R&D Data Insights into development practices, organizations should focus on three main aspects. First, establish a process for systematically collecting and managing data to ensure accuracy and relevance. Second, develop frameworks for analyzing and interpreting this data, allowing teams to identify meaningful trends. Lastly, actively incorporate findings into strategic planning, facilitating a cycle of continuous improvement that translates insights into actionable developments. By embracing these steps, teams can foster a culture of data-driven decision-making that fuels ongoing success.
Understanding Datas Role in R&D
Data plays a critical role in the R&D process, helping organizations drive innovation and optimize product development. R&D Data Insights offer a comprehensive understanding of market needs, consumer preferences, and technological advancements. By systematically gathering and analyzing relevant data, teams can unearth patterns that inform strategic decisions and enhance project outcomes.
For effective application, R&D should embrace the following components:
I. Data Sources: Use multiple data sources to capture a holistic view. This includes customer feedback, market trends, and competitive analysis.
II. Analysis Techniques: Employ diverse analytical methods such as statistical analysis, machine learning, and visualization tools. This aids in interpreting complex data sets effectively.
III. Continuous Feedback: Implement ongoing feedback loops to refine products and processes. Engaging stakeholders at every stage ensures that insights are actionable and innovations remain aligned with market demands.
By recognizing and harnessing the power of data in R&D, organizations can not only foster creativity but also ensure that their innovations meet the evolving needs of their users.
Identifying Key Metrics for Innovation
Identifying key metrics for innovation begins with understanding what aspects of R&D are critical to your goals. Innovators must look beyond basic performance indicators and focus on metrics that provide meaningful insights into their processes. These metrics should correlate closely with desired outcomes, such as efficiency, product quality, and market responsiveness. By focusing on these areas, organizations can leverage R&D data insights to inform decision-making.
To effectively capture relevant metrics, consider the following aspects:
Innovation Output: Measure the rate of new products or features launched. This reflects the creativity and productivity of your teams.
Time to Market: Track how quickly ideas transition from concept to launch. Shortening this time can lead to a competitive edge.
Customer Feedback: Utilize qualitative and quantitative data from customers to assess satisfaction and areas for improvement.
Resource Utilization: Evaluate how effectively resourcesโboth people and materialsโare used during the R&D process.
Collaboration Metrics: Assess the degree of cross-functional collaboration. This encourages diverse perspectives and drives innovation.
Examining these metrics will help organizations better understand their innovation landscape and support informed decisions in the R&D sphere.
Generate Journey maps, Mind maps, Bar charts and more from your data in Minutes
R&D Data Insights: Actionable Steps for Effective Implementation
To successfully implement R&D data insights, organizations must prioritize effective data collection and management. Start by establishing a robust system to gather relevant data, which includes customer queries, feedback, and performance metrics. This foundational step enables more informed decisions and strategy formulation. Maintaining organized data is vital, as it lays the groundwork for subsequent analysis and improves access for all team members.
Next, analyzing the gathered data is crucial. Utilize analytical tools to interpret trends and identify actionable insights. An effective analysis not only uncovers opportunities for innovation but also highlights areas needing improvement. Finally, turning insights into action entails developing targeted strategies based on these findings. This culminates in a systematic approach to implementing changes that drive operational efficiency and support ongoing innovation in R&D efforts. By diligently following these actions, organizations can harness the full potential of R&D data insights for effective implementation.
Step 1: Data Collection and Management
Data collection and management form the foundation of R&D Data Insights, ensuring that relevant information is gathered accurately and efficiently. Initially, identifying reliable data sources is vital; this can include customer feedback, market research, and internal records. Engaging in a systematic approach to collect these data sets facilitates better insights down the line. When gathering data, it's essential to maintain accuracy and consistency to enable effective analysis later in the process.
Furthermore, organizing and storing the collected data is equally significant. Utilizing data management systems can help categorize information, making it easier to access and analyze. Regularly updating the database ensures it remains relevant and reflects current trends. By establishing a solid framework for data collection and management, researchers enhance their ability to extract actionable insights that will inform strategic development decisions.
Step 2: Analyzing and Interpreting Data
In Step 2: Analyzing and Interpreting Data, the goal is to transform raw information into valuable R&D data insights. Start by identifying patterns and correlations within the dataset, which can lead to meaningful conclusions. Techniques such as data visualization help to illustrate trends, making data more accessible and understandable. This active interpretation phase is crucial, as it allows teams to focus on significant feedback while filtering out noise from the data.
Once the analysis is complete, synthesizing these insights into actionable strategies is essential. Assess which data points impact product development and innovation the most. By continuously evaluating and refining these insights, researchers can ensure their findings inform critical decisions effectively, driving the R&D process forward. Frequent revisiting of the data enhances understanding and fosters a culture of data-driven decision-making. This iterative approach is key to nurturing innovation and achieving successful outcomes in research and development.
Step 3: Turning Insights into Action
Incorporating R&D data insights into actionable plans requires a strategic approach. First, itโs essential to clearly define objectives based on analyzed data. This ensures that insights from research align with specific development goals. Engaging relevant stakeholders early in this process is crucial. Their input can refine the focus and enhance the success of action plans.
Next, prioritize insights that have the potential to drive innovation. This may include identifying areas for product development, process improvements, or customer engagement strategies. Once prioritized, create action plans that outline specific steps, assigned responsibilities, and timelines. Implementing feedback mechanisms can also enhance the process, allowing teams to adjust plans based on real-time data and evolving market conditions. Ultimately, turning insights into effective actions is an iterative journey that can lead to substantial improvements in R&D outcomes.
Conclusion: Integrating R&D Data Insights for Continued Success
Integrating R&D Data Insights into operational strategies is crucial for maintaining success in today's competitive landscape. As organizations increasingly rely on data to inform their decisions, understanding how to interpret these insights becomes essential. By focusing on key metrics and analysis methods, teams can foster innovation and enhance product development.
The process doesn't end with data analysis; it also involves creating a feedback loop to refine strategies continually. Emphasizing ongoing integration of R&D Data Insights allows organizations to adapt to market changes and customer demands effectively. This alignment will pave the way for sustained growth and improved outcomes in future projects.
Generate visualizations from your qualitative data. At Scale.
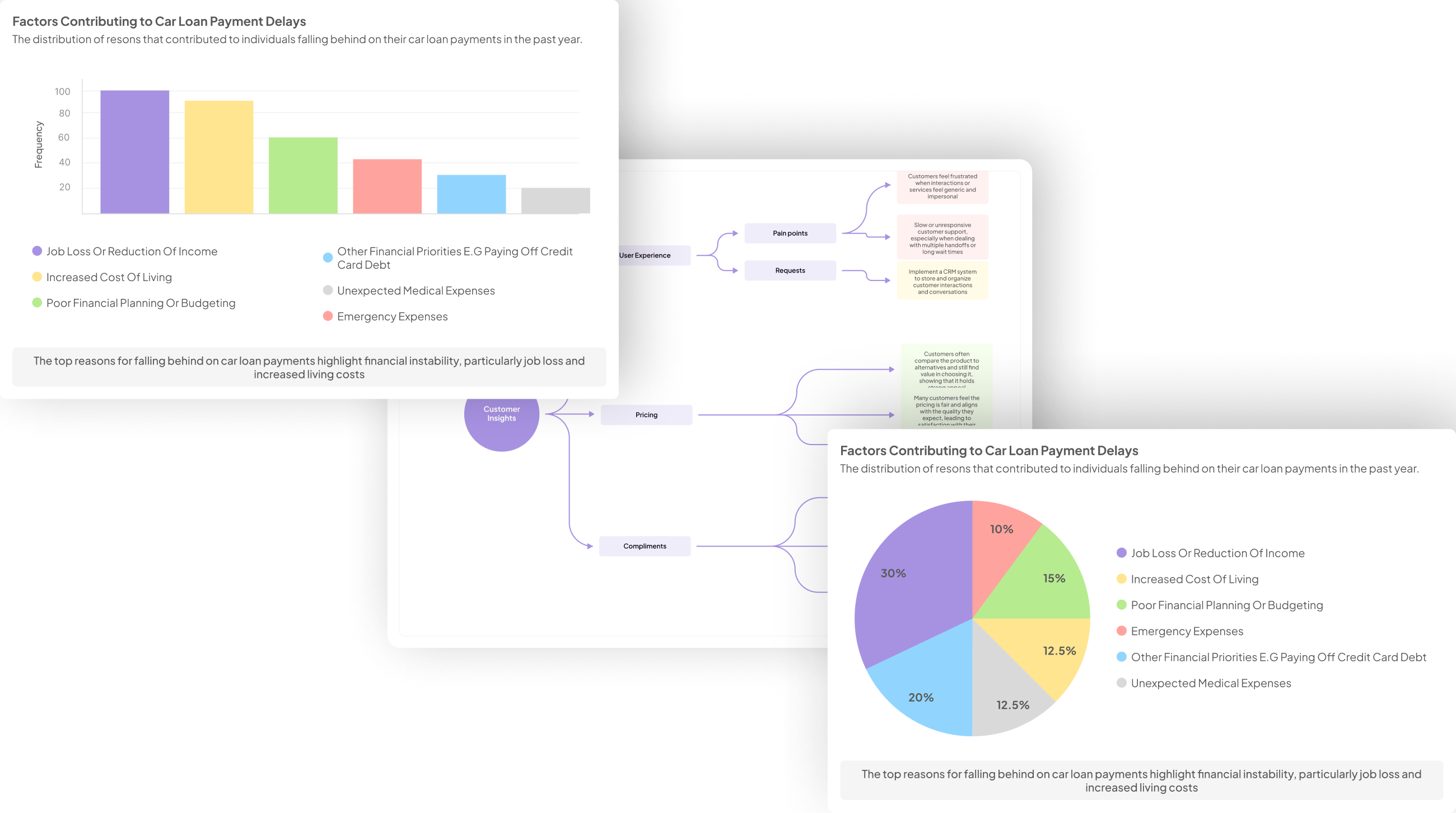