Customer retention is a core challenge for many businesses today, as losing customers can significantly impact revenue and growth. In this context, churn prediction strategies have emerged as crucial tools for understanding and mitigating customer attrition. By implementing effective strategies, organizations can identify at-risk customers early and take proactive measures to retain them.
Effective churn prediction begins with comprehensive data analysis that reveals customer behavior patterns and preferences. Strategic approaches involve selecting relevant metrics and predictive models tailored to a business's unique needs. By focusing on these strategies, businesses can not only predict churn but also enhance customer loyalty and satisfaction, ultimately driving long-term success.
Generate visualizations from your qualitative data. At Scale.
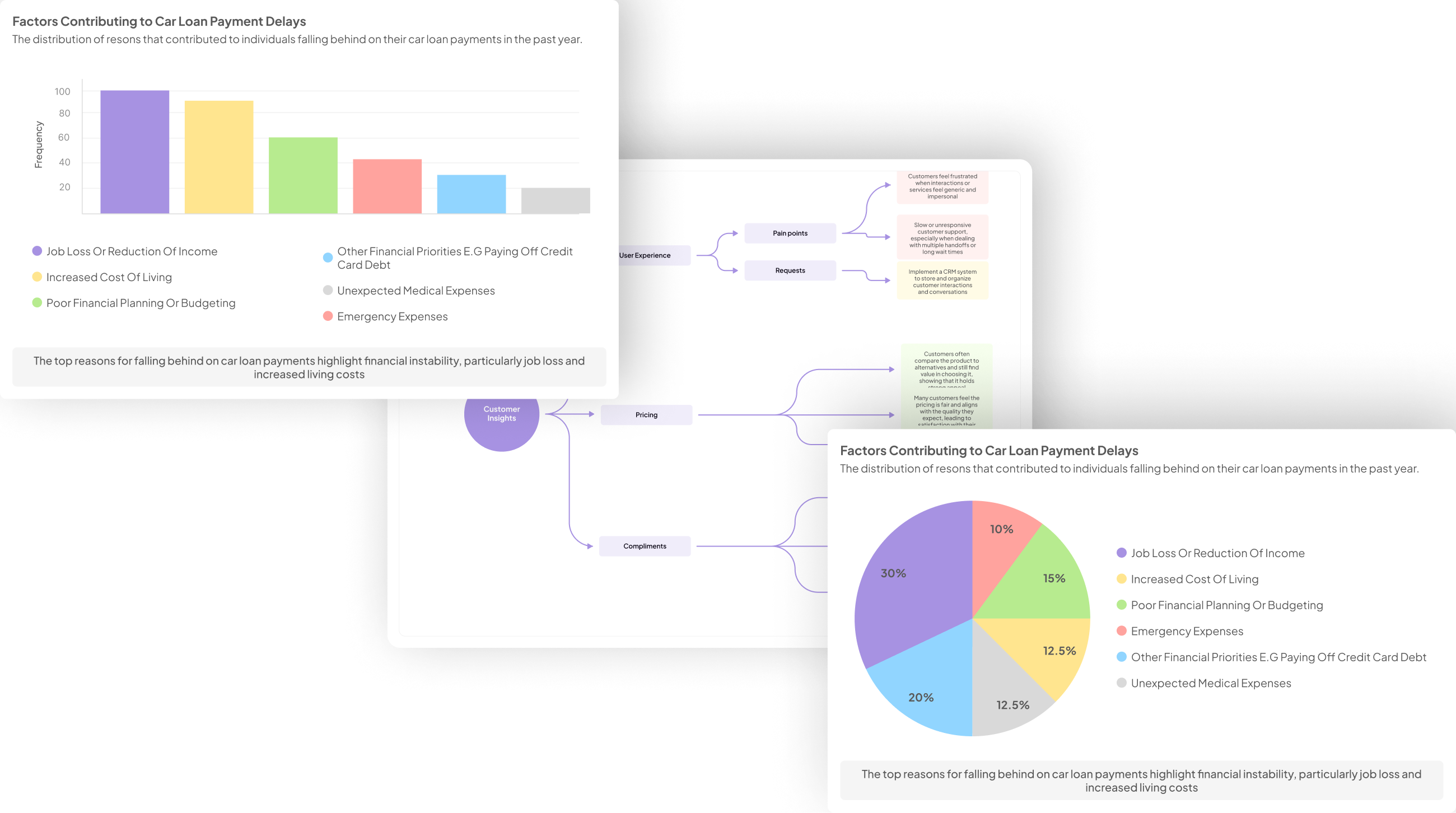
Understanding Churn Prediction Strategies
Understanding churn prediction strategies is essential for businesses aiming to retain customers and enhance their growth. Churn prediction involves analyzing customer data to identify patterns that signal potential disengagement. These insights allow organizations to implement targeted interventions that address customer concerns before they decide to leave. By focusing on customer behavior, businesses can better anticipate their needs and preferences, which leads to improved satisfaction and loyalty.
There are several core strategies to consider. First, employing advanced data analysis techniques can help uncover hidden factors contributing to churn. This might include customer demographics, purchase history, and interaction patterns. Additionally, utilizing machine learning models enables predictive insights that evolve with customer behavior. Finally, implementing customer feedback loops allows businesses to stay attuned to changing customer sentiments and expectations. Tailoring these strategies to the business's unique context ensures that intervention efforts are both relevant and effective.
Importance of Churn Prediction in Business
Understanding churn prediction is essential for businesses aiming to enhance customer retention and satisfaction. By identifying potential churners, companies can proactively engage these customers, addressing their concerns before they decide to leave. This strategic foresight allows businesses to cultivate loyalty and improve overall customer experiences.
Key metrics, such as Customer Lifetime Value (CLV) and Net Promoter Score (NPS), play a critical role in churn analytics. These indicators help quantify the effectiveness of customer retention efforts and pinpoint areas for improvement. Moreover, by monitoring these metrics over time, organizations can establish lasting relationships with their clientele.
Employing churn prediction strategies not only mitigates the risk of losing valuable customers but also fosters a customer-centric business model. In an increasingly competitive landscape, understanding and acting on churn insights can be the difference between thriving and merely surviving. Hence, investing in churn prediction analytics is indispensable for sustainable business growth.
- Discuss why understanding customer churn is vital for businesses.
Understanding customer churn is critical for any business seeking growth and sustainability. When customers leave, it significantly impacts revenues and profitability, making it important to identify the root causes behind churn. By implementing churn prediction strategies, businesses can proactively address these issues, ultimately improving customer retention and loyalty. Knowing why customers disengage allows companies to adapt their offerings and enhance the overall customer experience.
Moreover, understanding customer churn helps in resource allocation and strategic planning. By analyzing churn data, businesses can identify at-risk customers and develop targeted interventions to retain them. They can fine-tune marketing efforts, personalize customer interactions, and create loyalty programs that resonate with their audience. This data-driven approach not only decreases churn but also fosters long-term relationships, creating a more resilient business model amid changing market dynamics.
- Highlight key metrics and KPIs involved in churn analytics.
To effectively analyze churn, several key metrics and KPIs must be highlighted. First, the Customer Churn Rate serves as the most critical indicator, reflecting the percentage of customers lost over a specific period. Understanding this rate is essential for evaluating overall business health and operational effectiveness. Second, the Customer Retention Rate complements this metric, indicating the proportion of customers who remain loyal, thus showcasing the effectiveness of customer engagement strategies.
Additionally, the Net Promoter Score (NPS) is integral in understanding customer satisfaction and loyalty. High NPS scores often correlate with reduced churn, making it an invaluable tool in churn prediction strategies. Another vital metric is the Customer Lifetime Value (CLV), which projects the total revenue expected from a customer relationship. By examining these metrics, businesses can make data-driven decisions that refine their approach to customer retention and churn reduction. Ultimately, these insights empower organizations to better anticipate potential churn and implement proactive measures for customer satisfaction.
Key Churn Prediction Strategies for Success
Developing effective churn prediction strategies is essential for businesses seeking to retain customers and enhance profitability. A successful approach begins with identifying relevant data sources. Understanding customer behaviors, feedback, and engagement trends can illuminate patterns of potential churn, allowing businesses to act proactively.
Next, segmentation plays a vital role in churn prediction. By categorizing customers based on demographics, purchasing behavior, and engagement levels, businesses can tailor their strategies to target each segmentโs unique needs. Additionally, employing key predictive analytics tools enables companies to anticipate potential churn through advanced statistical techniques. Regularly analyzing the outcomes of these strategies ensures continuous improvement and demonstrates a commitment to customer satisfaction, further reducing churn rates over time. Importantly, these efforts create a more personalized experience, encouraging customer loyalty and long-term success.
- Outline various data analytic tools and their applications in churn prediction.
Data analytic tools play a crucial role in enhancing churn prediction strategies. One notable tool is customer relationship management (CRM) software, which allows businesses to track customer interactions and behaviors. Utilizing historical data from CRM systems helps identify patterns linked to customer retention and loss. Furthermore, machine learning algorithms, such as logistic regression and decision trees, are instrumental in predicting churn by analyzing customer demographics and payment histories.
Another effective tool is sentiment analysis software. By assessing customer feedback from surveys or social media, companies can gather valuable insights about their customersโ sentiments. This understanding aids in crafting personalized marketing campaigns that can potentially reduce churn rates. Additionally, data visualization platforms enable businesses to depict customer trends and behaviors clearly, thus allowing for timely interventions based on predictive insights. Ultimately, integrating these tools can empower organizations to develop robust strategies aimed at reducing customer churn.
- Explain how businesses can tailor these strategies to their specific needs.
Businesses can effectively tailor churn prediction strategies by first understanding their unique customer demographics and behavior patterns. Each organization has different challenges and opportunities, which necessitate a customized approach. For instance, a retail business might focus on seasonal trends, while a subscription service may prioritize user engagement metrics. By analyzing historical customer data relevant to their industry, businesses can identify which factors most significantly impact churn and refine their strategies accordingly.
Additionally, integrating feedback loops from customer interactions enhances these strategies. This involves continuously gathering insights through customer surveys and analyzing feedback to adapt predictive models in real-time. By adjusting their approach to fit their specific needs, such as targeting features that cause dissatisfaction or improving service delivery, businesses can reduce customer turnover. Ultimately, a tailored strategy not only forecasts churn more accurately but also fosters stronger customer loyalty, leading to sustained growth.
Generate Journey maps, Mind maps, Bar charts and more from your data in Minutes
Steps to Implementing Effective Churn Prediction Strategies
Implementing effective churn prediction strategies begins with a structured approach that considers various factors influencing customer retention. First, data collection and management are crucial. Establish reliable methods for gathering comprehensive customer information, which aids in building accurate predictive models. Utilizing diverse data sources, such as transaction histories and customer feedback, enhances the quality of insights gleaned.
Next, it's essential to choose the right predictive model tailored to your business goals. This might involve testing different algorithms to determine which best identifies customer behaviors and churn triggers. Once a model is in place, continuous monitoring and refinement are vital. Regularly assess the model's performance, adjusting parameters and strategies based on emerging trends and insights. This iterative process ensures that your churn prediction strategies remain relevant and effective, significantly enhancing customer retention efforts.
Step 1: Data Collection and Management
Gathering and managing data effectively is crucial for accurate churn prediction strategies. Start by identifying relevant data sources, such as customer interactions, demographic information, and purchase histories. Ensure that the data collected is both comprehensive and precise. High-quality data forms the backbone of any predictive model, enabling businesses to uncover insightful trends and patterns.
Next, implement a systematic approach to data management. Establish processes for regular data cleaning and validation to maintain accuracy over time. Utilize tools that can centralize your data, allowing easy access for analysis. Additionally, create a framework for tracking customer behavior and feedback, as this can provide rich insights into potential churn triggers. By prioritizing these steps, organizations can build a solid foundation for their churn prediction initiatives and enhance their ability to preemptively address customer concerns.
- Detail best practices for gathering and managing customer data for predictive modeling.
Gathering and managing customer data effectively is crucial for accurate predictive modeling in churn prediction strategies. Start by collecting diverse data points, including transaction history, feedback, and demographic information. This holistic approach enables a more comprehensive understanding of customer behavior. Encourage constant communication with your customers to collect qualitative data, such as satisfaction surveys, which can offer insights into potential churn triggers.
Once data is gathered, prioritizing data storage and organization is essential. Employ robust data management systems that can categorize and process information efficiently. Regularly audit your data for accuracy and relevance, as outdated information can skew predictive models. This foundational work not only enhances model performance but also builds trust with customers who share their data. Ultimately, effective management of customer data fosters insightful analysis, which is critical for developing targeted churn prediction strategies.
Step 2: Choosing the Right Predictive Model
Selecting the appropriate predictive model is crucial in implementing effective churn prediction strategies. A variety of models exist, including logistic regression, decision trees, and advanced machine learning techniques. Each model comes with its strengths and weaknesses, so the selection process should align with your business objectives. For instance, logistic regression provides simplicity and interpretability, making it suitable for quick insights, while decision trees can manage complex nonlinear relationships effectively.
When choosing a model, consider the volume and nature of your data, as well as your team's technical expertise. If your data set is vast and multidimensional, advanced algorithms like neural networks may prove beneficial. Additionally, testing multiple models can help determine which one best captures the nuances in your data. Ultimately, selecting the right predictive model will empower your business to identify at-risk customers more effectively and implement targeted interventions to reduce churn.
- Describe different predictive models and how to choose one based on business goals.
Choosing the right predictive model for customer churn analysis is essential to align with specific business goals. Various predictive modelsโsuch as logistic regression, decision trees, and neural networksโoffer unique advantages depending on data characteristics and operational needs. For example, logistic regression is excellent for binary classification problems, providing clear probabilities. Decision trees enable intuitive visualization, making them easier to interpret, while neural networks can capture complex patterns in large datasets.
When selecting a model, consider your business goals. If your priority is actionable insights, a simpler model might be best. Conversely, for high accuracy and handling complex relationships, more advanced models could be appropriate. Additionally, ensure that the model you choose can easily integrate with your existing systems for seamless implementation. Ultimately, the choice of predictive model in churn prediction strategies should focus on balancing complexity, interpretability, and alignment with the organizationโs strategic objectives.
Step 3: Monitoring and Refinement
Monitoring and refining churn prediction strategies is essential for maintaining their accuracy and effectiveness. As customer behavior evolves, so should your analytical approaches. Continuous monitoring involves regularly reviewing the prediction models against actual churn rates, allowing you to identify discrepancies and adjust your strategies accordingly. Ensure that the data you're using remains relevant and reliable, as outdated information can mislead your efforts.
Refinement entails making iterative enhancements to your predictive models. This could mean incorporating new data sources or adjusting algorithms based on recent findings. Engage with your team to gather insights and observe how well new interventions perform. By actively monitoring and refining your churn prediction strategies, you're not just reacting to customer behavior; you're proactively anticipating their needs, ultimately leading to more effective retention efforts. Regular updates and reviews will help you stay ahead in a competitive market.
- Discuss the importance of continuously monitoring churn predictions and refining strategies.
Continuously monitoring churn predictions is crucial for enhancing the effectiveness of churn prediction strategies. Regular assessments allow businesses to recognize shifts in customer behaviors and preferences, which might indicate an increased risk of churn. By promptly identifying these patterns, organizations can proactively intervene to retain valuable customers and enhance overall satisfaction. Consistent evaluation also aids in validating the accuracy of predictive models, ensuring they remain relevant in a dynamic market.
Moreover, refining strategies based on real-time data is essential. Feedback loops from customer interactions can inform strategy adjustments, aligning them with current customer needs. Adapting to these insights fosters a deeper connection with customers while reducing the likelihood of churn. Implementing a cycle of monitoring and refinement ensures that businesses are not just reacting to churn, but also anticipating future trends, ultimately leading to long-term success and customer loyalty.
Conclusion and Future Trends in Churn Prediction Strategies
In conclusion, Churn Prediction Strategies hold significant promise for improving customer retention and driving business growth. As organizations continue to adopt data-driven approaches, they must explore advanced analytics and machine learning techniques to refine their predictive capabilities. By understanding customer behavior and preferences, businesses can implement targeted interventions that effectively reduce churn.
Looking ahead, future trends in churn prediction will likely incorporate real-time data analytics and artificial intelligence. These innovations will empower companies to make timely decisions and personalize customer experiences, ultimately fostering loyalty and satisfaction. As these strategies evolve, it will be essential for organizations to remain agile, continuously adapting to the changing dynamics of customer expectations and market conditions.