Health Insurance Analytics plays a critical role in enhancing decision-making within the industry. As healthcare continues to evolve, organizations are increasingly turning to analytics to gain a deeper understanding of their customers. This analytical approach enables companies to identify trends, improve service delivery, and optimize operations for better patient outcomes.
By harnessing Health Insurance Analytics, stakeholders can explore vast datasets that reveal insights about customer behavior and preferences. These insights not only guide strategic initiatives but also empower organizations to tailor their offerings and address the unique needs of their clientele effectively. Understanding these fundamentals sets the stage for implementing comprehensive customer analytics strategies in health insurance.
Generate visualizations from your qualitative data. At Scale.
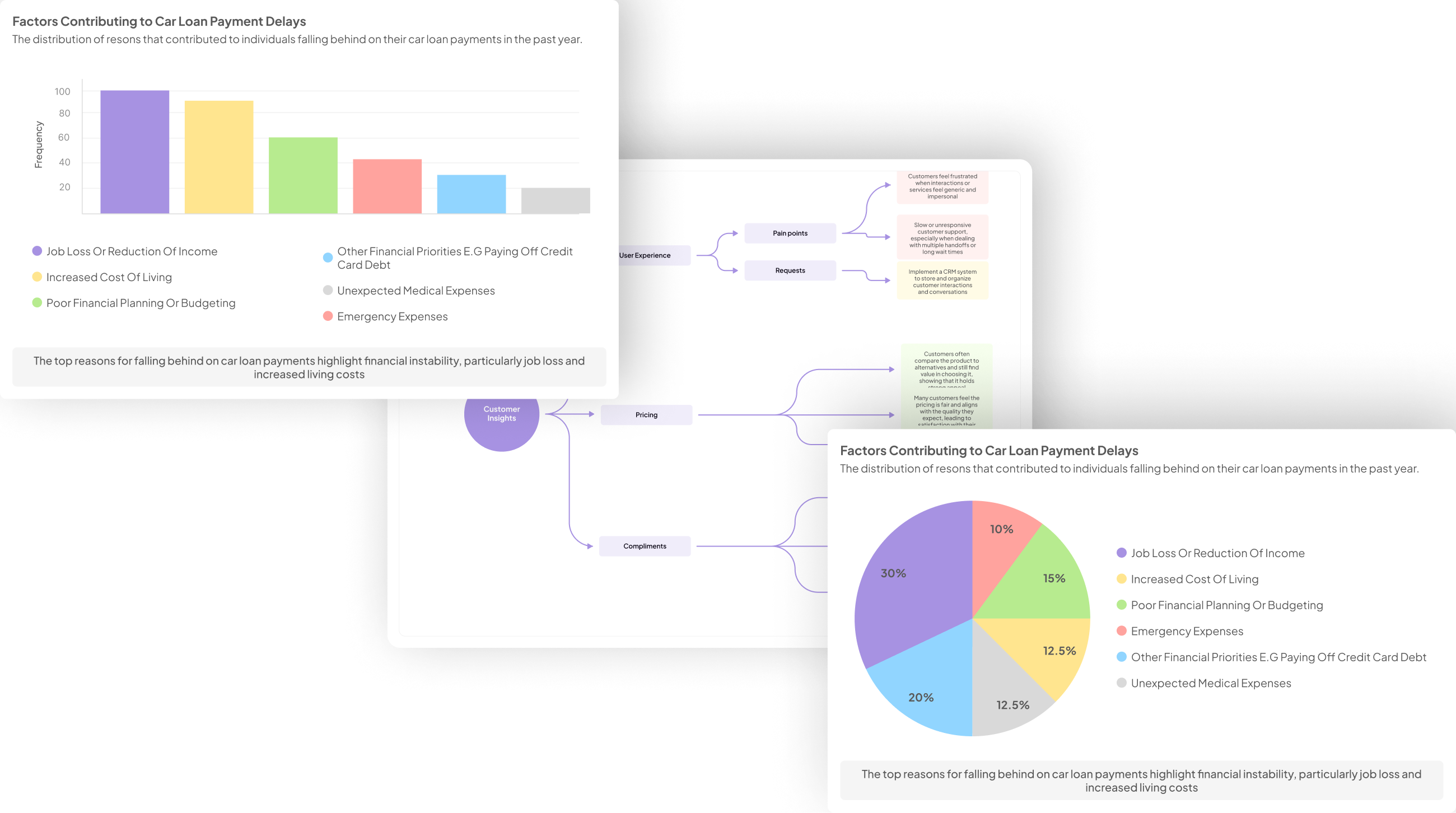
Understanding the Role of Health Insurance Analytics
Health Insurance Analytics plays a crucial role in transforming how health insurers understand and interact with their customers. By collecting and analyzing data, insurers can identify customer needs, preferences, and pain points. This understanding helps tailor services and improve customer satisfaction, ultimately fostering loyalty and trust.
Vital aspects of Health Insurance Analytics include data collection, analysis, and the extraction of actionable insights. Insurers utilize various data sources, such as claim history and customer feedback, to paint a comprehensive picture of customer behavior. Moreover, integrating analytical tools can help uncover trends, enabling organizations to address challenges proactively. For example, understanding common inquiries can streamline customer service operations, creating a smoother experience for customers. Overall, Health Insurance Analytics empowers insurers to not only react to customer needs but also anticipate them, ensuring a more personalized approach.
How Health Insurance Analytics Transforms Customer Experience
Health Insurance Analytics plays a crucial role in enhancing customer experience by providing valuable insights into customer behavior and preferences. This analytical approach helps health insurance providers understand the specific needs and expectations of their clients, allowing for more personalized service delivery.
One significant transformation is in the customization of plans. By analyzing data patterns, insurers can tailor offerings to meet individual health requirements. Additionally, prompt access to data enables faster claim processing, improving customer satisfaction. Clear communication, backed by analytics, fosters trust between customers and insurers. As analytics continues to evolve, it influences various touchpoints, from onboarding to ongoing support, ensuring customers receive optimal service at every stage of their insurance journey.
Moving forward, the ability to forecast trends and customer needs will further refine health insurance strategies, demonstrating that effective analytics results in a superior customer experience.
The Types of Data Used in Health Insurance Analytics
Health insurance analytics relies on diverse data types that play a crucial role in understanding customer behaviors and needs. Firstly, demographic data is fundamental, as it includes information about age, gender, location, and socioeconomic status. This data helps insurers to segment their customer base and tailor their offerings accordingly.
Secondly, claims data provides insight into patterns of healthcare utilization and related costs. Analyzing this data allows insurers to identify trends in treatment and preventive care. Furthermore, customer feedback—obtained through surveys or interviews—enables insurers to gauge satisfaction levels and pinpoint areas for improvement.
Lastly, external data sources, such as social determinants of health, enhance the analysis by showcasing factors that affect health outcomes. Integrating these various data types enables health insurance analytics to promote more personalized and efficient customer experiences. Understanding the types of data used in this field is essential for developing a robust analytics strategy that drives better health outcomes.
Generate Journey maps, Mind maps, Bar charts and more from your data in Minutes
Implementing Customer Analytics in Health Insurance
Implementing customer analytics in health insurance requires a structured approach to unlock its full potential. The first step is effective data collection, which involves gathering comprehensive information about customer interactions, claims history, and preferences. This wealth of data forms the foundation for meaningful analysis. Next, data analysis plays a critical role in identifying patterns and trends that can inform decision-making. Advanced analytical tools can help extract insights from complex datasets, enabling insurers to understand their customers better.
Once insights have been gathered, actionable implementation follows. This may involve adjusting insurance products, enhancing customer service, or developing targeted marketing campaigns. However, challenges such as data privacy concerns and integration of disparate data sources may arise. Addressing these challenges requires a robust strategy that prioritizes transparency and collaboration across departments. Ultimately, implementing customer analytics effectively can lead to improved customer satisfaction and loyalty in the health insurance industry.
Steps for Developing a Customer Analytics Strategy
To develop an effective customer analytics strategy within health insurance, organizations must follow a structured approach. The initial step is data collection, which involves gathering comprehensive data from multiple sources. This could include customer interactions, claims data, and feedback gathered through surveys. Gathering diverse data ensures a well-rounded understanding of customer needs and behaviors.
Next comes data analysis. It is crucial to apply analytical tools and methodologies to identify patterns and trends within the collected data. Using these insights allows health insurance providers to recognize key customer segments and their specific needs. Finally, the last step involves translating these actionable insights into implementation strategies. This could mean adjusting services, improving customer communication, or enhancing the claims process. By focusing on data collection, robust analysis, and actionable insights, organizations can significantly enhance their customer engagement and satisfaction in the health insurance domain.
Step 1: Data Collection
Data collection forms the foundation of Health Insurance Analytics, setting the stage for insightful analysis. The first step involves identifying the relevant data sources, which can include customer interactions, claims history, and demographic details. By collecting data from various touchpoints, organizations can capture a comprehensive view of customer behaviors and preferences, aiding in personalized service.
Once the data sources are identified, the next phase is to utilize the right tools and techniques for gathering this information. Accurate data collection ensures the reliability of insights derived later in the process. Techniques such as surveys, focus groups, and digital tracking can enhance the quality of data collected. By adopting a systematic approach to data collection, health insurers can effectively leverage customer insights to drive better outcomes and improve overall experiences.
Ultimately, thorough data collection not only supports analytical tasks but also empowers health insurers to make data-driven decisions, fostering a deeper understanding of their customers' needs and expectations.
Step 2: Data Analysis
Data analysis is a pivotal stage in utilizing Health Insurance Analytics effectively. At this point, organizations must sift through the collected data to extract meaningful insights. Analyzing customer interactions, feedback, and behavioral patterns allows insurance providers to understand their clients better and tailor services accordingly. This process involves various techniques, including descriptive statistics and advanced visualization tools. These methods help identify trends, spot anomalies, and highlight areas that require improvement.
In this step, key metrics are examined, such as customer retention rates and claims behavior. Additionally, segmenting the data based on demographics or geographical regions can reveal unique insights specific to different client groups. This nuanced understanding of customer needs will enable health insurance providers to make data-driven decisions, ultimately enhancing customer satisfaction and loyalty. By prioritizing data analysis, organizations position themselves to adapt to market changes and improve overall service delivery.
Step 3: Actionable Insights and Implementation
Transforming data into actionable insights is crucial in health insurance analytics, paving the way for effective implementation. The first step involves synthesizing the acquired data to identify key trends and patterns. These insights empower decision-makers to prioritize customer needs and improve their overall experience. For instance, analyzing customer feedback can reveal common pain points that require immediate attention, allowing insurers to address these issues proactively.
Once insights are identified, the next phase is implementation. This involves integrating the findings into existing processes and systems. Developing targeted strategies, such as personalized communications or tailored services, enhances customer engagement. Additionally, involving stakeholders in discussions about insights ensures everyone is on board, promoting a culture of data-driven decision-making. Timely and responsive actions from insurers can establish trust and loyalty among customers, ultimately leading to enhanced satisfaction and retention in the competitive health insurance market.
Challenges in Health Insurance Analytics and How to Overcome Them
Health Insurance Analytics presents several challenges that practitioners must navigate to unlock its full potential. One significant hurdle is data integration from various sources, which often leads to inconsistent data quality. Overcoming this involves implementing robust data governance frameworks to ensure data accuracy and reliability. Additionally, organizations frequently struggle with the analysis of complex datasets due to skill gaps within their teams.
Another challenge is ensuring compliance with privacy regulations while making data-driven decisions. Organizations can tackle this by prioritizing training in legal standards and utilizing advanced analytics tools that embed compliance checks. Finally, fostering a culture of data-driven decision-making is essential. Encouraging collaboration across departments can help demystify analytics and integrate it into the organizational fabric. By addressing these challenges, health insurance companies can better leverage analytics to enhance customer satisfaction and achieve operational efficiencies.
Conclusion: The Future of Health Insurance Analytics
As we look towards the future of health insurance analytics, the potential for enhancing customer experience becomes increasingly clear. Advanced analytical tools and methodologies promise more accurate insights into consumer behavior and health trends. This shift can empower insurers to tailor products more effectively while addressing individual needs.
Moreover, the integration of artificial intelligence and machine learning will redefine data analysis within the sector. These technologies can manage vast datasets, uncover hidden patterns, and generate actionable insights at unprecedented speeds. Ultimately, the evolution of health insurance analytics will foster a more responsive healthcare ecosystem, benefiting both insurers and consumers alike.