AI Call Benchmarking revolutionizes how businesses evaluate call quality and agent performance. By harnessing artificial intelligence, organizations can analyze conversations with unprecedented accuracy and speed. This technology enables the assessment of various metrics, such as engagement levels and compliance adherence, providing valuable insights that traditional methods often overlook.
In implementing an AI Call Benchmarking system, businesses benefit from a more streamlined approach to quality assurance. Advanced algorithms can deliver real-time evaluations, ranking agents based on their performance. Consequently, organizations can easily identify training needs, enhance customer experiences, and drive continuous improvement across their teams, making AI Call Benchmarking an essential tool for success in todayโs competitive landscape.
Analyze qualitative data. At Scale.
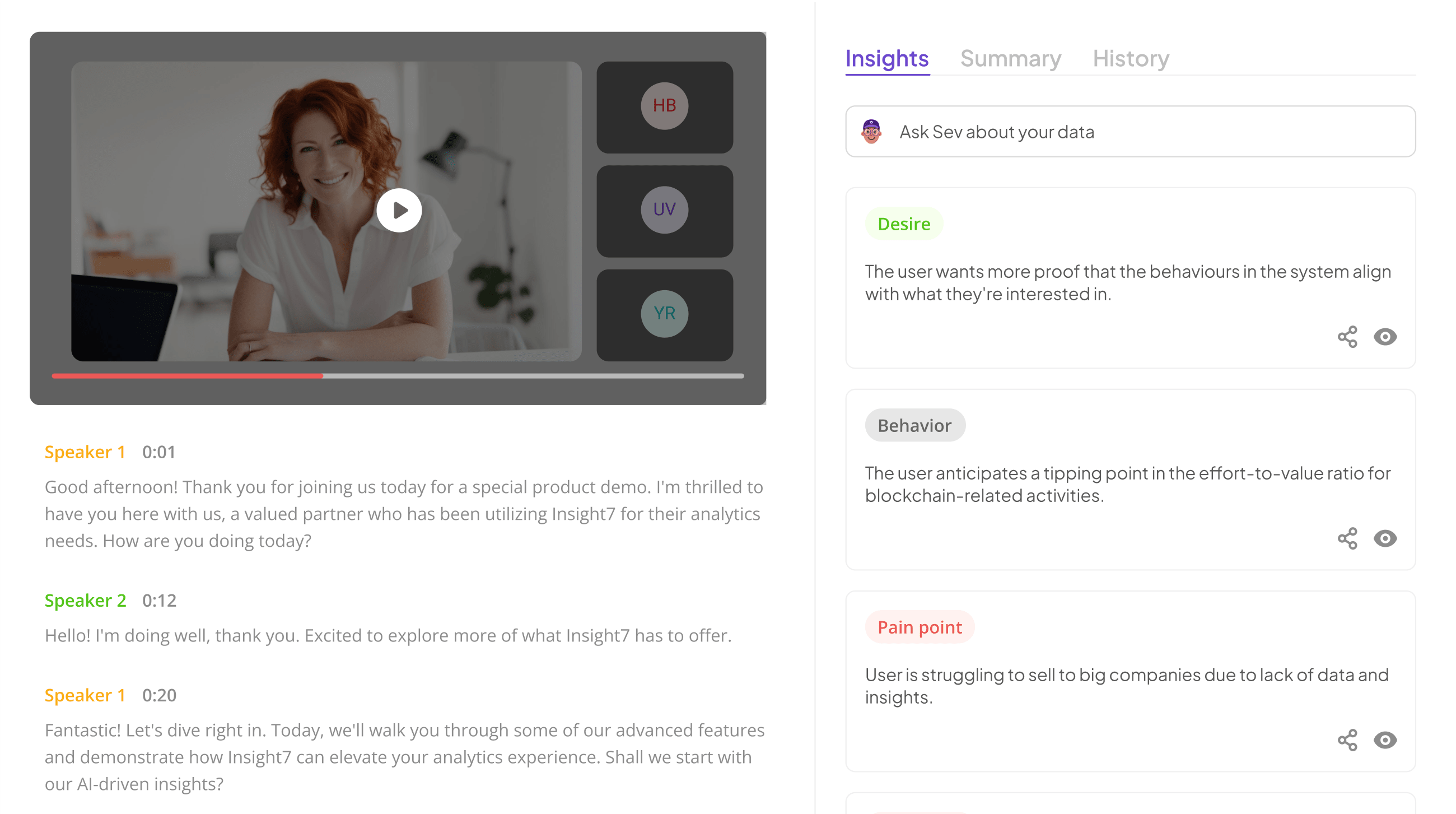
Understanding AI Call Benchmarking
AI Call Benchmarking refers to the process of evaluating and comparing call quality metrics using artificial intelligence technology. This method enhances the analysis of call recordings by automatically assessing key elements such as engagement levels and agent performance. It shifts the focus from traditional manual evaluations to a more systematic and data-driven approach, efficiently generating reports that highlight strengths and weaknesses in call interactions.
Understanding AI Call Benchmarking involves recognizing its ability to analyze vast amounts of communication data rapidly. The integration of AI facilitates accurate performance tracking across various metrics, enabling organizations to derive meaningful insights from caller interactions. Key components of effective benchmarking include defining clear quality metrics, managing and analyzing call data, and iteratively refining the system. By leveraging these practices, organizations can ensure continuous improvement in call quality and customer satisfaction, ultimately fostering a culture of accountability and growth.
What is AI Call Benchmarking?
AI Call Benchmarking refers to the systematic evaluation of call quality using artificial intelligence technologies. This process involves analyzing various aspects of calls, such as clarity, engagement levels, and compliance with standards. AI systems can review recorded calls, extracting valuable insights that help improve overall service quality and agent performance.
In practice, AI Call Benchmarking operates by utilizing algorithms that assess interactions between agents and customers. These algorithms identify patterns and determine trends that might otherwise go unnoticed. Additionally, results from the benchmarking process can be compiled into detailed reports, allowing organizations to make data-driven decisions for coaching agents and refining strategies. By embracing AI Call Benchmarking, organizations can not only enhance their service delivery but also boost customer satisfaction and retention rates.
Benefits of Implementing AI Call Benchmarking
Implementing AI call benchmarking offers several compelling advantages. First, it significantly reduces the time spent on manual evaluations. Teams can automatically analyze multiple calls, providing quick scores based on established parameters. This efficiency allows organizations to identify outliers and trends without dedicating extensive human effort to each call.
Moreover, AI call benchmarking enhances training programs. By extracting key data from a wide range of interactions, organizations can pinpoint common customer inquiries and adjust training materials accordingly. For instance, if specific questions frequently arise, training can focus on these topics to better prepare customer service representatives. This proactive approach ensures a more informed and responsive team, ultimately improving customer satisfaction and retention.
The integration of AI not only streamlines processes but also empowers organizations to make data-driven decisions, leading to continuous improvement in call quality and customer service effectiveness.
[ hfe_template id=22694]Building Your AI-Based Call Quality Benchmarking System
To build an effective AI-based call quality benchmarking system, start by defining the call quality metrics specifically tailored to your needs. Clearly outline which aspects, such as engagement or compliance, are most important for assessing agent performance. This foundational step enables effective communication of expectations and outcomes throughout the project.
Next, focus on data collection and management, ensuring you gather high-quality, relevant call data. This data can include audio recordings, transcripts, and agent performance metrics. With a robust dataset, implement AI algorithms capable of analyzing and interpreting this information efficiently. These algorithms should be designed to detect patterns, highlight areas needing improvement, and generate comprehensive reports for decision-making.
Finally, donโt forget about rigorous system testing and calibration. Regularly evaluate the system's accuracy and effectiveness by refining your metrics and algorithms based on the results. This iterative approach will ensure that you maintain high call quality standards and achieve your benchmarking goals effectively.
Essential Steps in AI Call Benchmarking System Design
Designing an effective AI Call Benchmarking system requires a structured approach that encompasses several essential steps. First, defining call quality metrics is crucial. Metrics may include factors like clarity, engagement, and compliance, which collectively help gauge call performance and identify improvement areas. Creating a precise set of metrics provides a foundation for evaluating agent performance consistently.
Next, data collection and management play a vital role. Ensuring comprehensive data is gathered from various sources enhances the accuracy of insights drawn from AI algorithms. Implementing robust AI algorithms is the third step, as these should be designed to process the collected data efficiently. Finally, system testing and calibration are critical to ensure that the benchmarks set are reliably assessed against real-world scenarios and provide meaningful feedback. By following these steps, you can create a robust AI Call Benchmarking system that maximizes call quality and performance insights.
Step 1: Define Call Quality Metrics
Defining call quality metrics is a foundational step in creating an effective AI-based call quality benchmarking system. It is essential to clearly articulate what constitutes a successful call within your organization. Metrics should encompass various elements, including customer satisfaction, compliance with call protocols, and overall effectiveness in lead qualification. Identifying these metrics requires a thorough understanding of your business objectives and the key performance indicators relevant to your call center operations.
Once the metrics are established, it is crucial to create a scoring system for evaluating each call. This system should weigh the importance of different criteria, such as clarity of communication and adherence to scripts. With well-defined call quality metrics, AI can analyze recordings efficiently and provide actionable insights, fostering improved performance. This systematic approach ensures your AI Call Benchmarking is both reliable and conducive to achieving high-quality customer interactions.
Step 2: Data Collection and Management
Effective data collection and management are fundamental to establishing a robust AI call benchmarking system. First, identify the sources of your call data to ensure comprehensive coverage. Utilize call recordings, transcripts, and customer feedback to gather a well-rounded dataset. Proper management of this data ensures that you can accurately evaluate call quality and extract meaningful insights.
Next, establish clear protocols for data organization. Categorizing data by parameters such as call duration, customer satisfaction scores, and agent performance allows for easier analysis. Automating data collection processes can enhance efficiency, freeing up resources for deeper analysis, which can help continuously improve call quality metrics. By effectively managing this data, your AI call benchmarking system can evolve, providing valuable insights that drive overall performance and customer satisfaction.
Step 3: Implement AI Algorithms
In Step 3, the focus shifts to the implementation of AI algorithms, crucial for developing an effective AI-based call quality benchmarking system. Start by selecting suitable algorithms that can process audio data, identify agents, and analyze call metrics. These algorithms will learn from historical call data and continuously improve their accuracy over time. Consider leveraging natural language processing to understand call contexts, sentiment, and engagement levels, which are essential for AI Call Benchmarking.
Next, train the chosen algorithms using a diverse dataset to enhance their performance. Implementing machine learning techniques enables the system to refine itself as more call data becomes available. Periodically evaluate algorithm performance against set benchmarks to ensure relevance and reliability in delivering insights. By incorporating these advanced algorithms, your benchmarking system will provide accurate assessments of call quality and agent performance, thereby enabling informed decision-making and improvements in customer interactions.
Step 4: System Testing and Calibration
After implementing the AI algorithms and collecting data, the next crucial phase is system testing and calibration. This step ensures that the AI call benchmarking system accurately evaluates call quality based on defined criteria. During this process, testers will run numerous calls through the system to verify its accuracy in scoring various aspects like greeting, engagement, and resolution skills. Itโs essential to assess whether the system identifies issues and delivers actionable feedback.
Calibration is equally important. Adjustments may be necessary if the AI consistently misclassifies calls or fails to highlight key performance indicators effectively. Continuous testing and fine-tuning help refine the system's ability to generate reliable evaluations. Gathering feedback from users during this phase can further enhance the system's accuracy, ensuring that the AI call benchmarking meets the evolving needs of your organization. This stage lays the foundation for effective analysis, ultimately driving better customer interactions.
Top Tools for AI Call Benchmarking
In the realm of AI call benchmarking, various tools can significantly enhance the accuracy and efficiency of your call quality evaluations. One of the top tools is call analytics software, which provides valuable insights into agent performance and customer interactions. Utilizing advanced algorithms, these platforms analyze calls and extract key performance indicators, allowing managers to make informed decisions.
Another invaluable resource includes Natural Language Understanding (NLU) and speech recognition APIs. These tools empower organizations to automatically identify dialogue, capture sentiments, and categorize conversations, all crucial for thorough benchmarking. Implementing machine learning platforms further aids in refining your analysis, allowing you to adapt and improve your benchmarking approach over time. When combined, these tools form an effective system for AI call benchmarking, offering a comprehensive view of call quality and enhancing overall customer service efficiency.
insight7
To establish a robust AI Call Benchmarking system, understanding the landscape of call quality metrics is pivotal. Insight7 provides a foundational approach where users can identify the most critical aspects of call performance. This involves defining key metrics, such as call clarity, response times, and customer satisfaction scores, ensuring that the evaluation process is targeted and efficient.
Next, seamless data collection becomes essential. Utilizing advanced technologies allows for real-time data gathering from various sources, ensuring comprehensive insights. With these insights, AI algorithms can then be implemented to analyze patterns and trends. This not only enhances call quality assessments but also provides actionable strategies for improvement. Ultimately, by methodically addressing these elements, businesses can refine their customer interaction processes, leading to increased satisfaction and operational efficiency in the competitive landscape.
NLU and Speech Recognition APIs
Natural Language Understanding (NLU) and Speech Recognition APIs are essential for creating an effective AI-based call quality benchmarking system. These technologies allow for the conversion of spoken language into text, enabling robust analysis. Effective use of NLU can enhance comprehension of caller intent, while speech recognition APIs ensure accurate transcripts of conversations between agents and customers.
To successfully implement these technologies, consider these key aspects:
Accuracy of Recognition: Choose APIs with high accuracy rates to ensure better data quality, which directly impacts benchmarking.
Integration Capabilities: Look for APIs that easily integrate with your existing systems for seamless data flow.
Language Support: Ensure the APIs support multiple languages and dialects to cater to a diverse customer base.
Customization Options: Select solutions that allow tailoring to specific industry needs or business metrics.
By focusing on these factors, you will optimize your AI call benchmarking efforts, leading to clearer insights and improved service quality.
Machine Learning Platforms
Machine learning platforms play a pivotal role in developing an effective AI call benchmarking system. These platforms provide the necessary infrastructure to analyze large sets of call data, facilitating the extraction of meaningful insights. By employing advanced algorithms, they help identify patterns related to call quality, customer sentiment, and service efficiency. This analysis can drive improvements in customer experiences and operational efficiency.
Selecting the right machine learning platform involves several key considerations. First, ease of use is essential; users should access tools without extensive training. Next, scalability matters, allowing the platform to grow alongside data needs. Additionally, integration capabilities are crucial for aligning machine learning tools with existing systems. Finally, support and documentation can significantly impact the platformโs usability. Together, these factors contribute to a robust AI call benchmarking ecosystem, offering actionable insights to optimize call quality.
Call Analytics Software
Call analytics software plays a crucial role in creating an AI-based call quality benchmarking system. This type of software collects and analyzes data from various calls, allowing businesses to assess their performance and compliance with regulations. By leveraging advanced algorithms, organizations can sift through large volumes of call data efficiently, identifying key metrics that impact call quality.
With call analytics, companies can focus on high-value interactions. They can choose to analyze lengthy calls that provide meaningful insights while disregarding shorter, less informative ones. This process aids in identifying patterns related to compliance, customer experience, and sales effectiveness. Furthermore, the software offers the ability to tag and categorize calls based on specific keywords or phrases, enhancing the ability to train staff effectively. In essence, effective call analytics software is vital for maintaining high standards in customer interactions and ensuring consistent quality in communication practices.
Conclusion: The Future of AI Call Benchmarking
The future of AI call benchmarking holds immense potential for redefining how organizations assess call quality. As AI technology continues to advance, we can expect increasingly sophisticated metrics and analytics tailored to precise business needs. This evolution will enhance the accuracy of identifying agent performance and overall customer satisfaction levels.
Moreover, integrating AI into call benchmarking will facilitate more personalized feedback and training for agents, allowing for targeted improvements. As industries embrace these advancements, AI call benchmarking will become essential for maintaining competitive advantages and fostering exceptional customer experiences. The next phase will encourage further innovations that address emerging challenges while ensuring compliance and quality standards are upheld.