Common Mistakes in Qualitative Survey Data Analysis and How to Avoid Them
-
Bella Williams
- 10 min read
Qualitative analysis pitfall can derail even the most well-intentioned research efforts. Imagine spending hours collecting rich data, only to find out later that biases skewed your results. Understanding these pitfalls is crucial for researchers, as they can significantly impact the interpretations and insights drawn from qualitative data.
This section explores common mistakes researchers encounter when analyzing qualitative survey data, offering insight into how to avoid these traps. By recognizing pitfalls such as selection bias and overgeneralization, you can enhance the validity and reliability of your findings. Implementing robust coding practices and triangulation methods will further fortify your analysis, ensuring your research delivers valuable insights.
Analyze & Evaluate Calls. At Scale.
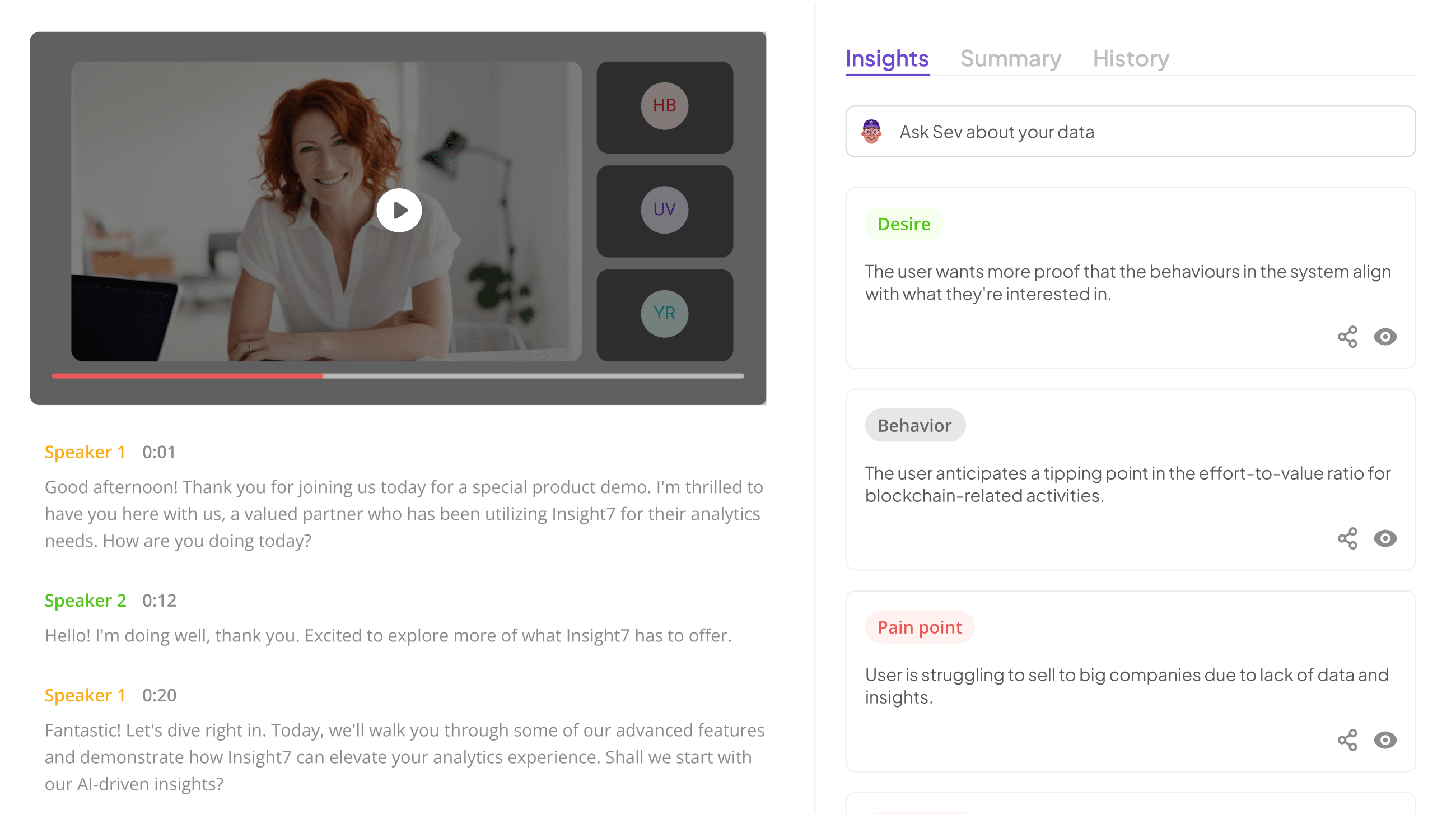
Identifying Qualitative Analysis Pitfalls in Data Collection
Qualitative analysis pitfalls can significantly impact data collection processes, leading to skewed results and misinterpretations. One prevalent issue is selection bias, which occurs when the participants chosen for the study are not representative of the broader target population. This bias can prevent researchers from gaining a well-rounded understanding of the subject matter. To address this, it's essential to ensure that participant diversity is prioritized, allowing for a more comprehensive exploration of varying perspectives.
Another common pitfall is overgeneralization, which arises when findings from a limited qualitative dataset are applied too broadly. It's crucial to recognize the inherent limitations of qualitative data, emphasizing that conclusions drawn should not be projected onto the wider population without sufficient evidence. Researchers can mitigate these pitfalls by employing robust strategies, such as thorough participant recruitment plans and mindful analysis approaches that acknowledge the scope of the data. By being vigilant about these common qualitative analysis pitfalls, researchers can enhance the reliability and validity of their findings.
Selection Bias: A Common Qualitative Analysis Pitfall
Selection bias is a significant challenge in qualitative analysis, particularly in survey data collection. This bias occurs when the participants selected for the study do not accurately represent the diverse group being researched. Consequently, the insights derived can often be skewed, leading to conclusions that may not reflect the broader populationโs views or experiences.
To mitigate selection bias, it is essential to prioritize participant diversity. Researchers should actively seek out a varied demographic, ensuring that voices from different backgrounds, perspectives, and experiences are included. Moreover, employing random sampling methods can significantly enhance the representativeness of the sample. Other strategies include ensuring transparency in participant recruitment approaches and continuously assessing who is being included in the study. By addressing selection bias head-on, researchers can produce more reliable and valid qualitative insights, moving beyond this common pitfall in qualitative analysis.
- Importance of Participant Diversity
In qualitative data analysis, participant diversity plays a critical role in ensuring that findings are well-rounded and representative. Including individuals from varied backgrounds enhances the richness of the data and enables the identification of nuanced perspectives that might otherwise go unnoticed. This breadth of experience helps mitigate qualitative analysis pitfalls, particularly selection bias, where certain views dominate the findings due to a lack of representation.
Diverse participants contribute different viewpoints, fostering a more comprehensive understanding of the research topic. This diversity can also enhance the credibility of the analysis, making it more relatable and applicable to a broader audience. To avoid the mistake of overgeneralization, it is essential to engage a spectrum of participants with varying demographics, experiences, and insights. By doing so, researchers can create a more robust and accurate portrayal of the subject matter, ultimately leading to more effective and inclusive decision-making processes.
- Strategies to Mitigate Selection Bias
Mitigating selection bias is critical to obtaining reliable qualitative survey data. One effective strategy is to ensure a diverse participant pool. This involves inviting individuals from various backgrounds and experiences, which enriches the data collected and ensures that multiple perspectives are represented. A homogeneous group may lead to insights that are not universally applicable, resulting in flawed interpretation of the data.
Additionally, carefully defining selection criteria can help in addressing potential biases. Establishing clear guidelines for participant selection allows researchers to identify and recruit a more representative sample. Researchers should also consider using random sampling techniques when feasible, as this can minimize bias and enhance the generalizability of the findings. Implementing these strategies not only counters selection bias but also strengthens the overall quality of qualitative analysis, leading to richer and more trustworthy insights.
Overgeneralization: Avoiding This Qualitative Analysis Pitfall
Overgeneralization is a significant pitfall in qualitative analysis that researchers must vigilantly guard against. It occurs when findings from a small subset are inappropriately extended to broader populations. Recognizing the limitations of qualitative data is crucial. Researchers must remain aware that individual experiences and sentiments do not always represent the views of larger groups.
To avoid this mistake, consider two main strategies. First, always contextualize your findings within the specific demographics of your participants. This assessment provides clarity and accuracy regarding the implications of your results. Second, use case studies to explore how overgeneralization has led to misinterpretations in past research. By examining these examples, you can learn valuable lessons on maintaining focus and precision in your qualitative analysis. Such reflections will strengthen your research and enhance the reliability of your qualitative insights.
- Recognizing the Limits of Qualitative Data
Qualitative data is invaluable for understanding complex human experiences, but it has noteworthy limits. A major issue arises when data is overgeneralized, leading to misleading conclusions. Researchers can fall into the trap of assuming that individual experiences reflect broader patterns. This overreach can skew results, prompting decisions based on anecdotal evidence rather than robust findings.
Moreover, qualitative analysis pitfalls often occur when researchers disregard the contextual richness of the data. Each participantโs response is influenced by their unique background and circumstances. Ignoring this context can lead to superficial interpretations that overlook deeper meanings. Acknowledging the limitations of qualitative data encourages a more cautious and informed approach to analysis. Researchers should aim to supplement qualitative findings with quantitative data whenever possible. This helps in creating a fuller picture, enabling more reliable conclusions and informed decision-making in future projects.
- Case Studies on Overgeneralization Issues
Overgeneralization poses significant challenges in qualitative data analysis. Through various case studies, we uncover how this issue manifests, often leading to misleading conclusions. For instance, in one scenario, a team analyzed participant interviews to ascertain customer satisfaction but assumed that positive feedback from a small subset represented all customers. This neglect of broader context led to incorrect assumptions about overall satisfaction levels and subsequent misguided strategies.
In another case, researchers identified a common pattern in interview responses, hastily generalizing these findings without considering demographic differences across their sample. Such an approach can obscure critical variations that significantly impact the results. Identifying the nuances within qualitative data is crucial in avoiding pitfalls associated with overgeneralization. By thoroughly examining context and striving for richness in qualitative analysis, researchers can ensure their findings more accurately reflect the perspectives of diverse audiences.
Extract insights from interviews, calls, surveys and reviews for insights in minutes
Steps to Avoid Qualitative Analysis Pitfalls in Data Interpretation
To avoid qualitative analysis pitfalls, it's essential first to adopt accurate coding practices. Coding serves as the backbone of qualitative analysis, categorizing information and drawing meaningful insights from raw data. Start by establishing a clear coding framework that reflects your research goals. Regularly revisit and refine your codes to maintain consistency throughout the analysis. Consider collaborative coding sessions, where multiple analysts contribute, as this can enhance the reliability of your results.
Another crucial step is incorporating triangulation into your analysis. This approach means using multiple data sources or perspectives to validate your findings. For instance, comparing interview responses with survey results can help confirm or question your initial interpretations. By integrating multiple viewpoints, you create a more nuanced understanding of the data while minimizing bias. Ultimately, these steps will bolster the credibility of your qualitative research and lead to richer insights.
Step 1: Ensuring Accurate Coding Practices
Accurate coding practices are vital for effective qualitative analysis. Coding transforms raw data into meaningful categories, creating a structure for interpreting complex responses. When coding is done incorrectly, it can lead to misclassified data, which ultimately contributes to qualitative analysis pitfalls. Establishing clear coding guidelines is essential to maintain consistency and accuracy throughout the analysis process.
To ensure accurate coding practices, consider the following strategies:
Develop a Codebook: Create a comprehensive guide that outlines each code's definition and application. This serves as a reference for all analysts involved in the study.
Pilot Testing: Test your coding scheme on a small sample; this helps identify potential inconsistencies and allows for adjustments before full-scale coding.
Continuous Reflection: Regularly revisit and revise your codes as new themes emerge throughout the analysis, ensuring that your coding evolves with the data.
Employing these strategies can significantly reduce the chances of coding errors, safeguarding the integrity of your findings.
- The Role of Coding in Qualitative Analysis
Coding plays a critical role in qualitative analysis, serving as a bridge between raw data and meaningful insights. It involves the systematic organization of responses, allowing researchers to identify themes, patterns, and connections within the data. However, errors in coding can lead to significant qualitative analysis pitfalls, such as misinterpretation of data and loss of valuable insights. Thus, understanding proper coding practices is essential for effective qualitative analysis.
To ensure successful coding, researchers should follow key practices. First, establish a clear coding framework that aligns with the research objectives. This framework should be comprehensive yet flexible enough to accommodate emerging themes from the data. Next, consistency is crucial; all team members involved in coding must apply the same criteria to maintain reliability. Regularly revisiting and refining codes based on feedback can further enhance accuracy. By avoiding these common pitfalls and emphasizing the importance of coding, researchers can achieve deeper, more reliable insights from their qualitative surveys.
- Tips for Effective and Consistent Coding
Effective and consistent coding in qualitative analysis is crucial for minimizing qualitative analysis pitfalls. To ensure clarity and reliability, start by developing a coding framework. This framework should outline specific themes and categories derived from your research questions. Itโs essential to keep your codes clear and concise. Consistency in coding allows you to compare data effectively and spot patterns that might otherwise go unnoticed.
Another valuable tip is to involve multiple coders in the coding process. This collaboration can help identify discrepancies and improve overall accuracy. Regular meetings to discuss coding decisions will foster a shared understanding among coders. Finally, continuously refine your coding framework based on feedback and new insights. By approaching coding systematically, you can avoid common pitfalls and enhance the validity of your qualitative analysis.
Step 2: Incorporating Triangulation for Robust Analysis
Incorporating triangulation into your qualitative analysis can significantly enhance data interpretation and reduce common pitfalls. Triangulation involves the use of multiple methods, data sources, or perspectives to validate findings. This approach not only strengthens the credibility of your results but also minimizes biases that may arise from relying solely on a single data source. By understanding the benefits of triangulation, researchers can ensure a more comprehensive examination of their qualitative data.
To implement triangulation effectively, consider three key methods: first, use various data collection techniques such as interviews, focus groups, and surveys to capture diverse viewpoints. Second, gather data from multiple participant groups to reflect a fuller range of experiences. Finally, engage different researchers in the analysis process to bring unique insights and counteract potential biases. By addressing qualitative analysis pitfalls through triangulation, you strengthen the reliability of your findings and enhance overall research quality.
- Understanding Triangulation and its Benefits
Triangulation is a critical strategy in qualitative analysis that safeguards against common pitfalls, including biases and misinterpretations. It involves using multiple data sources, methods, or perspectives to enhance the credibility of the findings. By combining interviews, surveys, and observation, researchers can gain a more holistic understanding of the subject under study. This varied approach not only balances any potential individual biases but also enriches the data, enabling a deeper analysis.
The benefits of triangulation are substantial. First, it bolsters the validity of the research by cross-verifying findings, which can reveal inconsistencies or gaps in the data. Second, it offers different insights that might otherwise be overlooked when relying on a single method. Finally, when done correctly, triangulation can lead to richer and more nuanced conclusions, ultimately reducing qualitative analysis pitfalls. By implementing triangulation, researchers can ensure more reliable and trustworthy outcomes in their qualitative survey data analysis.
- Methods to Implement Triangulation in Analysis
Implementing triangulation in qualitative analysis can significantly mitigate common pitfalls often encountered during data interpretation. Triangulation involves using multiple methods or data sources to enhance the credibility of your findings. To effectively incorporate triangulation, consider employing a variety of qualitative techniques, such as interviews, focus groups, and participant observation. By comparing and contrasting data collected through these diverse avenues, you can develop a more comprehensive understanding of your research topic.
Second, utilize different perspectives when analyzing the same data set. Involve team members with varying backgrounds to enrich the interpretation of results. This collaborative approach not only strengthens the analysis but also helps reduce individual biases that may skew findings. Lastly, always document the reasoning behind your triangulation choices, as this provides transparency and helps others to understand your analytical framework. By marrying these methods, you can effectively navigate the qualitative analysis pitfalls that may arise during your research journey.
Conclusion: Navigating and Overcoming Qualitative Analysis Pitfalls
Navigating and overcoming qualitative analysis pitfalls requires a strategic approach and a commitment to accuracy. To effectively address common mistakes, one must prioritize robust data collection methods and maintain a diverse participant base. This ensures that findings reflect a comprehensive range of perspectives, reducing biases that might skew results.
Moreover, researchers should employ clear coding practices and triangulation, combining multiple data sources to validate insights. By actively recognizing potential pitfalls and implementing preventative measures, analysts can derive meaningful conclusions that enhance the overall quality of qualitative analysis. Ultimately, fostering a reflective process will lead to more trustworthy and actionable findings.