Predictive Coaching Efficiency in QA is reshaping how organizations approach quality assurance training. In an era where data-driven decisions dictate success, harnessing predictive analytics can significantly enhance coaching strategies. By utilizing predictive models, teams can effectively identify areas for improvement and tailor their coaching efforts for better outcomes.
Implementing this model requires a strategic approach, beginning with meticulous data collection and analysis. Understanding relevant metrics not only supports performance evaluation but also aids in crafting more effective training programs. As we explore the intricacies of this approach, it becomes clear that integrating predictive coaching methods can lead to substantial efficiency gains in quality assurance processes.
Analyze qualitative data. At Scale.
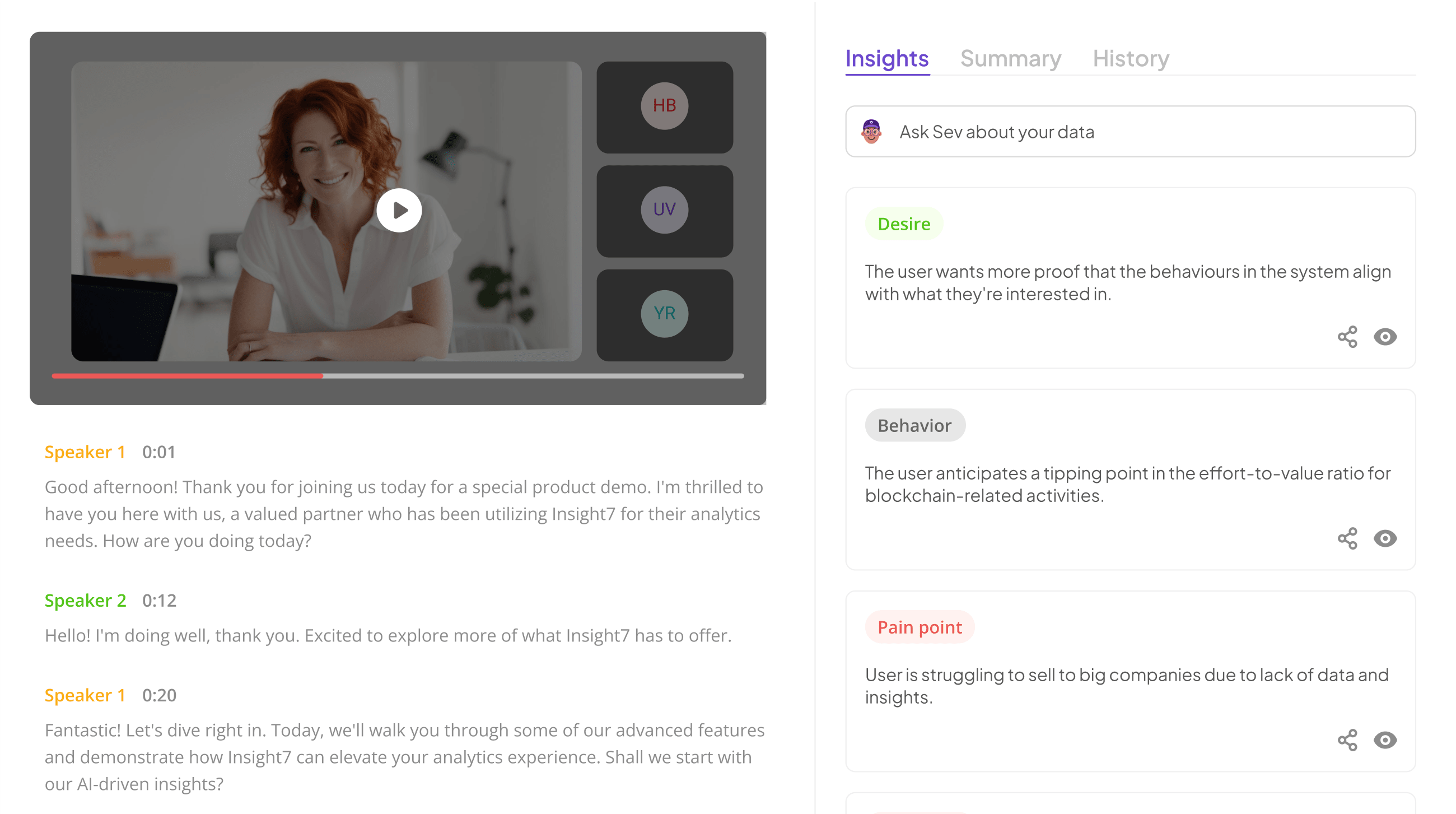
Understanding the Predictive Coaching Efficiency Model
The Predictive Coaching Efficiency Model serves as a blueprint for enhancing coaching practices within quality assurance (QA). By leveraging data analytics, this model allows organizations to anticipate the needs of their coaching staff and improve overall performance. The integration of analytics tools helps to identify patterns and trends, enabling coaches to tailor their strategies based on predictive insights.
Understanding this model involves recognizing key components, such as initial data assessment and ongoing analysis. Effective coaching starts with collecting the right data on employee performance, customer feedback, and market trends. From there, predictive techniques can be used to analyze this data, allowing organizations to implement strategies that promote continuous improvement and elevate coaching standards. By embracing this predictive approach, organizations can significantly increase their coaching efficiency and ultimately enhance their customer service outcomes.
The Role of Predictive Analytics in Coaching
Predictive coaching efficiency integrates analytics to enhance the coaching process. By utilizing historical data alongside real-time insights, coaches can better anticipate challenges and opportunities for development. This analytical approach allows for a more structured and informed coaching regimen, transforming subjective evaluations into objective assessments.
Incorporating predictive analytics into quality assurance processes involves identifying key performance indicators and leveraging advanced tools. These analytics provide valuable insights into individual and team performance, helping coaches tailor their strategies to meet specific needs. For instance, regular assessments of employee interactions and feedback empower coaches to detect trends, enabling interventions that lead to improved overall effectiveness. As coaching becomes more data-driven, organizations can achieve significant advances in efficiency, making predictive analytics an essential component of modern coaching frameworks.
- Discuss the importance of analytics in predicting coaching outcomes.
Analytics play a crucial role in predicting coaching outcomes by providing insights into performance patterns and behaviors. Through data analysis, organizations can identify key trends that impact coaching effectiveness, enabling targeted interventions. This makes Predictive Coaching Efficiency not just a theoretical concept but a practical approach to enhance coaching programs.
By employing analytics, coaches can evaluate multiple dimensions of performance, including strengths and weaknesses, thus personalizing their coaching strategies. Additionally, understanding these metrics allows for a more streamlined coaching process, where adjustments can be made in real-time based on data-driven insights. As organizations embrace predictive analytics, they can significantly enhance decision-making, leading to more successful coaching outcomes and improved team performance.
In this way, analytics essentially becomes the backbone of effective coaching, allowing organizations to refine methods and ultimately achieve higher performance levels.
- Explain how analytics tools are integrated into QA processes.
Analytics tools play a pivotal role in integrating predictive quality assurance (QA) into coaching processes. These tools enable the collection, analysis, and visualization of data, providing actionable insights that drive efficiency. With predictive analytics, organizations can forecast coaching needs and tailor strategies accordingly. By using data to understand performance trends and individual strengths, coaches can develop personalized training frameworks that enhance outcomes.
The integration of analytics begins with data collection from various sources, such as call recordings and customer interactions. Selected criteria, such as compliance and service quality, are analyzed against these datasets. This process not only identifies areas for improvement but also measures coaching effectiveness over time. Overall, employing analytics tools in QA processes significantly boosts predictive coaching efficiency. The structured use of data empowers organizations to make informed decisions that continuously enhance their coaching methodologies.
Steps to Implementing Predictive QA for Coaching Efficiency
To implement Predictive Coaching Efficiency effectively, start with an initial assessment and data collection. It’s essential to gather comprehensive data relevant to your coaching objectives. Focus on metrics like call compliance, customer satisfaction rates, and agent performance indicators to set a solid foundation. This initial stage helps you understand the landscape of your current coaching practices and identify gaps needing attention.
Next, analyze the collected data with predictive models tailored to your needs. Techniques such as regression analysis and machine learning algorithms can provide valuable insights into patterns and outcomes. Utilizing these predictive models allows you to identify trends and anticipate coaching needs, ensuring you can respond proactively rather than reactively. By following these steps, organizations can harness the power of Predictive Coaching Efficiency to create a more focused and effective coaching strategy, ultimately leading to enhanced performance within their teams.
Step 1: Initial Assessment and Data Collection
In the initial step of adopting Predictive Coaching Efficiency, it is crucial to gather pertinent data. This phase involves identifying the specific metrics that will influence coaching outcomes. By focusing on key performance indicators, such as call quality, customer satisfaction, and agent performance, organizations can establish a baseline for effective coaching strategies. Understanding these metrics enables teams to create targeted coaching plans that align with their specific objectives.
Once the relevant data has been collected, it is essential to analyze it comprehensively. This analysis should include reviewing individual calls and aggregating data across various projects to uncover trends and insights. Utilizing advanced analytics and reporting tools can facilitate this process, allowing coaches to ask questions about customer interactions and receive immediate feedback. The insights generated from this initial assessment set the foundation for implementing predictive models that further enhance coaching efficiency in the future.
- Highlight the importance of gathering the right data.
Gathering the right data is a critical element in enhancing Predictive Coaching Efficiency. Effective coaching relies on accurate, relevant information to draw insights about performance and areas for improvement. Without appropriate data, coaching efforts may misalign with organizational goals or fail to address the specific challenges faced by teams. Therefore, a structured approach to data collection is essential.
To optimize coaching results, it’s crucial to focus on several key areas when gathering data. First, identify specific metrics that directly impact performance, such as call handling times, customer satisfaction ratings, and compliance scores. Second, ensure the data is comprehensive and representative of various scenarios that employees encounter. Finally, use these insights to develop targeted strategies that promote continuous improvement and employee engagement. By prioritizing the right data, organizations can significantly enhance coaching efficiency and foster a culture of performance excellence.
- Identify what metrics to focus on for coaching.
To enhance coaching effectiveness, focusing on the right metrics is crucial. Begin with quantitative metrics such as call volume, average handling time, and resolution rates. These figures provide a solid foundation for assessing performance and identifying areas needing improvement. Additionally, consider qualitative metrics, including customer satisfaction scores and agent confidence levels. These insights can reveal more than numbers alone, highlighting the emotional aspects influencing coaching effectiveness.
Next, evaluate the training needs based on historical data to target specific skills or knowledge gaps. For example, if data indicates common errors in product knowledge, prioritize coaching around those areas. Implement regular evaluations, ensuring you track progress over time. This combination of quantitative and qualitative assessments allows leaders to tailor coaching interventions, driving predictive coaching efficiency. Engaging both data points leads to a comprehensive approach, ensuring every coaching session is relevant and impactful.
Step 2: Analyzing Data with Predictive Models
Efficiency in coaching relies heavily on data analysis, guiding coaches in identifying trends and areas for improvement. Predictive models allow for the transformation of raw data into actionable insights that inform coaching strategies. By leveraging advanced analytics, organizations can better understand performance metrics, leading to more targeted interventions.
A few key techniques enhance the effectiveness of predictive models in coaching environments. First, predictive analytics involves examining historical performance data to forecast future outcomes based on existing patterns. Second, machine learning algorithms can be employed to tailor coaching sessions based on individual trainee needs, improving engagement and retention. Lastly, visualizing data through dashboards helps coaches quickly grasp essential insights, fostering informed decision-making. By focusing on these approaches, organizations will see a marked improvement in predictive coaching efficiency and, ultimately, enhanced coaching outcomes.
- Discuss techniques for analyzing data.
Analyzing data effectively is crucial for enhancing predictive coaching efficiency. To begin, it's essential to collect relevant data, ensuring it captures the specific metrics that influence coaching outcomes. An initial assessment will help identify areas needing focus, allowing coaches to prioritize their efforts based on insightful patterns. For example, positive and negative feedback gathered from various interactions can serve as key indicators for coaching interventions.
Next, utilizing various analytical techniques can maximize the value derived from the data. Methods such as trend analysis, sentiment analysis, and comparative studies allow coaches to identify coaching efficiencies across different scenarios. By running predictive models, analysts can forecast potential coaching outcomes and assess the effectiveness of strategies implemented. This comprehensive approach not only informs immediate coaching decisions but also fosters a long-term improvement strategy, ultimately driving enhanced predictive coaching efficiency.
- Outline predictive models commonly used in coaching.
Predictive models play a crucial role in enhancing coaching efficiency through structured analysis of data. Commonly utilized models include regression analysis, decision trees, and clustering algorithms, each serving specific purposes. Regression analysis helps identify relationships between variables, enabling coaches to predict future performance based on historical data. Decision trees provide a visual breakdown of decisions and consequences, assisting coaches in tailoring their approaches based on anticipated outcomes. Clustering algorithms group similar data points together, enabling coaches to identify trends and behaviors in their teams and adjust their strategies accordingly.
Implementing these predictive models leads to improved predictive coaching efficiency by allowing coaches to make informed decisions. This proactive approach not only enhances coaching methods but also increases accountability and fosters a data-driven culture. By utilizing these models effectively, organizations can continually refine their coaching processes and achieve better results. The integration of predictive analytics in coaching provides a systematic framework for development that benefits both coaches and their teams.
Tools for Enhancing Predictive Coaching Efficiency
To enhance predictive coaching efficiency, organizations must utilize various tools designed for optimal data analysis and insight extraction. These tools not only streamline workflows but also provide valuable and actionable insights. A primary advantage is that they allow employees, regardless of their technical proficiency, to access and analyze customer interactions seamlessly. This democratization of data empowers teams to identify pain points and opportunities without extensive training.
Key tools include advanced analytics platforms that offer features specifically tailored for quality assurance. These platforms integrate data from multiple sources, allowing for comprehensive analysis and reporting. Additionally, AI-driven insights tools can automate trend recognition, making it faster to derive meaningful conclusions from datasets. By harnessing these tools, teams can elevate their coaching efficiency, ensuring that they focus on key areas for improvement and ultimately achieve better outcomes.
Extract insights from interviews, calls, surveys and reviews for insights in minutes
insight7: Leading the Charge
Leading the charge in predictive coaching efficiency involves harnessing advanced data analytics to transform the coaching landscape. By implementing such innovative strategies, organizations can significantly enhance their QA processes. This approach shifts the focus from reactive problem-solving to a proactive understanding of client needs, allowing for deeper engagement during interactions.
Central to this transformation is the ability to analyze customer conversations systematically. By capturing and interpreting significant customer signals, organizations can derive actionable insights promptly. These insights empower coaches to tailor their tactics effectively, ensuring greater alignment with client requirements. Ultimately, leading the charge in predictive coaching not only streamlines the coaching process but also cultivates a competitive edge by facilitating timely and informed decision-making. This ensures that organizations are not just responding to customer queries but actively driving meaningful conversations.
- Describe how insight7 can be utilized for predictive QA.
Insight7 serves as a powerful tool for predictive QA by streamlining the analysis of customer interactions and feedback. Its self-service platform allows users to easily gather and examine data from various customer conversations, making it simpler to extract actionable insights. This timely access to data plays a crucial role in enhancing predictive coaching efficiency, enabling organizations to stay ahead of their competition by swiftly adapting to customer needs and emerging trends.
To effectively leverage Insight7 for predictive QA, organizations can focus on a few key steps. First, they should ensure comprehensive data collection by capturing relevant metrics from customer interactions. Next, Insight7’s advanced analytic capabilities can be utilized to identify patterns in the data, helping to forecast coaching outcomes and inform decision-making. By employing these strategies, businesses can cultivate a more proactive coaching approach, turning insights into effective business strategies and creating lasting improvements in overall performance.
Other Key Tools in the Market
In exploring Other Key Tools in the Market, it’s essential to recognize the growing array of software designed to boost Predictive Coaching Efficiency. Advanced analytics platforms stand out by offering features that streamline data collection and analysis. These platforms enable users to visualize data trends, providing actionable insights that can be used to enhance coaching methods swiftly. By employing such tools, organizations can ensure that their coaching strategies are continuously aligned with market demands.
AI-driven insights tools are another valuable addition to the coaching toolkit. These tools process large sets of data to identify patterns and make real-time recommendations for coaching improvements. Utilizing AI can significantly reduce the time coaches spend on analysis, allowing them to focus on training and mentoring. This transformation not only enhances the coaching experience but also leads to more effective outcomes for the organization, ensuring they remain competitive in an evolving market.
Tool 1: Advanced Analytics Platform
The Advanced Analytics Platform plays a critical role in enhancing Predictive Coaching Efficiency within Quality Assurance (QA). This tool offers an intuitive interface, allowing users from various departments to easily access and utilize the platform without specialized training. By supporting straightforward data gathering and report generation, it empowers teams to act swiftly based on customer insights.
One standout feature is its library system, where calls and conversations are stored for analysis. This functionality allows users to extract meaningful insights like pain points and customer desires, enabling targeted coaching interventions. Beyond individual calls, the platform aids in analyzing multiple datasets simultaneously, facilitating a comprehensive understanding of coaching needs. With its user-friendly design and robust analytical capabilities, this platform significantly boosts the efficiency of QA coaching processes, driving timely and actionable improvements in performance.
- Features and benefits of the platform for QA.
The platform for QA enhances coaching efficiency through its user-friendly design, allowing easy access and navigation. This accessibility empowers team members at all levels to utilize the tool without extensive training. Predictive Coaching Efficiency is achieved as users can readily extract insights from various call transcripts, which helps identify key customer pain points and desires. By democratizing data, the platform ensures that insightful decision-making is not limited to a select few.
Moreover, the platform’s robust analytical capabilities allow users to visualize conversations and gather actionable insights quickly. Team members can analyze multiple calls within a project, providing a comprehensive overview of customer interactions. Such streamlined workflows significantly save time and elevate the quality of coaching feedback. The intuitive interface combined with powerful analytics fosters a culture of continuous improvement, ultimately driving higher performance across teams.
Tool 2: AI-Driven Insights Tool
The AI-Driven Insights Tool plays a crucial role in enhancing Predictive Coaching Efficiency within Quality Assurance frameworks. This tool is designed to simplify data analysis, making it accessible to all team members without requiring specialized training. By allowing users to analyze calls and extract vital insights, it facilitates a deeper understanding of customer experiences. This understanding aids in identifying pain points, desires, and behaviors, all of which are essential for effective coaching.
Furthermore, the AI tool enables the aggregation of insights across multiple interactions, offering a comprehensive view of trends and anomalies. Users can quickly generate reports and visualizations from conversations, making it easier to pinpoint areas for development. Ultimately, the AI-Driven Insights Tool empowers teams to make informed decisions that significantly enhance their coaching efforts, driving towards improved outcomes and efficiency in QA processes.
- How AI accelerates the coaching process.
Artificial Intelligence plays a transformative role in enhancing coaching efficiency, especially through its capabilities in predictive analytics. By analyzing past performance and identifying trends, AI can forecast potential coaching outcomes. This enables coaches to set more relevant goals tailored to each individual's needs, which accelerates the development process significantly.
The integration of AI-driven insights into the coaching framework streamlines assessment and engagement. Coaches can receive real-time feedback on their methods, allowing for continuous adjustments and improvements. This dynamic approach not only increases the effectiveness of the coaching sessions but also fosters a more proactive learning environment. As a result, Predictive Coaching Efficiency can be maximized, aligning coaching strategies with specific performance metrics to ensure sustained growth and success.
Results from Scaling Predictive QA
Results from scaling predictive QA reveal significant improvements in coaching efficiency. By implementing predictive analytics, organizations have streamlined their processes, leading to quicker evaluations of customer service representatives (CSRs). With fewer manual reviews required, team leaders can now focus more on strategy, enhancing overall performance.
The integration of key performance metrics has proven to be transformative. For instance, coaches can now access real-time reports detailing CSR scores based on specific parameters. This automation not only reduces the time spent on assessments but also ensures that feedback is grounded in data. Moreover, identifying patterns from customer interactions allows for targeted training, aligning preparation with the actual queries being raised. By systematically applying predictive models, organizations have not only increased coaching efficiency but also enhanced team member engagement through informed and relevant training sessions.
The results clearly illustrate the benefits of predictive coaching efficiency in QA, emphasizing a future driven by data and improved customer interactions.
- Case study results demonstrating improved efficiency.
Implementing predictive coaching has resulted in significant refinements in coaching efficiency. By utilizing data analytics tools, organizations transformed their coaching practices, leading to better outcomes for teams. This case study reflects how the analysis of call data allowed teams to identify pain points and customer concerns more effectively.
The results underscore a marked improvement in coaching effectiveness, with teams achieving their targets more consistently. For instance, coaches reported that their ability to pinpoint key issues during reviews improved dramatically. This shift toward predictive coaching efficiency not only enhanced performance metrics but also fostered a more engaged team culture. Consequently, the integration of predictive analytics and coaching practices streamlined decision-making, ensuring both time and resources were optimized for maximum impact. Ultimately, these improvements illustrate the power of leveraging data to drive coaching strategies and outcomes.
- Statistical evidence of improved coaching outcomes.
Statistical evidence of improved coaching outcomes highlights the transformative impact of utilizing Predictive Coaching Efficiency. Quantitative measures taken before and after implementing predictive analytics demonstrate a marked increase in key performance indicators. For instance, teams have reported up to a 30% increase in customer satisfaction scores, attributed to targeted coaching based on analytics-driven insights.
Moreover, improved call handling times were documented, with an average reduction of 15%. This suggests that agents equipped with tailored coaching are more adept at addressing customer needs promptly. Additionally, retention rates of high-performing agents showed a significant uptick, reinforcing the value of investing in a structured coaching framework backed by data. Overall, the integration of predictive analytics not only streamlines coaching processes but also empowers teams to achieve measurable success.
Conclusion on Predictive Coaching Efficiency in QA
The findings from our case study underscore the significant role predictive coaching efficiency plays in quality assurance. By implementing predictive analytics, organizations can better identify coaching opportunities and gaps in performance. This proactive approach not only streamlines the coaching process but also ensures tailored guidance for each team member, ultimately enhancing their capabilities.
Moreover, the integration of advanced analytics tools supports continuous improvement in QA processes. As teams become more adept at utilizing data-driven insights, they foster a culture of accountability and excellence. Emphasizing predictive coaching efficiency is essential for organizations aiming to optimize their workforce and achieve their quality assurance goals more effectively.