In today's rapidly evolving tech environment, the question of AI vs QA Analysts has sparked significant debate among software testing professionals. As organizations aim for higher efficiency and lower costs, the potential of AI in automating quality assurance processes looms large. Many wonder if AI can entirely replace QA Analysts or merely supplement their roles.
QA Analysts bring critical human insight to software testing that AI currently cannot replicate. While AI excels in speed and data processing, understanding nuanced user experiences and addressing contextual challenges remains a domain where human testers shine. This section will explore the balance between leveraging AI technologies and maintaining the irreplaceable value of human expertise in quality assurance.
Analyze & Evaluate Calls. At Scale.
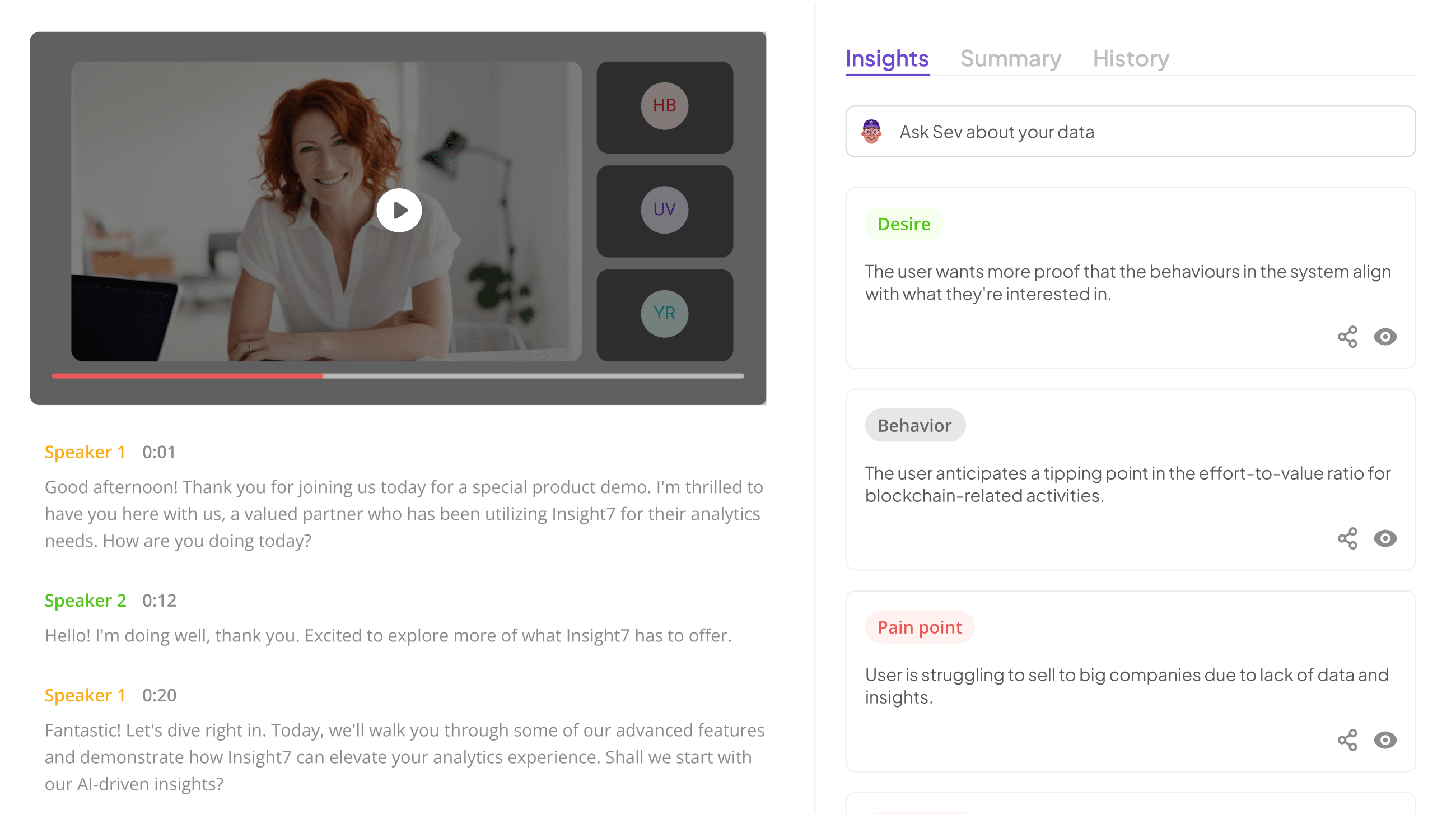
Understanding the Role of QA Analysts
Quality Assurance (QA) Analysts play a crucial role in ensuring that software meets the desired standards before it is released to users. Their key responsibilities encompass testing applications, identifying bugs, and verifying functionalities. While AI vs QA Analysts is a popular debate, the human touch remains essential in understanding user experiences and interpreting test results in ways machines simply cannot.
QA Analysts bring valuable expertise to the table, combining technical knowledge with critical thinking skills. They execute test cases, but also contextualize findings within user scenarios, thereby offering insights that inform product improvements. Furthermore, their ability to collaborate with development teams enhances communication, leading to more robust solutions. As we explore the capabilities of AI, it becomes clear that while automation can improve efficiency, the nuanced understanding provided by QA Analysts remains irreplaceable. Their role extends beyond mere testing; it ensures that the final product resonates with users, bridging the gap between technology and human experience.
Key Responsibilities of QA Analysts
QA Analysts play a crucial role in ensuring software quality, and their responsibilities are multifaceted. They begin by developing test plans based on project requirements, thereby identifying key metrics and targets. This initial phase is critical, as it sets the standard for the entire QA process. They also execute tests, meticulously documenting outcomes to ensure adherence to specifications. This documentation becomes essential when evaluating whether AI can effectively replace QA Analysts.
Furthermore, QA Analysts conduct regression testing to identify potential issues after software updates. They often collaborate with development teams to address bugs and enhance user experience. In exploring AI vs QA Analysts, itโs essential to recognize that while AI can automate some testing processes, the critical thinking and contextual understanding of human analysts remain irreplaceable. Ultimately, QA Analysts not only ensure compliance but also elevate the user experience by providing valuable insights that AI tools may overlook.
The Importance of Human Insight in Quality Assurance
Human insight remains a crucial element in quality assurance, complementing the emerging capabilities of AI. While AI can enhance efficiency, it lacks the nuanced understanding that human QA analysts bring to the table. The complexities of human experience, emotions, and context cannot merely be coded into algorithms, thus emphasizing the irreplaceable role of QA analysts.
When it comes to evaluating software quality, human analysts connect dots that AI might overlook. Their ability to interpret ambiguous user feedback and recognize subtle user experience issues ensures that quality is not merely about functionality but also about user satisfaction. For example, QA analysts can assess the emotional impact of a software feature, something AI cannot adequately measure. Therefore, the synergy between AI technologies and QA analysts is essential, ensuring that products not only meet technical criteria but also resonate well with users. This unique blend of human insight and AI efficiency helps foster better products and, ultimately, satisfied customers.
AI vs QA Analysts: Can AI Take Over?
As we delve into the discussion of AI vs QA Analysts, the question emerges: can AI take over this critical role? The capabilities of AI in software testing are both impressive and concerning, prompting a thorough exploration of its potential to replace human analysts. While AI can deliver exceptional speed and efficiency, it often lacks the deep contextual understanding and intuitive judgement that seasoned QA analysts bring to the table.
One major advantage of AI is its ability to analyze vast amounts of data quickly and consistently, enabling scalability that human analysts may struggle to achieve. However, reliance on initial programming means AI might not grasp nuanced situations, leading to potential inaccuracies in judgment. Thus, while AI tools can significantly enhance the QA process, the importance of human oversight remains paramount. Achieving a productive collaboration between AI and QA analysts could be the key to leveraging the strengths of both entities effectively.
Extract insights from interviews, calls, surveys and reviews for insights in minutes
Advantages of AI in QA
AI's role in quality assurance presents several compelling advantages that can support, but not replace, QA analysts. One significant benefit is speed and efficiency. AI can automate repetitive testing tasks, enabling quicker identification of bugs and performance issues. This capability not only streamlines the QA process but also allows analysts to focus on areas requiring human intuition and expertise, enhancing overall productivity.
Another advantage lies in scalability and consistency. AI tools can handle extensive testing across multiple environments simultaneously, ensuring that quality standards remain high regardless of project size. This level of automation helps maintain consistency in testing results, minimizing human error and subjectivity. While AI exhibits remarkable capabilities, it lacks the contextual understanding that seasoned QA analysts provide, which is vital for nuanced decision-making in quality assurance. Recognizing these strengths and limitations is essential when considering AI vs QA analysts in any software testing strategy.
- Speed and Efficiency
In the realm of software testing, speed and efficiency are pivotal. AI technology excels in processing vast amounts of data quickly, significantly outpacing human capabilities. For instance, while a QA analyst might take hours or days to execute tests and analyze results, AI can perform the same functions in mere minutes. This rapid turnaround allows organizations to identify potential issues early in the development cycle, enhancing overall productivity.
Moreover, the automation of routine tasks by AI allows QA analysts to focus on more complex and nuanced aspects of testing. Humans bring valuable contextual understanding and critical thinking to the table, but they cannot match AI's ability to work tirelessly and consistently. As the landscape of quality assurance evolves, the integration of AI resources is set to transform how QA analysts operate, allowing for a more efficient testing process while still leveraging human insight to guide decision-making.
- Scalability and Consistency
Scalability and consistency are two crucial aspects where AI technologies show significant promise in the realm of quality assurance. As organizations grow, they accumulate vast amounts of data from user interactions, which traditional QA methods struggle to process effectively. AI tools can analyze this data at scale, providing insights that manual processes may overlook. Companies utilizing AI can expect quicker turnaround times, ensuring that quality assurance keeps pace with rapid development cycles.
However, the challenge lies in maintaining consistent output in AI systems. AI algorithms can produce varying results based on the input they receive. This inconsistency may lead to unreliable quality checks if not meticulously monitored. Therefore, while AI can enhance scalability in QA processes, human oversight is essential to maintain the intended quality standards.
Together, AI and human input can forge a path to a more efficient, scalable, and reliable QA environment. By understanding both the strengths and limitations of AI, organizations can better navigate the evolving landscape of software quality assurance.
Limitations of AI Compared to QA Analysts
AI cannot fully replace QA analysts due to several inherent limitations. One significant challenge is AI's lack of contextual understanding. While AI excels at processing data, it often fails to grasp the nuances of human experience and emotions, which are crucial when assessing user interactions. Human QA analysts can interpret subtle user feedback and adapt their strategies based on intricate details of the overall context, something AI struggles with.
Another limitation is the dependence on initial programming and existing biases in AI models. They operate within the confines of their training data and can inadvertently perpetuate biases reflected in that data. In contrast, QA analysts bring a human perspective that allows them to question assumptions, explore edge cases, and innovate solutions beyond preset patterns. Therefore, the dynamic capabilities of QA analysts remain essential for comprehensive quality assurance in software testing.
- Lack of Contextual Understanding
When considering AI vs QA Analysts, a significant drawback for AI is its lack of contextual understanding. Unlike experienced QA analysts, AI lacks the ability to grasp nuanced situations or assess the broader implications of a defect. This limitation can lead to inadequate testing results, where AI may overlook critical context that only a human can perceive. Quality assurance often requires an understanding of user perspectives and experiences, an area where AI fundamentally struggles.
Moreover, QA analysts rely on their experience to navigate complex scenarios and adapt to unique challenges. They can identify the underlying issues in customer dissatisfaction, driven by factors beyond the immediate technical concerns. While AI can process vast amounts of data quickly, it cannot replicate the intuitive and empathetic insights that human analysts bring to the table. Consequently, while AI tools can enhance efficiency and accuracy in specific tasks, the absence of contextual understanding in AI limits their potential to fully replace QA analysts in the quality assurance process.
- Dependence on Initial Programming and Bias
Initial programming and inherent biases significantly influence AI systems, affecting how they perform in quality assurance roles. AI is trained based on pre-defined algorithms and datasets, meaning its performance is closely tied to the quality and diversity of the input data it receives. If initial datasets contain biases or gaps, the AI will likely perpetuate those issues, leading to imperfect testing outcomes. This limitation contrasts with the nuanced, contextually aware approach of traditional QA analysts, who can adapt to unique scenarios and user requirements.
Moreover, biases embedded in AI systems can undermine the reliability of testing results. Algorithms may favor certain outcomes or overlook critical test cases, resulting in a skewed analysis. Unlike QA analysts, who utilize intuition and comprehensive understanding, AI lacks the cognitive abilities to interpret complex scenarios in testing environments. This dependence on initial programming and the risk of bias raise crucial questions about whether AI can truly replace QA analysts in ensuring software quality and functionality.
Tool Comparison: Exploring AI Options for QA
When exploring AI options for quality assurance, a critical evaluation of various tools is essential. Each AI solution offers unique features that can enhance testing processes, but their suitability may vary depending on specific project needs. For instance, Insight7 provides remarkable capabilities in recording and analyzing data on a large scale. Its user-friendly interface allows teams to swiftly adapt without extensive training, making it a strong contender in the AI vs QA Analysts debate.
Other prominent tools, such as Testim and Applitools, also offer distinct advantages like automated visual testing and seamless integration capabilities. It's vital to consider criteria like ease of integration and support needs when comparing these tools. By assessing these factors, organizations can identify the right tools that support their QA processes. Understanding the strengths and weaknesses of each AI option can help ensure effective collaboration between automated systems and human analysts.
Top AI Tools for Quality Assurance
Identifying the right AI tools for quality assurance can significantly enhance testing processes and outcomes. AI-driven solutions like Insight7, Testim, and Applitools are gaining traction for their ability to automate routine tasks. These tools help QA analysts save time while ensuring higher accuracy in testing protocols. By using advanced algorithms, these solutions not only automate testing but also provide insights that can influence product development and quality improvements.
When comparing tools, consider factors such as ease of integration and ongoing support. For instance, tools like Functionize and Mabl offer seamless setups that enhance collaboration between AI and human QA analysts. This collaboration ensures that while AI handles volume and speed, analysts can focus on critical judgment and contextual understanding. Ultimately, the right AI tools do not replace QA analysts but instead serve to augment their effectiveness in ensuring software quality.
- Insight7: Leading the Charge in AI-Driven QA
In the evolving landscape of software testing, AI is increasingly stepping up to transform quality assurance (QA). As organizations seek faster insights and enhanced efficiency, AI offers tools that automate extensive testing processes, promising quicker turnaround times for deployments. This capability can significantly benefit companies striving to remain competitive in a fast-paced digital world. However, while automation tools can streamline repetitive tasks, they must work hand-in-hand with human analysts who provide essential contextual understanding.
AI-driven QA technologies excel in speed and scalability, handling vast amounts of data more effectively than human counterparts. Yet, the nuanced decision-making and insights that QA analysts contribute remain invaluable. Human intuition can identify unnoticed patterns and implications that a purely algorithmic approach might miss. Thus, the discussion of AI vs QA Analysts highlights a collaborative future where both elements synergize to ensure software quality, ultimately leading to superior customer outcomes.
- Testim
In the realm of AI vs QA Analysts, insights reveal the capabilities and roles of automation in testing processes. One of the noteworthy tools in this space specializes in automating quality assurance tasks, streamlining workflows for efficiency. It allows users to conduct evaluations effortlessly by executing tests based on customizable templates that align with various compliance standards. This capability not only optimizes accuracy but also speeds up the initial assessment phases.
However, while automation undeniably enhances productivity, it lacks the nuanced understanding that human QA Analysts provide. For instance, human testers can discern complexities and emotions embedded in user interactions, which AI may overlook. The tool excels at handling repetitive tasks and ensures thorough testing coverage, yet it is crucial to balance its usage with human insight to ensure a well-rounded quality assurance strategy. This collaborative approach stands to elevate the effectiveness of testing in software development.
- Applitools
In the ongoing discussion of AI vs QA Analysts, Applitools emerges as a noteworthy tool that enhances automated visual testing. This technology allows teams to streamline the process of visual verification, which is essential for delivering a consistent user experience across diverse platforms. By utilizing advanced AI algorithms, it identifies visual discrepancies that may otherwise escape conventional testing methods.
Moreover, Applitools not only supports the automation of visual tests but also integrates seamlessly into existing development workflows. Its capability to perform comprehensive checks across device types amplifies efficiency and precision in testing. While AI tools like this provide significant advantages in terms of speed and accuracy, they are not infallible. The subjective nature of user experience still requires human insight, making the collaboration between AI tools and QA Analysts vital for optimal outcomes. This synergy underscores the ongoing debate about whether AI can fully replace QA Analysts or merely serve as a sophisticated assistant in their work.
- Functionize
Functionize stands out as a notable contender in the conversation around AI versus QA analysts. This platform leverages advanced AI to automate various aspects of testing, rigorously analyzing software functionality while reducing human error. By employing techniques such as natural language processing and machine learning, it streamlines the testing process and enhances efficiency, significantly decreasing the time required for product validation.
However, it's essential to recognize that while Functionize excels in speed and scalability, it may lack the nuanced understanding that a QA analyst possesses. Human insight plays a critical role in discerning complex issues that AI might overlook, especially in scenarios requiring contextual awareness. The balance between AI and QA analysts is vital; thus, Functionize should be seen as a complement to skilled analysts, rather than a complete substitute. By combining the strengths of AI with human expertise, businesses can achieve more thorough and reliable quality assurance outcomes.
- Mabl
Mabl is a notable player in the realm of AI-driven quality assurance. It offers automated testing solutions designed to simplify the testing process while ensuring robust product quality. By employing machine learning, this tool enables users to create tests that adapt over time, addressing the dynamic nature of software development.
While discussing AI vs QA Analysts, it's important to highlight Mabl's advantages in enhancing test efficiency and maintaining consistent performance. The platform excels in allowing teams to execute tests quickly without compromising accuracy, making it invaluable in fast-paced environments. However, it should be noted that Mabl, like other AI tools, may lack the nuanced understanding and contextual judgment that human QA analysts offer. Thus, a hybrid approach, blending automated tools with human oversight, might prove most effective in achieving comprehensive quality assurance.
Criteria for Evaluating AI Tools for QA
Evaluating AI tools for quality assurance involves a clear set of criteria essential for assessing their value compared to traditional QA analysts. First, ease of integration is crucial; an AI tool should seamlessly adapt to existing workflows without significant disruption. Second, support and maintenance needs should be minimal, allowing teams to focus on quality assurance tasks rather than troubleshooting issues.
Additionally, assessing the tool's capability in data accuracy is key. Tools must efficiently process data while minimizing errors. Finally, evaluating the AI's capability for contextual understanding is vital. AI tools should demonstrate an ability to comprehend the nuances of QA tasks, as their effectiveness hinges on understanding specific industry requirements. These criteria help outline the strengths and limitations of AI in the debate of AI vs. QA analysts, guiding organizations in making informed decisions about their quality assurance strategies.
- Ease of Integration
Integrating AI into quality assurance processes is essential for maximizing efficiency. When examining AI vs QA Analysts, ease of integration plays a significant role in determining the effectiveness of AI tools. Companies seek solutions that minimize disruption while enhancing operational workflows.
AI tools designed for QA can often be seamlessly integrated into existing systems, allowing analysts to transition smoothly into a hybrid working model. With user-friendly interfaces and minimal training requirements, these technologies empower QA teams to harness AI capabilities without extensive technical knowledge. Moreover, the ability to centralize data input from multiple sources enhances overall analysis and reporting, streamlining the workflow.
By carefully assessing the ease of integration of AI tools, businesses can enhance their QA processes without sacrificing the valuable insights provided by human analysts. This balance ultimately leads to improved product quality and a more efficient testing lifecycle, combining the best of both worlds.
- Support and Maintenance Needs
In evaluating the support and maintenance needs of AI tools in the realm of quality assurance, it's crucial to consider how these tools interact with human QA analysts. Both require ongoing maintenance to function effectively, but the nature of these needs differs significantly. While AI tools can automate routine tasks and offer scalability, they still require regular updates and monitoring to ensure accuracy and alignment with evolving business needs.
Human QA analysts play a vital role in bridging the gaps where AI falls short, particularly in areas requiring contextual understanding. For instance, AI tools often depend on well-defined parameters, making them less effective in nuanced scenarios. Moreover, when selecting AI tools for Quality Assurance, one must consider key factors such as ease of integration and ongoing technical support. Regular training and adjustments based on human feedback remain essential, reinforcing the collaborative potential of AI and QA analysts rather than viewing them as replacements.
Use Cases: AI vs QA Analysts in Real-world Applications
In real-world applications, the dynamic between AI and QA analysts reveals an evolving landscape of collaboration and innovation. Notably, AI-driven quality assurance tools excel in large enterprises, where they automate repetitive tasks and analyze vast data sets at unprecedented speeds. This efficiency enables QA teams to focus on strategic analysis instead of mundane testing processes, enhancing overall productivity.
However, the value of QA analysts cannot be understated. Human expertise brings essential contextual understanding to the testing process, allowing for nuanced evaluations that AI tools may overlook. For example, a QA analyst can interpret customer behavior and provide insights on user experience that AI lacks. In this comparative landscape, organizations successfully utilize AI to complement the skills of QA analysts, ensuring both speed and human insight in achieving superior quality outcomes. Thus, the integration of AI vs QA analysts not only optimizes processes but also enriches the end-product quality.
AI-Driven QA in Large Enterprises
In large enterprises, the integration of AI-driven quality assurance (QA) is transforming traditional testing methodologies. AI systems can provide significant enhancements in terms of speed and efficiency, automating repetitive tasks while ensuring high standards. However, AI vs QA Analysts presents a complex dynamic where collaboration often yields the best results. While AI can process vast amounts of data swiftly, it lacks the contextual understanding that human analysts bring to the table.
The symbiotic relationship between AI and QA analysts is vital for success in large organizations. Firstly, AI enhances scalability, making it easier to manage extensive testing processes across diverse environments. Secondly, QA analysts contribute unique insights into user behavior and application nuances, which AI tools currently struggle to grasp. By combining their strengths, enterprises can achieve a comprehensive quality assurance strategy that meets both speed and contextual accuracy requirements. Thus, rather than replacing QA analysts, AI seeks to assist and empower them in delivering superior software quality.
Success Stories: Collaborative Efforts Between AI and Human QA
In recent times, numerous organizations have successfully combined the strengths of AI and human QA analysts, leading to remarkable outcomes in quality assurance. One such success story involves the implementation of AI algorithms capable of analyzing vast datasets of customer interactions. This technology significantly reduced the time taken to identify trends and potential issues. Meanwhile, human QA analysts brought their expertise in interpreting these findings, adding contextual insights and understanding the nuanced nuances that AI might overlook.
Another example highlights a project where AI tools facilitated rapid initial testing, flagging potential issues for deeper investigation. Following the AIโs analysis, QA analysts conducted thorough reviews, ensuring attention to detail and maintaining quality standards. The collaboration of AI and humans enriched the QA process, elevating product quality beyond what either could accomplish alone. These stories illustrate that rather than a contest of AI vs QA analysts, the synergy between technology and human insight drives the industry forward.
Conclusion: The Future of AI vs QA Analysts in Software Testing
Looking ahead, the future of AI vs QA Analysts in software testing presents a fascinating dynamic. While AI brings unmatched speed and efficiency to the testing process, it lacks the nuanced understanding that human QA analysts provide. This dichotomy suggests a collaboration rather than a competition, as both elements play crucial roles in delivering quality software.
As AI continues to evolve, it is likely to take on more repetitive tasks, freeing QA analysts to focus on complex problem-solving and creative thinking. By embracing AI as a partner, QA analysts can enhance their effectiveness and ensure that software testing remains thorough and insightful. Together, they pave the way for a more efficient and reliable software development landscape.