Conversational Quality Analysis is becoming essential as businesses aim to extract meaningful insights from customer interactions. In a world where customer feedback is generated at an unprecedented scale, the ability to assess conversation quality effectively can make a significant difference. Companies are inundated with data from various communication channels, making traditional analysis methods inadequate.
Harnessing AI for this purpose can streamline how organizations examine conversations. By automating the evaluation of dialogue, companies can uncover patterns and trends that inform strategic actions. Through Conversational Quality Analysis, businesses not only improve customer relationships but also enhance their competitive advantage in a rapidly evolving marketplace.
Analyze & Evaluate Calls. At Scale.
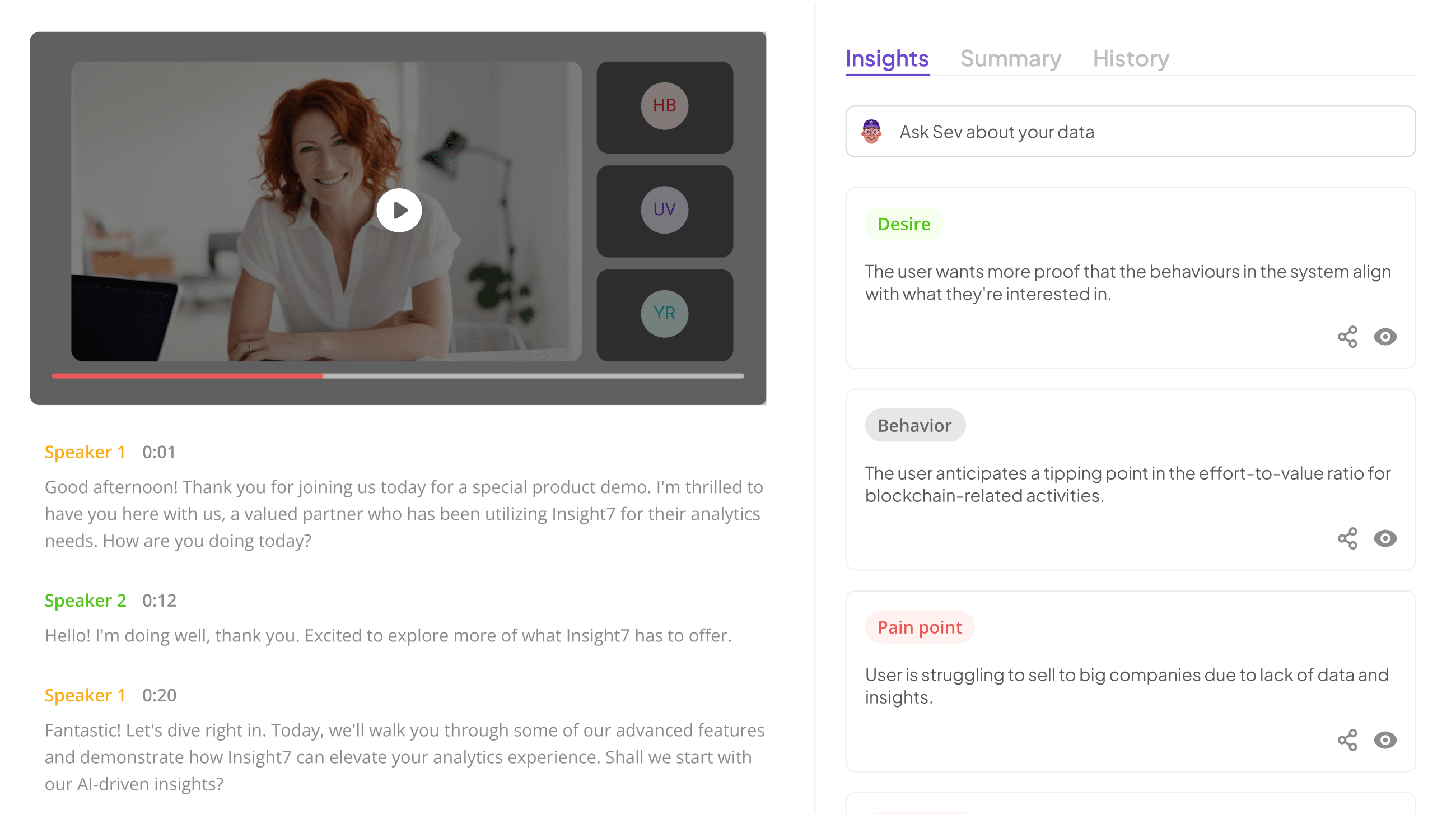
The Role of AI in Conversational Quality Analysis
AI plays a crucial role in enhancing Conversational Quality Analysis, making it possible to effectively evaluate large volumes of customer interactions. By processing extensive datasets, AI enables organizations to uncover valuable insights from conversations that would otherwise remain buried in raw data. Conventional methods often fall short, leaving valuable customer signals overlooked or misinterpreted.
Through natural language processing and sentiment analysis, AI can evaluate tone, context, and engagement levels in conversations. This allows businesses to gain deeper insights into customer needs and preferences. Additionally, AI systems can automate tedious tasks, like transcribing and categorizing conversations, significantly speeding up the analysis process. As organizations increasingly depend on timely and accurate insights, the efficiency brought by AI positions them to outperform their competitors. Ultimately, AI-driven Conversational Quality Analysis not only transforms raw data into strategic actions but also enhances customer engagement, helping businesses build stronger relationships with their audiences.
Understanding Conversational Quality Analysis
Conversational Quality Analysis focuses on evaluating the quality of interactions between individuals or systems. This analysis encompasses various elements, such as clarity, engagement, and adherence to guidelines. By systematically assessing these components, businesses can determine the effectiveness of their communication strategies and identify areas for improvement.
One crucial aspect of Conversational Quality Analysis is defining evaluation criteria. This can include aspects like understanding customer needs and providing relevant information. Additionally, it is essential to utilize technology to streamline the collection and analysis of conversational data. By doing so, organizations can gain insights into conversation patterns and customer sentiment. Implementing such analysis allows companies to enhance the overall customer experience, ultimately leading to improved satisfaction and loyalty. With the evolution of AI tools, measuring conversation quality at scale has never been more attainable.
Steps for AI-Driven Conversational Quality Analysis
To implement AI-driven Conversational Quality Analysis effectively, it's vital to follow a structured approach. First, initiate by gathering all relevant conversation data from various channels. This data typically includes recording and transcribing interactions to ensure comprehensive insights. Once the conversations are transcribed, the next step is to categorize the discussions into specific evaluation criteria, such as compliance, customer service, or sales effectiveness.
Following categorization, employ AI algorithms to analyze these conversations against the established criteria. This analysis can highlight key metrics, identify recurring themes, and extract significant quotes from participants. Finally, generate detailed reports summarizing the findings, which can aid in pinpointing areas for improvement and refining future interactions. By methodically applying these steps, organizations can scale their Conversational Quality Analysis while improving customer engagement and service performance efficiently.
Tools for Measuring Conversational Quality at Scale
In today's fast-paced digital environment, tools for measuring conversational quality at scale are essential for businesses to stay competitive. These tools enable organizations to analyze customer interactions effectively and derive meaningful insights. By utilizing AI-driven platforms, companies can process vast amounts of conversation data, allowing them to identify trends, customer sentiments, and areas for improvement.
Several tools are suited for this task. First, AI-based analytics platforms streamline the analysis of customer interviews, making it easier to extract valuable insights. Second, speech recognition technologies facilitate the review of verbal interactions, highlighting key moments in conversations. Finally, sentiment analysis tools evaluate emotional tones, providing a deeper understanding of customer experiences. Each of these tools contributes significantly to comprehensive conversational quality analysis, empowering businesses to respond proactively to customer needs.
Extract insights from interviews, calls, surveys and reviews for insights in minutes
insight7 for Conversational Quality Analysis
Insight7 serves as a pivotal platform for Conversational Quality Analysis, enabling companies to analyze customer interactions at scale effectively. As organizations accumulate vast amounts of customer conversations, traditional analysis methods fall short. With insights often scattered across files, businesses struggle to derive actionable strategies from these interactions. Insight7 addresses these challenges by providing a user-friendly, self-service platform that harnesses data from interviews and conversations, helping organizations synthesize valuable insights quickly.
💬 Ask About This Article
Have questions? Get instant answers about this article.
Through its efficient analysis capabilities, the platform empowers customer service teams to engage proactively with clients, enhancing the quality of conversations. This transformation fosters a deeper understanding of customer needs, allowing organizations to tailor their approaches and strategies effectively. As businesses shift towards data-driven decision-making, the value of Conversational Quality Analysis becomes increasingly evident. By leveraging Insight7, companies can stay competitive and insightful in their customer interactions, ensuring they turn conversations into meaningful actions.
Other Leading Tools for Analyzing Conversations
In the realm of analyzing conversations, various tools excel in achieving high standards of Conversational Quality Analysis. These tools are designed to streamline the process and provide insightful feedback, ensuring that organizations can understand interactions on a deeper level. By integrating advanced analytics, these solutions facilitate a more efficient examination of dialogues, enabling businesses to make informed decisions based on clear data and trends.
One notable tool employs natural language processing capabilities, effectively identifying sentiment and key themes within conversations. This allows companies to gauge customer satisfaction in real-time and adapt their strategies accordingly. Another tool specializes in transcription and analysis, transforming raw dialogue into actionable insights that can be easily shared and discussed among teams. Lastly, a third option focuses on collaborative features, enhancing communication within organizations to ensure that valuable insights do not get lost in individual silos. Each of these tools plays a crucial role in refining overall communication, paving the way for improved customer engagement and satisfaction.
- Tool 1
Tool 1 introduces a powerful solution designed to enhance the accuracy and efficiency of Conversational Quality Analysis. This tool can automatically analyze dialogues, pinpointing nuances in communication that may otherwise go unnoticed. By employing advanced algorithms, it parses conversations to identify trends, sentiment, and adherence to predefined quality metrics, providing invaluable insights.
Users will find that this tool is adaptable to various contexts, effectively evaluating different scripts and scorecards tailored for diverse clients. As conversations unfold, the tool captures essential data, enabling organizations to refine their communication strategies. Ultimately, Tool 1 offers a streamlined approach to measuring conversation quality at scale, ensuring that businesses can maintain high standards and continuously improve customer engagement. It serves as a pivotal component in modern analytics, transforming raw data into actionable insights that drive success.
- Tool 2
In exploring the realm of conversational analysis, Tool 2 stands out for its robust capabilities in measuring conversation quality at scale. This tool is designed to assess key metrics such as engagement, clarity, and relevance within conversational exchanges. By utilizing advanced AI algorithms, it provides valuable insights that can help organizations refine their communication strategies.
One of the notable features of Tool 2 is its ability to process large volumes of data efficiently. This means organizations can quickly analyze interactions across various platforms, gathering insights on customer sentiment and conversation flow. Additionally, its customizable scoring system allows teams to tailor evaluations based on specific client needs. Such adaptability enhances the tool's effectiveness in different contexts, ensuring that it meets varied conversational objectives. Ultimately, Tool 2 plays a critical role in enabling businesses to enhance their conversational quality analysis and improve overall customer experience.
- Tool 3
Tool 3 offers a unique approach to Conversational Quality Analysis by utilizing advanced machine learning algorithms. This tool can automatically assess multiple conversations simultaneously, providing insights into key metrics such as engagement level, clarity, and emotional tone. By analyzing these factors, organizations can better understand how effectively their representatives connect with clients.
To maximize the benefits of this tool, consider the following aspects: First, identify specific criteria for evaluation based on your unique scripts and scorecards. Second, integrate the insights gleaned from the tool into training programs for your team, ensuring they constantly improve their interactions. Lastly, leverage the data to inform decision-makers about ongoing performance trends. As AI continues to evolve, tools like this will become pivotal in shaping conversational strategies, ultimately enhancing customer satisfaction and loyalty.
Conclusion: The Future of Conversational Quality Analysis with AI
As we look toward the future of Conversational Quality Analysis with AI, several possibilities emerge. The integration of advanced AI technologies can significantly enhance the accuracy and efficiency of measuring conversation quality at scale. Sophisticated algorithms will analyze discourse patterns, identify key performance indicators, and deliver actionable insights swiftly, enabling businesses to make informed decisions.
Moreover, the adaptability of AI systems will allow organizations to customize their assessment criteria based on specific goals. As AI continues to evolve, it promises to revolutionize how we understand and improve communication strategies, fostering deeper connections and stronger relationships between organizations and their clients. The future of Conversational Quality Analysis is not only bright but also filled with immense potential for growth and innovation.
💬 Ask About This Article
Have questions? Get instant answers about this article.