Can AI Detect Customer Frustration in Call Tone?
-
Hello Insight
- 10 min read
Frustration Detection AI is revolutionizing the way call centers interact with customers. Imagine a scenario where an agent can sense a customer’s frustration during a call, enabling timely intervention to resolve issues before they escalate. This technology analyzes vocal tones, pitch, and speech patterns to gauge emotional states, offering insights that can lead to enhanced customer satisfaction.
As businesses face increasing demands for effective communication, Frustration Detection AI provides a proactive solution. By employing advanced algorithms, this AI-driven approach helps in understanding customer emotions, fostering improved engagement. Ultimately, recognizing frustration early can transform the customer experience and bolster a company’s reputation.
Transcribe & extract insights from interviews. At Scale.
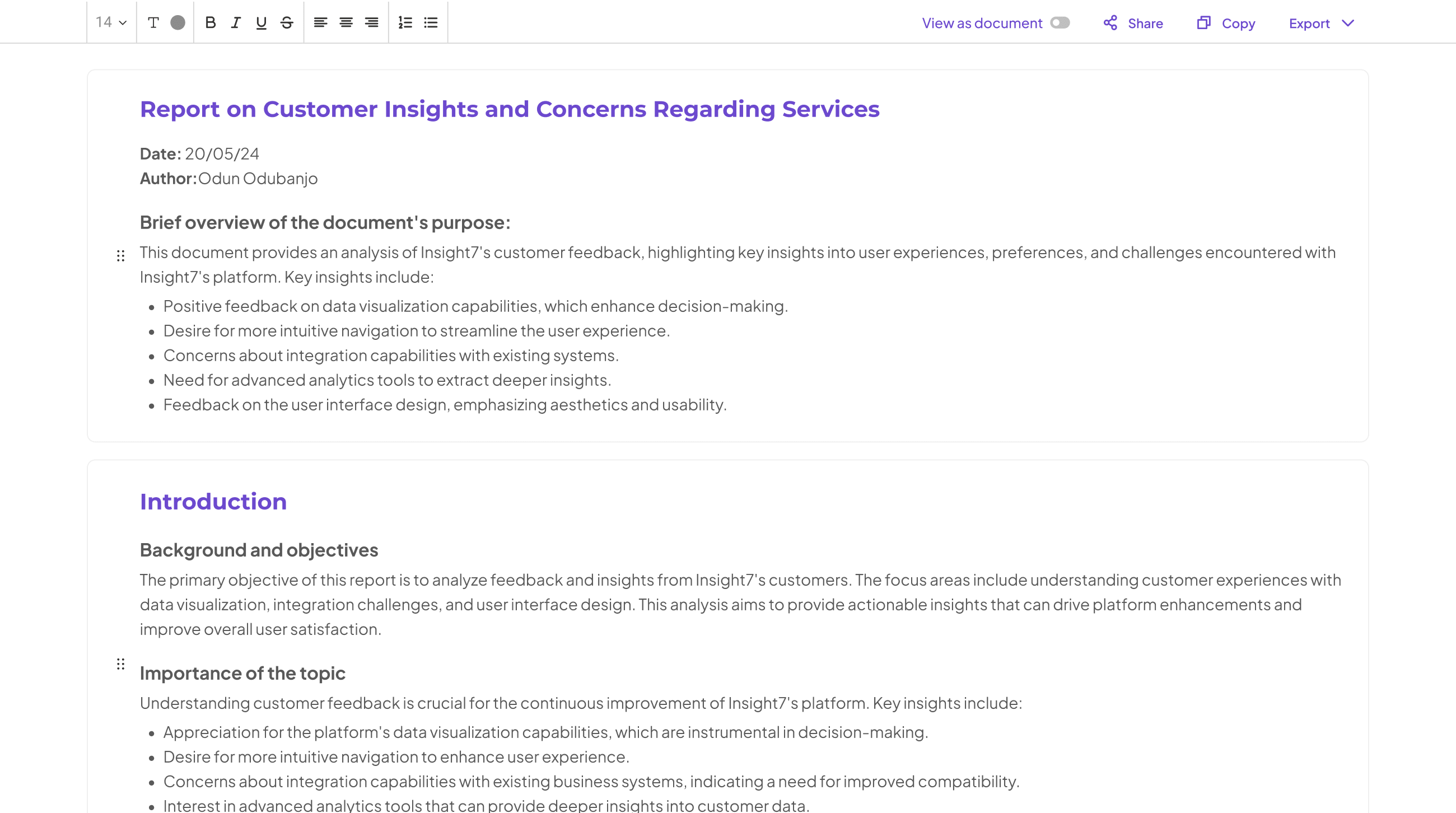
How Frustration Detection AI Works in Identifying Customer Emotion
Frustration Detection AI employs sophisticated speech analysis techniques to understand customer emotions effectively. By analyzing vocal patterns, tone, and pitch, this technology can detect underlying frustration during interactions. Machine learning algorithms play a crucial role in interpreting these emotional cues, allowing companies to respond proactively to customer needs and improve service quality.
The development of Frustration Detection AI involves several critical steps. First, data collection and labeling are conducted to create a robust training set. Next, models undergo rigorous training and testing to enhance accuracy and reliability in real-world applications. Finally, real-time integration and a feedback mechanism ensure that the system continuously evolves, adapting to varying customer interactions. Through these methods, businesses can transform customer frustration into actionable insights, ultimately fostering a better customer experience and enhancing operational efficiency.
Speech Analysis Techniques
AI utilizes sophisticated speech analysis techniques to understand customer emotions during calls. These methods involve monitoring vocal patterns and tones, allowing the AI to discern signs of frustration effectively. For instance, variations in pitch, volume, and speech rate can indicate distress. By analyzing these vocal cues, frustration detection AI constructs a clearer picture of the customer's emotional state, enabling more informed responses from service agents.
To enhance this process, machine learning algorithms play a vital role. They are trained on substantial datasets of emotional expressions in speech, learning to identify and interpret subtle emotional signals. Over time, these algorithms refine their ability to differentiate between frustration and other emotions, ensuring more accurate assessments. Consequently, these speech analysis techniques provide organizations with valuable insights, paving the way for improved customer experiences.
- Discuss how AI analyzes vocal patterns and tone to detect frustration.
AI analyzes vocal patterns and tone by employing advanced speech analysis techniques to identify signs of frustration in customer interactions. The technology captures various voice attributes, such as pitch, volume, and speech rate, which are indicators of emotional stress. For instance, a raised voice or rapid speech can signify agitation. By recognizing these vocal changes, Frustration Detection AI can ascertain when a customer may be experiencing dissatisfaction.
Additionally, machine learning algorithms play a vital role in interpreting these emotional cues. They are trained on vast datasets containing labeled examples of frustrated and non-frustrated speech. This training enables the AI to refine its ability to detect nuanced vocal inflections that typically accompany frustration. Consequently, businesses can proactively address customer concerns, enhancing overall service efficiency by identifying issues before they escalate.
- Explore machine learning algorithms roles in interpreting emotional cues.
Machine learning algorithms play a pivotal role in interpreting emotional cues, particularly in contexts like frustration detection. By analyzing patterns in speech, these algorithms can effectively identify signs of frustration in customer interactions. Frustration Detection AI utilizes techniques such as vocal tone analysis and speech pattern recognition to discern emotions, providing insights that help enhance customer experience.
The process begins with extensive data collection, where diverse voice samples are recorded and labeled based on emotional content. Following this, machine learning models are trained on these datasets, allowing them to recognize subtle emotional indicators. As these models are tested and refined, they can seamlessly integrate into call center operations, offering real-time feedback and interaction analysis. This approach not only enables a deeper understanding of customer emotions but also fosters proactive measures to address dissatisfaction promptly. By leveraging Frustration Detection AI, organizations can significantly improve customer satisfaction and loyalty.
Steps in Developing Frustration Detection AI
To develop effective Frustration Detection AI, several critical steps must be undertaken. Initially, data collection and labeling play an essential role. This involves gathering a comprehensive dataset containing diverse customer interactions, both frustrated and satisfied. Proper labeling of this data enables the AI to learn and differentiate between emotional tones, which is pivotal for accurate detection.
The second step is model training and testing. In this phase, machine learning algorithms are utilized to analyze the labeled dataset. The AI must refine its ability to recognize vocal patterns and emotional cues associated with frustration, ensuring that it can accurately identify subtle differences in call tone.
Finally, real-time integration and feedback mechanisms are essential. The AI system should easily integrate into existing communication platforms. Implementing a feedback loop allows for continuous improvement of the model, enabling adjustments based on new data and refining its ability to detect frustration over time. These steps collectively create a robust framework for effective Frustration Detection AI in customer service settings.
- Step 1: Data Collection and Labeling
The journey of developing Frustration Detection AI begins with the critical phase of data collection and labeling. To train an effective AI model, it is essential to gather a diverse set of audio recordings from customer interactions. This data should include examples of varying customer emotions including frustration, satisfaction, and neutrality. Ensuring that these recordings accurately represent different tones and contexts will provide the foundation needed for precise analysis.
Once the data is collected, the labeling process comes into play. Each audio clip must be annotated with the respective emotional state it represents. This could involve marking phrases or tones indicative of frustration versus calmness. Precise labeling not only helps the AI recognize patterns within the data but also plays an essential role in improving the model's recognition accuracy over time. By laying this groundwork, organizations can significantly enhance their capability to detect customer frustration in call tone.
- Step 2: Model Training and Testing
Model training and testing is a crucial phase in developing a reliable Frustration Detection AI. In this step, algorithms are trained on datasets that include various vocal patterns and tones, which enables them to recognize signs of customer frustration effectively. The training process utilizes labeled data, where instances of frustration are tagged, allowing the AI to learn from these examples.
Once the model is trained, testing involves evaluating its performance on a separate dataset to measure accuracy and reliability. During testing, the AI's ability to identify frustrated tones is assessed, helping to refine its algorithms. This iterative process of training and testing ensures that the Frustration Detection AI evolves to provide actionable insights, ultimately enhancing customer experience in call centers. By focusing on this systematic approach, organizations can enhance their understanding of customer emotions and respond proactively to frustrations.
- Step 3: Real-time Integration and Feedback Mechanism
To establish an effective framework for real-time integration and feedback, organizations should focus on three key elements: continuous monitoring, responsive adjustments, and actionable insights. Continuous monitoring ensures that conversations are assessed in real-time, allowing the Frustration Detection AI to identify emotional signals as they occur. This generates an immediate understanding of customer sentiment and enhances the customer experience.
Responsive adjustments involve the AI system suggesting alterations in interaction strategies based on detected frustration levels. By adapting the script or tone, customer service representatives can defuse tensions effectively. Furthermore, actionable insights gathered from call data allow teams to refine their strategies continuously. Regular feedback processes, such as monthly reports highlighting trends in customer interactions, empower organizations to make informed decisions that address common issues and enhance service quality. This holistic approach makes it easier to turn customer feedback into opportunities for improvement.
Generate Detailed Reports from Your Qualitative Data in Minutes.
Top Tools for Frustration Detection AI
Frustration Detection AI tools are transforming the way businesses engage with customers. These advanced systems utilize sophisticated algorithms to analyze vocal characteristics, allowing them to infer emotional states such as frustration during calls. By assessing tone, pitch, and speech pace, these tools provide valuable insights that can significantly enhance customer service experiences.
Key players in this space include Cogito, which excels at analyzing interactions in real-time and providing actionable feedback. Beyond Verbal focuses on emotional analytics, offering deep insights into customer feelings. CallMiner Eureka processes sentiment with precision, evaluating customer satisfaction continuously. Lastly, NICE Nexidia applies robust methods to detect frustration and sentiment, helping businesses tailor their responses effectively. Utilizing these tools can lead to improved customer experiences and a better understanding of customer needs, ultimately fostering loyalty and satisfaction.
insight7
Frustration Detection AI plays a pivotal role in understanding customer emotions during call interactions. This technology analyzes vocal patterns and tones, providing insights into customers' emotional states. By examining fluctuations in pitch, speed, and volume, AI can effectively identify when frustration arises. This capability empowers businesses to address issues proactively and enhance customer satisfaction.
The benefits of implementing Frustration Detection AI are significant. First, real-time insights enable call center representatives to adapt their approach instantly. Second, it fosters a more empathetic customer service experience by anticipating and addressing frustration before it escalates. Finally, the analysis of emotional cues can lead to data-driven decisions that improve overall service strategies. By harnessing these insights, businesses can not only retain customers but also turn potentially negative experiences into opportunities for engagement and loyalty.
- Overview of features and benefits related to detecting customer frustration.
Frustration Detection AI offers several features that significantly enhance customer interaction analysis in call centers. This technology employs advanced speech analysis techniques, pinpointing vocal patterns and tone variations indicative of frustration. By interpreting these emotional cues, businesses can identify customer sentiments effectively and respond in real time.
The benefits of implementing such a system are substantial. First, it allows for immediate feedback and resolution strategies, ensuring that customer concerns are addressed promptly. Second, insights derived from Frustration Detection AI can inform training programs for customer service representatives, helping to refine their communication methods. Lastly, analyzing frustration trends over time enables businesses to improve product or service offerings, leading to enhanced customer satisfaction and loyalty. By investing in this technology, companies can create a more empathetic response framework, ultimately resulting in better customer experiences.
Other Leading Tools
In the realm of frustration detection, various advanced tools enhance the customer experience by identifying emotional signals during calls. One notable option is Cogito, which specializes in analyzing real-time call center interactions, offering insights into customer sentiments and frustrations. By leveraging adaptive AI, Cogito empowers representatives to engage more effectively with distressed customers, improving overall service quality.
Another essential tool is Beyond Verbal, which focuses on emotional analytics by evaluating vocal tone and inflections. This technology helps in understanding the emotional state of customers better, enabling tailored responses that meet their needs. Additionally, CallMiner Eureka provides robust sentiment analysis through its sophisticated processing capabilities, ensuring each customer’s concerns are addressed promptly. Finally, NICE Nexidia excels in sentiment detection by integrating seamlessly with various systems, allowing for comprehensive frustration detection and ensuring businesses maintain high customer satisfaction levels.
- Cogito: Describes its capabilities in analyzing call center interactions.
Cogito effectively analyzes call center interactions by employing advanced voice recognition and machine learning technologies. It captures vocal tone, pitch, and pace, which are essential in identifying signs of customer frustration. By converting these vocal attributes into quantifiable data, it offers actionable insights to improve training and enhance customer service quality.
In practice, Cogito processes thousands of calls, extracting key patterns and repetitive questions that indicate common customer issues. This information can be correlated with specific training modules, enabling teams to prepare their customer service representatives better. Furthermore, it helps managers gauge the emotional state of their callers in real-time, facilitating timely interventions to resolve frustrations. Ultimately, this Frustration Detection AI not only streamlines operations but also helps foster a more empathetic and responsive customer service environment.
- Beyond Verbal: Discusses its focus on emotional analytics.
Beyond Verbal explores the realm of emotional analytics by analyzing the nuances in customer interactions, specifically through their tone and vocal patterns. With the rise of Frustration Detection AI, the need for understanding emotions during customer communications has never been greater. This emotional insight allows organizations to proactively address customer grievances and enhance service interactions, leading to improved customer satisfaction.
Understanding the emotional context in conversations is crucial. Emotional analytics not only captures the essence of what customers are feeling but also provides actionable insights. By identifying stressors within customer communications, organizations can tailor their responses, ensuring a more empathetic approach. As businesses continue to adapt to the demands of their clients, integrating Frustration Detection AI into their systems offers a competitive edge in anticipating customer needs and improving overall service quality. In essence, this technology empowers companies to transform emotions into meaningful actions.
- Callminer Eureka: Highlights how it processes and evaluates customer sentiment.
Callminer Eureka highlights the sophisticated processes involved in evaluating customer sentiment. It excels at analyzing conversational data to identify tones and emotional cues indicative of frustration. By transforming spoken interactions into text, this platform applies advanced algorithms to assess factors like pitch, tone, and pacing, shedding light on customer emotions in real time.
The system categorizes voice data into projects, enabling a comparative analysis across various demographics or product interactions. This function allows businesses to gain insights that inform training and coaching efforts, ultimately enhancing customer engagement strategies. The nuanced analysis aids in transitioning call center teams from mere order-takers to consultative partners, better aligning services with customer needs. Such capabilities reinforce the toolkit for organizations leveraging Frustration Detection AI, propelling them toward improved customer satisfaction and loyalty.
- NICE Nexidia: Explains its application in sentiment and frustration detection.
NICE Nexidia plays a significant role in sentiment and frustration detection through advanced analytical capabilities. By leveraging AI-driven algorithms, the technology analyzes vocal tone, speech patterns, and contextual cues from customer interactions. This method allows organizations to identify subtle signs of frustration, enabling proactive customer service enhancements.
The application of frustration detection AI is crucial for improving customer relationships. By categorizing emotions from customer calls, businesses can better understand pain points and respond more effectively. Moreover, this technology supports the development of tailored strategies for engagement, ultimately leading to higher satisfaction rates. The integration of these insights helps organizations adapt operations, ensuring that they meet customer needs and mitigate frustration in real-time. Enhancing the customer experience in such a targeted manner can create lasting loyalty and trust.
Conclusion: Harnessing the Power of Frustration Detection AI for Improved Customer Experience
As we conclude, the potential of Frustration Detection AI in enhancing customer experiences is substantial. By accurately identifying emotional cues through vocal patterns, businesses can tailor their responses to mitigate customer issues effectively. This technology empowers organizations to discern frustration before it escalates, allowing timely interventions and smoother interactions.
Moreover, implementing Frustration Detection AI not only improves customer satisfaction but also fosters loyalty. By actively addressing emotions expressed during calls, companies can transform negative experiences into positive ones. Therefore, harnessing this technology is not just an innovation; it is a crucial step towards delivering exceptional customer service and understanding their needs better.