Can AI Detect Agent Frustration in Voice Calls?
-
Bella Williams
- 10 min read
Agent Frustration Detection is emerging as a critical area of interest in understanding the dynamics of voice calls. As customer service environments evolve, the emotional state of agents can significantly impact performance and customer interactions. Recognizing when agents feel frustrated may lead to timely interventions that enhance their well-being and improve service quality.
By employing advanced AI technologies, organizations can analyze voice calls to identify signs of stress or frustration in agents. This detection process can help managers make informed decisions, ultimately creating a supportive working environment that prioritizes both agent and customer satisfaction. Engaging with this technology provides valuable insights that can transform call center operations and client relationships.
Transcribe & extract insights from interviews. At Scale.
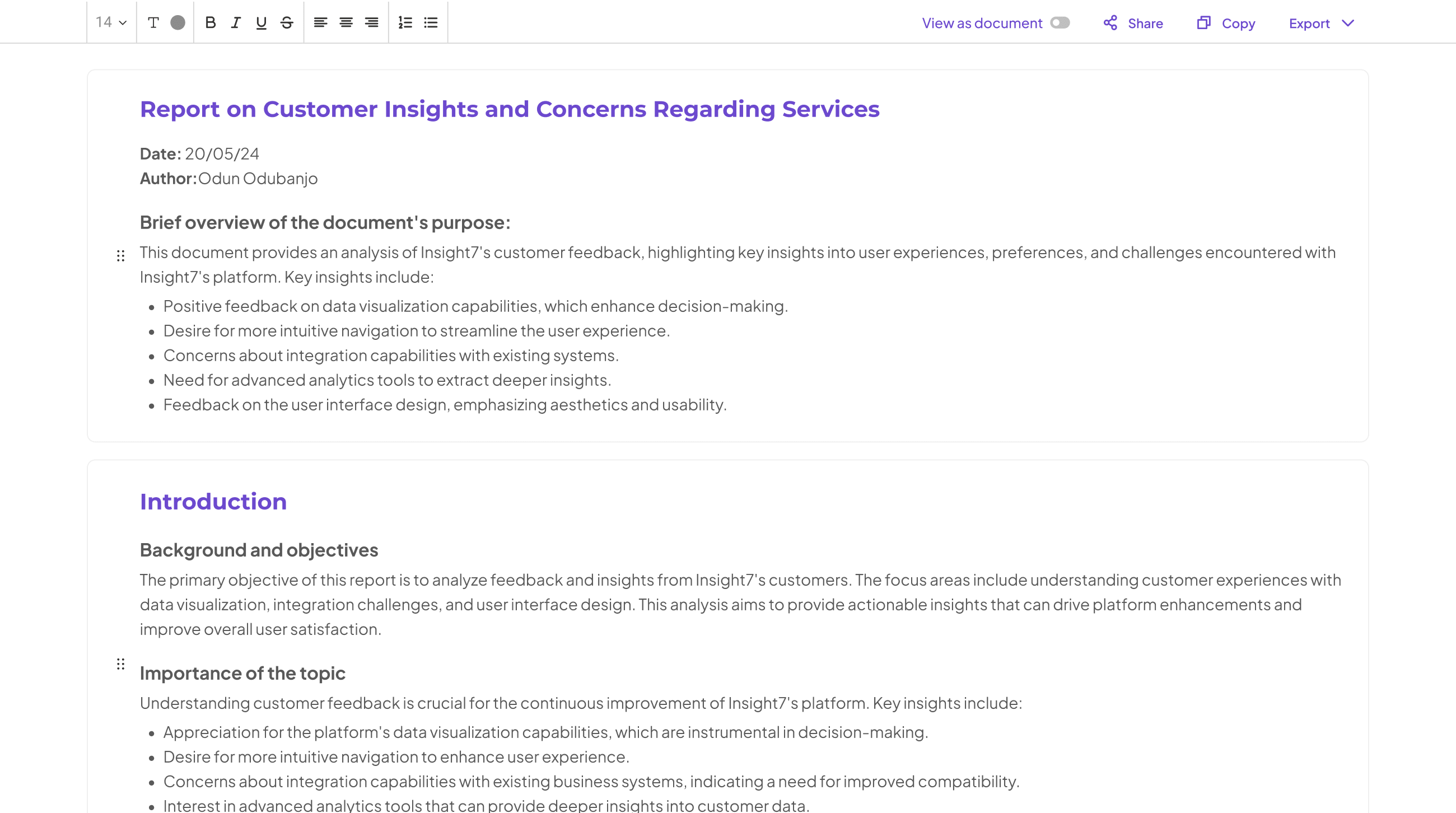
Understanding Agent Frustration Detection
Detecting agent frustration within voice calls is essential for maintaining effective communication. Understanding Agent Frustration Detection involves recognizing signals that indicate an agent's stress levels during interactions. This detection can arise from varied emotional cues, including tone of voice, speech patterns, and pauses in conversation. These elements can reveal when an agent is overwhelmed, allowing necessary interventions to improve their experience.
Recognizing these signs can have a significant impact on call center performance and employee satisfaction. The sooner we can identify an agent's frustration, the better equipped we are to address the situation. This could involve providing immediate support, adjusting workloads, or re-evaluating customer interactions. By comprehending how agent frustration manifests, we can enhance not only workplace morale but also overall client experiences. Effectively mitigating frustration ensures that agents remain productive and engaged, ultimately benefiting both the employees and the organization.
What is Agent Frustration Detection?
Agent Frustration Detection refers to the process of identifying emotional cues indicating when an agent may be experiencing frustration during voice calls. This detection can be crucial for improving customer interactions and enhancing operational efficiency. By closely analyzing tonal variations, speech patterns, and conversation dynamics, AI systems can assess whether the agent is becoming overwhelmed or agitated in real time.
Understanding agent frustration involves recognizing both verbal and non-verbal signals, which can be subtle yet impactful. For instance, shifts in voice pitch, hesitations, or abrupt changes in speech tempo can indicate distress. Furthermore, environmental factors, such as complicated customer requests or unexpected system issues, can exacerbate this frustration. By effectively identifying these signals, organizations can provide timely support to agents, fostering a more positive work environment and improving overall customer satisfaction.
The Importance of Detecting Agent Frustration
Detecting agent frustration is crucial in maintaining a positive working environment and ensuring high-quality customer service. When agents feel overwhelmed, it can adversely affect their performance, leading to miscommunication and customer dissatisfaction. Recognizing the signs of agent frustration can help organizations provide timely support and appropriate training, thereby fostering a more effective workforce.
Moreover, understanding agent frustration can lead to better resource allocation and improved interaction strategies. By incorporating effective measures to identify potential stressors, organizations can enhance both agent well-being and customer experiences. This proactive approach benefits everyone involved, leading to increased productivity and higher quality interactions. Ultimately, prioritizing agent frustration detection not only enhances workplace morale but also strengthens customer relationships, creating a positive loop of improvement and satisfaction.
💬 Questions about Can AI Detect Agent Frustration in Voice Calls??
Our team typically responds within minutes
Generate Detailed Reports from Your Qualitative Data in Minutes.
How AI Can Detect Agent Frustration in Voice Calls
AI can effectively detect agent frustration in voice calls through several advanced technologies that analyze verbal and non-verbal cues. Key technologies include natural language processing (NLP), tone analysis, and biometric feedback systems. These systems monitor voice patterns and emotional signals, enabling the identification of stress levels and frustration in real-time. By evaluating factors such as tone, pitch, and speech pace, AI can flag potential frustrations that may impair an agent's performance.
Implementing AI for agent frustration detection involves integrating these technologies into communication platforms. For example, the system can be trained to recognize keywords and phrases associated with frustration. This setup allows for instantaneous feedback, helping supervisors address agent stress before it escalates. Regular monitoring can also yield valuable insights into common sources of frustration, facilitating proactive solutions to enhance both agent well-being and customer service quality.
Key Technologies Behind Agent Frustration Detection
Agent Frustration Detection relies on several advanced technologies that work together to identify agents' emotional states during voice calls. These technologies encompass natural language processing (NLP), sentiment analysis, and voice analytics. NLP allows the AI to understand the context of conversations, while sentiment analysis evaluates the emotions behind spoken words. Together, they can recognize signs of frustration based on the tone, pace, and choice of words used by the agents.
Moreover, voice analytics adds another layer by assessing vocal characteristics such as pitch and volume. This data helps in determining stress levels and overall emotional well-being. Additionally, machine learning models continuously improve through feedback, allowing for more accurate detection over time. By integrating these technologies, organizations can proactively address agent frustration, leading to improved workplace morale and enhanced customer service.
Implementing AI for Effective Detection
Implementing AI for effective detection involves utilizing advanced algorithms that can analyze vocal cues to identify signs of agent frustration. By focusing on audio patterns such as tone, pitch, and speech rate, AI systems can discern subtle emotional shifts during calls. This analysis is crucial since frustrated agents may express dissatisfaction through changes in their vocal expressions, impacting overall performance and customer interactions.
To successfully implement this technology, several steps should be followed. First, data collection is essential; gathering a representative sample of voice calls can enhance model accuracy. Second, model training involves teaching the AI to recognize frustration indicators based on this data. Third, real-time monitoring can provide immediate feedback, allowing supervisors to intervene when necessary. Lastly, continuous refinement of the AI system ensures it adapts to new patterns and maintains effectiveness over time, optimizing support for agents and improving customer experiences.
Conclusion: The Future of Agent Frustration Detection in Voice Calls
The potential for Agent Frustration Detection continues to evolve as advancements in AI technology enhance our analytical capabilities. Future systems may incorporate more refined algorithms that can interpret not only vocal tone but also contextual elements from the dialogue. This could lead to more accurate assessments of agent emotions, ultimately improving customer service experiences and employee well-being.
By anticipating frustration signals, organizations can proactively support agents, minimizing stressors during challenging calls. The integration of real-time feedback mechanisms will likely empower supervisors to intervene in crucial moments, leading to a healthier work environment. As AI becomes increasingly sophisticated, Agent Frustration Detection will play a pivotal role in reshaping call center dynamics.
💬 Questions about Can AI Detect Agent Frustration in Voice Calls??
Our team typically responds within minutes