Forecasting Intelligence Integration marks a significant step towards enhancing decision-making abilities within organizations. This integration combines data from forecasting and quality assurance (QA) into a cohesive framework, streamlining the analytical process. In a world where accurate insights drive business strategies, organizations often struggle with siloed information. By breaking down these barriers, Forecasting Intelligence Integration empowers teams to not only predict outcomes with greater accuracy but also ensure the quality of these forecasts.
Implementing a unified intelligence layer is crucial. It enables stakeholders to view comprehensive data effortlessly, fostering collaboration and improved communication. The result is a more insightful perspective that supports strategic planning and resource allocation. As businesses face increasing demands for agility and precision, embracing Forecasting Intelligence Integration can lead to more informed decisions, ultimately enhancing operational efficiency and driving success.
Analyze qualitative data. At Scale.
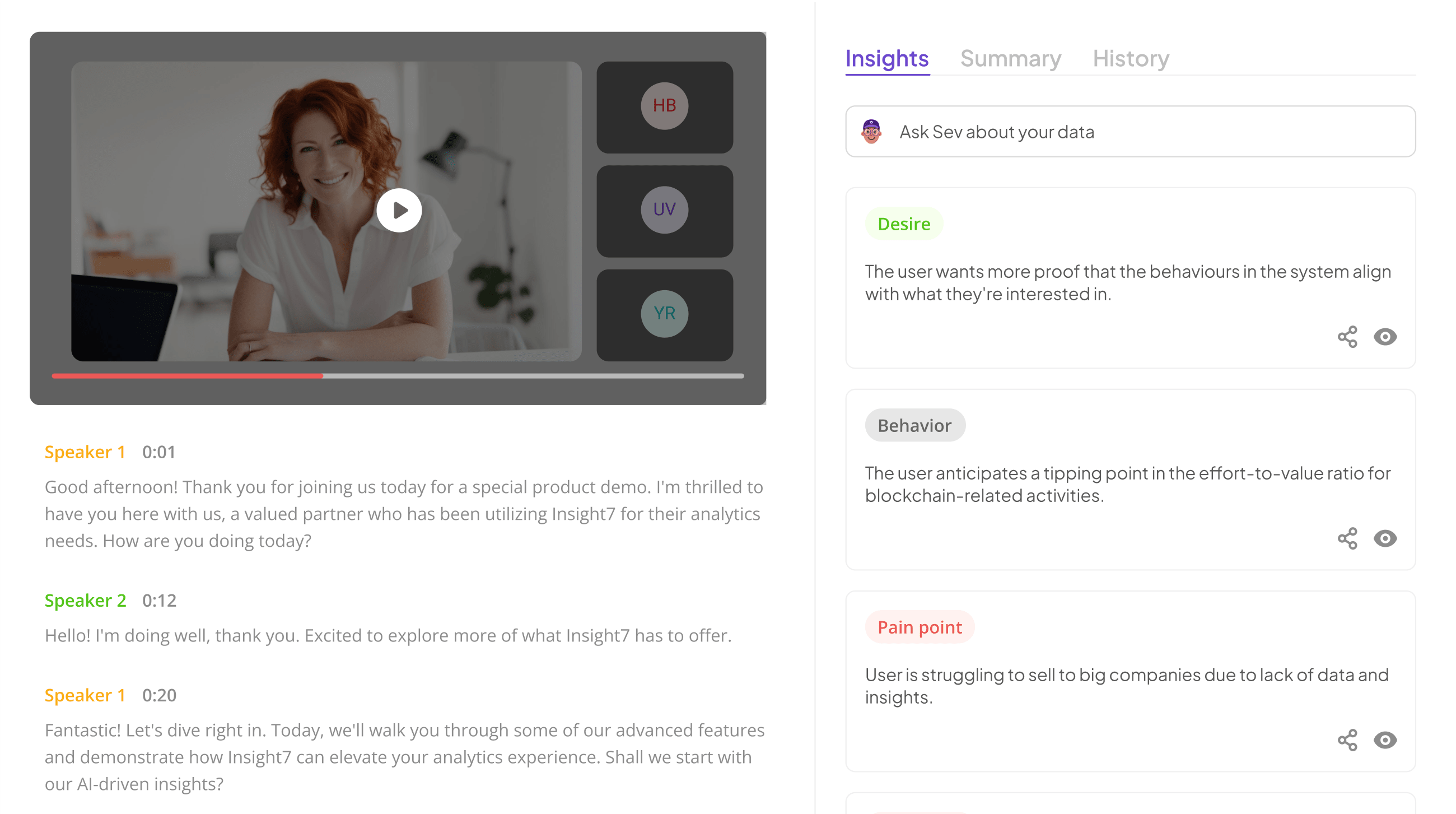
Understanding the Need for a Unified Intelligence Layer
The need for a unified intelligence layer stems from the challenges faced in separate forecasting and quality assurance processes. Traditional approaches often result in fragmented insights, causing delays in decision-making. When forecasting intelligence integration is disconnected from QA feedback, organizations risk missing critical customer signals and insights. This disconnect can lead to inefficiencies and slower responses to market changes, ultimately hindering competitiveness.
A unified intelligence layer ensures seamless data flow and enhanced collaboration between forecasting and QA teams. By consolidating insights from various sources, organizations can achieve a holistic view of customer behavior, leading to timely and informed business decisions. This integration also fosters better communication across departments, enabling teams to respond adaptively to emerging trends and customer needs. Ultimately, understanding the need for a unified intelligence layer is crucial for thriving in todayโs dynamic business environment.
Challenges in Separate Forecasting and QA Processes
Separate forecasting and quality assurance (QA) processes often lead to significant challenges in organizational efficiency and decision-making accuracy. One of the primary issues arises from the lack of communication between these two functions. When teams operate in silos, it hampers the overall insight generation process, affecting both forecasting precision and quality metrics. Employees may find themselves using different sets of data, leading to inconsistencies and misaligned objectives.
Additionally, time delays occur when necessary adjustments and insights are not shared promptly. These delays impact the ability to respond quickly to market changes, causing missed opportunities. By recognizing these gaps, organizations can initiate efforts toward Forecasting Intelligence Integration, creating a more cohesive and responsive approach. This process will foster better collaboration and enhance data accuracy, streamlining decision-making processes and ultimately driving better business outcomes.
Benefits of Forecasting Intelligence Integration
Integrating forecasting intelligence offers numerous advantages that directly impact decision-making processes. First, it enhances the accuracy of predictions by providing a comprehensive view of relevant data. This integration ensures that insights derived from forecasting are closely aligned with quality assurance metrics, which enhances overall reliability. By unifying these elements, businesses can anticipate market changes more effectively.
Moreover, the collaboration between forecasting and quality assurance fosters a proactive approach to problem-solving. Teams equipped with integrated insights can identify potential challenges early on and adjust strategies accordingly. Additionally, streamlined communication between departments minimizes information silos, allowing for more cohesive efforts. Ultimately, the benefits of forecasting intelligence integration lead to improved strategic alignment, ensuring that organizations remain agile and responsive to an ever-evolving market.
Building a Unified Intelligence Layer
A Unified Intelligence Layer facilitates seamless communication between forecasting and quality assurance processes. This integration allows organizations to harness critical data insights efficiently, leading to quicker decision-making and improved operational efficacy. The core of this approach lies in the incorporation of diverse data sources, which enriches the forecasting process and enhances quality checks simultaneously.
To build this effective layer, several essential steps must be taken. First, identify key data inputs that feed into both forecasting and QA. It is vital to ensure that these inputs are relevant and accessible. Next, design a robust intelligence architecture that fosters connectivity. Implementing this integration should follow a meticulous testing phase, which ensures the system is refined and well-optimized. Ultimately, a well-structured Unified Intelligence Layer not only streamlines operations but also boosts overall accuracy in both forecasting and quality assurance efforts.
Extract insights from interviews, calls, surveys and reviews for insights in minutes
Step 1: Identifying Key Data Inputs
Recognizing vital data inputs is fundamental to effective Forecasting Intelligence Integration. It starts with pinpointing what data sources will drive accurate insights. To build a robust intelligence layer, focus on gathering diverse inputs, such as customer feedback, sales trends, and market analysis. These inputs create a rich tapestry of information that enhances the quality of forecasting.
Next, consider structuring the data into different categories for clarity. For example, categorize inputs into demographic data, behavioral data, and sentiment analysis. This step allows you to easily identify patterns and correlations, which are crucial for formulating reliable forecasts. By meticulously curating these inputs, organizations can ensure that their forecasting processes are not only accurate but also agile, adapting to changes efficiently. This careful selection and categorization of data serve as the foundation for a unified intelligence strategy, ultimately leading to informed decision-making and operational excellence.
Step 2: Designing the Intelligence Architecture
In this stage of designing the intelligence architecture, it is crucial to outline a clear framework that harmonizes forecasting with quality assurance. A unified intelligence layer ensures seamless data flow, facilitating more accurate analysis and insights. By integrating these processes, we can address discrepancies that often arise when forecasting tools and QA practices operate in silos.
To achieve an effective Forecasting Intelligence Integration, consider the following key components:
Data Sources: Identify and connect relevant data sources to ensure comprehensive information accessibility. This allows for richer insights and reduces the risk of missing critical metrics.
Evaluation Framework: Establish guidelines for evaluating data against defined standards. Implementing this will ensure consistency and reliability in both forecasting and QA efforts.
User Interface: Design a user-friendly interface that can accommodate various user needs, whether for generating reports automatically or enabling self-service options. This feature can enhance user engagement and promote data-driven decision-making.
By focusing on these elements, you create an architecture that fosters collaboration between forecasting and QA, ultimately leading to more informed, effective business strategies.
Step 3: Implementing the Integration
Implementing the integration of forecasting intelligence requires a systematic approach to ensure seamless data flow and actionable insights. This step not only combines data sources but also builds a foundation for comprehensive analysis. Begin by aligning the goals of both forecasting and quality assurance functions. This ensures all stakeholders understand the desired outcomes and can contribute effectively.
Next, focus on integrating the necessary tools and platforms that facilitate data exchange. Establish connections between systems that hold critical forecasting data and those that support quality assessment. Regular feedback loops should be integrated, allowing teams to refine workflows based on real-time insights. By prioritizing collaboration and transparency throughout this process, the effectiveness of the forecasting intelligence integration will significantly enhance, ultimately leading to better decision-making and improved outcomes across the organization.
Step 4: Testing and Refining the System
In the journey of Forecasting Intelligence Integration, Step 4: Testing and Refining the System plays a pivotal role. This phase involves assessing the initial implementation to ensure it operates as intended. Start by conducting rigorous tests on your system, identifying any discrepancies or inefficiencies that could impact the quality of insights. Through this testing process, validate that each component is functioning harmoniously and that data flows seamlessly throughout the framework.
Once you identify areas for improvement, refining the system becomes essential. Gather feedback from end users and stakeholders, and analyze their experiences with the integrated solution. This step is not only about fixing issues but also about enhancing user engagement and ensuring that the system meets its intended goals. By iterating on the design and addressing any shortcomings, you ultimately elevate the effectiveness of your forecasting efforts and create a robust intelligence layer. Embrace this testing phase as an ongoing process that will significantly contribute to the success of your unified forecasting and QA initiatives.
Tools for Effective Forecasting Intelligence Integration
The integration of tools for effective forecasting intelligence is crucial in creating a robust unified layer. By employing the right technology, organizations can streamline data collection and analysis. This not only enhances the accuracy of forecasts but also improves the efficiency of quality assurance processes. A seamless integration allows various stakeholders to access critical insights without needing extensive training, democratizing the power of data.
To achieve effective forecasting intelligence integration, several tools can be employed. For instance, analytics platforms enable real-time data processing, allowing teams to visualize trends dynamically. Collaboration tools facilitate communication across departments, ensuring everyone is aligned. Additionally, user-friendly dashboards offer stakeholders quick access to essential metrics, promoting informed decision-making. Together, these tools form a cohesive ecosystem that empowers organizations to harness intelligence and drive strategic outcomes effectively.
insight7
A robust unified intelligence layer is essential for optimizing forecasting processes. Insight7 focuses on how this layer can enhance data collaboration between forecasting and quality assurance teams. When teams operate in silos, critical insights often get lost, leading to ineffective strategies and delayed decisions. By integrating forecasting intelligence, organizations can ensure timely access to valuable insights that drive effective business actions.
The integration process begins by identifying key data inputs, creating an efficient architecture, and implementing the system. Continuous testing and refining are paramount for maintaining accuracy and relevance in data interpretation. Through effective forecasting intelligence integration, organizations can recognize trends, anticipate customer needs, and respond proactively. This approach not only improves operational efficiency but also provides a significant competitive edge in the market.
Other Integration Tools
Integrating diverse tools is essential for enhancing the effectiveness of forecasting intelligence integration. Various integration tools assist in bridging the gap between forecasting systems and quality assurance processes. These tools enable seamless data flow, ensuring that insights derived from forecasting are accessible across teams for informed decision-making.
Firstly, consider integration tools that streamline data aggregation from multiple sources. These tools consolidate information, making it easier to analyze trends and predict future outcomes. Secondly, automation tools enhance the efficiency of data processing, allowing teams to focus on strategic actions rather than mundane tasks. Thirdly, visualization tools present data in a digestible format, facilitating better communication and quicker comprehension of insights. Lastly, collaboration platforms foster teamwork, enabling stakeholders to share findings and collaborate in real-time. By harnessing these integration tools, organizations can significantly improve their forecasting accuracy and the quality of their decisions.
- Tool A
Tool A serves as a pivotal component in the journey toward achieving effective Forecasting Intelligence Integration. This tool not only facilitates the seamless merging of forecasting and quality assurance processes but also enhances data visibility and accessibility. By leveraging its capabilities, users can extract actionable insights from diverse data sources, enabling informed decision-making across projects.
The implementation of Tool A allows teams to unify their analytical efforts, moving away from siloed data management. It empowers users to conduct in-depth analyses, identifying trends and patterns that can inform future strategies. For instance, visualizing customer feedback can reveal both positive and negative sentiments, which are crucial for product development and refinement. The tool's advanced querying features further enable users to compare datasets and derive relevant insights, ensuring that all aspects of forecasting and QA are aligned and optimized for better business outcomes.
- Tool B
Tool B
In the realm of building a unified intelligence layer, Tool B emerges as a vital component. This integration assists organizations in combining data from various forecasting and quality assurance processes seamlessly. The intuitive design of Tool B facilitates real-time data synchronization, ensuring that users can access accurate insights promptly.
Moreover, Tool B supports advanced analytics features, allowing teams to predict trends accurately and improve decision-making processes. By employing this tool, organizations can address inconsistencies often found when managing separate datasets. It fosters collaboration among teams, enabling them to align their objectives and strategies effectively. Integrating Tool B into the existing systems will significantly enhance forecasting intelligence integration, driving better business outcomes.
Consider Tool B as an indispensable part of creating an effective intelligence framework, centralizing information for improved forecasting and quality assurance synergy.
- Tool C
In the context of Building a Unified Intelligence Layer Across Forecasting + QA, Tool C plays a pivotal role in enhancing productivity and accuracy. This tool is specially designed to facilitate seamless Forecasting Intelligence Integration, ensuring stakeholders have access to real-time insights. By focusing on data coherence, Tool C bridges gaps between various systems, making it easier for teams to collaborate and make informed decisions.
Tool C offers several key features:
- Customizable Dashboards: These allow users to visualize forecasting metrics tailored to their needs, enhancing clarity and accessibility.
- Real-time Data Processing: This ensures that information is current, enabling timely interventions and adjustments in forecasting models.
- User-friendly Interface: A simplified design promotes ease of use, making it accessible for all team members, regardless of technical expertise.
Implementing Tool C not only streamlines operations but also empowers teams to harness the power of integrated data for improved forecasting accuracy. This positions organizations to respond more effectively to dynamic market needs and client expectations.
- Tool D
Tool D
In the realm of Forecasting Intelligence Integration, Tool D stands out as a key resource for enhancing operational efficiency. Designed to bridge gaps in data collection and analysis, it fosters collaboration between forecasting and QA teams. By integrating various data sources, Tool D enables a comprehensive view of performance metrics, assisting decision-makers in refining strategies effectively.
The functionalities of Tool D include advanced analytics capabilities, seamless data visualization, and real-time reporting. These features empower users to track critical KPIs and prompt timely interventions when discrepancies arise. Furthermore, it offers customizable options that cater specifically to the distinct needs of each project or client, ensuring flexibility and adaptability in usage. Implementing Tool D could significantly streamline workflows, promote accuracy in forecasting, and ultimately lead to smarter business outcomes. Embracing such tools is essential for organizations aiming to cultivate a unified intelligence layer across their operations.
Conclusion: The Future of Forecasting Intelligence Integration
The future of forecasting intelligence integration holds great promise for organizations aiming to enhance their decision-making processes. By creating a unified intelligence layer, businesses can seamlessly blend forecasting with quality assurance, leading to more accurate predictions and refined strategies. This integration allows teams to harness diverse data sources, transforming them into actionable insights that drive efficiency.
As technology evolves, the capabilities of forecasting intelligence will advance, enabling organizations to leverage real-time data and adaptive algorithms. Embracing these innovations will empower businesses to be more responsive, fostering a culture of continuous improvement and agile decision-making within the marketplace. The journey towards a unified intelligence layer is essential for any organization that seeks to thrive in today's dynamic business environments.