Best Tools for Predictive Behavioral Insights in Financial Services
-
Bella Williams
- 10 min read
Predictive Finance Analytics has emerged as a transformative force within financial services, enabling firms to anticipate customer behavior and market trends effectively. As competition intensifies, organizations are increasingly turning to data-driven strategies to enhance decision-making processes. By leveraging advanced algorithms and historical data, financial institutions can derive actionable insights that foster better client engagement and risk management without compromising on compliance.
In today's rapidly changing financial landscape, understanding and utilizing predictive finance analytics is crucial for success. This analytical approach not only refines operational efficiency but also personalizes customer experiences. As we delve into the best tools available for obtaining predictive behavioral insights, financial professionals will discover a wealth of opportunities to harness data for informed decision-making and sustained growth.
Analyze & Evaluate Calls. At Scale.
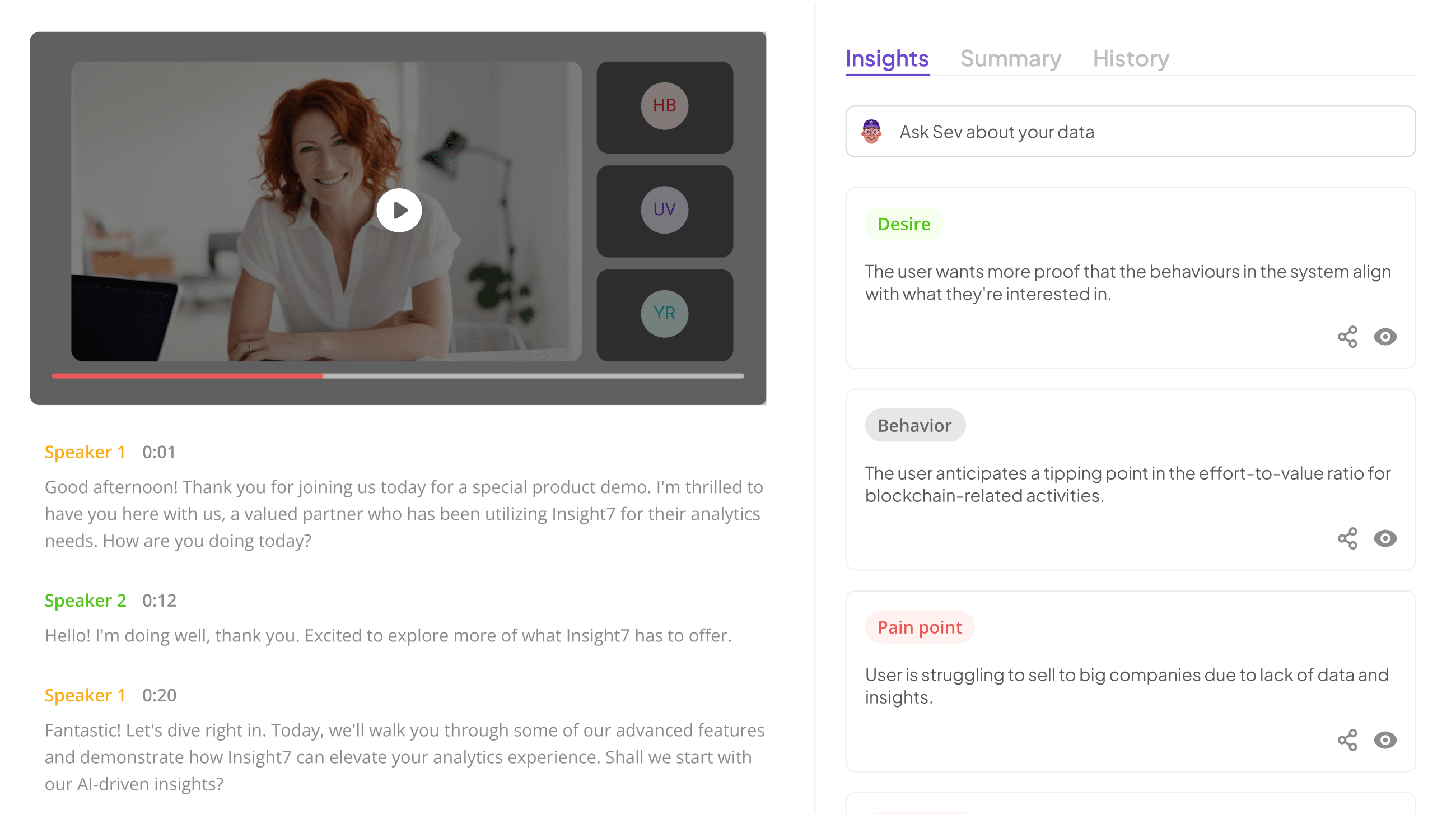
Understanding Predictive Finance Analytics
Predictive Finance Analytics involves utilizing advanced analytical techniques to forecast potential financial outcomes based on historical data and behavioral insights. This approach enables financial institutions to anticipate customer needs and make strategic decisions, ultimately leading to enhanced customer satisfaction and operational efficiency. By analyzing vast amounts of data, organizations can identify trends and patterns that inform future financial behaviors.
In innovation-driven financial services, understanding the mechanics behind predictive models is vital. These models leverage statistical methods and machine learning algorithms to create predictions that guide financial planning and risk management. The insights gained not only assist in personalizing services but also improve the accuracy of financial forecasts, reducing uncertainty in decision-making. As financial markets continue to evolve, the importance of adopting effective predictive finance analytics tools becomes evident, driving companies towards data-informed strategies.
The Role of Behavioral Insights
Behavioral insights play a critical role in shaping the effectiveness of predictive finance analytics. By understanding customer behaviors and preferences, financial services can tailor their approaches to consumer needs. This knowledge helps organizations anticipate trends and make informed decisions that align with customer expectations. Behavioral insights allow companies to move ahead of their competition by offering personalized services that resonate with their target audiences.
Several key aspects highlight the importance of integrating behavioral insights into financial analytics. First, identifying customer segments based on behavior aids in crafting targeted marketing strategies. Second, understanding pain points leads to enhanced customer service experiences. Third, analyzing decision-making patterns enables businesses to improve product offerings. Lastly, timely insights from customer interactions foster an environment of continuous improvement. Embracing these behavioral insights ultimately drives better business outcomes and builds lasting customer relationships.
How Predictive Models Work
Predictive models operate on the premise of utilizing historical data to forecast future outcomes. In financial services, these models analyze customer behavior patterns, market trends, and economic indicators to generate actionable insights. The process begins with data collection, where relevant information is gathered from various sources, including transactions, customer interactions, and external datasets.
Once the data is collected, it undergoes preprocessing to clean and format it for analysis. After that, algorithms are applied to identify patterns and relationships within the data. These algorithms can vary, but they typically include regression analysis, decision trees, and machine learning techniques. By applying predictive finance analytics, financial institutions can make informed decisions, segment their customer base more effectively, and tailor services to meet specific client needs. Ultimately, predictive models serve as essential tools for enhancing decision-making and driving business success.
Extract insights from interviews, calls, surveys and reviews for insights in minutes
Exploring the Top Tools for Predictive Behavioral Insights
In exploring the top tools for predictive behavioral insights, it’s essential to consider how these instruments can transform financial services. Various tools are designed to analyze vast amounts of data, offering actionable insights that refine decision-making processes. By leveraging predictive finance analytics, businesses can anticipate customer behaviors and market trends, ultimately enhancing their services.
Among the standout tools are insights visualization platforms that facilitate easy data analysis. These platforms often feature user-friendly interfaces, allowing even non-experts to generate detailed reports or identify key pain points in the customer experience. Advanced machine learning tools can model complex financial behaviors, providing organizations with the ability to predict future trends based on historical data. Understanding these toolsets and their functionalities can greatly improve strategic planning and customer engagement efforts within the financial industry.
insight7
Understanding how tools for predictive behavioral insights can transform financial services is crucial. By integrating predictive finance analytics, organizations can better analyze customer behavior patterns and anticipate future needs. As customer signals increase in volume and complexity, traditional analysis methods often falter. Therefore, adopting advanced tools is essential for timely decision-making in this competitive landscape.
To successfully implement predictive finance analytics, consider the following key aspects:
- Real-Time Data Analysis: Optimize your ability to interpret and act on customer data promptly. This is crucial for staying ahead.
- User-Friendly Interfaces: Choose tools that offer intuitive navigation, enabling teams to engage with insights directly and effectively.
- Collaboration Features: Ensure that your selected tools facilitate easy sharing and discussion of insights among team members, enhancing teamwork.
By focusing on these elements, financial institutions can better leverage predictive finance analytics for improved strategy development and customer engagement.
Tool 2: IBM Watson Analytics
IBM Watson Analytics is a powerful tool for exploring predictive finance analytics. It empowers financial services to uncover meaningful insights from complex datasets. With its intuitive interface, users can visualize data and identify patterns without needing extensive technical knowledge. This ability is essential for making informed decisions based on customer behaviors and trends.
One key feature of this tool is its natural language processing capability, allowing users to ask questions in plain language. Additionally, the analytics engine can perform in-depth queries on datasets, enabling users to analyze customer feedback deeply. By examining both positive and negative sentiments, financial firms can better tailor their products and services to meet customer needs. In essence, IBM Watson Analytics transforms raw data into actionable insights, enhancing strategic decision-making within financial services. This tool ultimately supports organizations in leveraging predictive analytics effectively.
Tool 3: Microsoft Azure Machine Learning
Microsoft Azure Machine Learning is a robust platform designed for building, training, and deploying machine learning models in the financial sector. This tool simplifies the process of predictive finance analytics by offering an array of features tailored to financial services. By using Azure, institutions can harness data from various sources, gaining insights into customer behavior and market trends.
One key aspect of Azure is its ability to integrate seamlessly with existing data ecosystems. This means financial organizations can import data easily and leverage built-in algorithms to analyze behavioral patterns. Furthermore, Azure allows for collaboration among data scientists and business analysts, enhancing the overall quality of insights produced. The platform also supports real-time telemetry, enabling institutions to adapt quickly to changing market conditions.
Overall, implementing Microsoft Azure Machine Learning can significantly boost predictive finance analytics capabilities, leading to better decision-making and improved customer experiences.
Tool 4: RapidMiner
RapidMiner stands out as a powerful tool for businesses aiming to harness predictive finance analytics effectively. This platform offers a user-friendly interface that facilitates data preparation, machine learning model development, and visual analytics. Users can seamlessly manipulate large datasets, allowing them to identify trends and patterns that are crucial for making informed financial decisions.
💬 Questions about Best Tools for Predictive Behavioral Insights in Financial Services?
Our team typically responds within minutes
One of the significant advantages of RapidMiner is its support for a wide range of predictive modeling techniques. This flexibility enables organizations to tailor their analytics according to specific needs and objectives. Furthermore, it integrates with various data sources, ensuring that users can easily access diverse datasets for comprehensive analysis. RapidMiner's robust community and extensive resources also provide valuable support, enhancing the user experience and resulting in deeper insights into behavioral patterns in finance. By utilizing this tool, financial services can better navigate the evolving landscape and unlock actionable insights from their data.
Tool 5: DataRobot
DataRobot is an intuitive platform that simplifies the process of predictive finance analytics. Designed for users of all skill levels, it enables teams to access valuable insights without the need for extensive training. It democratizes access to predictive analytics, making it possible for non-experts to generate data-driven reports efficiently.
Within the platform, users can easily manage and analyze multiple datasets, allowing for a comprehensive view of customer interactions and experiences. The system automatically extracts key insights, such as pain points and customer desires, by analyzing conversations and other data sources. This feature facilitates better decision-making by providing actionable intelligence that informs strategies and enhances customer experiences. By enabling a seamless analysis of vast data quantities, DataRobot stands out as a vital tool for financial services seeking to leverage predictive behavioral insights.
Steps to Implementing Predictive Finance Analytics
Implementing Predictive Finance Analytics involves a structured approach to effectively harnessing data for better financial decisions. The first step is to define clear objectives, as this will guide your analysis and ensure alignment with business goals. Next, selecting appropriate tools tailored to your needs is crucial. Tools should offer robust analytics capabilities and the flexibility to adapt to your specific requirements.
Once the tools are in place, data collection and preparation become the focus. Ensure that the data is clean, relevant, and formatted correctly, as this plays a vital role in the accuracy of your predictive models. The fourth step is model development, where quantitative techniques are used to create models that forecast future financial trends or behaviors. Finally, deployment and monitoring are essential to validate the models against real-world performances and adjust them as necessary for continuous improvement. By following these steps, you can effectively implement Predictive Finance Analytics in your financial services organization.
Step 1: Define Your Objectives
Defining your objectives is the crucial first step toward successful predictive finance analytics. Before diving into data and tools, take the time to clarify what you aim to achieve. Establish clear targets that align with your business goals, whether that involves enhancing customer relationships or improving risk assessment. A well-defined objective provides direction and builds a solid foundation for deeper analyses.
Start by identifying key performance indicators (KPIs) that matter most to your financial services. These could include customer retention rates or portfolio performance metrics. Once you've pinpointed these KPIs, it’s essential to understand the specific questions you want predictive models to answer. This clarity not only optimizes data collection but also enhances your ability to make informed decisions based on behavioral insights. Consider how each objective ties back to overall business strategy and customer needs, ensuring that predictive finance analytics efforts are strategic and impactful.
Step 2: Select Appropriate Tools
Selecting the appropriate tools for implementing predictive finance analytics is a crucial step in deriving valuable insights. This decision hinges on your specific objectives, target audience, and desired outcomes. Each tool comes with unique capabilities that cater to varying needs, such as data analysis, customer segmentation, or forecasting trends. Therefore, it’s essential to assess these tools based on their features, ease of use, and scalability.
There are several critical factors to consider when choosing the right tools. First, evaluate the alignment of the tool’s features with your organizational goals. Then, consider user-friendliness, which can impact the speed of adoption within your team. Finally, assess the scalability of the tool, ensuring it can grow and adapt as your predictive analytics needs evolve. By making informed choices at this stage, you position your organization to extract actionable insights that drive decision-making and enhance customer experiences.
Step 3: Data Collection and Preparation
Data Collection and Preparation is crucial for leveraging predictive finance analytics effectively. This step involves gathering and organizing data from various sources, ensuring it is reliable and relevant. Start by identifying the types of data needed, which can include customer demographics, transaction histories, and behavioral patterns. This foundational data will serve as the basis for building predictive models that offer valuable insights.
Once the data is collected, the next phase is to clean and preprocess it. This involves handling missing values, standardizing formats, and eliminating duplicates. Having clean data is essential because errors can lead to misleading predictions. Prepare the dataset for analysis by transforming it into a structured format suitable for modeling. Thorough data collection and preparation ultimately empower organizations to make informed financial decisions, maximize efficiency, and enhance customer experiences.
Step 4: Model Development
Developing a robust model is a crucial step in harnessing Predictive Finance Analytics effectively. This phase involves identifying and validating the most relevant data points that influence behavioral patterns in financial services. By carefully examining historical data and identifying key variables, organizations can begin to construct analytical models that provide insights into future behaviors.
There are several key considerations to keep in mind during model development:
- Data Quality: Ensure that the data used is accurate and relevant.
- Algorithm Selection: Choose appropriate algorithms that can best interpret the data.
- Model Testing: Rigorous testing and validation are necessary to confirm the model's effectiveness.
- Iteration: Be prepared to adjust the model based on feedback and new data.
By focusing on these elements, organizations can refine their predictive models, ultimately enabling better decision-making in financial services. This focused approach facilitates the understanding of consumer behaviors and enhances overall business strategies.
Step 5: Deployment and Monitoring
Once the predictive models are developed, the next crucial phase is deployment and monitoring. This step involves integrating the predictive finance analytics tools into existing financial systems to ensure seamless functionality. It is essential to establish automated reporting processes so stakeholders receive insights regularly; this might typically be done on a monthly basis.
Monitoring the system is equally important. This includes assessing the accuracy of predictions and making necessary adjustments based on feedback and performance metrics. Organizations should be equipped to identify when the model's relevance may wane over time. By actively monitoring these predictive analytics, firms can ensure they derive actionable insights and maintain a competitive advantage in the financial services sector. Thus, deployment and monitoring are fundamental to harnessing the full potential of predictive finance analytics effectively.
Conclusion: Harnessing the Power of Predictive Finance Analytics in Financial Services
In conclusion, the integration of predictive finance analytics in financial services presents a transformative opportunity for institutions to understand customer behaviors and trends. Organizations can harness these analytics to enhance decision-making processes, ensuring they remain competitive in a rapidly evolving market.
By implementing the right tools, financial services can unlock actionable insights that drive better customer engagement. These advancements not only improve operational efficiency but also foster trust and loyalty among clients, ultimately leading to sustainable growth in this dynamic sector. Embracing the power of predictive finance analytics is essential for future success.
💬 Questions about Best Tools for Predictive Behavioral Insights in Financial Services?
Our team typically responds within minutes