Effective sentiment assessment optimization plays a vital role in extracting actionable insights from survey feedback. Understanding feelings—ranging from enthusiasm to frustration—enables organizations to address customer needs accurately. To unlock the potential of this data, one must expertly analyze the emotional tone embedded in responses. This process involves several strategies that enhance the clarity and relevance of customer sentiments, ensuring that voices do not get lost amid noise.
By focusing on optimizing sentiment assessment, companies can significantly improve their responsiveness and overall customer satisfaction. Techniques such as identifying emotional indicators and utilizing advanced analytics tools contribute to more meaningful insights. Ultimately, an optimized sentiment assessment not only aids in understanding user experience but also fosters more informed decision-making.
Analyze qualitative data. At Scale.
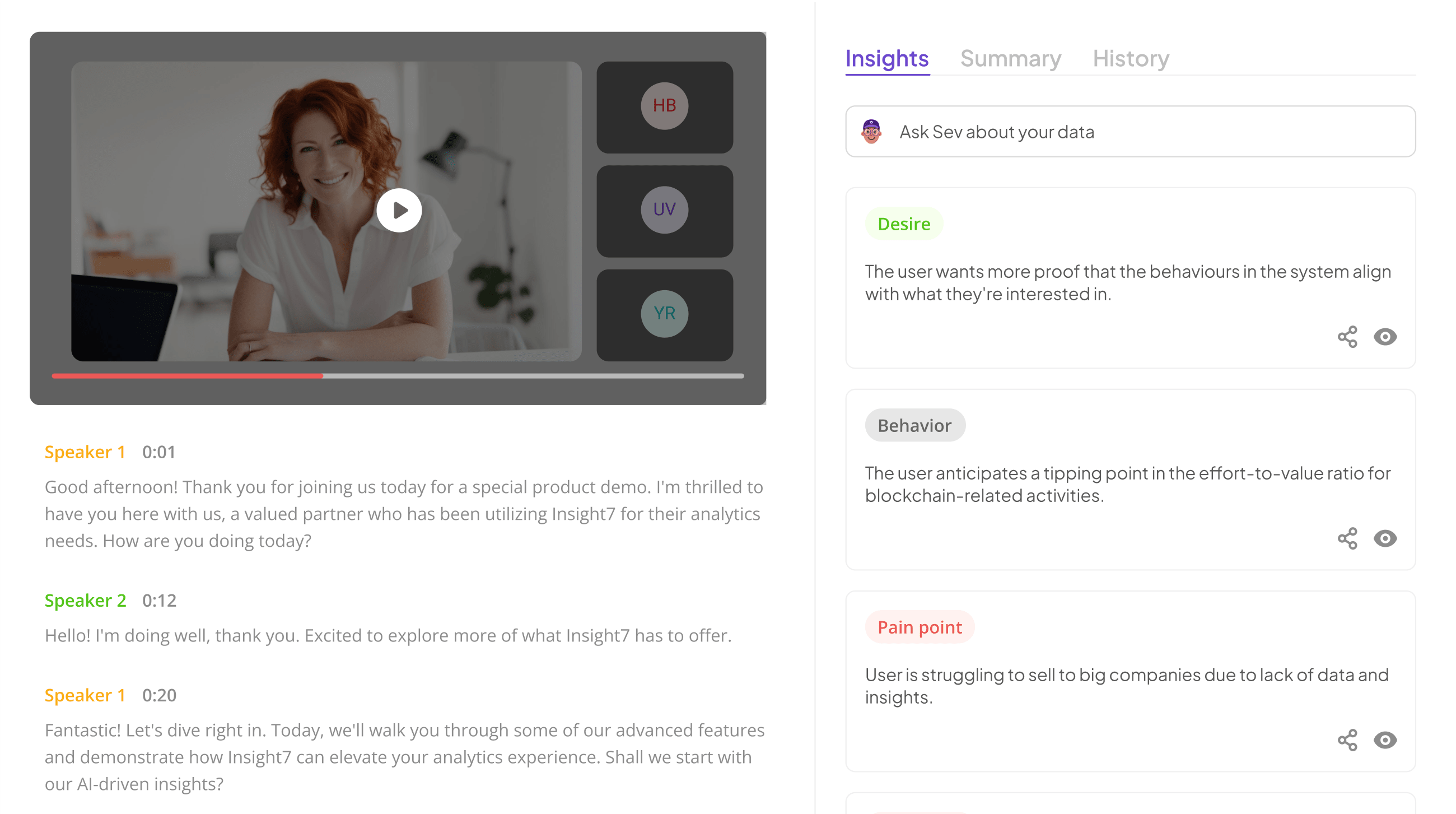
Understanding Emotional Tone in Surveys
Understanding emotional tone in surveys is pivotal for accurately interpreting feedback. Emotional tone encompasses the sentiments expressed by respondents, reflecting their feelings toward products, services, or experiences. By analyzing emotional tone, organizations can uncover deeper insights into customer satisfaction, loyalty, and overall sentiment. This analysis is instrumental in tailoring responses, enhancing customer experiences, and driving brand loyalty.
To successfully navigate the complexities of emotional tone analysis, it is beneficial to focus on a few key strategies. First, ensure a robust method for collecting survey data, which lays the groundwork for a reliable analysis. Second, identify essential emotional indicators such as happiness, anger, or frustration that resonate with survey responses. Lastly, applying machine learning models can enhance the accuracy of sentiment assessment optimization. These strategies create a structured approach, enabling organizations to evaluate emotional tone more effectively, resulting in actionable insights that can drive improvements.
The Role of Sentiment Assessment Optimization in Emotional Tone Analysis
In emotional tone analysis, Sentiment Assessment Optimization plays a crucial role in accurately interpreting survey feedback. Understanding the nuances of language is essential for discerning the emotional states of respondents. By refining how sentiment is assessed, organizations can gain deeper insights into customer feelings and perceptions. This optimization involves employing systematic methods to analyze responses, focusing on how word choice and tone convey underlying emotions.
To effectively apply Sentiment Assessment Optimization, it is helpful to consider several key elements. First, it involves data collection and preparation, ensuring that the feedback is comprehensive and organized. Next, identifying key emotional indicators is vital, enabling analysts to pinpoint what drives sentiment. Finally, applying advanced analytical techniques, such as machine learning models, enhances the accuracy of tone detection. By embracing these practices, organizations can create more meaningful and actionable insights from their survey data.
Common Challenges in Emotional Tone Analysis
In the realm of emotional tone analysis, organizations frequently encounter complex challenges that hinder effective sentiment assessment optimization. One primary issue is the subjectivity involved in interpreting emotional expressions. Respondents may convey emotions that differ significantly from the perceived sentiment of their words, leading to misinterpretations. This ambiguity poses difficulties in accurately categorizing feedback, which can ultimately affect decision-making.
Additionally, data variability can complicate emotional tone analysis. Surveys might yield responses that fluctuate based on factors such as mood, context, or even phrasing. Moreover, many existing frameworks face limitations in effectively capturing nuanced emotional expressions. These challenges necessitate robust strategies to enhance sentiment assessment optimization, enabling organizations to derive insights that accurately reflect customer emotions. Addressing these issues is critical to creating meaningful customer experiences and driving impactful outcomes.
Sentiment Assessment Optimization Techniques for Accurate Analysis
To achieve effective sentiment assessment optimization, employing structured techniques is essential for accurate analysis. One crucial technique is to ensure proper data collection and preparation. Gather feedback from surveys comprehensively, including both quantitative ratings and qualitative comments. This dual approach allows for a richer understanding of emotional tones present.
Another important step in sentiment assessment optimization is identifying key emotional indicators within the data. Look for specific phrases or words that signal positive or negative sentiments. Techniques such as natural language processing (NLP) can help in this regard, as they enable the system to discern emotional subtleties.
Moreover, utilizing machine learning models can significantly enhance the precision of sentiment analysis. These algorithms can be trained to recognize patterns in emotional expressions based on historical data, vastly improving the analysis. By integrating these optimization techniques, businesses can ensure they gain valuable insights from survey feedback, enhancing decision-making and customer satisfaction.
Extract insights from interviews, calls, surveys and reviews for insights in minutes
Step-by-Step Guide to Sentiment Assessment Optimization
To embark on the journey of Sentiment Assessment Optimization, begin by clearly defining your objectives. Understanding the specific emotional tones you wish to assess is crucial to guiding your analysis process. This foundation will help you evaluate the feedback effectively and ensure that the assessment aligns with your goals.
Next, adopt a methodical approach in handling the data. Start with thorough data collection and preparation that includes cleaning and structuring your survey responses. Identifying key emotional indicators, such as positive, negative, or neutral tones, is vital. This will streamline the analysis process. Lastly, apply machine learning models that can enhance the accuracy of your sentiment assessment, adapting them as necessary based on the nuances observed in the feedback.
By systematically following these steps, you are well-equipped to optimize your sentiment analysis and gain valuable insights from survey feedback.
Step 1: Data Collection and Preparation
Effective data collection and preparation is the cornerstone of any successful emotional tone analysis in survey feedback. Initially, it is crucial to gather diverse and comprehensive data that accurately reflects customer sentiments. This includes verbal feedback, numeric ratings, and additional qualitative insights that provide context. The richer the dataset, the more precise your Sentiment Assessment Optimization will be.
Once the data is collected, it needs careful preparation. This involves cleaning the data to eliminate errors and inconsistencies, ensuring that no biases skew the analysis. Structured formatting of responses is also essential, as it allows for easier processing and analysis. Additionally, categorizing data based on themes or keywords can significantly enhance the insights derived from the feedback. Preparing data systematically will ultimately facilitate a smoother transition to the next stages of analysis, yielding more reliable emotional tone outcomes.
Step 2: Identifying Key Emotional Indicators
Recognizing key emotional indicators is crucial for effective sentiment assessment optimization in survey feedback. These indicators help pinpoint the feelings and moods present in respondents' answers, leading to better insights. Begin by analyzing specific words or phrases that evoke strong emotions, such as joy, frustration, or confusion. It is essential to categorize these expressions systematically to ensure consistent interpretation across your data.
Next, evaluate the context surrounding these indicators. How do particular emotional cues relate to overall feedback trends? Understanding the correlation between emotions and feedback can help you paint a more accurate picture of customer sentiment. By refining this process, you can strategically enhance the quality of your emotional tone analysis. Ultimately, this structured approach leads to deeper insights and more meaningful actions based on the feedback collected. Properly identifying emotional indicators sets the foundation for effective sentiment assessment optimization.
Step 3: Applying Machine Learning Models
Applying machine learning models marks a pivotal step in refining sentiment assessment optimization for emotional tone analysis. When entering this stage, organizations should focus on selecting the most effective algorithms tailored for the specific emotional indicators identified previously. This may involve experimenting with various models, such as Support Vector Machines or neural networks, to determine which yields the most accurate sentiment interpretations.
To further enhance the emotional tone analysis process, organizations must ensure proper training of their models with high-quality, labeled datasets. Regularly evaluating model performance is also crucial. This ensures consistent and reliable results over time. Enhancements and adjustments will be necessary as more feedback is collected, and emotional tones evolve. Ultimately, the application of machine learning not only optimizes sentiment analysis but also deepens understanding of customer sentiments, leading to more informed decision-making.
Enhancing Sentiment Assessment Optimization with Top Tools
To enhance Sentiment Assessment Optimization, utilizing top-notch tools is essential for effective emotional tone analysis in survey feedback. The right tools streamline data collection, allowing organizations to capture customer sentiments accurately and efficiently. They facilitate the identification of emotional patterns, enabling teams to gauge feedback in real-time. With such capabilities, businesses can make informed decisions that resonate with their audience.
Key tools in the realm of sentiment analysis include Insight7, MonkeyLearn, Lexalytics, and IBM Watson Natural Language Understanding. Insight7 offers an intuitive platform for analyzing large volumes of text data, making emotional tone recognition straightforward. MonkeyLearn enables teams to customize their sentiment analysis models, ensuring a tailored approach to feedback interpretation. Similarly, Lexalytics provides advanced language processing capabilities that help decode customer emotions effectively. Lastly, IBM Watson Natural Language Understanding leverages AI to deliver in-depth insights from survey responses. By integrating these tools, organizations can significantly enhance their sentiment assessment strategies and ultimately improve customer satisfaction.
Insight7
Understanding the nuances of emotional tone is essential for effective survey feedback analysis. Insight7 explores how to enhance sentiment assessment optimization by focusing on key indicators that reveal genuine customer feelings. Effective emotional tone analysis goes beyond surface-level interpretations, delving deeper into the sentiments that drive customer satisfaction or dissatisfaction.
To optimize sentiment assessment, consider the following strategies: first, prioritize data collection and preparation to ensure high-quality input. Second, identify emotional indicators that provide valuable insights into customer experiences. Third, leverage advanced machine learning models to automate the analysis and improve accuracy. These steps work together to streamline the analysis process, making it more efficient and insightful. By focusing on these practices, organizations can better understand customer sentiments, thus transforming insights into actionable strategies for improvement and engagement.
MonkeyLearn
In the quest for effective emotional tone analysis, utilizing the right tools can greatly enhance your results. These tools facilitate the collection and interpretation of feedback data, allowing businesses to better understand customer sentiments. One tool in particular stands out for its user-friendly interface and robust analytical capabilities. Designed to democratize insights, it enables anyone within an organization to access and derive value from complex data sets without prior technical training.
The platform excels in transforming raw survey data into actionable insights. Users can easily upload files and conduct detailed analyses to identify key emotional indicators, pain points, and overall sentiment. This process not only streamlines the extraction of meaningful information but also promotes a culture of data-driven decision-making. By integrating such tools into your survey feedback process, you can optimize sentiment assessment and unlock valuable customer insights in a more efficient manner.
Lexalytics
The journey toward effective emotional tone analysis often leads to exploring innovative tools and platforms. Among these, one such platform stands out for its remarkable capabilities in sentiment assessment optimization. Designed with user-friendliness in mind, this tool allows anyone within an organization to access and utilize its features without requiring specialized training or expertise. This democratization of insights enables seamless data transformation, making it easier for teams to generate reports and analyze customer feedback.
Users can upload their audio files, which the platform processes to extract meaningful insights drawn from conversations. From identifying pain points to revealing customer desires, this system excels at providing valuable feedback. Additionally, it organizes data into insightful cards, summarizing key themes and feedback trends. This level of analysis not only enhances understanding but also empowers users to take informed, actionable steps based on the emotional tone evident in survey feedback.
IBM Watson Natural Language Understanding
Employing advanced natural language understanding techniques can significantly enhance the process of sentiment assessment optimization. By utilizing sophisticated algorithms, organizations can effectively analyze survey feedback, identifying the emotional tone expressed by respondents. This understanding is crucial in making informed decisions that resonate with customer experiences and perceptions.
One major benefit of natural language understanding systems is their ability to discern nuances in sentiment, capturing subtle emotions that might otherwise be overlooked. Additionally, incorporating context into the analysis helps surface underlying themes and trends, refining sentiment assessment optimization. For effective usage, it's recommended to follow a structured approach: gather diverse feedback, apply consistent emotional tone indicators, and leverage machine learning models that continuously learn from new data. By embracing these best practices, organizations can drive meaningful engagement and foster stronger connections with their audience, ultimately improving overall satisfaction and loyalty.
Conclusion: Mastering Sentiment Assessment Optimization in Survey Feedback
Mastering Sentiment Assessment Optimization is a pivotal step toward unlocking valuable insights from survey feedback. By implementing effective techniques for emotional tone analysis, organizations can better understand customer sentiments, ultimately leading to improved experiences. This mastery necessitates a comprehensive approach, including rigorous data collection, the identification of emotional indicators, and the deployment of advanced analytical tools.
Moreover, leveraging these insights enables strategic decisions that resonate with target audiences. Continuous refinement of sentiment assessment processes ensures that organizations remain attuned to customer needs. As we conclude, it's evident that optimizing sentiment assessment is not just beneficial; it is essential for fostering meaningful connections and driving organizational success in today's dynamic landscape.