Best Conversational AI Agents for Predictive Qualitative Analysis
-
Bella Williams
- 10 min read
Predictive Conversational Insights serves as a vital tool for organizations seeking deeper understanding through qualitative analysis. In an era where data drives decisions, the ability to analyze conversations efficiently can unveil hidden patterns and actionable insights. With advanced conversational AI agents, businesses can transform raw dialogue into meaningful data that influences strategic planning.
Exploring predictive conversational insights not only enhances the clarity of data but also increases its accuracy. By employing natural language processing and machine learning algorithms, these AI systems can extract nuances from conversations that traditional methods might overlook. This section highlights the importance of integrating these insights into your analysis framework, paving the way for informed decision-making and improved outcomes.
Analyze & Evaluate Calls. At Scale.
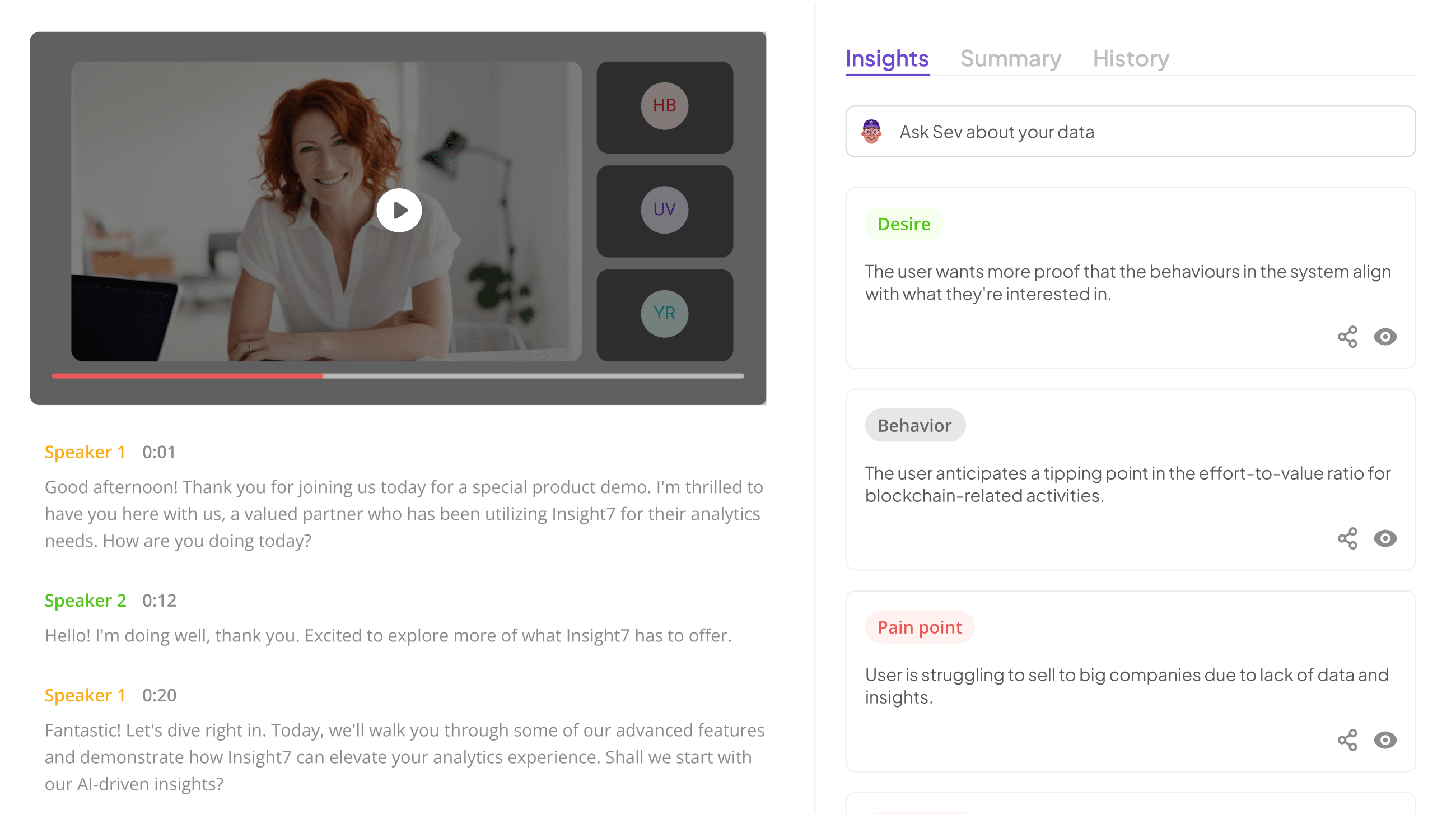
The Power of Predictive Conversational Insights in AI
In the realm of conversational AI, the capability to derive predictive conversational insights is transformative. This technology harnesses data from customer interactions, making it possible to predict trends and behaviors effectively. Organizations leveraging these insights are empowered to make timely decisions, enhancing their competitive edge in ever-evolving markets. The ability to unearth qualitative data through AI-driven analysis streamlines workflows, allowing teams to focus on actionable strategies rather than time-consuming data crunching.
Predictive conversational insights serve as a bridge between vast amounts of customer data and meaningful business outcomes. By employing natural language processing and machine learning, AI can analyze conversations to identify patterns and anticipate customer needs. Consequently, this not only improves data accuracy but also fosters deeper customer understanding. As businesses navigate the complexities of modern customer relationships, embracing predictive insights is key to harnessing the full power of conversational AI and driving innovation.
Understanding Predictive Conversational Insights
Predictive Conversational Insights play a pivotal role in transforming qualitative analysis into actionable strategies. These insights facilitate a deeper understanding of customer feedback by analyzing conversation patterns and preferences. As organizations navigate the complexities of customer interactions, harnessing these insights allows for more precise predictions of future behaviors and needs.
Incorporating advanced technologies, such as natural language processing and machine learning, enhances the effectiveness of predictive conversational insights. These tools process vast amounts of textual data, ensuring a sharper focus on key trends and emerging themes. By improving data accuracy, companies can respond to consumer demands swiftly and efficiently. This agility creates a competitive advantage in today’s fast-paced market, enabling teams to transform gathered insights into effective business actions and strategies seamlessly. Embracing these innovations can dramatically improve both customer experiences and organizational successes.
- Definition and relevance in qualitative analysis
Predictive conversational insights play a crucial role in qualitative analysis by providing a framework for interpreting human interactions and intentions. At its core, this concept involves the use of AI-driven technology to gather and analyze data from conversations, empowering businesses to derive meaningful insights. These insights allow organizations to capture the nuances of customer sentiments, preferences, and behaviors, fostering improved decision-making processes.
The relevance of predictive conversational insights extends beyond mere data collection; it transforms the qualitative analysis landscape. By utilizing advanced algorithms and natural language processing, organizations can automate evaluations and enhance their understanding of qualitative data. This not only increases efficiency but also improves the accuracy of insights derived from diverse conversations. Ultimately, implementing these insights helps businesses develop customer-centric strategies, optimize user experiences, and drive overall engagement.
- How predictive conversational insights enhance data accuracy
Predictive conversational insights play a vital role in enhancing data accuracy in various analytical processes. By capturing and interpreting customer interactions in real-time, these insights enable organizations to discern patterns and trends that traditional methods might overlook. As conversations unfold, data is dynamically generated, allowing for a more nuanced understanding of client needs and preferences.
Moreover, the integration of natural language processing and machine learning algorithms ensures that these insights are not only accurate, but also actionable. For example, as conversational AI agents analyze dialogues, they can detect sentiment, identify key pain points, and predict future behaviors. These capabilities pave the way for more informed decision-making, ultimately leading to better strategic initiatives. When organizations harness predictive conversational insights effectively, they can achieve significant improvements in data accuracy and overall performance.
In conclusion, the proactive approach offered by predictive conversational insights transforms raw data into valuable knowledge, enhancing the ability to respond to customer needs in real-time. This responsiveness is crucial in today's fast-paced business environment, making predictive insights a cornerstone for success.
Key Components of Effective Predictive Conversational Insights
Effective predictive conversational insights rely on several key components that enhance their performance in qualitative analysis. The first crucial element is natural language processing (NLP), which allows conversational AI agents to understand and interpret human language nuances effectively. By processing text or speech, NLP helps the AI discern intent and context, enabling it to provide more accurate responses. This understanding is essential for generating insights that reflect authentic customer sentiments.
Another vital component is the integration of machine learning algorithms. These algorithms analyze patterns from vast datasets, helping the AI evolve and improve over time. They facilitate predictive analysis, allowing the system to offer forecasts based on historical conversational data. This predictive capability empowers organizations to respond proactively to customer needs, ultimately driving better decision-making and strategic planning. Together, NLP and machine learning equip businesses with actionable insights, transforming raw data into valuable information that fuels growth and innovation.
- Natural language processing
Natural language processing (NLP) stands as a foundational element in creating effective predictive conversational insights. At its core, NLP enables machines to understand, interpret, and generate human language in a meaningful way. This understanding transforms raw data from customer interactions into valuable insights that can inform business strategies.
Implementing NLP allows organizations to analyze language patterns and sentiments in customer responses. By identifying key phrases and emotional undertones, businesses can gain a deeper understanding of customer needs and preferences. This capability not only enhances the quality of data analysis but also accelerates the pace at which companies can act on actionable insights. For instance, a machine learning model can recognize specific trends in customer sentiment, allowing businesses to adjust their services swiftly. Ultimately, marrying NLP with predictive conversational insights empowers organizations to better engage with customers and stay ahead in a competitive marketplace.
- Machine learning algorithms
Machine learning algorithms serve as the backbone of predictive conversational insights. These algorithms analyze large datasets to identify patterns and trends, enabling conversational AI agents to generate insightful responses. By employing advanced techniques, machine learning enhances the accuracy and reliability of insights derived from customer interactions. This capability is vital in qualitative analysis, where understanding nuances in user feedback can significantly influence decision-making.
One key benefit of using machine learning algorithms is their ability to continuously improve over time. As they process more data, these systems learn from previous interactions, refining their predictive capabilities and delivering more personalized user experiences. Additionally, the integration of natural language processing within these algorithms enhances their understanding of context and sentiment, further enriching the insights they provide. Ultimately, leveraging these technologies equips organizations with the tools to gain deeper, actionable predictive conversational insights that drive innovation and responsiveness.
Extract insights from interviews, calls, surveys and reviews for insights in minutes
Top Conversational AI Agents for Predictive Qualitative Analysis
The world of predictive qualitative analysis is evolving dramatically, with conversational AI agents taking center stage. These advanced tools leverage sophisticated algorithms to provide predictive conversational insights, enabling organizations to derive meaningful patterns from extensive datasets. By analyzing conversations, these AI agents can reveal sentiments and trends that drive strategic decisions.
Several top conversational AI agents stand out in this space. IBM Watson Assistant offers robust natural language processing capabilities tailored for specific industries, enhancing user interactions. Google Dialogflow excels in integration and customization, making it an excellent choice for businesses seeking adaptable solutions. Microsoft Bot Framework shines with its scalability, providing extensive options for organizations of all sizes. Finally, Amazon Lex stands out for its seamless integration with AWS, making it suitable for various real-world applications. Collectively, these agents drive the future of qualitative analysis through innovative predictive conversational insights.
Insight7: Leading the Way
In the realm of predictive conversational insights, it's crucial to lead with innovation and adaptability. This section focuses on driving engagement through advanced AI technology, reshaping how organizations interpret data and enhance their decision-making processes. By utilizing predictive conversational insights, businesses can uncover valuable trends within customer interactions, transforming them into actionable strategies for growth.
A successful implementation hinges on three main components: comprehensive natural language processing, adaptive machine learning algorithms, and strong user collaboration. Each element plays a significant role in interpreting the nuanced language of customer feedback. For instance, natural language processing allows the AI to understand context and sentiment, while machine learning continuously improves analysis accuracy over time. Lastly, fostering collaboration among stakeholders leads to meaningful discussions rooted in real-time insights. These foundational aspects empower companies to take the lead in their respective industries, ensuring their strategies remain both relevant and impactful.
- Unique features and capabilities
The unique features and capabilities of conversational AI agents significantly enhance the potential for predictive qualitative analysis. These agents leverage advanced natural language processing (NLP) and machine learning to transform raw dialogue into meaningful predictive conversational insights. By understanding context, intent, and tone, they offer a nuanced approach to analyzing customer interactions, thereby facilitating a deeper understanding of user sentiments and preferences.
Moreover, these systems are designed to adapt to various industries, making them versatile tools for businesses. They can automatically generate reports that identify recurring themes and suggest areas for improvement. Their ability to operate in real time ensures that organizations can swiftly respond to customer feedback and refine their strategies accordingly. With features like customizable frameworks and integration capabilities, these conversational AI agents stand out as crucial partners in achieving qualitative excellence.
- Case studies of successful implementations
In exploring the successful implementations of predictive conversational insights, we uncover transformative use cases that highlight their impact. Various organizations have effectively utilized conversational AI agents to analyze vast amounts of qualitative data, ultimately leading to enhanced decision-making processes. These agents employ advanced technologies, enabling them to extract meaningful insights from customer interactions and feedback.
One notable example involves an organization that integrated a conversational AI platform to streamline its customer service operations. By analyzing the data generated from customer inquiries, they identified common themes and trends, which directly informed their marketing strategies. This feedback loop not only improved customer satisfaction but also led to meaningful adjustments in their product offerings. Another case illustrates how predictive conversational insights helped a company benchmark employee performance against established criteria, providing insights that facilitated targeted training and development initiatives. These implementations demonstrate the potential of predictive conversational insights in realizing significant operational benefits.
Other Top AI Agents
Several AI agents stand out in the realm of predictive conversational insights, each offering unique features tailored for qualitative analysis. IBM Watson Assistant excels in natural language understanding, making it suitable for various industries from healthcare to finance. Its ability to incorporate industry-specific terminology enhances the interaction quality, leading to richer insights.
Google Dialogflow is another prominent agent, praised for its robust integration capabilities and user-friendly design. This platform allows businesses to easily customize their conversational interfaces, ensuring that predictive conversational insights are tailored to specific customer needs. Microsoft Bot Framework also deserves mention; it's known for its scalability and adaptability, making it a strong choice for organizations aiming to expand their AI implementations. Lastly, Amazon Lex, with its seamless integration with AWS services, provides numerous use cases, particularly for e-commerce and customer service sectors. Each of these agents contributes significantly to the field by enabling more informed decision-making through predictive conversational insights.
- IBM Watson Assistant
The IBM Watson Assistant serves as a powerful tool in the field of predictive qualitative analysis. This conversational AI agent leverages advanced natural language processing and machine learning technologies to generate predictive conversational insights. Through its ability to understand user interactions, it effectively recognizes patterns and sentiments in conversations, helping businesses make informed decisions based on user feedback.
💬 Questions about Best Conversational AI Agents for Predictive Qualitative Analysis?
Our team typically responds within minutes
One of the standout features of this AI agent is its adaptability across various industries. Whether in healthcare, finance, or customer service, the assistant can tailor its responses to meet specific sector needs. Furthermore, its user-friendly interface allows organizations to visualize insights easily, driving smarter engagement strategies. By utilizing IBM Watson Assistant, companies can significantly enhance the quality of their qualitative research, ultimately leading to more accurate and reliable insights.
- Overview of features
Conversational AI agents play a crucial role in delivering Predictive Conversational Insights, enabling organizations to unlock deep understanding from qualitative data. These advanced technologies harness natural language processing and machine learning to analyze vast amounts of conversational data. This analysis provides actionable insights that enhance decision-making, driving better business outcomes.
Key features of these agents include user-friendly interfaces that democratize data access across teams. Users do not require extensive training or technical expertise to engage with the platforms. Additionally, the ability to transcribe and categorize conversations into actionable reports simplifies the process of identifying critical pain points and customer sentiments. Real-time analysis allows teams to swiftly address issues and adapt strategies accordingly. Collectively, these features empower businesses to improve customer experiences and drive innovation, solidifying the essential role of Predictive Conversational Insights in a data-driven ecosystem.
- Industry-specific applications
Industries today are increasingly recognizing the potential of Predictive Conversational Insights to drive meaningful outcomes in their specific contexts. For instance, the healthcare sector utilizes these insights to facilitate patient interactions and enhance care delivery. By analyzing patient conversations, healthcare professionals can identify concerns and tailor their approaches accordingly, leading to improved patient satisfaction and outcomes.
Similarly, in retail, businesses leverage conversational insights to fine-tune customer engagement strategies. By interpreting customer feedback from conversations, retailers can adjust their product offerings and services to meet evolving consumer needs. This not only enhances customer loyalty but also optimizes inventory management and sales strategies.
A few industry-specific applications include:
- Healthcare: Streamlining patient inquiries and improving treatment adherence.
- Retail: Personalizing customer experiences and boosting sales through targeted recommendations.
- Finance: Analyzing customer sentiments to enhance service offerings and mitigate risks.
Each of these examples underscores the versatility of Predictive Conversational Insights across different industries, affirming its role as a catalyst for informed decision-making and strategic growth.
- Google Dialogflow
With its remarkable integration capabilities, robust architecture, and user-friendly interface, Google Dialogflow stands out as a leading conversational AI agent for enabling predictive conversational insights. This platform facilitates the creation of intelligent chatbots and virtual assistants by utilizing natural language understanding (NLU). As a result, businesses can engage their customers in meaningful conversations, extracting valuable data while enhancing user experience.
One of its key strengths is customizability, allowing organizations to tailor the conversational flow and response strategies to align with specific goals. This adaptability plays a significant role in qualitative analysis, as businesses can adjust their engagement techniques based on real-time insights. Ultimately, Google Dialogflow empowers organizations to harness predictive conversational insights, turning customer interactions into actionable data that drives decision-making and improves service delivery. Such capabilities make it an invaluable tool for organizations seeking to enhance their predictive analytics strategies.
- Integration capabilities
Integration capabilities play a pivotal role in maximizing the potential of Predictive Conversational Insights. Various conversational AI agents have unique strengths in seamlessly integrating with existing systems and workflows. These integrations enable businesses to harness insights derived from customer conversations, ensuring efficient data analysis and prompt decision-making.
Several key factors stand out in evaluating integration capabilities. First, compatibility with existing CRM systems facilitates real-time data exchange. This helps companies merge conversational insights with customer profiles effectively. Second, support for various communication channels allows insights to flow smoothly across platforms, enhancing user experience and feedback collection. Third, intuitive APIs empower teams to customize workflows, adapting the conversational AI to meet specific business needs without inherent complexities. By focusing on these integration aspects, organizations can fully leverage the power of Predictive Conversational Insights to shape strategies, drive engagement, and gain a competitive edge.
- Customizability and ease of use
Customizability and ease of use are vital when assessing conversational AI agents for predictive qualitative analysis. A user-friendly interface allows non-technical users to easily navigate and leverage the full potential of the technology. When tools are designed with flexibility in mind, they empower users to tailor the experience to specific needs and business contexts. Predictive conversational insights become more accessible and actionable when the underlying systems allow for extensive personalization.
Moreover, the ability to customize responses and data inputs enhances the insights derived from conversations. Users can adjust parameters according to the nuances of their industry or target audience, ensuring more relevant findings. This adaptability fosters a more intuitive user experience, reducing barriers for both seasoned analysts and newcomers. Consequently, the seamless integration of these features elevates the overall effectiveness of conversational AI in delivering meaningful predictive insights.
- Microsoft Bot Framework
Microsoft Bot Framework enables organizations to create sophisticated conversational AI agents tailored for predictive qualitative analysis. Its versatile infrastructure provides tools for building chatbots capable of handling varied tasks. By leveraging this framework, businesses can gather Predictive Conversational Insights, transforming customer interactions into actionable intelligence.
The framework's strengths lie in its scalability and extensibility, allowing developers to craft solutions that grow alongside business needs. Integrated with natural language processing capabilities, the Bot Framework enhances the accuracy of data collected during customer interactions. This ensures that organizations can continuously refine their offerings based on real-time feedback and emerging trends. Consequently, businesses can expect improved engagement and a better understanding of consumer behavior, driving their marketing initiatives and strategic decisions.
- Scalability and extensibility
Scalability and extensibility are crucial for any platform aiming to deliver predictive conversational insights effectively. A scalable solution can handle increasing amounts of data and user interactions without sacrificing performance. This capability allows organizations to grow seamlessly while accessing intricate customer feedback at scale. By using advanced AI tools, businesses can capture a wider array of conversational data, ensuring that the insights generated remain relevant as demand rises.
Extensibility, on the other hand, refers to the ability to enhance and adapt the platform as business needs evolve. This may include integrating with additional applications, or incorporating novel analytical techniques. As organizations continually refine their strategies, they require tools that can evolve alongside their insights processes. By prioritizing scalability and extensibility, companies not only improve operational efficiency but also strengthen their competitive edge through timely and actionable predictive conversational insights.
- Strengths in business solutions
In an era driven by data, the strength of business solutions lies significantly in their ability to transform information into actionable insights. Predictive conversational insights empower organizations by enabling them to analyze customer interactions in real time. This not only enhances communication strategies but also fosters a deeper understanding of customer needs and preferences. As technology evolves, businesses are shifting from traditional roles to become trusted advisors, guiding customers toward optimal choices.
One of the key strengths of predictive conversational insights is their adaptability across various industries. Businesses can harness data from diverse sources, resulting in tailored solutions that reflect unique market demands. These insights support decision-making through enhanced accuracy and timely feedback tailored to specific queries and market conditions. Additionally, the application of advanced analytics provides businesses with the tools to anticipate trends, ensuring they remain competitive. Adopting these insights can significantly elevate business efficacy, transforming challenges into opportunities.
- Amazon Lex
Amazon Lex offers a powerful framework for building conversational interfaces using advanced machine learning capabilities. This technology specializes in understanding natural language, which significantly enhances the extraction of predictive conversational insights. By providing developers with tools to create chatbots and voice assistants, it supports applications across various industries such as customer service, healthcare, and finance.
The platform seamlessly integrates with numerous AWS services, helping businesses analyze customer interactions more efficiently. This allows organizations to harness predictive conversational insights through data gathered from conversations. Companies can optimize their services, personalize the user experience, and improve customer satisfaction by analyzing patterns in these interactions. Overall, Amazon Lex simplifies the process of creating meaningful and engaging conversational agents that can adapt to evolving customer needs. As such, it stands out as a vital tool in predictive qualitative analysis.
- Seamless integration with AWS
Integrating conversational AI agents seamlessly with AWS revolutionizes how organizations utilize Predictive Conversational Insights. By leveraging AWS's robust cloud infrastructure, AI agents can access vast data storage solutions and computing power, enabling faster processing of qualitative data. This integration allows for efficient retrieval and analysis of valuable insights derived from customer interactions.
When utilizing Amazon Lex, businesses benefit from quality enhancements and scalability. The natural language processing capabilities inherent in AWS ensure high accuracy in understanding user intent and sentiment. Moreover, AWS's security features protect sensitive data, fostering trust and compliance with regulations.
This seamless integration empowers organizations to refine their consulting approaches, moving beyond simple query responses. Instead, they can engage customers in meaningful conversations that uncover deeper insights, improving overall decision-making and strategic outcomes. As a result, companies can evolve into adaptive entities that meet real-time market demands effectively.
- Use cases and industry relevance
Predictive conversational insights have become essential in various industries, transforming how organizations analyze qualitative data. They empower companies to extract deeper meanings from conversations, generating actionable insights that drive decision-making. Businesses in sectors like customer service, healthcare, and finance are particularly benefitting from these advancements. For instance, analyzing customer interactions can illuminate trends and concerns, helping brands enhance their offerings and support.
Several use cases highlight the relevance of predictive conversational insights. First, quality assurance evaluations in customer service allow organizations to assess agent performance and compliance with established guidelines. Second, in healthcare, these insights can refine patient interactions, ensuring that critical information is captured and understood. Lastly, in finance, institutions utilize conversational insights to improve client communications, ensuring transparency and trust. As industries continue to evolve, harnessing these insights will prove invaluable for strategic growth and improved customer engagement.
Conclusion: Harnessing Predictive Conversational Insights for Qualitative Success
In conclusion, harnessing predictive conversational insights is vital for qualitative success. These insights empower organizations to transform vast amounts of customer dialogue into actionable strategies that resonate with target audiences. By automating the analysis of customer interactions, businesses can swiftly identify trends and adapt their approaches, staying ahead of the competition.
Ultimately, the effectiveness of predictive conversational insights lies in their ability to reveal not just the data itself, but the underlying customer sentiments. Embracing these insights facilitates more meaningful interactions, leading to enhanced decision-making and improved customer experiences. Organizations that prioritize this innovative approach can unlock significant qualitative advantages.
💬 Questions about Best Conversational AI Agents for Predictive Qualitative Analysis?
Our team typically responds within minutes