In today’s fast-paced world, qualitative research plays a crucial role in understanding customer behavior, preferences, and experiences. However, analyzing qualitative data can be time-consuming and labor-intensive. Fortunately, advancements in artificial intelligence (AI) have led to the development of powerful tools that can streamline the qualitative research process, making it easier for researchers to extract valuable insights from their data. In this guide, we will explore some of the best AI qualitative research analysis tools available today, starting with Insight7, a leading platform in this space.
Understanding the Need for AI in Qualitative Research
Qualitative research plays a vital role in understanding human behavior, perceptions, and experiences. However, the challenges of analyzing vast amounts of qualitative data can be overwhelming. Traditional methods of manual analysis often lead to biases and inefficient workflows, hindering the reliability of insights. This is where AI comes in, transforming qualitative research through automation and advanced analytics.
AI can streamline transcription, help identify patterns, and extract meaningful insights from various data formats, such as text, audio, and video. By utilizing top AI analysis tools, researchers can ensure a smoother and more accurate analysis process. These tools improve efficiency and accuracy, allowing teams to focus on interpreting the data rather than getting bogged down in tedious manual work. Embracing AI not only enhances research quality but also empowers teams to derive actionable insights from complex qualitative datasets.
Top AI Analysis Tools in the Market
1. Insight7
Insight7 is an innovative platform designed to help teams analyze qualitative data at scale. With its user-friendly interface and powerful features, Insight7 enables researchers to quickly synthesize insights from interviews, focus groups, and open-ended survey responses. Here are some key features of Insight7:
- Automated Transcription: Insight7 offers a built-in transcription service that converts audio and video recordings into text with high accuracy (up to 99%). This feature saves researchers significant time and effort in manual transcription.
- Thematic Analysis: The platform automatically extracts themes, pain points, and desires from the data, allowing researchers to quickly identify key insights without sifting through lengthy transcripts.
- Multi-Project Analysis: Insight7 allows users to group related files into projects, enabling them to analyze multiple interviews or focus groups collectively. This feature helps researchers identify patterns and trends across different data sets.
- Customizable Reporting: Users can generate reports that summarize key findings, insights, and recommendations based on the analysis. This feature is particularly useful for presenting results to stakeholders.
- Data Privacy Compliance: Insight7 takes data privacy seriously, ensuring that all customer data is stored securely and in compliance with regulations such as GDPR.
To get started with Insight7, you can sign up here for a free trial and experience the platform’s capabilities firsthand.
Extract insights from interviews, calls, surveys and reviews for insights in minutes
2. NVivo
NVivo is a widely used qualitative data analysis software that helps researchers organize and analyze unstructured data. It offers a range of features, including:
- Data Organization: NVivo allows users to import various data types, including text, audio, video, and images, making it easy to manage all research materials in one place.
- Coding and Tagging: Researchers can code data segments and tag them with themes or categories, facilitating thematic analysis.
- Querying and Visualization: NVivo provides powerful querying tools that enable users to explore relationships between codes and visualize data through charts and models.
3. MAXQDA
MAXQDA is another robust qualitative data analysis tool that supports researchers in analyzing qualitative and mixed-methods data. Key features include:
- Flexible Data Import: Users can import data from various sources, including surveys, interviews, and focus groups, as well as social media and web content.
- Visual Tools: MAXQDA offers a range of visualization tools, such as word clouds, charts, and models, to help researchers present their findings effectively.
- Team Collaboration: The software supports collaborative work, allowing multiple users to work on the same project simultaneously.
4. Dedoose
Dedoose is a web-based qualitative data analysis tool that is particularly useful for mixed-methods research. Its features include:
- User-Friendly Interface: Dedoose is designed for ease of use, making it accessible for researchers with varying levels of technical expertise.
- Real-Time Collaboration: The platform allows multiple users to collaborate on projects in real-time, making it ideal for team-based research.
- Data Visualization: Dedoose provides various visualization options to help researchers present their findings clearly and effectively.
5. Atlas.ti
Atlas.ti is a powerful qualitative data analysis software that helps researchers analyze complex data sets. Key features include:
- Data Management: Atlas.ti allows users to manage large volumes of data, including text, audio, video, and images, in a single project.
- Coding and Memoing: Researchers can code data segments and create memos to capture insights and reflections throughout the analysis process.
- Network Views: The software offers network views that help researchers visualize relationships between codes and themes.
6. Qualaroo
Qualaroo is a qualitative research tool that focuses on gathering customer feedback through targeted surveys and interviews. Its features include:
- Targeted Surveys: Qualaroo allows users to create targeted surveys that can be embedded on websites or sent via email to gather customer insights.
- Real-Time Feedback: The platform enables researchers to collect real-time feedback from customers, helping them understand their needs and preferences.
- Data Analysis: Qualaroo provides basic data analysis tools to help researchers make sense of the feedback collected.
7. UserTesting
UserTesting is a platform that specializes in user experience research, allowing researchers to gather insights from real users interacting with products or services. Key features include:
- Video Feedback: Researchers can watch videos of users completing tasks and hear their thoughts in real-time, providing valuable qualitative insights.
- Customizable Tests: UserTesting allows users to create customized tests based on specific research goals and objectives.
- Analytics Dashboard: The platform provides an analytics dashboard that summarizes key findings and trends from user feedback.
Conclusion:
The future of qualitative research analysis is undeniably intertwined with technology, particularly through the use of top AI analysis tools. By embracing these advancements, researchers can drastically enhance their capabilities, transforming how they gather and interpret valuable insights. The integration of AI tools not only streamlines the data collection process but also improves the accuracy of findings, enabling organizations to make informed decisions more swiftly.
As we look ahead, it is essential to recognize the impact of adopting these innovative tools. With top AI analysis methods, researchers are empowered to discover deeper patterns in qualitative data, thereby enriching their understanding of human behavior and preferences. Embracing these technologies will undoubtedly position organizations for greater success in their research endeavors, allowing them to remain competitive in a rapidly evolving landscape.
Analyze & Evaluate Calls. At Scale.
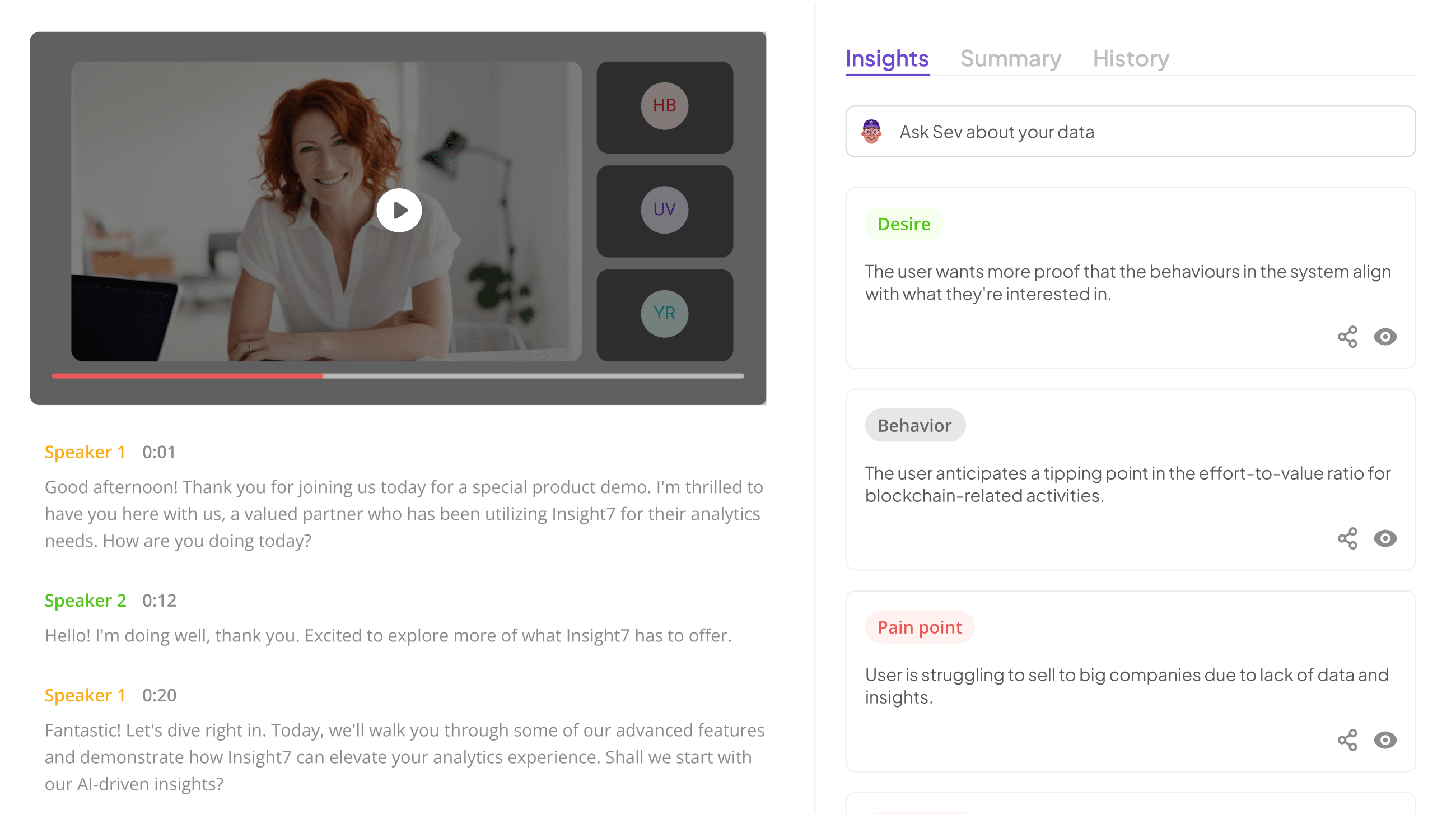