Best AI Methods to Analyze Product Positioning from Prospect Calls
-
Hello Insight
- 10 min read
Prospect Call Insights serve as a valuable resource for understanding customer opinions and preferences, ultimately guiding product positioning. Engaging with potential customers through calls not only sheds light on their needs but also highlights opportunities for product improvements. These insights allow businesses to create tailored messaging that resonates effectively with their target audience.
By analyzing the conversations that unfold during prospect calls, organizations can identify key product affinities and customer sentiments. This understanding enables them to refine their strategies and align offerings more closely with market demands. As we explore the best AI methods to analyze these insights, we will uncover techniques that drive actionable outcomes and enhance overall product positioning.
Analyze & Evaluate Calls. At Scale.
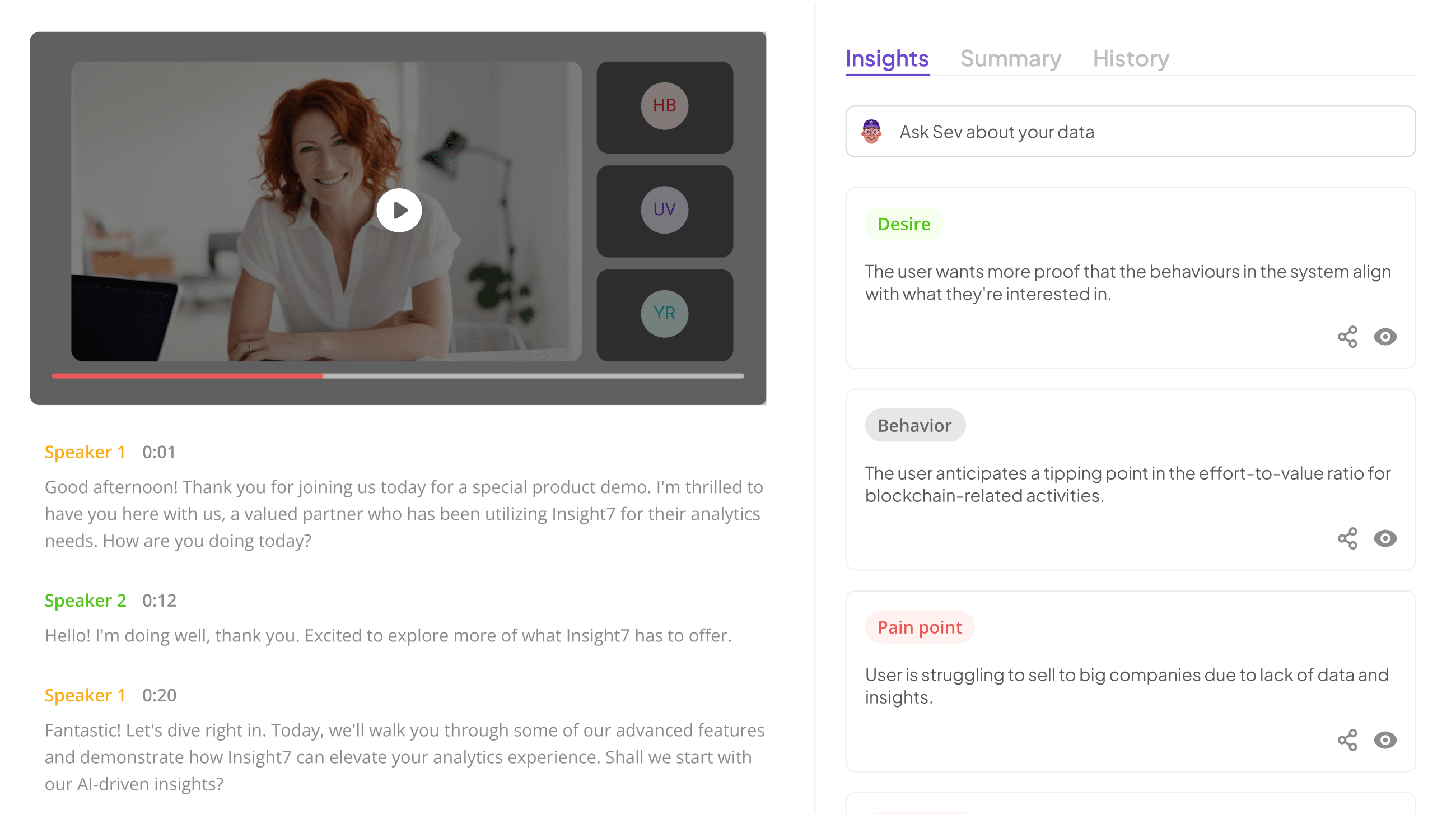
Understanding the Role of Prospect Call Insights
Prospect Call Insights play a vital role in understanding customer needs and preferences during product positioning. By analyzing these calls, businesses can grasp the emotions and attitudes conveyed by potential customers. This valuable information helps organizations fine-tune their messaging and identify how their offerings align with market demands.
The insights gathered from prospect calls facilitate decision-making processes in product development. First, they help clarify customer sentiments, revealing strengths and weaknesses in product offerings. Second, these insights uncover key product affinities by highlighting which features resonate most with customers. This dual approach fosters a deeper understanding of the target audience, ultimately guiding more effective product positioning strategies. Emphasizing both sentiment analysis and product affinity assessment ensures a comprehensive grasp of customer expectations, leading to better alignment with market trends.
Analyzing Customer Sentiments
Analyzing customer sentiments provides a unique lens through which businesses can gauge the effectiveness of their product positioning strategies. Prospect Call Insights illuminate how potential clients perceive offerings, highlighting emotional undertones and preferences. By examining the nuances in customer language, organizations can uncover valuable feedback that goes beyond surface-level comments.
To capture a comprehensive understanding of customer sentiments, consider the following approaches:
Transcription of Calls: First, utilize advanced tools to transcribe calls accurately. This step ensures that every detail is accounted for.
Sentiment Analysis: Next, apply AI-driven sentiment analysis to detect positive, negative, or neutral emotions within discussions. This analysis reveals how customers feel about the product.
Follow-up Interviews: Finally, conducting follow-up interviews can provide deeper context, enhancing the initial insights gathered from the calls. This triangulation of data fosters more informed decision-making around product positioning.
By effectively analyzing customer sentiments, businesses can better align their products with consumer expectations and needs, significantly enhancing engagement and satisfaction.
Identifying Key Product Affinities
Understanding key product affinities is crucial for optimizing product positioning based on prospect call insights. By analyzing conversations, businesses can discover patterns that reveal how products resonate with potential customers. Identifying these affinities allows organizations to tailor their marketing strategies, enhancing messaging and product features to meet customer preferences.
There are several steps to consider for effective identification of product affinities. First, analyze sentiment during prospect calls to gauge initial impressions of product offerings. Next, categorize the features and benefits highlighted by prospects, drawing connections between their needs and your products. Finally, leverage tools like Insight7 for quantitative analysis of this qualitative data, enabling a deeper understanding of how specific attributes align with customer desires. By following these steps, businesses can make more informed decisions and create stronger connections with their target audience.
Extract insights from interviews, calls, surveys and reviews for insights in minutes
Best AI Methods for Extracting Prospect Call Insights
Extracting prospect call insights effectively hinges on utilizing advanced AI methods designed for comprehensive data analysis. First, employing Natural Language Processing (NLP) techniques is vital. This begins with robust data collection, where every recorded call is transcribed for in-depth analysis. Once the data is gathered, sentiment analysis follows, enabling businesses to gauge customer emotions and opinions during the interactions, providing invaluable context.
Following sentiment analysis, topic modeling is conducted to uncover prevalent themes and keywords mentioned by prospects. This allows for a better understanding of customer interests and pain points. Furthermore, machine learning algorithms can be utilized to train models with historical call data. This step helps in identifying patterns and making predictive analyses for future product positioning. By integrating these methods, organizations can derive meaningful insights that directly inform product strategies and enhance customer engagement.
Natural Language Processing Techniques
Natural Language Processing (NLP) techniques play a pivotal role in extracting valuable Prospect Call Insights. These techniques allow businesses to analyze conversations and decipher sentiments, providing a deeper understanding of customer perspectives. By leveraging text analysis, vendors can categorize feedback and identify prevalent themes, enabling them to refine their product positioning.
First, data collection is essential. Utilizing tools like Insight7 simplifies gathering raw text from prospect calls. Following this, sentiment analysis evaluates positive or negative sentiments expressed during discussions. Lastly, topic modeling reveals common subjects discussed by prospects, presenting clear insights into customer interests and pain points. By systematically applying these NLP techniques, organizations can significantly improve their ability to translate call data into actionable strategies for product positioning.
Step 1: Data Collection
Data collection is a critical first step in extracting valuable insights from prospect calls. This process involves gathering and recording a wide range of call data, which can include customer feedback, inquiries, and preferences. Understanding the nuances of these interactions is essential for recognizing trends and patterns that may inform product positioning. Aim to capture data not only from the conversation itself but also from any supporting materials shared during the calls.
To effectively collect prospect call insights, consider the following approaches:
Transcription of Calls: Accurately transcribing conversations allows for in-depth analysis of language use and sentiment. Automated tools, such as Insight7, can streamline this process.
Data Storage: Ensure that your data is organized and easily accessible for further analysis. A structured database can facilitate efficient retrieval and examination of insights.
Categorization of Insights: Grouping data into relevant categories can help identify common customer pain points and preferences, enabling targeted strategies for product positioning.
Through careful data collection, businesses can lay the foundation for a comprehensive understanding of their prospects, ultimately improving decision-making and product alignment in the market.
Step 2: Sentiment Analysis
Sentiment analysis plays a crucial role in understanding Prospect Call Insights, helping businesses grasp customer attitudes and experiences. By utilizing AI tools, organizations can study emotions expressed during conversations and identify underlying sentiments toward products or services. This step allows for a nuanced understanding of customer perspectives, which is vital in tailoring effective marketing strategies.
To perform sentiment analysis effectively, focus on the following aspects:
- Data Processing: Convert call recordings into transcriptions for accurate analysis. Tools like Insight7 can assist in transcription and data organization.
- Emotion Detection: Utilize natural language processing to analyze words and phrases that convey emotions, identifying positive or negative sentiments.
- Trend Analysis: Monitor changes in sentiment over time, gaining insights into how shifts in customer opinions might impact product positioning and marketing strategies.
By thoroughly analyzing sentiment, companies can better align their products with customer preferences, enhancing overall market strategies.
Step 3: Topic Modeling
The process of topic modeling plays a crucial role in extracting valuable Prospect Call Insights from your conversations. By clustering similar discussions, this method helps in identifying the primary themes that resonate with your prospects. Understanding these themes allows businesses to tailor their messaging and product offerings, ensuring they align more closely with customer expectations.
To implement effective topic modeling, consider the following steps:
- Data Preparation: Begin by cleaning and organizing your call transcripts. Remove irrelevant content to ensure clarity.
- Model Selection: Choose an appropriate algorithm, such as Latent Dirichlet Allocation (LDA), to uncover the hidden topics within your data.
- Thematic Analysis: Analyze the output to understand which topics are most prevalent and relevant to your target audience.
- Actionable Insights: Finally, integrate these insights into your marketing strategy to enhance product positioning and address customer needs more effectively.
By systematically employing topic modeling, you can derive profound insights from prospect calls, fostering a more nuanced understanding of your market.
Machine Learning Algorithms
Machine learning algorithms play a pivotal role in analyzing prospect call insights for informed product positioning. These algorithms can process vast amounts of data to uncover hidden patterns. By leveraging historical call data, models can be trained to interpret key topics and sentiments that emerge during conversations. This allows businesses to gain insights into customer preferences and pain points, which is essential for shaping effective marketing strategies.
In the implementation phase, predictive analytics is employed to forecast customer behavior based on analyzed data. For instance, models can identify which product features resonate most with prospects, enabling tailored messaging. This approach not only streamlines communication but also enhances overall customer engagement. By integrating machine learning into the analysis of prospect call insights, businesses can refine their product offerings and improve market relevance, ultimately driving sales and customer satisfaction.
Step 1: Training Models with Historical Data
Training models with historical data is a crucial first step in analyzing prospect call insights. By leveraging past conversations, organizations can identify patterns, preferences, and behaviors that inform product positioning strategies. This process begins with collecting and preparing the relevant historical data, which can include transcripts, recordings, and notes from prospect calls. Ensuring this data is well-organized is essential for effective analysis.
Once the historical data is gathered, it can be used to train machine learning models. These models learn from existing patterns and relationships in the data, enabling them to predict outcomes and understand customer sentiments more accurately. This training phase allows the model to develop a nuanced perspective that informs future strategic decisions in product positioning. Therefore, investing time and resources in this foundational step can significantly enhance the effectiveness of subsequent analyses and ultimately improve product alignment with customer needs.
Step 2: Predictive Analytics for Positioning
Effective predictive analytics can transform the way organizations approach product positioning by utilizing insights gleaned from prospect calls. By analyzing patterns and trends in customer conversations, businesses can anticipate needs and preferences, leading to better-targeted marketing strategies. This method not only improves decision-making but also enhances customer relationships through personalized experiences.
To implement predictive analytics for positioning, there are several key steps to consider:
- Data Aggregation: Integrate data from various sources to create a comprehensive view of prospect interactions.
- Model Development: Use machine learning algorithms to create predictive models based on historical call data.
- Forecasting Outcomes: Analyze the predictions to identify potential shifts in customer sentiment or market demand.
Each step plays a vital role in refining product strategies and ensuring alignment with consumer expectations. Utilizing tools like Insight7 can further enhance this process, enabling more accurate data evaluation and analysis.
Top Tools for Analyzing Prospect Call Insights
When analyzing prospect call insights, utilizing the right tools is essential for extracting valuable information from conversations. Key tools such as Insight7, CallMiner, Gong.io, Chorus.ai, and Tethr stand out in this space. These platforms leverage advanced technologies to transcribe, analyze, and evaluate call data, providing actionable insights that can significantly influence product positioning strategies.
Insight7 excels in quantitative analysis, offering robust features for evaluating customer sentiments and product affinities. Similarly, CallMiner provides detailed analytics that can uncover trends in customer feedback. Gong.io and Chorus.ai specialize in conversation analytics, allowing users to assess call dynamics and uncover key themes. Finally, Tethr offers a comprehensive approach to performance tracking, highlighting areas for improvement. By employing these tools, businesses can transform prospect call insights into targeted strategies that enhance product relevance and customer engagement.
insight7
Insight7 focuses on the significance of harnessing Prospect Call Insights to fine-tune product positioning strategies. By delving into these insights, organizations can better understand customer needs and preferences. This information helps in identifying gaps within the market and determining how products can meet those specific demands.
AI methods, such as natural language processing and machine learning algorithms, play a vital role in extracting meaningful insights from prospect conversations. For instance, sentiment analysis captures the emotional undertones of customer feedback, revealing preferences that may not be explicitly stated. Additionally, topic modeling can uncover underlying themes that frequently arise in calls, offering deeper context for product adjustments. By regularly integrating these insights, organizations can strategically position their products, ensuring they resonate effectively with their target audience.
CallMiner
The effectiveness of analyzing prospect calls can be greatly enhanced using advanced technology. CallMiner stands out as a powerful tool for extracting valuable insights from these conversations. By analyzing tones and sentiments present in calls, this approach reveals key aspects of customer preferences and behaviors. Utilizing machine learning models, CallMiner can identify trends and patterns, enabling businesses to better understand their audience.
Through the process of data aggregation, specific metrics such as sentiment scores and call duration are generated. This information aids in pinpointing how prospective customers perceive products, allowing teams to adjust their positioning strategies effectively. By integrating insights from prospect calls into their overall marketing strategies, brands can align their offerings more closely with customer needs. This method not only enhances product positioning but also fosters stronger customer relationships through data-driven decision-making.
Gong.io
Gong.io serves as a pivotal tool for extracting profound insights from prospect calls, driving meaningful improvements in product positioning. By transcribing calls and employing advanced analytics, organizations can distill valuable information that directly impacts sales strategies. This platform captures customer interactions, enabling teams to go beyond surface-level metrics and understand the underlying motivations and sentiments expressed by prospects.
The core functionality includes analyzing conversation patterns, identifying prevalent topics, and assessing customer sentiments. This intelligence helps refine product messaging, ensuring that offerings resonate with the target audience. With efficient data collection and robust analytical capabilities, teams can pinpoint key areas of improvement and optimize their responses. Utilizing Gong.io's advanced features encourages a proactive approach to understanding prospect call insights, ultimately enabling businesses to align their product positioning with market demands more effectively. For organizations looking to enhance their decision-making processes, this tool stands out as a vital component in deriving actionable insights from prospect conversations.
Chorus.ai
Analyzing prospect call insights through AI tools offers valuable opportunities for organizations to refine their product positioning. One advanced tool that effectively captures these insights is designed to analyze conversational data, extract key points, and summarize discussions. This technology not only provides automation but also significantly enhances the depth of analysis by identifying patterns and sentiments that are not easily discernible in raw data.
To maximize the effectiveness of AI in analyzing prospect calls, several key strategies stand out. First, ensuring that data is accurately captured from calls is essential. Accurate transcription allows for reliable sentiment analysis and topic modeling. Second, employing machine learning algorithms can help tailor insights based on historical interactions, guiding future product positioning. By implementing these methods, organizations can transform prospect calls into actionable insights, enabling informed decision-making and enhanced market strategy development.
Tethr
Tethr is an insightful tool specifically designed for analyzing prospect call insights through advanced AI-driven techniques. It captures and analyzes conversations between sales teams and potential customers to extract meaningful data. This capability enables businesses to identify patterns, preferences, and pain points that may influence product positioning.
The Tethr platform employs natural language processing to break down dialogues into actionable insights. By evaluating customer sentiments and responses, it assists teams in honing their messaging strategies. Additionally, Tethr provides analytics that help correlate prospect reactions with specific product features, enhancing the understanding of market demands. In conclusion, Tethr stands out as a critical resource in leveraging prospect call insights to inform and refine product positioning efforts.
Conclusion: Leveraging Prospect Call Insights for Enhanced Product Positioning
Understanding how to effectively utilize prospect call insights is crucial for optimizing product positioning. When businesses analyze these insights, they can uncover preferences and pain points that are pivotal in shaping their market strategies. By synthesizing feedback from potential customers, companies can better align their products with market demands, enhancing relevancy and appeal.
Incorporating AI methods to analyze these insights allows for a more nuanced understanding of customer sentiment, leading to informed decision-making. Tools like Insight7 contribute significantly by providing robust analysis capabilities. Ultimately, the actionable data derived from prospect calls empowers teams to refine their messaging, ensuring that products not only meet but exceed customer expectations.