In an era of rapid technological advancement, businesses are increasingly seeking innovative solutions to enhance their quality assurance processes. The introduction of AI-Driven QA Assignment marks a pivotal shift in how organizations assess and improve their service quality. By integrating artificial intelligence into scorecard evaluations, companies can streamline their assessment processes, leading to higher efficiency and accuracy.
AI-Driven QA Assignment enables organizations to analyze data from various channels seamlessly. This approach not only automates tedious tasks but also ensures consistent evaluation against predefined criteria. As companies embrace this technology, they can focus on continuous improvement, enhancing customer satisfaction and driving overall success in their operations.
Analyze qualitative data. At Scale.
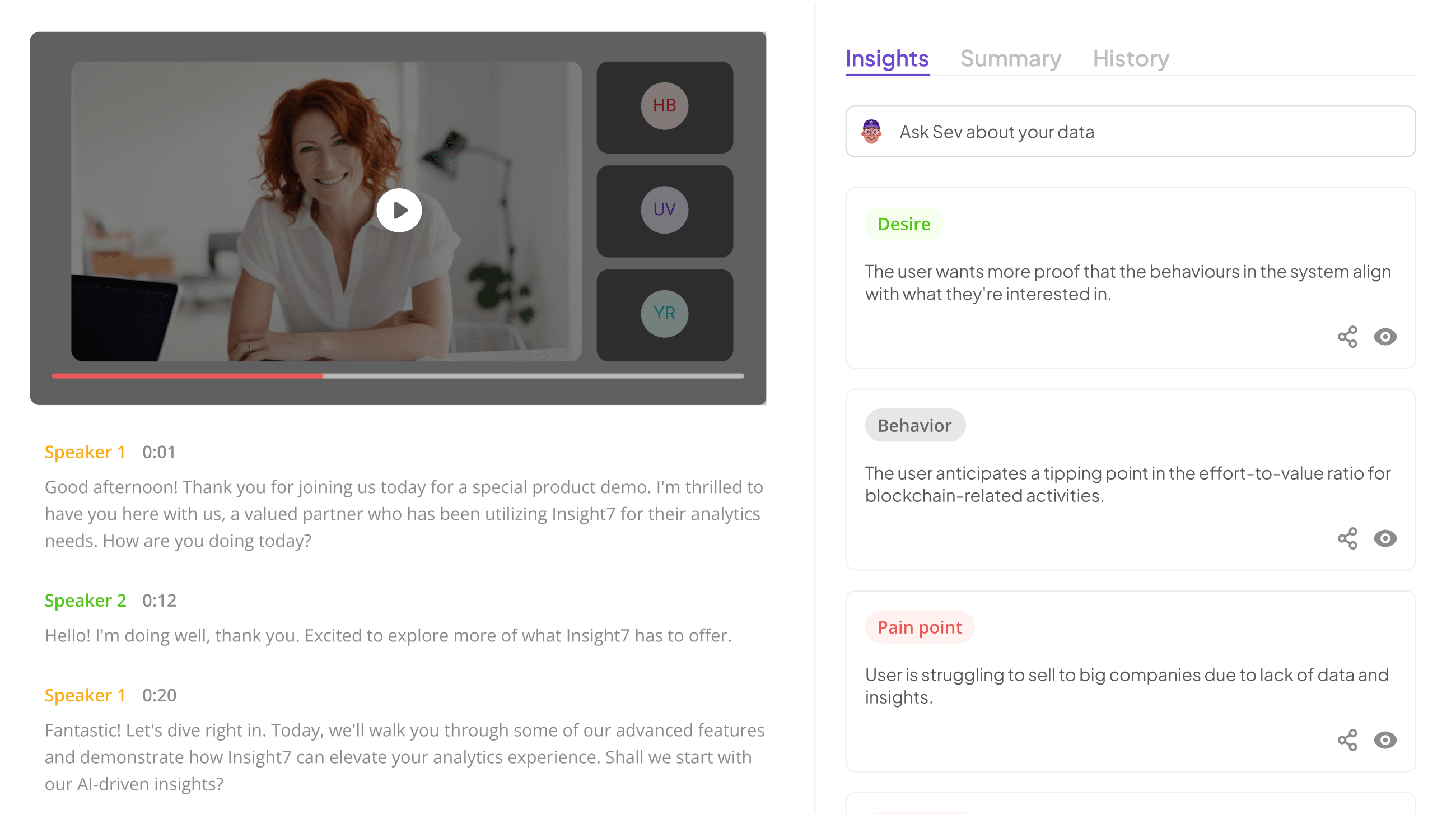
The Rise of AI in Quality Assurance
The emergence of AI in quality assurance marks a significant turning point in how organizations manage their processes. AI-driven QA assignment enhances traditional methods by offering real-time insights, streamlined workflows, and data-driven decision-making capabilities. This technology enables automation of repetitive tasks, allowing teams to focus on strategic evaluations rather than manual scorecard assignments.
Historically, quality assurance relied heavily on manual data entry and subjective assessments. As AI technologies advance, these tasks can now be performed with remarkable accuracy and speed. By harnessing machine learning algorithms, organizations can not only improve the reliability of their evaluations but also gain actionable insights that lead to continuous improvement. Consequently, AI-Driven QA Assignment is transforming the quality assurance landscape, making it more efficient and effective. Embracing this transformation is essential for organizations looking to stay competitive in a rapidly evolving digital environment.
AI-Driven QA Assignment: Transforming Traditional Processes
AI-Driven QA Assignment is redefining how organizations approach quality assurance processes. Traditionally, the task of scoring quality metrics depended heavily on manual input, which was time-consuming and often subjective. However, with the integration of AI, the assignment of QA scorecards can now be automated, leading to increased efficiency and accuracy.
One of the key transformations involves the ability to analyze vast amounts of data quickly. AI technologies can process call transcripts or other relevant materials, evaluating them against predefined criteria in real-time. This method enhances compliance monitoring and ensures consistency in evaluations. Furthermore, organizations benefit from valuable insights generated by AI, allowing them to refine their training programs and improve agent performance. Through AI-Driven QA Assignment, the transition from traditional practices to automated systems not only streamlines operations but also fosters a more reliable quality assurance framework in the workplace.
Benefits of Automating QA Scorecard Assignment
Automating QA scorecard assignment through AI technology enhances accuracy and efficiency significantly. By streamlining the assignment process, organizations reduce human error and enable teams to focus on value-added tasks. With AI-driven QA assignment, real-time data analysis unveils insights, ensuring a more robust evaluation of performance metrics.
Moreover, automation allows for consistent scorecard application across different teams and projects. This uniformity fosters collaboration and improves training processes, as feedback becomes clearer and more actionable. Organizations benefit from improved turnaround times, allowing quality assurance teams to respond swiftly to challenges. Ultimately, embracing AI-driven QA assignment facilitates data-driven decision-making, which is crucial for maintaining high standards within the organization while enhancing overall productivity.
Implementing AI-Driven QA Assignment in Your Organization
Implementing AI-Driven QA Assignment in Your Organization begins with understanding the specific quality assurance requirements. It is essential to clarify the metrics and standards you want the AI system to evaluate. Involving your team in this process fosters ownership and defines accountability, ensuring all stakeholders align on the performance goals. After establishing clear requirements, selecting the right AI tools tailored to your needs is the next critical step. This involves exploring various options that can integrate seamlessly into your existing workflows.
Once you've chosen an AI tool, develop and train your AI models using historical data and set performance benchmarks. Regular monitoring of system outputs ensures continuous improvement and adaptation to changing conditions. By following these steps, you can streamline your quality assurance processes and enhance efficiency through an AI-driven approach. This not only saves time but also allows for consistent evaluation based on defined standards, paving the way for better quality outcomes across your organization.
Extract insights from interviews, calls, surveys and reviews for insights in minutes
Steps to Automate QA Scorecard Assignment Using AI
To successfully implement AI-Driven QA Assignment, start by clearly identifying your quality assurance requirements. This involves recognizing the specific metrics and standards that will guide the scoring process. Understanding these needs will tailor the AI to your organization's goals, ensuring the system aligns perfectly with existing frameworks.
Next, choose the right AI tools that fit your operational demands. Consider software that can seamlessly integrate and support your existing processes. Once the tools are selected, develop and train your AI models using relevant data. This training will help improve accuracy and efficiency in scorecard assignments.
Finally, focus on deploying and monitoring your AI system. Regular monitoring is crucial to ensure the AI continues to perform effectively and adapt to new challenges. By following these steps, organizations can harness AI's potential to streamline their QA assignments, leading to enhanced outcomes and insights.
Step 1: Identify QA Requirements
Identifying QA requirements is a crucial first step in automating the QA scorecard assignment process. This process begins with understanding the specific goals and objectives for quality assurance within your organization. Gather input from various stakeholders to pinpoint what aspects of quality are essential to evaluate, like compliance, customer service, or product performance. Knowing these needs will guide the configuration of AI systems, ensuring they align with performance standards.
Next, categorize the identified criteria into primary and secondary levels. Primary criteria might include core objectives such as issue identification and process resolution, while secondary criteria encompass more nuanced elements, like customer education and case management. This structured approach will not only enhance the effectiveness of AI-driven QA assignment but also ensure a tailored analysis that meets the distinct needs of your organization. By laying a strong foundation of QA requirements, you set the stage for successful implementation and adoption of AI technologies.
Step 2: Choose the Right AI Tools
Selecting the appropriate AI tools is crucial for effective automating QA scorecard assignment. Each tool boasts unique features, so it’s important to align your choice with your organization's specific needs. Consider factors such as user-friendliness, integration capabilities, and scalability when evaluating options. A tool with strong analytical capabilities can ensure accurate assessments, while one that offers robust reporting features can provide valuable insights for continuous improvement.
Further, look for tools that support customization. The ability to tailor the AI to your specific QA standards enhances its effectiveness. After narrowing down your choices, consider conducting trial runs to see how well each tool performs in practice. This hands-on experience can provide insight into which AI-driven QA assignment tool truly meets your requirements. Ultimately, the right choice will empower your team to streamline processes and elevate quality assurance efforts.
Step 3: Develop and Train AI Models
Developing and training AI models is a crucial step in automating QA scorecard assignments. In this phase, it’s essential to gather historical data on quality assurance evaluations. This data will serve as the foundation for training the AI models effectively, allowing them to recognize patterns and correlations within the QA processes. The models should be tailored to identify key performance indicators that align with your organization's quality assurance criteria.
Next, focus on refining the AI algorithms through iterative training cycles. By continuously testing the models with new data, you enhance their accuracy and reliability. In the context of AI-driven QA assignment, incorporating feedback from QA specialists during this process is invaluable. Their insights can help fine-tune the AI's decision-making capabilities, ultimately leading to a more effective system that enhances overall quality assurance performance. In summary, a robust model development process is key to achieving successful automation in QA assignments.
Step 4: Deploy and Monitor the System
Deploying and monitoring the AI-driven QA assignment system is a vital step in ensuring its effectiveness. After implementing the system, it is essential to create a deployment strategy that fits organizational needs. This could involve establishing specific metrics for evaluating performance, such as accuracy rates and feedback loops from users. A clear communication plan should also be in place, enabling team members to understand how to use the system effectively and report any issues they encounter.
Once the system is live, continuous monitoring is critical. Regularly collect data on performance and utilize this information to make adjustments as needed. Conduct periodic reviews to assess how the AI is performing against expected benchmarks. Actively engaging with users will also contribute valuable insights, enabling the team to refine the approach and enhance the overall effectiveness of the AI-driven QA assignment process. Adapting to feedback fosters a culture of continuous improvement, which is essential for long-term success.
Tools for AI-Driven QA Assignment
Automating QA scorecard assignment using artificial intelligence can significantly streamline the evaluation process. The tools designed for AI-driven QA assignment play a critical role in enhancing efficiency and accuracy. These tools enable organizations to assess quality assurance metrics by automating the evaluation of calls and interactions based on designated criteria.
Firstly, industry-leading solutions often provide features such as transcript analysis and real-time reporting. These capabilities allow users to quickly identify strengths and weaknesses in customer interactions. Secondly, customizable templates enable teams to establish specific scoring criteria, ensuring compliance and relevance to organizational standards. Finally, by integrating AI-driven systems within existing workflows, companies can continuously monitor quality metrics, making ongoing improvements more manageable. Embracing these tools can transform how organizations approach quality assurance processes for the better.
insight7
As organizations strive for efficiency, AI-Driven QA Assignment has emerged as a pivotal solution in the quality assurance landscape. By automating the assignment of scorecards, businesses can quickly adapt to ever-changing demands, reducing the reliance on manual processes. The journey begins with identifying specific QA needs, allowing for a more tailored approach to integrating AI into existing frameworks.
To fully realize the potential of AI in quality assurance, there are several key steps to consider. First, it's essential to assess the unique requirements of your QA process. Next, selecting appropriate AI tools that align with these needs ensures a seamless transition. This is followed by developing and training AI models that accurately reflect your quality standards. Finally, deploying and continuously monitoring the system is crucial to ensure consistent performance and adaptability. Embracing this AI-driven approach not only enhances efficiency but also drives insightful analysis, fostering a culture of continuous improvement.
Tool 2: [Other AI Tool Name]
The integration of AI-Driven QA Assignment introduces a new level of efficiency in quality assurance processes. By utilizing advanced algorithms and data analytics, organizations can automate the assignment of scorecards in real-time, allocating resources effectively. This tool not only streamlines the workflow but also enhances accuracy, providing detailed insights into individual performances and compliance ratings.
One of the standout features of this tool is its capacity for customization. Users can adapt the AI-driven system to meet their specific compliance needs. This flexibility allows QA teams to generate editable reports, ensuring that the final outputs align closely with organizational standards. Furthermore, as data is collected, the AI continually learns and improves its assessments, becoming more precise over time. With its user-friendly interface and quick turnaround, AI-Driven QA Assignment makes it easier than ever to maintain quality standards while saving time.
Tool 3: [Other AI Tool Name]
Tool 3: [Other AI Tool Name]
The selection of an AI-powered tool is crucial for optimizing QA scorecard assignments. Automating this process using the right technology enhances efficiency and accuracy in evaluation. [Other AI Tool Name] stands out by offering advanced features that streamline the assignment of quality assurance metrics. This tool can analyze conversations for key performance indicators, ensuring that every interaction is scored against established benchmarks.
[Other AI Tool Name] works seamlessly by integrating with existing systems. It allows managers to monitor quality in real time, providing immediate feedback to agents. Layered with customizable options, this tool can tailor reports to match specific compliance needs while enabling modifications for personalized client interactions. By adopting [Other AI Tool Name], organizations can expect not only increased productivity but also a higher level of insight into their team's performance, making AI-Driven QA Assignment an indispensable asset for any organization aiming to boost quality standards.Tool 4: [Other AI Tool Name]
In your journey towards automating QA scorecard assignment, Tool 4 introduces an innovative AI solution designed to enhance efficiency and accuracy. This tool streamlines the process by intelligently analyzing call data and generating customized reports in minutes. Upon processing, users can easily download and review comprehensive performance insights, making it easier to identify areas for improvement.
What sets this tool apart is its adaptability to your specific compliance needs. Users can not only receive formatted documents but also edit them for personalized feedback. This level of customization ensures that compliance officers can provide tailored evaluation reports to clients. By embracing this AI-driven QA assignment tool, you can significantly reduce manual effort and improve overall quality assurance processes within your organization.
AI-Driven QA Assignment is transforming the way organizations approach quality assurance. Traditionally, this process has relied on manual assessments, which can be time-consuming and prone to human error. With AI, automated systems can analyze quality metrics swiftly and accurately, ensuring consistency in evaluations. This advancement not only reduces the workload of QA teams but also enhances the quality of insights derived from the data.
To implement AI-driven QA assignments effectively, organizations should follow specific steps. Firstly, identifying the QA requirements is essential to set clear goals. Secondly, selecting the right AI tools that align with organizational needs can make a significant difference. Thirdly, developing and training AI models ensures they are tailored to the unique challenges posed in quality assurance. Finally, deploying and continuously monitoring the AI system allows for adjustments based on performance and feedback. By adhering to these steps, organizations can harness the full potential of AI-driven quality assurance in their operations.
Conclusion: Embracing the Future with AI-Driven QA Assignment
As we conclude our exploration of AI-Driven QA Assignment, it's clear that this innovation marks a significant shift in quality assurance practices. By automating scorecard assignments, organizations can significantly reduce time spent on manual evaluations, enhancing efficiency and accuracy. This capability not only streamlines the review process but also empowers teams to focus on insights and continuous improvement.
Embracing AI-driven solutions positions businesses to adapt to a rapidly changing landscape. The future of QA promises data-driven insights and automated reporting, allowing for more strategic decision-making. By integrating AI, organizations can enhance their quality assurance frameworks, ensuring they remain competitive and responsive to customer needs. Ultimately, the journey toward intelligent QA practices is an exciting opportunity that promises to elevate standards across industries.
Analyze qualitative data. At Scale.
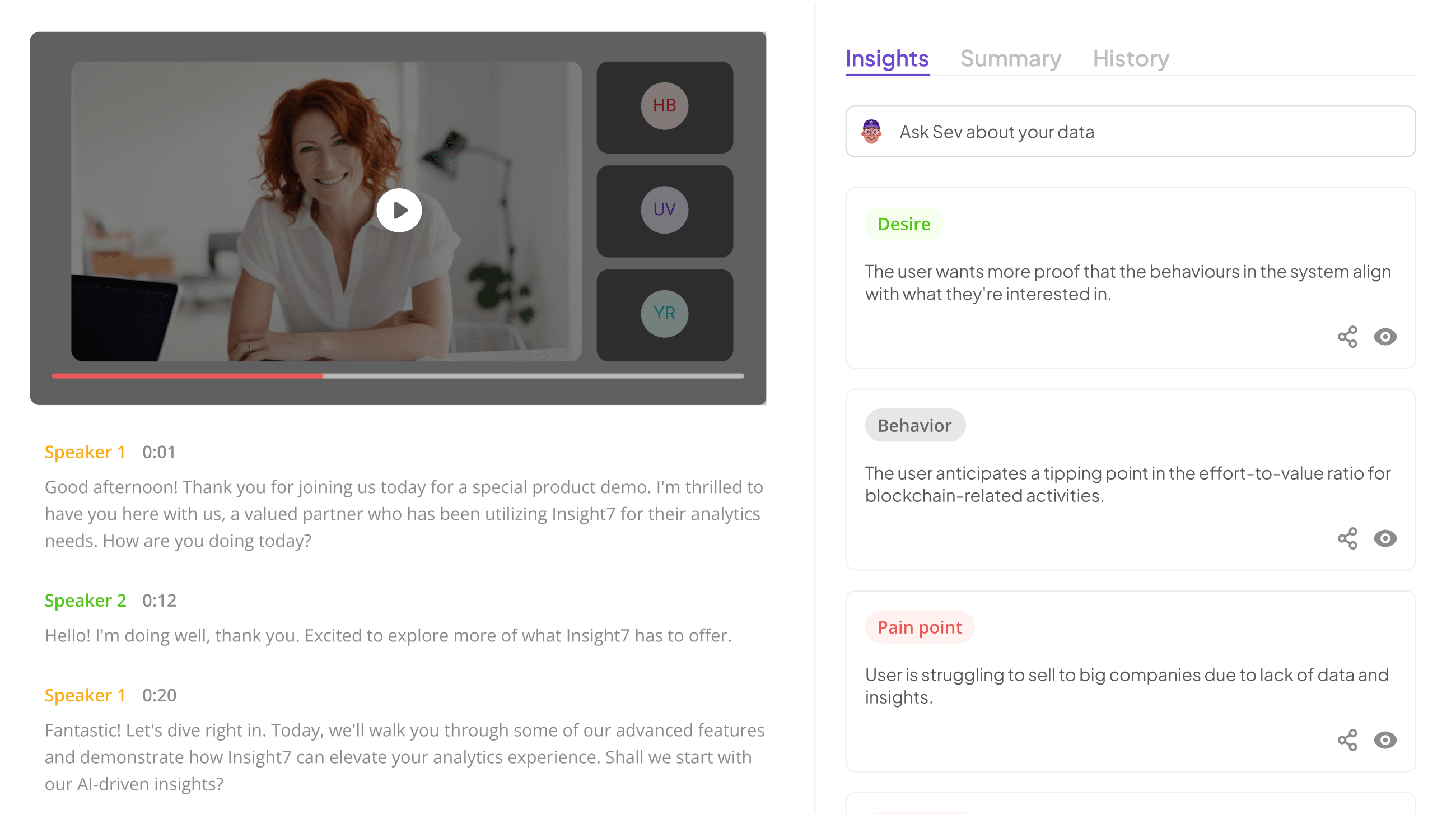