AI vs Workflow Automation: Which QA Software Capability Matters More?
-
Hello Insight
- 10 min read
In the ever-evolving landscape of quality assurance (QA), professionals often grapple with the distinction between AI vs Automation. Both technologies promise enhanced efficiency and accuracy, yet their implications for QA software capabilities run deep. As organizations strive for excellence in quality control, understanding these differences is essential to making informed tool choices.
AI focuses on intelligent decision-making and predictive analytics, enabling smarter testing processes. In contrast, automation emphasizes systematic task execution, streamlining repetitive workflows to save time. Each capability holds unique advantages, so identifying which aligns with your specific QA objectives can shape your approach and ultimately, success in your quality assurance efforts.
Analyze & Evaluate Calls. At Scale.
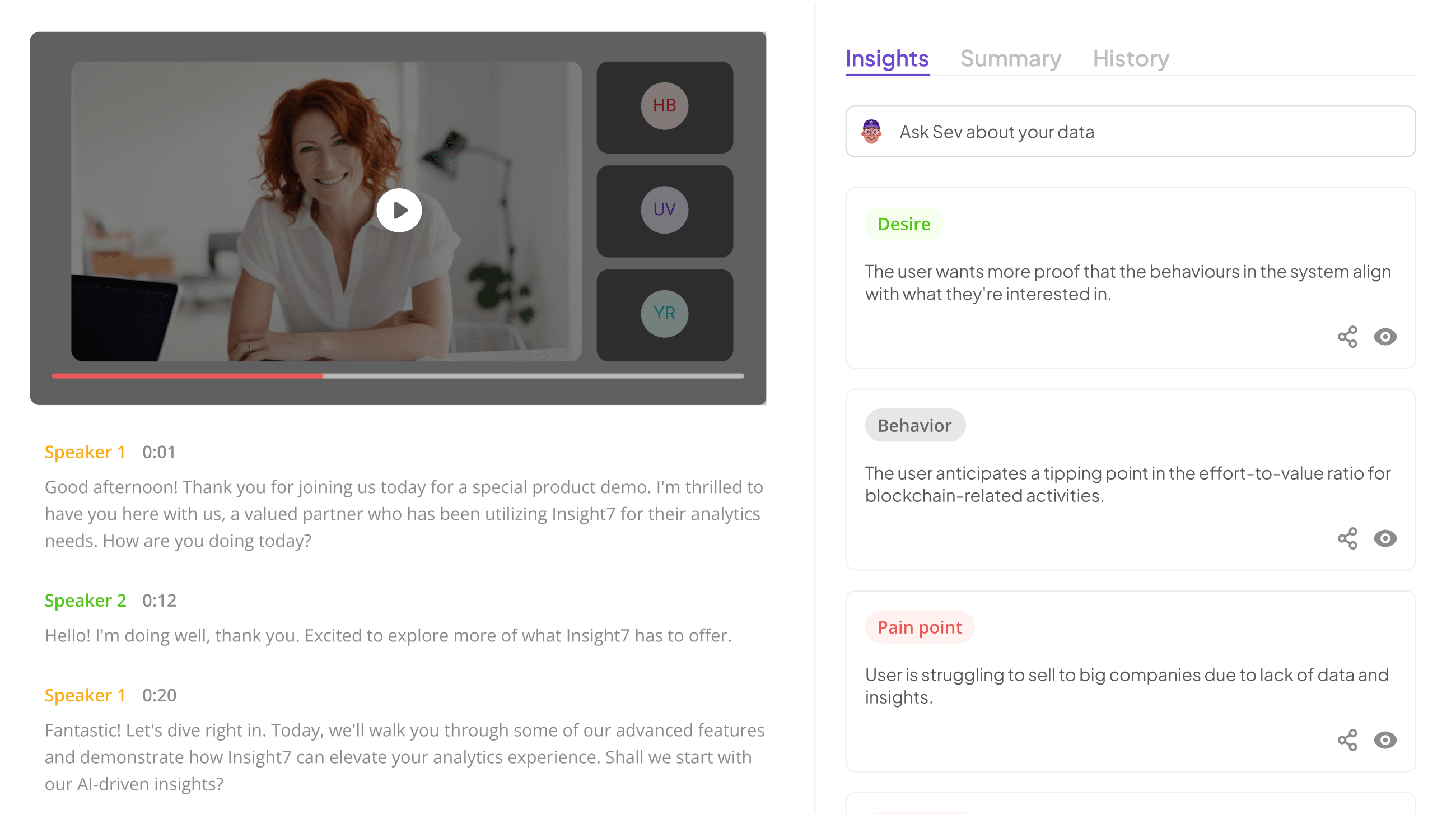
Understanding the Landscape
To navigate the debate of AI vs Automation, it's essential to recognize that each offers distinct benefits within Quality Assurance (QA) software capabilities. AI excels at analyzing large datasets swiftly, providing insights that inform better decision-making. Automation, on the other hand, focuses on streamlining repetitive tasks, enhancing operational efficiency. The choice between these two often hinges on the specific needs of a business, as both technologies can complement each other effectively.
Understanding the core attributes of both AI and Automation is critical for organizations aiming to improve their QA processes. AI can drive deeper customer insights through sophisticated data analysis, while Automation facilitates quicker task completion, reducing the burden on QA teams. By assessing your operational objectives and aligning them with these capabilities, you can create a tailored approach that leverages the strengths of both technologies to achieve optimal results.
Key Differences: AI vs Automation in QA
When exploring key differences between AI and automation in QA, it's essential to understand their distinct roles and capabilities. AI technologies focus on mimicking human intelligence, enabling systems to learn from data, identify patterns, and provide insights that improve overall quality assurance processes. In contrast, automation aims to streamline repetitive tasks, enhancing efficiency without the need for human input or decision-making.
AI excels in contexts requiring adaptability and complex decision-making, such as analyzing customer feedback or predicting potential issues. Automation shines in executing routine tasks, such as test scripts or data validation, which can be performed consistently at scale. Both AI and automation hold value in QA, yet their applicability depends on specific project needs. Choosing the appropriate approach is vital for ensuring quality while maximizing resource utilization. Understanding these differences can guide teams in effectively integrating AI and automation into their QA workflows.
The Role of AI in QA Software
AI plays a transformative role in quality assurance software, fundamentally reshaping testing processes. When considering AI vs automation, it becomes evident that AI enhances the capability of traditional QA tools significantly. Machine learning algorithms analyze vast amounts of data, identifying patterns and predicting potential defects. This predictive ability helps teams make informed decisions and address issues proactively rather than reactively.
Furthermore, AI improves efficiency by automating repetitive testing tasks, freeing up QA professionals to focus on more complex problem-solving. It also tailors testing approaches based on historical data, enhancing the accuracy of outcomes. By integrating AI into their workflows, organizations can achieve a higher level of quality in their products while reducing time and costs. In contrast to traditional workflow automation, AI brings an added layer of intelligence that enhances the overall effectiveness of quality assurance processes.
AI vs Automation: How AI Enhances QA Testing
AI vs Automation plays a crucial role in enhancing QA testing, with AI introducing transformative capabilities that surpass traditional automation. While automation focuses on executing repetitive tasks, AI enhances these processes by incorporating intelligent decision-making and learning from historical data. This synergy allows for more sophisticated test scenarios, significantly improving the accuracy and efficiency of QA processes.
One major advantage of AI is its ability to analyze vast amounts of test data, identifying patterns and anomalies that might go unnoticed by standard automation. Additionally, AI-driven tools can adapt to changing requirements, minimizing the need for constant manual adjustments. This dynamic capability not only accelerates testing cycles but also leads to more reliable test outcomes. In essence, the integration of AI in QA testing marks a significant advancement beyond what automation alone can achieve, prompting teams to reconsider their strategies for effective quality assurance.
Benefits of AI-Driven QA Tools
AI-driven QA tools provide significant advantages that can enhance quality assurance processes. Unlike traditional automation methods, these tools utilize machine learning and natural language processing to analyze data and identify issues with greater accuracy. This proactive approach enables QA teams to spot potential problems early in the development cycle, reducing costly fixes later.
One major benefit of AI-driven QA tools is their ability to handle vast amounts of data effortlessly. With their analytical capabilities, these tools can generate valuable insights from transcripts of customer interactions, ensuring compliance and improving customer engagement. Furthermore, AI can automate repetitive tasks while learning from past performance, resulting in continuous process improvement. By integrating AI into QA, organizations can make more informed decisions while streamlining workflows, ensuring a smoother balance between AI vs automation in achieving superior quality outcomes.
Extract insights from interviews, calls, surveys and reviews for insights in minutes
Workflow Automations Value in QA
Workflow automations have significant value in Quality Assurance (QA) by streamlining processes and enhancing efficiency. Automating routine tasks allows QA teams to focus on more complex issues. This shift not only saves time but also reduces the potential for human error, leading to more reliable outcomes. Additionally, workflow automation can help create consistency in QA processes, ensuring that all necessary steps are followed without deviation.
Moreover, when integrated effectively, workflow automation interoperates with AI tools. This combination allows for a more comprehensive approach to testing, as AI can analyze large datasets while automation handles repetitive tasks. The balance of AI vs automation in QA is crucial; each has its strengths and can work together to maximize the overall effectiveness of quality assurance efforts. With thoughtful implementation, organizations can harness these technologies to improve product quality and enhance user satisfaction.
AI vs Automation: Leveraging Automation for Efficiency
In the ongoing discussion of AI vs Automation within QA software capabilities, it's crucial to recognize how each contributes to efficiency. Automation streamlines repetitive tasks, reducing human error and freeing up valuable time for QA teams. Processes such as testing, monitoring, and reporting benefit significantly from the systematic nature of automation. This not only accelerates operations but also enhances accuracy, creating a more dependable workflow.
Meanwhile, while automation handles routine functions, AI adds an analytical layer that can identify patterns and make predictions. For teams aiming to improve overall efficiency, a blend of both technologies holds the most promise. By employing targeted automation processes alongside AI's advanced analytics, organizations can achieve a higher caliber of quality assurance. Adopting this dual approach ensures that the benefits of AI vs Automation are maximized within QA practices.
Benefits of Workflow Automation Tools
Workflow automation tools offer significant advantages in streamlining processes within quality assurance (QA) environments. Firstly, they enhance efficiency by automating repetitive tasks, allowing teams to focus on higher-value activities. Rather than manually analyzing data or generating reports, automation tools expedite these processes, leading to quicker decision-making and optimal resource utilization.
Additionally, workflow automation promotes consistency and accuracy in QA processes. Automated workflows reduce the likelihood of human error, leading to higher quality outputs. In terms of collaboration, these tools allow team members to access insights and project updates in real-time, facilitating better communication and teamwork. By integrating automation systems within QA frameworks, companies can better understand the nuances of AI vs automation, ultimately enhancing their quality assurance capabilities. As organizations seek to improve their operational effectiveness, harnessing the benefits of workflow automation becomes increasingly vital.
Selecting the Right Tools: Balancing AI and Automation
Selecting the right tools in the realm of QA software hinges on a careful assessment of both AI and automation. Understanding how to strike the right balance between AI capabilities and workflow automation can significantly impact the overall testing process. AI excels at pattern recognition and predictive analysis, while automation enhances speed and accuracy in repetitive tasks.
To effectively balance these two forces, consider the following steps: First, identify your specific QA needs to determine where improvement is essential. Next, evaluate the AI and automation capabilities of potential tools, ensuring they align with your goals. This dual-focused approach helps in selecting a toolset that not only performs efficiently but also integrates adaptive AI features, fostering continuous improvement in quality assurance. A thoughtful selection process can lead to enhanced productivity and a more resilient QA strategy.
Step 1: Identifying Your QA Needs
Identifying your QA needs is a crucial first step in enhancing quality assurance processes. Start by assessing the specific challenges your team faces; these may involve manual testing inefficiencies or inconsistent output quality. Consider whether your organization prioritizes speed, accuracy, or scalability, and how each factor relates to AI vs workflow automation capabilities. By understanding these elements, you can tailor your QA strategy to address the most pressing issues effectively.
Next, gather input from your QA team and other stakeholders. Their insights will help illuminate the specific functionalities that are needed in a QA tool. Evaluate whether an AI-driven solution will best address complex testing scenarios or whether a workflow automation tool would streamline repetitive tasks. This step sets the foundation for making informed decisions on QA software that aligns with your team's unique needs and objectives.
Step 2: Evaluating AI and Automation Capabilities
To evaluate AI and automation capabilities, it’s essential to assess their specific roles in quality assurance. Begin by understanding how AI can enhance QA processes through advanced analytics and decision-making. AI enables more nuanced data interpretations and predictions, giving teams powerful insights into potential issues before they arise. On the other hand, workflow automation simplifies repetitive tasks, offering efficiency gains and freeing up time for more strategic activities.
Next, consider the integration of both technologies. Some software may excel at AI-driven insights, while others focus on automation efficiencies. An ideal solution often combines the strengths of both, allowing organizations to capitalize on AI's analytical prowess alongside automation's operational reliability. Assess your specific quality assurance objectives to determine which capabilities matter most, thus guiding you in selecting the appropriate tools for your needs. Balancing these capabilities will ultimately lead to more effective and responsive QA practices.
Recommended QA Tools
Selecting the right QA tools is crucial in navigating the debate of AI vs Workflow Automation. Various tools can cater to specific requirements, optimizing the testing process. Some tools effectively integrate AI for enhanced analysis, while others focus on automating repetitive tasks, thereby boosting efficiency.
Firstly, tools like Selenium and TestComplete excel in automating browser interactions, making them ideal for regression testing. QTest provides a comprehensive test management platform with analytics capabilities, crucial for organizations emphasizing both AI and automation. Additionally, Ranorex offers user-friendly automation and is especially effective for teams requiring quick setups. On the AI front, Applitools harnesses visual AI to ensure visual correctness across applications. Each tool serves a unique purpose, enabling teams to balance AI advancements with efficient automation, ultimately ensuring high-quality outputs.
- insight7
In the ongoing conversation about AI vs automation in QA software, understanding the unique contributions of each is essential. AI enhances the capability of QA tools through intelligent data analysis, helping businesses derive meaningful insights from vast amounts of customer feedback. This enables companies to respond more quickly to market demands, refining their strategies for improved customer satisfaction.
On the other hand, workflow automation streamlines processes, reducing manual tasks and ensuring consistency across QA practices. Automated workflows can help teams collaborate more efficiently by centralizing insights and action points. By combining the strengths of AI and automation, organizations can enhance their QA capabilities, allowing them to address customer needs more effectively and stay competitive in a fast-paced environment. Ultimately, the right balance between these technologies will determine which aspects become crucial for success in quality assurance.
- Selenium
Selenium stands out as a prominent tool in the QA software space, particularly in the context of automation. Its ability to facilitate browser-based automation for web applications makes it invaluable for quality assurance teams looking to enhance efficiency. Selenium allows testers to quickly execute automated tests across different web browsers, which is critical in a landscape increasingly influenced by rapid technological advancements.
When comparing AI vs automation, it's essential to recognize that while Selenium excels at process automation, it lacks the intelligent capabilities that AI brings to the table. Traditional workflows may benefit from Selenium’s straightforward automation scripting capabilities, ensuring thorough test coverage. However, integrating AI can further elevate these automation processes by offering insights driven by data analysis and machine learning. This hybrid approach encourages collaboration between Selenium's automation prowess and AI’s analytical strengths, ensuring that quality assurance is both efficient and insightful.
- QTest
When considering advancements in quality assurance (QA), QTest stands out as a pivotal tool that bridges the gap between artificial intelligence (AI) and workflow automation. At its core, QTest facilitates seamless integration of various testing processes, enhancing collaboration among teams while ensuring that workflows remain efficient and organized. This is particularly important in the debate of AI vs automation, as QTest supports both methodologies, allowing teams to benefit from intelligent insights as well as streamlined task management.
In practice, QTest excels in providing real-time analytics and comprehensive reporting, essential for making data-driven decisions. It empowers QA teams to automate routines while utilizing AI capabilities for predictive analysis and defect detection. Hence, understanding QTest as a versatile platform aids organizations in navigating the complex dynamics of AI vs automation, ultimately fostering a more robust and efficient QA process. Balancing these elements can drive quality improvements and optimize entire workflows.
- TestComplete
TestComplete stands out as a powerful tool in the ongoing discussion of AI vs Automation within QA software capabilities. It combines both automated testing and intelligent features, offering users a flexible framework for their testing requirements. TestComplete enables teams to create, manage, and execute automated tests across various platforms with ease, enhancing overall productivity.
The platform's emphasis on usability is vital, as manual testers can quickly adapt its functionalities without extensive programming knowledge. With AI-driven capabilities, TestComplete enhances test execution by identifying patterns and optimizing test coverage, which ultimately leads to more reliable results. This integration signifies a shift toward a more intelligent automation approach, reaffirming the importance of balancing both AI and automation in the quest for efficient quality assurance processes. This balance is crucial for organizations looking to streamline their workflows while ensuring robust software quality.
- Ranorex
In the discussion of AI vs Automation, one tool that stands out is Ranorex, especially in the realm of quality assurance (QA). Ranorex blends powerful automation capabilities with a user-friendly interface, catering to both beginners and experts. It allows teams to easily create automated tests across various platforms, ensuring comprehensive coverage of their applications without extensive coding knowledge.
Ranorex's approach to automation emphasizes efficiency and reliability. Users can generate detailed reports and analyze test results, which is essential for informed decision-making. The seamless integration with CI/CD pipelines enhances its appeal in a fast-paced development environment. Therefore, as teams weigh the benefits of AI vs Automation, Ranorex exemplifies how effective automation can streamline QA processes, reducing the time and effort needed to ensure software quality. In this context, organizations can thoughtfully evaluate the extent to which automation may complement their testing strategies.
- Applitools
In the ongoing debate of AI vs Automation in QA software capabilities, one solution stands out due to its unique approach to visual testing. This tool excels in ensuring that user interfaces remain consistent across various platforms, thereby enhancing overall quality assurance efforts. Its visual validation techniques allow teams to quickly identify discrepancies during the development process, preventing issues before they escalate.
This software leverages automated visual testing powered by AI, leading to significant efficiency gains in QA workflows. By comparing screenshots from different versions of the application, it ensures that updates do not negatively impact the user experience. In a landscape where precision and speed are crucial, combining AI-driven insights with workflow automation provides a powerful advantage, facilitating effective quality assurance processes that align with user expectations.
Conclusion: AI vs Automation – What Really Matters in QA?
In assessing the merits of AI vs Automation in QA, it becomes clear that each serves distinct yet complementary roles. AI tackles complex tasks such as contextual understanding and pattern recognition, enhancing the quality of insights gained from testing data. Automation, on the other hand, focuses on streamlining repetitive processes, enhancing operational efficiency and productivity.
Ultimately, the choice between AI vs Automation hinges on specific project requirements and goals. Organizations must weigh the benefits of intelligent analysis against the need for efficient workflow management. Finding the right balance ensures a robust quality assurance approach that leverages the strengths of both capabilities effectively.