Objective-Driven Auto-Tagging transforms how researchers analyze qualitative data, addressing common challenges in manual assessment. Traditionally, researchers sift through extensive interview transcripts, leading to inconsistencies and time inefficiencies. The introduction of AI tools that auto-tag research interviews by objectives promises to streamline this process significantly, offering precise tagging that aligns closely with research goals.
By employing AI-driven solutions, researchers can maintain focus and clarity in their analysis. These tools utilize sophisticated algorithms to identify and tag crucial segments of interaction based on predefined objectives. This heightens both the accuracy and relevancy of insights drawn from interviews, empowering teams to extract actionable information quickly and efficiently.
Analyze qualitative data. At Scale.
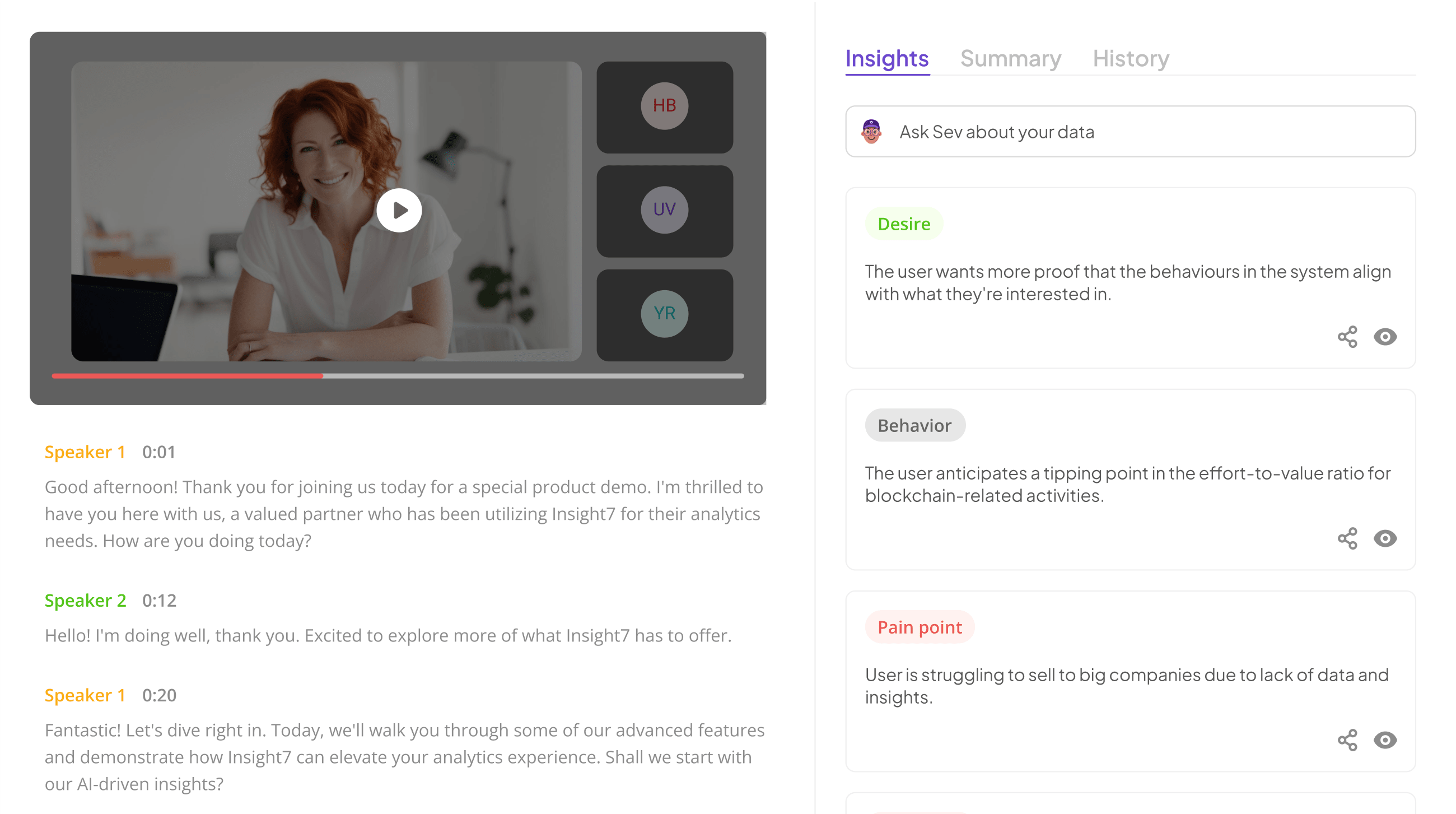
Understanding Objective-Driven Auto-Tagging
Objective-Driven Auto-Tagging serves as a bridge between research objectives and the actionable insights derived from qualitative data. This process emphasizes the alignment of tagging methods with specific research goals, ensuring that insights remain relevant and focused. When researchers know what they want to achieve, auto-tagging tools can effectively categorize and extract pertinent information, promoting consistency throughout the analysis. By staying true to these objectives, researchers can unlock deeper insights and streamline the interpretation of their findings.
Moreover, the mechanisms underpinning Objective-Driven Auto-Tagging are fascinating. These tools utilize advanced algorithms that analyze interview transcripts, identifying key segments that relate to predefined research criteria. This automated approach significantly reduces manual effort, allowing researchers to concentrate on deriving insights. Ultimately, understanding how Objective-Driven Auto-Tagging functions not only enhances research efficiency but also optimizes the overall user experience in qualitative analysis.
The Role of Objective-Driven Auto-Tagging in Research
Objective-Driven Auto-Tagging transforms how researchers analyze qualitative data by systematically assigning tags that align with specific research objectives. This targeted approach not only streamlines the analysis process but also enhances the consistency of insights derived from interviews. When researchers utilize auto-tagging tools, they can efficiently categorize responses and pinpoint critical themes that directly contribute to their project's goals.
In addition to improving efficiency, Objective-Driven Auto-Tagging maintains a clear focus on the core research objectives, which minimizes bias during analysis. Traditional methods may introduce inconsistencies when multiple researchers interpret data differently. However, by implementing an objective-driven tagging framework, teams can achieve reliable and actionable insights faster. This approach empowers researchers to respond swiftly to client demands for insights while ensuring that their findings remain aligned with their initial research goals. Overall, it enables a more structured and coherent analysis, fostering better decision-making based on solid evidence.
- Explanation of how auto-tagging aligns with research objectives.
Objective-Driven Auto-Tagging enhances the alignment between research interviews and specific research goals. By categorizing insights based on predefined objectives, it streamlines the analysis process and ensures that relevant data is easily accessible. When researchers define their goals—be it improving member experiences or identifying challenges—the auto-tagging tools pull related insights that directly correspond to these objectives. This method not only organizes data efficiently but also enhances the quality of findings.
Additionally, the use of auto-tagging saves valuable time for researchers. Instead of sifting through all the data manually, researchers can rely on the system to pinpoint relevant segments. As insights are clustered into themes—or "buckets"—it becomes easier to derive conclusions. Each theme is accompanied by sentiment analysis, allowing researchers to understand participant perspectives more profoundly. Overall, Objective-Driven Auto-Tagging provides a powerful mechanism to align qualitative research with actionable insights, effectively bridging the gap between data collection and practical outcomes.
- Importance of maintaining focus and consistency during qualitative analysis.
During qualitative analysis, it is essential to maintain focus and consistency to ensure the reliability of findings. This process helps researchers accurately capture insights aligned with specific research objectives. When qualitative data is analyzed without this consistency, it may yield different interpretations of the same information across team members. This can lead to fragmented insights that do not serve the study’s goals effectively.
Integrating Objective-Driven Auto-Tagging into qualitative research enhances focus and consistency by applying standardized protocols for tagging interviews. This automation minimizes the risk of bias and misunderstanding in data interpretation. With clearly defined objectives, AI tools can identify and tag relevant segments seamlessly, allowing researchers to identify themes rapidly. This approach not only saves time but also streamlines the analysis process, delivering accurate and actionable insights more efficiently. Thus, maintaining focus and consistency becomes crucial to deriving meaningful conclusions from qualitative data.
Mechanisms of Objective-Driven Auto-Tagging Tools
Objective-Driven Auto-Tagging tools employ sophisticated algorithms to ensure that research interviews are categorized according to specific research goals. These tools utilize natural language processing techniques, which enable them to analyze conversational data and automatically assign relevant tags. As segments of interviews are processed, the system evaluates keywords, sentiment, and themes that align with predefined objectives, facilitating a more focused analysis.
The technology behind Objective-Driven Auto-Tagging relies on machine learning models that continuously improve as they encounter more data. By integrating user-defined tags and contexts, the system becomes adept at recognizing nuance in discussions. This approach not only streamlines the analytical process but also enhances the depth of insights derived from the interviews. Consequently, researchers can easily navigate vast amounts of data, quickly identifying critical patterns that support their objectives, and thereby maximizing the value derived from their research efforts.
- How AI discerns and tags segments by objective.
AI systems effectively employ advanced algorithms to discern and tag interview segments based on specific research objectives. By utilizing natural language processing (NLP), these tools analyze spoken or written language to identify relevant themes. This process ensures that the insights derived from qualitative data align closely with the predefined objectives of the research. Hence, AI can swiftly organize the data, making it easier for researchers to navigate through vast amounts of information.
The mechanisms of objective-driven auto-tagging rely on contextual understanding and semantic analysis. AI tools assess the intention behind conversation segments, not just their keywords, to achieve accurate tagging. This capability allows researchers to filter insights by specific topics, such as process management, ensuring the findings remain focused and relevant. By automating this process, AI enhances the efficiency and reliability of qualitative analysis, ultimately leading to actionable insights tailored to the research goals.
- The algorithms behind accurate tagging based on pre-defined criteria.
Accurate tagging is crucial for effective qualitative analysis, especially in research interviews. The algorithms behind accurate tagging are designed to enhance the efficiency of the Objective-Driven Auto-Tagging process. These algorithms utilize natural language processing (NLP) to identify key themes and segments within the conversation. By recognizing patterns in the data, they ensure that the relevant information is tagged according to pre-defined criteria that align with the research objectives.
In this context, machine learning models are trained on vast datasets to refine their understanding of language nuances. As a result, they can effectively differentiate between concepts, ensuring precise tagging. This capability not only improves consistency but also minimizes the risk of bias and misinterpretation. Ultimately, robust algorithms empower researchers by transforming raw interview data into structured insights, paving the way for informed decision-making and strategic outcomes in their projects.
Extract insights from interviews, calls, surveys and reviews for insights in minutes
Top AI Tools for Objective-Driven Auto-Tagging
Objective-Driven Auto-Tagging is transforming the way researchers analyze qualitative data by streamlining the tagging process. Utilizing advanced AI algorithms, various tools can automatically segment and tag interview transcripts based on predefined research objectives. This ensures that insights are not only consistent but also aligned closely with the goals of the research, alleviating the burdens of manual analysis.
Several powerful tools are available to facilitate objective-driven auto-tagging. For instance, Quirkos offers an intuitive interface designed for thematic analysis, making it easier to classify qualitative data efficiently. Similarly, Dovetail stands out for its user-friendly approach while maintaining robust auto-tagging features that cater to diverse research needs. Auris AI is particularly noteworthy for its multilingual support, adapting to various languages and cultures. Meanwhile, NVivo integrates traditional qualitative methods with modern AI capabilities, enhancing the durability of the analysis. Lastly, Atlas.ti provides comprehensive data management, ensuring researchers have advanced tagging options at their fingertips. By employing these tools, research teams can generate faster, more accurate insights, directly responding to the growing demand for timely reporting.
insight7: Leading the Way in Auto-Tagging
Objective-Driven Auto-Tagging is revolutionizing the way researchers manage insights from interviews. It streamlines the initial stages of qualitative analysis by automating the tagging process, which aligns closely with specific research objectives. This innovation not only enhances efficiency but also ensures that researchers maintain focus on the queries they need to address. By minimizing time spent on sorting through transcripts, investigators can dedicate their efforts to deeper analysis and interpretation of data.
The mechanisms behind Objective-Driven Auto-Tagging rely on sophisticated algorithms capable of discerning content relevance based on predefined criteria. These AI-driven tools intelligently identify and label key segments, making it easier for researchers to extract meaningful insights. The user feedback surrounding these tools highlights increased productivity and improved accuracy in research outputs, making them indispensable in modern research environments. By integrating effective auto-tagging solutions, researchers can navigate complex data landscapes with greater ease and precision.
- Unique features of insight7 that facilitate objective-driven tagging.
The unique features of this auto-tagging tool make it stand out in enabling effective and accurate data analysis. One key aspect is its native transcription capability, which simplifies data ingestion. Users can transcribe audio and video files directly into their projects, thus ensuring seamless integration of insights without needing external software. This enhances the workflow and keeps all analysis tools in one place.
Furthermore, this platform employs advanced algorithms to analyze the transcribed text and identify themes relevant to specific research objectives. These algorithms are designed to discern context, allowing for precise tagging based on predefined criteria. This means researchers can easily extract meaningful insights that align directly with their objectives, enabling them to maintain focus and consistency throughout their qualitative analysis. The user-friendly interface ensures that these features are accessible, even for those less familiar with data analysis tools.
- User feedback and case studies showcasing its efficiency.
User feedback reveals that many researchers find remarkable efficiency in Objective-Driven Auto-Tagging tools. Participants in various case studies noted significant improvements in the speed and accuracy of their data analysis processes. For example, one organization reported they reduced their analysis time by over 50% when using these tools. By leveraging AI technology, users can automatically categorize insights based on specific research objectives, enabling them to focus on strategic decision-making.
In another case study, a B2B SaaS client highlighted how auto-tagging allowed them to aggregate qualitative data effectively. They expressed their satisfaction with the clarity gained through auto-tagging, leading to enhanced insights into customer perspectives. These experiences illustrate the transformative potential of Objective-Driven Auto-Tagging, showcasing its effectiveness in streamlining the research process and fostering actionable outcomes. As the landscape of research evolves, these tools emerge as vital assets for enhancing efficiency and depth in qualitative analysis.
Additional Tools Revolutionizing Research Interviews
The rise of new tools is transforming research interviews, enabling more efficient data collection and analysis. Researchers no longer navigate lengthy transcripts manually. Instead, innovative software actively tags conversations by research objectives, facilitating focused analysis. This paradigm shift enhances the speed and accuracy of insights, streamlining the decision-making process.
Several noteworthy applications are leading this revolution. Quirkos stands out by simplifying thematic analysis, effectively categorizing responses based on objectives. Dovetail merges user-friendliness with powerful auto-tagging properties, making it accessible for researchers across experience levels. Auris AI showcases impressive multilingual capabilities, adapting to diverse research environments. Meanwhile, NVivo integrates traditional qualitative methodologies with contemporary auto-tagging, ensuring comprehensive analysis. Lastly, Atlas.ti excels in data management while offering advanced tagging features. Together, these tools exemplify how objective-driven auto-tagging is reshaping qualitative research, ushering in a more efficient era for researchers.
- Quirkos: Simplifying thematic analysis with objective tagging capabilities.
The process of thematic analysis can often feel overwhelming, particularly when sifting through large volumes of qualitative data. Quirkos transforms this experience by integrating objective-driven auto-tagging capabilities that streamline the entire analysis process. When researchers input their objectives, the tool systematically categorizes and tags responses based on these predefined goals. This not only saves time but also ensures that the insights generated are highly relevant to the specific research questions at hand.
Through intuitive clustering of data into themes, Quirkos allows researchers to visualize insights effectively. For instance, researchers can tag themes such as "collaboration" or "member experience" while receiving sentiment analysis alongside. Each tag can be expanded to reveal direct quotes and references, supporting transparency and traceability in the analysis. This structured tagging approach highlights how objective-driven auto-tagging enhances the clarity and focus of qualitative research, ultimately leading to deeper insights and more impactful findings.
- Dovetail: Balancing user-friendliness with robust auto-tagging features.
In the realm of qualitative research, the challenge lies in balancing user-friendliness with advanced features, specifically when it comes to robust auto-tagging systems. Effective auto-tagging enhances the experience by allowing researchers to focus on their objectives rather than getting lost in labor-intensive categorization. This alignment makes Objective-Driven Auto-Tagging essential, streamlining the qualitative analysis process through clear, structured tagging.
To achieve this balance, several key principles come into play. First, user interface design must prioritize intuitive navigation, allowing users to easily harness complex functionalities. Second, the underlying algorithms must consistently deliver accurate tags that are directly related to research objectives. These features together ensure that researchers can trust the system, making it both powerful and accessible. As a result, users can efficiently analyze interviews, transforming insights into actionable findings without compromising ease of use.
- Auris AI: Notable for multilingual support and adaptability.
In the realm of research interviews, adaptability is key to gaining valuable insights. Notably, one tool stands out for its multilingual support and versatility, enabling users to auto-tag data according to specific research objectives. This capability significantly enhances the analysis process, allowing researchers to focus on deriving meaningful conclusions from diverse data sources. Adaptability in this context means the software can operate seamlessly across different languages, ensuring that the nuances of various cultures are respected during analysis.
Moreover, the focus on auto-tagging driven by research objectives ensures that relevant themes and topics are accurately captured. This allows researchers to optimize their workflow, making it easier to sift through vast amounts of qualitative data. By integrating intelligence that recognizes patterns and context, the system not only improves efficiency but also fosters nuanced understanding, enabling research teams to respond effectively to their findings and explore new avenues for inquiry.
- NVivo: Combining traditional qualitative analysis with modern AI auto-tagging.
In the evolving realm of qualitative research, NVivo stands out for its capability to blend traditional analysis methods with cutting-edge AI auto-tagging. This integration enables researchers to efficiently connect insights with distinct research objectives, enhancing overall analysis quality. By implementing Objective-Driven Auto-Tagging, users can feel assured that their data is being tagged accurately, aligning with specific study goals and maintaining focused themes throughout the process.
The combination of human expertise with AI technologies ensures that the researcher's input remains vital. While AI can manage repetitive tasks such as tagging, it is crucial for researchers to oversee the quality and fidelity of these tags. This collaboration between traditional qualitative approaches and AI supports researchers in deepening their understanding and engagement with the data, paving the way for impactful insights that can drive decisions and strategic directions in various fields.
- Atlas.ti: Comprehensive data management with advanced tagging options.
Atlas.ti provides a powerful platform for research data management, tailored to meet the specific objectives of qualitative analysis. With its advanced tagging options, researchers can organize and cluster data according to pre-defined themes, allowing for efficient data retrieval and deeper insights. This comprehensive system automates the tagging process, aligning with the research goals and maintaining focus throughout the analysis.
Customized tagging enhances the research experience by enabling users to specify what themes or sentiments are most relevant. For instance, researchers can prioritize insights related to challenges or collaboration, ensuring that the data reflects their immediate objectives. This precision not only saves time but also enriches the analysis, ultimately leading to more actionable outcomes. With these advanced features, Atlas.ti stands out as an essential tool for effective objective-driven auto-tagging in research interviews.
Conclusion: Embracing Objective-Driven Auto-Tagging for Enhanced Research Insights
As research methodologies continue to evolve, embracing objective-driven auto-tagging becomes essential for enhanced insights. This innovative approach empowers researchers to sift through qualitative data with remarkable efficiency, allowing them to pinpoint significant themes tied to their specific objectives. By focusing on these key insights, researchers can present findings more accurately, ensuring their conclusions are robust and actionable.
Moreover, the adaptability of objective-driven auto-tagging tools not only streamlines the analysis process but also increases the overall quality of the insights generated. As AI technologies become integral to research practices, adopting these tools can significantly enhance the clarity and relevance of findings, ultimately leading to more informed decision-making. Emphasizing this strategy will undoubtedly yield richer, more nuanced understanding across varied research contexts.