Bias Detection AI is becoming increasingly essential in the realm of academic and market research. As researchers strive for objectivity and accuracy, the influence of bias can undermine the integrity of their findings. With the advent of advanced AI technologies, researchers have gained powerful tools to identify and mitigate biases present in their studies.
This introduction highlights the importance of Bias Detection AI in improving research quality. By analyzing data patterns and identifying inconsistencies, these AI tools also enhance decision-making processes. Consequently, researchers can achieve more nuanced insights and promote transparency in their work, ultimately benefiting the entire research community.
Analyze & Evaluate Calls. At Scale.
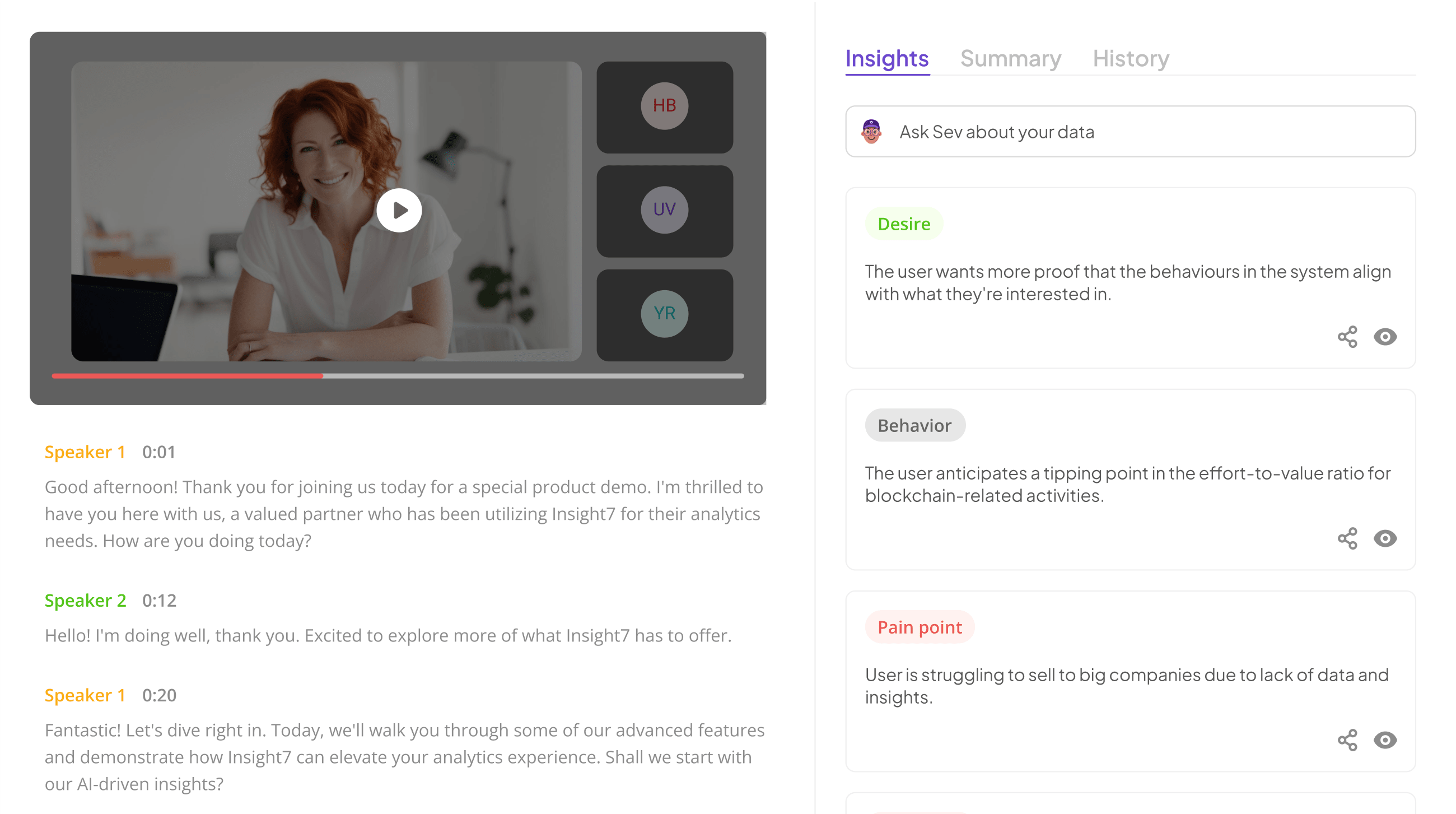
Understanding Bias Detection AI
Bias Detection AI plays a vital role in uncovering hidden biases in research processes. Understanding this technology involves recognizing how it analyzes data patterns to identify potential prejudices that may influence outcomes. These AI systems utilize advanced algorithms to detect inconsistencies and imbalances in datasets, ultimately leading to more objective results.
Variables that Bias Detection AI examines include sample representation, survey design, and respondent demographics. By systematically reviewing these elements, researchers can uncover subtle biases that may skew their findings. The importance of Bias Detection AI cannot be overstated, as it enhances the reliability of research conclusions, promoting fair and equitable outcomes for all stakeholders involved. This understanding empowers researchers to implement strategies that mitigate bias, ensuring their work contributes meaningfully to knowledge and decision-making.
What is Bias Detection AI?
Bias Detection AI refers to innovative technologies designed to identify, analyze, and mitigate biases in research. These systems leverage machine learning algorithms to review vast amounts of data and discern patterns that signal bias. By examining language, data representation, and sampling methods, Bias Detection AI can provide insights into potential skewness in research findings.
In essence, this technology aims to enhance research integrity and transparency. It helps researchers understand where biases may have influenced results, allowing for more accurate conclusions. Moreover, Bias Detection AI empowers researchers to proactively address these issues before publishing findings, ensuring that their work is credible and trustworthy. As research plays a critical role in decision-making across various fields, the implementation of Bias Detection AI is becoming increasingly essential for producing reliable and impactful outcomes.
How Bias Detection AI is Transforming Research
Bias Detection AI is fundamentally changing how research is conducted by identifying and analyzing biases that may affect outcomes. Researchers can now harness these powerful tools to enhance the rigor and reliability of their findings. By systematically detecting biases, these AI systems can reveal unintentional preferences that may skew data interpretation, paving the way for more objective analyses.
This transformation occurs in several ways. First, Bias Detection AI streamlines the process of data evaluation, allowing researchers to focus on meaningful insights rather than sifting through raw data. Second, it increases transparency in research by providing quantitative metrics on biases, which can be shared with stakeholders for validation. Lastly, it empowers researchers to refine methods and improve data collection techniques based on insights derived from AI analysis. Overall, Bias Detection AI signifies a leap toward enhanced integrity and trust in research.
Key AI Tools for Research Bias Detection
Bias Detection AI is an essential component of modern research methodologies, helping to ensure that findings are accurate and representative. Various AI tools have emerged to support researchers in identifying and mitigating bias, which is crucial for maintaining the integrity of data analysis. These tools employ sophisticated algorithms to analyze large datasets, highlighting potential biases that human analysts might overlook.
Several key AI tools stand out in the realm of bias detection. First, Insight7 offers advanced features tailored specifically for identifying biases in qualitative data. Secondly, Tool 2 incorporates machine learning to detect subtle biases in language and sentiment. Tool 3 uses statistical analysis to evaluate dataset representativeness, while Tool 4 specializes in visualizing bias patterns for easier interpretation. By understanding these tools and their functionalities, researchers can select the most suitable options for their specific needs, streamlining the process of detecting and addressing bias in their research projects.
Extract insights from interviews, calls, surveys and reviews for insights in minutes
Top Tools for Detecting Bias in Research
Identifying bias in research has become increasingly important in today's data-driven environment. The use of advanced tools allows researchers to uncover hidden biases, leading to more accurate results and insights. These tools utilize cutting-edge AI to analyze data sets and flag potential biases in methodologies and outcomes.
Several top tools have emerged as leaders in Bias Detection AI. Each tool brings unique capabilities, such as natural language processing for analyzing text or machine learning algorithms for assessing quantitative data. For example, Insight7 offers advanced features that empower researchers to delve deep into their data, pinpointing areas where bias may skew results. Other key tools include platforms specializing in data visualization and sentiment analysis, both vital for understanding user feedback and research trends. Selecting the right tool involves consideration of the specific needs and nuances of your research project, ensuring a robust analysis that upholds scientific integrity.
- Insight7: Advanced Features for Bias Detection
Advanced features for bias detection are essential in today's research environment. With the growth of data collection methods, traditional evaluation techniques often fall short. Advanced AI tools now provide an efficient means to sift through vast amounts of information, identifying biases that may impact research outcomes. These tools actively analyze patterns, revealing insights that are commonly overlooked during manual evaluations.
Key functions of Bias Detection AI include sentiment analysis, identifying linguistic biases, and discovering demographic disparities within data sets. By utilizing natural language processing, these features deliver more accurate insights, ultimately helping researchers make informed decisions. Moreover, automated reports streamline collaboration, allowing teams to focus on implementing findings rather than getting bogged down by data. Such capabilities empower researchers to advance their projects with confidence, ensuring that their conclusions are based on comprehensive and unbiased interpretations of the data.
- Tool 2: [Tool Name] Overview and Features
Tool 2 offers a robust overview of how Bias Detection AI can enhance research methodologies. This tool simplifies the analysis process, making it accessible for users with varying levels of expertise. Its intuitive home interface allows users to begin tasks such as transcribing calls or generating insightful reports without requiring extensive training.
Among its standout features is the comprehensive library, where users can store and analyze multiple files simultaneously. The system skillfully extracts key insights, including pain points and customer desires, facilitating an understanding of biases present in gathered data. Furthermore, users can visualize conversations and access contextual quotes from interviews, strengthening their decision-making processes. Tool 2 is designed to democratize data analysis, helping researchers uncover essential insights and improve their methodologies effectively. This allows for a clearer understanding of biases that may impact research outcomes, ultimately driving better decisions and research integrity.
- Tool 3: [Tool Name] Overview and Features
Tool 3: [Tool Name] Overview and Features
This tool specializes in Bias Detection AI, offering a user-friendly platform that empowers researchers and analysts. It seamlessly integrates with existing workflows, allowing users to start accessing valuable insights without extensive training or technical expertise. The intuitive interface facilitates the analysis of vast amounts of data, making it easier to identify potential biases in research studies.
Key features encompass automated transcription and analysis of interviews or calls, where meaningful patterns and insights are extracted. For instance, users can quickly pinpoint common pain points, desires, and even customer compliments through visual analytics. Furthermore, the tool enables users to group multiple files under projects for broader analysis, enhancing collaborative research efforts. Overall, [Tool Name] promotes a more equitable approach to insight generation, ensuring that the findings derived are as reliable and unbiased as possible.
- Tool 4: [Tool Name] Overview and Features
Tool 4: [Tool Name] Overview and Features
[Tool Name] serves as a pivotal resource in the realm of Bias Detection AI, addressing the crucial need for unbiased insights in research. This tool provides intuitive features designed to simplify the detection of biases within datasets, making it accessible to researchers at all levels. With its user-friendly interface, it allows users to upload their research material quickly and receive actionable insights with minimal effort.Key functionalities of [Tool Name] include the ability to generate detailed reports that pinpoint potential biases across various dimensions. Users can analyze customer feedback and interactions, visualizing key trends and pain points. This comprehensive analysis not only aids in identifying biased narratives but also fosters a more equitable research process. By embracing [Tool Name], researchers can enhance their credibility and trustworthiness through more balanced insights. With constant updates and innovations on the horizon, this tool promises to play an essential role in the future of bias detection in research.
Step-by-Step Guide to Using Bias Detection Tools
Using Bias Detection AI effectively requires a systematic approach to ensure accurate results. The first step is the selection of an appropriate tool. Research the various options available and choose one that aligns with your specific needs and objectives. Each tool may offer distinct features like transcription, analysis, and reporting capabilities, crucial for evaluating data effectively.
Once you have chosen your tool, proceed to configure and set it up. This may involve uploading datasets and customizing templates for evaluation purposes. After setup, run your analysis to identify potential biases in the collected data. Finally, carefully interpret the results, focusing on key themes and discrepancies. This will empower you to address any detected biases, enhancing the overall reliability of your research process. Following these steps will equip you to harness the full potential of bias detection tools effectively.
Step 1: Selection of Appropriate Tool
Selecting the right tool is crucial for effective bias detection in research. The initial step involves identifying your specific research needs and the types of bias that may influence your findings. Bias Detection AI tools come with various features tailored to different contexts, such as qualitative analysis or data-driven assessments. Understanding the functionality that each tool offers will greatly enhance your ability to evaluate and address potential biases.
Next, consider the usability of the tools in question. An ideal Bias Detection AI tool should be intuitive and accessible, enabling users to conduct comprehensive analyses without extensive training. Furthermore, assess the support available from the tool's developers, as ongoing assistance can be invaluable during the research process. Thoroughly evaluating these aspects will empower you to select the most suitable tool for your bias detection needs and enhance the overall integrity of your research outcomes.
Step 2: Configuration and Setup
Setting up Bias Detection AI requires careful configuration to ensure accurate results. First, define your specific research needs and objectives. This clarity will guide the tool selection and setup process. It's essential to determine whether you require automated monthly reports or an interactive evaluation dashboard. An automated system saves time by providing consistent reports, while a dashboard allows for deeper analysis through user interaction.
Once you choose the approach, proceed with data integration. Input the relevant data sources, ensuring they align with your research goals. Customizing the report format according to your preferences can further enhance the usability of your Bias Detection AI. Finally, test the setup using a subset of data before full implementation. This will help identify any adjustments needed for optimal performance, providing you with reliable insights in the long run. Take your time during this step, as a solid configuration lays the groundwork for effective bias detection.
Step 3: Interpreting Results
Interpreting results from your Bias Detection AI analysis is crucial for deriving meaningful insights. Start by reviewing the high-level summaries and key themes that the AI outputs, as these highlight patterns within the data. For example, identifying the frequency of specific pain points helps understand prevalent issues among respondents.
Next, take a close look at the generated reports, which provide detailed insights and quotes aligned with major themes. These reports enable you to visualize customer journeys and highlight critical recommendations for improvement. By systematically analyzing the insights, you're equipped to make informed decisions that mitigate bias in future research efforts. Ultimately, interpreting these results not only clarifies your findings but also enhances the overall reliability and impact of your research outcomes.
Conclusion on Bias Detection AI in Research
Bias Detection AI plays a pivotal role in ensuring the integrity of research outcomes. By identifying and mitigating biases in data collection and analysis, these AI tools foster greater reliability in research findings. This not only enhances the credibility of studies but also supports more equitable decision-making processes across various fields.
As researchers increasingly adopt Bias Detection AI, they can expect improved transparency and accountability in their work. Ultimately, integrating these advanced tools will pave the way for more robust insights, guiding researchers towards making informed conclusions that reflect a comprehensive understanding of their subject matter. Embracing this technology signifies a commitment to advancing research standards and ethical practices.