AI Sampling Methods play a pivotal role in enhancing the efficiency of cluster tools. As industries evolve, the need for effective data analysis becomes more pronounced. Understanding how AI sampling techniques streamline this process can help professionals obtain actionable insights for informed decision-making.
These methods are essential in managing and analyzing vast datasets, allowing users to identify patterns and trends with ease. By employing AI sampling, businesses significantly reduce the time and effort required for data analysis, ultimately leading to more accurate results. As we delve deeper into this topic, we will explore various AI sampling methods and their applications in clustering, enriching your understanding of their transformative impact.
Analyze qualitative data. At Scale.
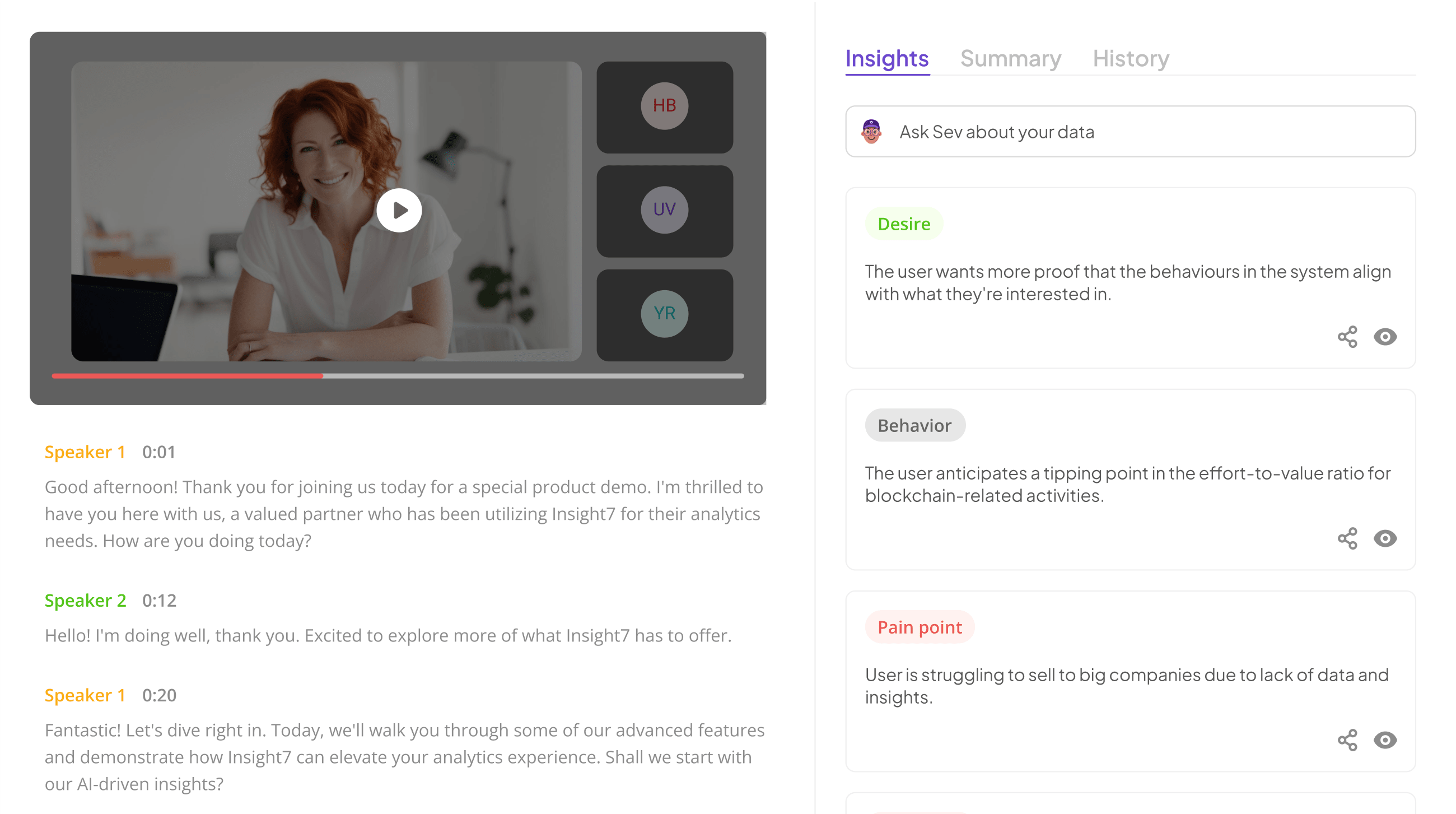
Understanding AI Sampling Methods
AI Sampling Methods play a crucial role in effectively analyzing large datasets, particularly in clustering tasks. These methods help in selecting representative subsets from vast amounts of data, allowing for meaningful insights without processing the entire dataset. Understanding these methods involves knowing how they function and why they are essential. For instance, random sampling may give insights without biases, while stratified sampling ensures vital data segments are adequately represented.
To appreciate the importance of AI Sampling Methods, it is key to recognize how they improve efficiency and accuracy. By systematically selecting samples, they help in identifying patterns and connections within data, leading to better decision-making. Furthermore, these methods can be tailored based on the specific requirements of the clustering task, thus enhancing the overall quality of the analysis. Understanding these methods equips professionals with the tools to harness the power of AI effectively in their projects.
What are AI Sampling Methods?
AI Sampling Methods are essential techniques used to select representative subsets of data for analysis in machine learning and data clustering. These methods help improve the efficiency and accuracy of algorithms by focusing processing power on relevant information, thus reducing the overall data load.
There are several key AI sampling methods commonly utilized in clustering tasks. First, there's random sampling, which selects data points at random, allowing for a straightforward representation of the dataset. Second, stratified sampling involves dividing the data into distinct subgroups and sampling from each one, ensuring that all segments are represented. Third, systematic sampling uses a fixed interval to select items, improving the consistency and structure of the sample.
Each of these methods plays a vital role in ensuring data integrity and reducing bias, leading to more reliable insights in clustering applications. By carefully considering the appropriate sampling method, practitioners can enhance the outcomes of their clustering efforts significantly.
Importance in Clustering Tasks
In clustering tasks, the importance of effective AI Sampling Methods cannot be overstated. These methods play a crucial role in how data sets are grouped and interpreted. By intelligently selecting representative samples, AI sampling enhances the quality of cluster analysis, allowing for deeper insights and better decision-making. This process simplifies the complex world of data, transforming it into actionable information that organizations can leverage for strategic purposes.
There are several key aspects that highlight the importance of AI sampling in clustering. First, it improves computational efficiency by reducing the overall data size while maintaining essential characteristics of the original dataset. Second, it minimizes noise, ensuring that the clusters formed are more reflective of true patterns. Finally, using appropriate AI sampling techniques enables more accurate predictions in machine learning models, which is vital for achieving reliable outcomes. These aspects underline why incorporating AI sampling methods is essential for effective clustering tasks.
AI Sampling Streams in Clustering
AI Sampling Streams in Clustering explores innovative techniques that enhance the analysis of complex data sets. By utilizing AI sampling methods, organizations can efficiently identify patterns and group similar data points, leading to improved insights. These methods reduce the time spent on data processing while ensuring high accuracy in clustering results.
One key aspect of AI sampling streams is their ability to adapt dynamically to varying data volumes. This flexibility allows organizations to process large datasets without sacrificing quality, making it easier to extract valuable insights rapidly. Similarly, these methods enable the combination of various algorithms, such as k-means or hierarchical clustering, to optimize the clustering process further. In essence, AI sampling methods streamline data analysis, fostering a more intelligent approach to understanding consumer behavior and trends.
In summary, embracing these innovative sampling techniques empowers businesses to stay ahead in a competitive landscape, ultimately translating raw data into actionable strategies.
Overview of Key AI Sampling Methods
AI sampling methods play a crucial role in effectively managing data analysis within the realm of clustering tasks. These methods allow researchers and analysts to derive insights from large datasets by selecting representative samples that reflect the overall characteristics of the population. By utilizing AI techniques, the efficiency and accuracy of data collection improve significantly, resulting in more informed decision-making.
Several key AI sampling methods are commonly applied in clustering. These include random sampling, stratified sampling, and systematic sampling. Random sampling involves selecting individuals from a population in such a way that every member has an equal chance of being chosen. Stratified sampling divides the population into subgroups and samples from each subgroup, ensuring representation across key segments. Systematic sampling entails selecting samples based on a fixed interval, providing a structured approach to data collection. Understanding these methodologies enables businesses to refine their data strategies and enhance the quality of insights derived from their analyses.
Case Study: Applying AI Sampling in Clustering
In the case study on applying AI sampling methods in clustering, specific techniques are employed to derive actionable insights from complex datasets. These methods facilitate the grouping of similar data points, thereby enhancing the efficiency of analysis processes. By strategically selecting samples, analysts can represent larger populations while minimizing analysis time and resource expenditure. This is particularly useful in scenarios where data is extensive and diverse.
To illustrate, consider a scenario where customer feedback is analyzed across different regions. AI sampling methods can be used to extract representative samples from this feedback, allowing for the identification of regional trends and patterns. By clustering these samples, organizations can more effectively tailor their products and marketing strategies to meet the unique needs of each customer segment. This approach not only enhances understanding but also drives more informed decision-making, ultimately fostering a more agile and responsive business environment.
Extract insights from interviews, calls, surveys and reviews for insights in minutes
What Makes a Great AI Sampling Tool?
A great AI sampling tool is grounded in its ability to deliver precision and adaptability. First and foremost, it should offer a range of AI sampling methods that cater to various data types and clustering needs. Flexibility in sample selection enables users to extract meaningful insights tailored to their specific objectives. Furthermore, an intuitive user interface is crucial as it enhances the user experience, allowing both novice and expert users to navigate the tool effortlessly.
In addition to usability, the effectiveness of an AI sampling tool hinges on its analytical capabilities. This includes robust algorithms that ensure the reliability and accuracy of sampling results. Also, features such as real-time data processing can significantly enhance decision-making. Ultimately, a great AI sampling tool seamlessly integrates these attributes, driving impactful outcomes in clustering projects. By combining advanced technology with user-centric design, these tools empower organizations to harness the full potential of their data analysis endeavors.
Essential Features to Look For
When exploring AI sampling methods, itโs crucial to identify features that enhance usability and effectiveness in cluster tools. First, consider the tool's data integration capability. The ability to ingest data from diverse sources ensures comprehensive analysis and robust insights. Second, user-friendly dashboards play a significant role. A clear and intuitive interface allows users to easily navigate complex datasets without steep learning curves.
Next, look for real-time analytics. This feature enables quick decision-making by providing immediate feedback on sampling results, which is vital for adapting strategies promptly. Equally important is the tool's ability to generate detailed reports. Well-structured reporting provides clarity, helping users understand trends and make informed decisions. Lastly, ensure that the tool complies with data privacy regulations. Security and compliance are essential for maintaining trust and safeguarding sensitive information. By focusing on these essential features, users can select the best AI sampling methods to meet their specific needs in cluster analysis.
Insight7 and Other Leading Tools for AI Sampling
Insight7 stands out as a premier solution among various tools designed for AI sampling methods. Its user-friendly platform empowers businesses to analyze data gathered from customer conversations with remarkable efficiency. This capability is crucial in today's fast-paced market where timely insights can significantly influence competitive advantages. Many organizations struggle to manage the vast amounts of customer signals, and conventional analysis techniques often fall short in meeting these demands.
Other leading tools complement Insight7 by providing comprehensive features that cater to diverse AI sampling needs. For instance, Tool 1 offers advanced data visualization capabilities that simplify complex datasets. Tool 2 focuses on enhancing collaboration, ensuring that insights are readily accessible across teams. Tool 3 emphasizes automated reporting, making the translation of data into actionable strategies quicker and more efficient. Finally, Tool 4 specializes in integrating feedback from various sources, ensuring a holistic view. Together, these tools create a robust ecosystem, enhancing organizationsโ abilities to derive meaningful insights from their customer interactions.
- Insight7: The Top Choice
Choosing the right AI sampling methods can significantly impact the effectiveness of clustering tasks. Insight7 stands out as a top choice due to its user-friendly platform that simplifies data analysis at scale. When organizations grapple with immense volumes of customer interactions, traditional techniques may falter. This is where AI sampling methods come into play, providing timely and valuable insights that enable companies to stay ahead of competition.
One significant reason for Insight7's popularity is its ability to transform vast amounts of qualitative data into actionable business strategies. Users report frustration with manual analysis, finding it laborious and slow. By employing AI sampling methods within Insight7, businesses can swiftly translate insights into effective actions. Moreover, this tool promotes collaboration, ensuring that critical insights are easily accessible instead of scattered across various documents. Thus, Insight7 epitomizes the seamless integration of AI sampling methods into daily operations, driving efficiency and better decision-making.
- Tool 1: Detailed Overview
Tool 1 offers a comprehensive solution for effective AI sampling methods. It streamlines data analysis by enabling users to visualize insights extracted from various datasets. Users can easily identify positive and negative customer feedback, aiding in decision-making processes that drive product development. The tool's functionality allows for quick queries on different data points, enhancing the team's ability to draw correlations across multiple sources.
To make the most of Tool 1, consider the following aspects:
Data Visualization: This feature helps users interpret complex data faster. Visuals can highlight key trends and feedback, making insights more accessible.
Comparative Analysis: This allows users to assess differences in feedback between various datasets, providing a deeper understanding of customer sentiment.
Scalability: The ability to handle larger datasets is crucial. This tool streamlines the input of multiple files, facilitating extensive analysis efficiently.
By focusing on these functionalities, professionals can optimize their utilization of AI sampling methods for more robust outcomes.
- Tool 2: Detailed Overview
Tool 2 plays a vital role in the landscape of AI Sampling Methods, facilitating effective data analysis and clustering. This tool enhances understanding by offering refined techniques to sift through large datasets, allowing users to extract pertinent insights efficiently. By leveraging various sampling strategies, it optimizes the selection process, ensuring that the most representative data points are used for analysis.
In addition to improving efficiency, Tool 2 introduces innovative visualizations and analytical capabilities. Users can conduct comparative analysis across different datasets, enabling them to identify patterns and trends. This aspect is particularly crucial in market research, where nuanced insights can inform product development decisions. By deploying AI Sampling Methods through this tool, analysts can uncover hidden relationships within data, paving the way for actionable strategies and enhanced decision-making.
- Tool 3: Detailed Overview
In this section, we delve into Tool 3, which provides a detailed overview of an essential AI sampling method. Understanding the capabilities of AI sampling methods is crucial for efficient data analysis and clustering tasks. This tool translates complex data interactions into manageable insights, enabling users to make data-driven decisions more effectively.
The key aspects of this tool include its adaptability and user-friendly interface. Users can query datasets to uncover patterns, trends, and opinions expressed, regardless of the data set's scale. Additionally, it offers features that allow comparisons across different data groups, enhancing the ability to discern unique insights. Such functionalities empower organizations to utilize rich data for informed strategic planning while maintaining a focus on precision and reliability. With AI sampling methods, teams can extract actionable insights from large datasets, streamlining research and boosting organizational effectiveness.
- Tool 4: Detailed Overview
A thorough understanding of AI sampling methods is crucial for anyone involved in clustering tasks, and Tool 4 provides an in-depth exploration of this concept. This section examines how these methods effectively facilitate the extraction of meaningful insights from complex datasets. By employing systematic sampling techniques, users can identify patterns and trends that influence decision-making processes.
When utilizing AI sampling methods, consider the following key components:
- Data Quality: Ensure that the data is accurate and relevant for effective analyses.
- Sampling Techniques: Familiarize yourself with various sampling methods such as random, stratified, and cluster sampling.
- Implementation: Understand the practical applications of these methods in real-world scenarios.
- Evaluation: Assess the outcomes of the sampling process to ensure reliability and validity in insights gained.
By grasping these components, users can implement AI sampling methods effectively, thereby enhancing the quality and effectiveness of their clustering analyses. This knowledge ultimately supports more informed strategic decisions based on sound data interpretations.
Conclusion on AI Sampling Methods in Cluster Tools
AI Sampling Methods offer a powerful solution for enhancing the efficiency and accuracy of cluster tools. By selecting representative data samples, these methods allow for better analysis and insights, ultimately improving decision-making processes. As introduced in earlier sections, effective sampling is crucial for managing large datasets, which can easily overwhelm traditional analysis techniques.
In summary, the implementation of AI Sampling Methods not only streamlines the clustering process but also helps avoid potential biases, thus ensuring reliable outputs. Understanding and applying these sampling techniques can significantly advance your analytical capabilities, leading to more informed strategies and business outcomes.