Automated Insight Compilation revolutionizes the way AI research reports are generated, transforming complex data into actionable knowledge. In a world where vast amounts of information can overwhelm researchers, automated processes simplify reporting by extracting essential insights efficiently. This technology not only saves time but also enhances the accuracy and reliability of report generation, allowing researchers to focus more on strategic decision-making.
At its core, Automated Insight Compilation employs advanced algorithms to sift through large datasets and academic materials. By utilizing natural language processing and machine learning, this system identifies patterns and trends that would be difficult to discern manually. Ultimately, the capacity to automate insight generation empowers researchers to produce comprehensive reports swiftly, making significant strides in the field of AI research.
Transcribe & extract insights from interviews. At Scale.
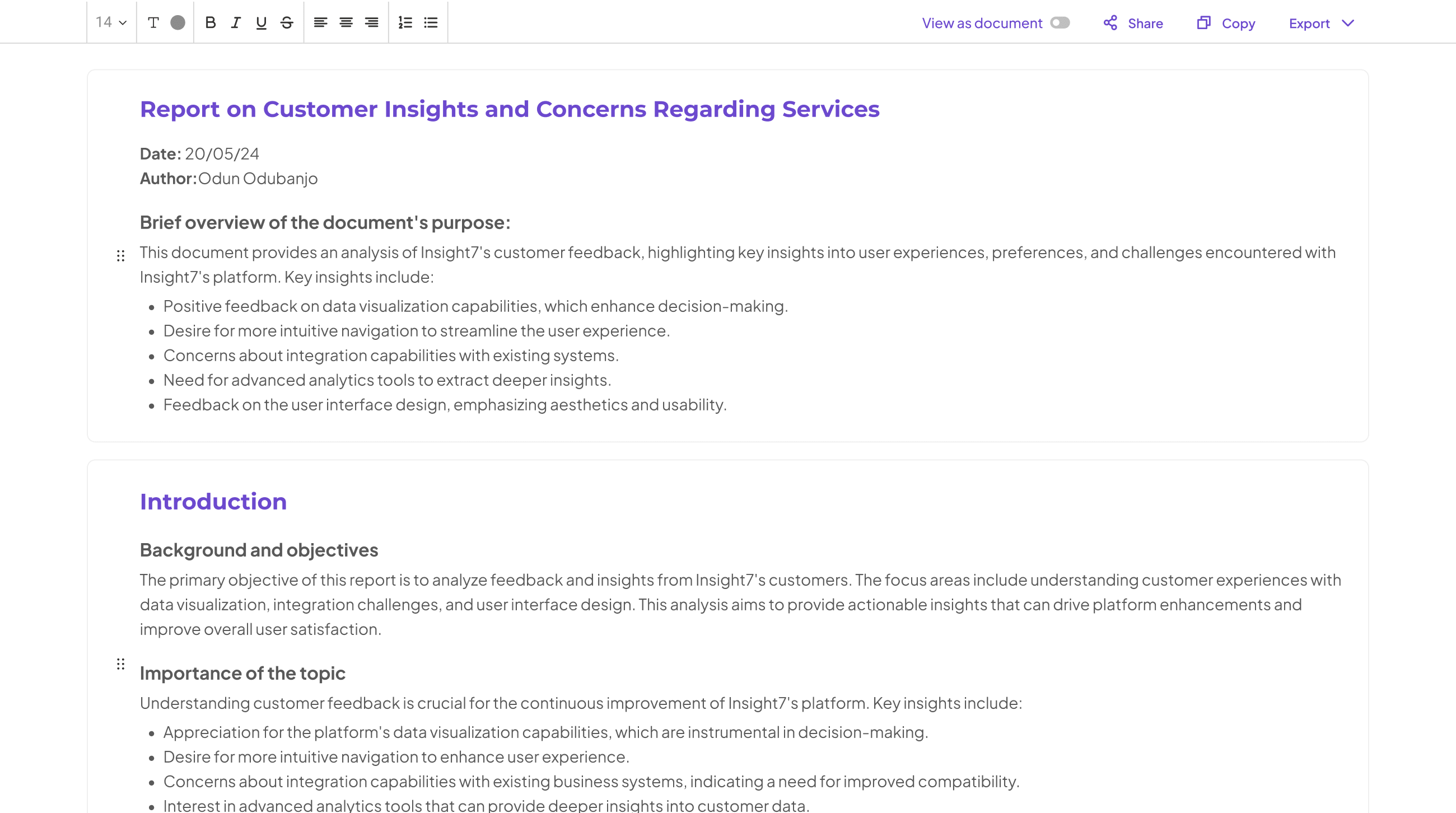
Understanding the Basics: Automated Insight Compilation
Automated Insight Compilation streamlines the process of extracting valuable insights from large datasets. By utilizing advanced technologies, this approach allows users to quickly analyze and interpret information without needing specialized skills. The combination of natural language processing and machine learning plays a crucial role in enhancing the efficiency of data analysis and report generation.
In practice, users can upload various data types, including interviews and surveys, which are then processed to uncover key trends and sentiments. The platform automatically identifies pain points and desired outcomes, providing clear visuals of customer experiences. This simplifies decision-making for teams and enables them to focus on strategic actions rather than manual data sorting. Ultimately, Automated Insight Compilation transforms how organizations leverage information, making insights accessible and actionable for everyone involved.
The Purpose Behind Report Generators
Automated Insight Compilation plays a crucial role in modern report generation. The growing amount of data in AI research necessitates efficient methods to extract valuable insights. This is where report generators come into play, enabling researchers to automate the compilation of complex information into coherent reports. Instead of spending countless hours in manual analysis, researchers can focus on interpreting results and making informed decisions.
The primary purpose behind these report generators is to save time and increase efficiency. By automating the process, teams can produce reports that are not only accurate but also tailored to their specific needs. This leads to more timely decision-making and allows researchers to stay ahead in their respective fields. Consequently, report generators significantly enhance the quality and speed of research, making them indispensable tools for contemporary AI-related inquiries.
- Discusses the need for automated report generation in AI research.
In the realm of AI research, the demand for automated report generation becomes increasingly evident. Automated Insight Compilation streamlines the tedious process of gathering and analyzing extensive data sets, allowing researchers to focus on more critical aspects of their work. When reports are generated automatically, researchers can quickly access impactful insights and trends, facilitating faster decision-making and boosting overall productivity.
Moreover, the ability to produce reports consistently and accurately reduces human errors that often occur in manual compilation. Automation not only saves time but also enhances collaboration among research teams, as shared insights can be easily formatted into cohesive documents. By investing in automated report generation systems, organizations can ensure they remain competitive in the rapidly evolving field of AI, ultimately propelling innovation forward. The integration of AI technologies to automate reports fosters a more efficient research environment, empowering professionals to derive actionable insights with confidence.
- Highlights the efficiency and time-saving benefits.
The efficiency and time-saving benefits of Automated Insight Compilation transform the research report generation process into a streamlined experience. By eliminating the need for manual data handling, researchers can focus on analyzing trends and meaningful patterns rather than getting bogged down with data entry. This not only accelerates the report creation process but also ensures that vital insights are pinpointed correctively and without delay.
Automated Insight Compilation empowers users to quickly analyze vast volumes of data, making it accessible to personnel without specialized expertise. With advanced functionalities like real-time transcriptions and insightful summaries at their fingertips, users gain the ability to generate actionable reports faster than ever before. This democratization of insight generation leads to increased productivity, enabling organizations to make informed decisions based on robust, real-time data analysis and insights instead of relying on outdated methods.
Core Technologies Powering Insight Compilation
Automated Insight Compilation relies on powerful core technologies that streamline data analysis and result generation. At the forefront, natural language processing (NLP) plays a crucial role in transforming unstructured text into actionable insights. This technology enables the system to understand context, interpret sentiments, and extract key themes from vast amounts of data efficiently.
In addition to NLP, machine learning algorithms enhance the analysis process by identifying patterns and trends within the input data. These algorithms learn from previous datasets, improving accuracy over time. Together, these technologies facilitate the synthesis of insights by comparing vast quantities of information, thus ensuring comprehensive and relevant results. By integrating these core technologies, Automated Insight Compilation becomes an invaluable tool in crafting insightful, dynamic, and user-friendly AI research reports. This not only saves time but also democratizes access to insights across different users and disciplines.
- Explores natural language processing (NLP) as a key technology.
Natural Language Processing (NLP) is a transformative technology that enables machines to understand and interpret human language seamlessly. This capability is pivotal for Automated Insight Compilation as it allows for the extraction of valuable information from vast datasets, including customer interactions and market research inputs. By analyzing natural language, NLP technologies can identify sentiments, themes, and key patterns that inform decision-making processes.
The implementation of NLP in report generation democratizes access to insights, making it easier for anyone within an organization to generate reports. Users can query specific datasets and receive tailored insights without needing extensive expertise. This ease of use facilitates quick adaptations to shifting market needs, ultimately enhancing the quality of strategic decisions. Understanding NLP's role reflects its significance in harnessing the power of language, driving efficiency, and supporting data-driven insights in AI research report generation.
- Details the role of machine learning algorithms in data analysis.
Machine learning algorithms play a crucial role in data analysis within automated insight compilation. These algorithms analyze vast amounts of data, identifying patterns and correlations that human analysis might overlook. By processing this information, machine learning algorithms can generate valuable insights swiftly and efficiently. This automation significantly reduces the time spent on manual data evaluation, allowing researchers to focus on interpretation rather than data gathering.
In the realm of report generation, machine learning fosters a data-driven approach to decision-making. Advanced models can process both qualitative and quantitative data, providing a comprehensive view of the information landscape. As a result, automated insight compilation not only enhances the speed of analysis but also improves the accuracy of the insights produced. Ultimately, the integration of machine learning in data analysis leads to more informed conclusions and facilitates better strategic planning across various domains.
Key Steps in the Automated Insight Compilation Process
The Automated Insight Compilation process involves several key steps that streamline the extraction and presentation of meaningful insights from diverse data sources. The first step is data collection and input, where relevant information, such as academic papers and research datasets, is gathered. This data is then pre-processed to ensure it is clean and ready for analysis.
Next comes the information analysis phase. Here, sophisticated AI models sift through the data, identifying patterns and trends that would otherwise remain hidden. This stage is crucial, as it transforms raw data into actionable insights, addressing specific research questions. Finally, generating the report wraps up the process. Automated systems craft coherent narratives, ensuring that the insights are not only accurate but also presented in a compelling manner, complete with visual aids and templates for easy comprehension. This structured approach enhances the overall efficiency and effectiveness of AI-driven research report generation.
Step 1: Data Collection and Input
Effective data collection and input serve as the foundation for successful automated insight compilation in AI research report generation. This initial step involves gathering relevant information from various sources, such as academic papers, customer interactions, or survey results. It is crucial to ensure that the data is accurate, comprehensive, and pertinent to the research objectivesโ.
After accumulating the data, the next phase is pre-processing, where the collected information is cleaned and organized for analysis. This preparation stage helps eliminate irrelevant data and noise, allowing for more precise insights. By structuring the data in a manageable format, researchers can efficiently analyze trends and patterns that emerge, forming the basis for the report generation process. Ultimately, these efforts in data collection and input pave the way for generating insightful, actionable reports that contribute to informed decision-making.
- Describes the gathering of relevant academic papers and data sources.
The gathering of relevant academic papers and data sources is vital for effective automated insight compilation. Initially, researchers need to identify reliable academic sources that align with their specific topics. This could include journals, conference proceedings, governmental reports, and credible online databases. Collecting diverse perspectives enriches the dataset, ensuring that the generated reports offer a well-rounded view of the subject matter.
Next, the process of inputting these documents into the report generator involves careful pre-processing. This step entails converting data into a format suitable for analysis. It may include transcribing audio calls, organizing documents, or integrating files from cloud storage. Whether extracting quotes or summarizing findings, the structure of the input data significantly impacts the quality of insights derived from it. Robust data management practices lay a solid foundation for meaningful output in AI-driven research reports.
- How input data is pre-processed for analysis.
The pre-processing of input data is a crucial initial step in the Automated Insight Compilation process. This phase ensures that data collected from various sources is organized and suitable for effective analysis. The first action is data cleaning, which involves eliminating irrelevant information, duplicates, and errors. This step helps in refining the dataset, increasing its overall quality and reliability.
Next, the data undergoes normalization, a process where varying units and formats are standardized. This is essential for maintaining consistency, especially when merging data from diverse sources. Finally, categorization allows for segmenting data into predefined themes or topics, facilitating targeted analysis. By adopting these strategies, the data becomes ready for insightful examination, allowing AI models to extract meaningful trends and patterns, which ultimately enhances the quality of generated reports.
Step 2: Information Analysis
In this phase, automated insight compilation takes center stage as data transforms into actionable intelligence. This process begins with gathering data from interviews and calls, which are systematically organized into projects. By analyzing this data, users can identify key patterns and themes that emerge consistently across different interactions. This reflective analysis allows for a high-level summary of insights, highlighting prevalent issues and needs expressed by customers.
The information is further enriched through AI models that sift through vast amounts of data to uncover underlying trends. Each identified theme, such as feedback analysis or resource allocation, is detailed with direct customer quotes, ensuring that the insights are both comprehensive and relatable. Through visualizations and reports, this structured approach not only simplifies the information but also enhances understanding, enabling informed decision-making. Ultimately, the outcomes of this analysis serve as a foundation for generating impactful research reports.
- Outlines the process of extracting meaningful insights from data.
The process of extracting meaningful insights from data involves several essential steps that transform raw information into valuable knowledge. Initially, diverse data sources are analyzed to uncover patterns and trends. Automated Insight Compilation employs advanced AI models that sift through vast amounts of data, identifying correlations that may not be immediately apparent. This analytical phase is crucial as it determines the relevance and significance of the information gathered.
Following analysis, the next step centers on synthesizing these insights into understandable narratives. This might include visualizations that highlight key themes, trends, and customer feedback. By presenting these findings effectively, stakeholders can make informed decisions that drive project outcomes, product improvements, or strategic directions. Ultimately, the automated generation of reports ensures that valuable insights are easily accessible, propelling efficient communication and fostering a data-driven culture within organizations.
- Utilizes AI models to identify patterns and trends.
Automated Insight Compilation revolutionizes how AI models are used to identify patterns and trends in data analysis. Through advanced algorithms, these models sift through vast datasets and highlight recurring themes, offering a comprehensive overview of vital information. This approach allows researchers to grasp critical insights quickly, transforming raw data into actionable knowledge.
In this process, multiple inputs are analyzed collectively, enabling AI systems to pinpoint prevalent issues or opportunities. For example, by examining call transcripts from various sources, AI can reveal common pain points mentioned by customers. Such detailed analysis not only streamlines reporting but enhances the precision of insights, ensuring that stakeholders can make informed decisions based on solid evidence. Consequently, leveraging these AI capabilities fosters a deeper understanding of trends over time, significantly benefiting research and strategic planning efforts.
Step 3: Generating the Report
In this crucial step of the automated insight compilation process, you'll learn how to generate a comprehensive report seamlessly. The AI research report generator utilizes advanced algorithms to craft clear narratives from your gathered data. By leveraging automation, the system collates key insights into structured formats, making the information both accessible and actionable. Templates provide a consistent framework, ensuring your reports look professional and are easy to understand.
There are several essential elements involved in generating the report. First, data visualization tools are employed to represent findings graphically, enhancing comprehension. Second, the generator streamlines the report format, allowing for quick adjustments based on user-defined preferences. Finally, automated summaries synthesize large volumes of information, delivering concise insights effectively. By focusing on these features, the automated report generator not only saves time but also produces high-quality documents that meet the needs of various stakeholders.
- Automation in crafting coherent narratives and reports.
Automation plays a crucial role in crafting coherent narratives and reports by synthesizing complex data into understandable formats. Using advanced technology, automated systems streamline the process of creating reports, significantly reducing the time and effort required. These tools analyze vast amounts of information and distill it into clear, actionable insights, ensuring that the final output maintains a logical flow and structure.
The process begins with data collection, where relevant sources are identified and organized. Once the data is collected, algorithms sift through it to identify key trends and patterns. This analysis culminates in report generation, where automated insight compilation brings together findings in a cohesive manner. By addressing the โwho, how, and whyโ behind the information, these systems enable users to produce high-quality reports that are both insightful and engaging, ultimately enhancing decision-making and strategic planning.
- Use of templates and visualization tools.
Templates and visualization tools play a crucial role in the automated insight compilation process. They streamline the way researchers access and analyze complex data sets. By using structured formats, these tools help ensure consistency across reports, allowing users to focus on the insights rather than formatting details.
Moreover, visualization tools transform raw data into understandable graphs and charts. This visual representation can illuminate trends, highlight comparisons, and provide clarity in presenting results. When researchers view positive and negative feedback through visual aids, they can quickly identify actionable insights. The integration of these templates enhances collaboration among teams, ensuring everyone can interpret data uniformly. Ultimately, these resources not only facilitate a smoother workflow but also lead to more informed decision-making in AI research.
Top Tools for Automated Insight Compilation
Automated Insight Compilation is transforming how businesses interpret data and generate reports. This process is essential for organizations seeking to harness diverse data streams effectively. Several tools stand out in this arena, simplifying the collection, analysis, and dissemination of insights.
First, consider Insight7, which allows users to easily transcribe calls and highlight specific pain points and behaviors from customer interactions. Its user-friendly interface enables anyone to access and analyze insights without needing extensive training. Next, tools like Lexalytics use natural language processing to extract sentiments and themes from vast datasets. MonkeyLearn offers customizable machine learning capabilities, empowering users to automate their data classification projects. Yseop Compose excels in crafting human-like narratives from structured data, ensuring clarity and coherence in reports. Each of these tools provides unique functionalities to support automated insight compilation, driving informed decision-making in organizations.
Insight7
Automated Insight Compilation significantly transforms how businesses process and harness customer feedback. The reliance on traditional methods has become a bottleneck, as they struggle to keep pace with the vast amounts of data generated from customer conversations. By automating insights compilation, businesses can streamline their analysis, making it more efficient and timely.
This innovation addresses common challenges such as lengthy manual analysis and fragmented insights. By integrating advanced technologies, companies can easily gather and analyze data, leading to actionable insights. These insights empower organizations to make informed decisions and strategies, enabling them to stay ahead of their competition. Ultimately, Automated Insight Compilation is essential for adapting to the rapidly changing market landscape, ensuring that businesses can respond effectively to customer needs and enhance overall service quality.
- Highlighting its features and functionalities in AI report generation.
The features and functionalities of an AI report generator focus on transforming significant data sets into actionable insights efficiently. Central to this process is Automated Insight Compilation, which streamlines research, enhances accuracy, and reduces time commitment. With capabilities like natural language processing and advanced analytics, the system enables users to extract and compile insights from diverse sources seamlessly, ensuring a comprehensive overview of relevant information.
Furthermore, the user interface allows for easy customization and editing of generated reports, providing flexibility in presentation. This includes the option to download insights in various formats, such as PDF or editable documents. As a result, compliance officers can refine and tailor the reports to meet specific client needs, adding a layer of personalization. Overall, the intuitive design and powerful analytics make AI report generation a vital tool for enhancing research efficiency and effectiveness.
Additional Tools
In the realm of Automated Insight Compilation, additional tools play a crucial role in enhancing overall efficiency and effectiveness. Various advanced platforms, such as Lexalytics and MonkeyLearn, allow researchers to scrutinize large volumes of text data effortlessly. These tools employ sophisticated algorithms capable of processing qualitative and quantitative data, enabling users to derive significant insights swiftly.
Moreover, Yseop Compose and Automated Insights extend the capabilities of traditional analysis by transforming raw data into comprehensible reports. These tools not only streamline the data interpretation process but also help maintain a structured approach to extracting valuable insights. By utilizing such advanced technologies, researchers can greatly improve the speed and accuracy of their findings, ensuring a competitive edge in their respective fields. Adopting these tools is essential for anyone serious about maximizing the potential of automated research report generation.
- Discuss tools like Lexalytics, MonkeyLearn, Yseop Compose, and Automated Insights and their uses.
Various tools are designed to aid in Automated Insight Compilation, each serving unique functions to streamline the reporting process. For instance, some applications analyze vast datasets by employing advanced natural language processing techniques. These technologies help in identifying key trends and sentiments within the data, making it simpler to extract actionable insights for reports.
Other tools focus on transforming unstructured data into structured formats, enabling users to easily visualize results. They can convert interviews or survey responses into narrative summaries and highlight crucial findings. Additionally, several platforms offer the capability to automate repetitive tasks, allowing teams to direct their efforts toward more complex analysis, ensuring that insights derived are relevant and timely. Ultimately, leveraging these technologies enhances efficiency and minimizes human error in the report generation process.
Conclusion: The Future of Automated Insight Compilation in AI Research
The future of automated insight compilation in AI research promises to transform how we analyze and report findings. As technology evolves, we can expect even greater accuracy and proficiency in extracting relevant information from vast datasets. This shift not only enhances productivity but also increases the reliability of insights drawn from research, making it easier for professionals to make informed decisions.
Moreover, automated insight compilation will likely continue to improve user experience by integrating more intuitive interfaces and customizable options. As researchers become adept at utilizing these tools, the potential for innovation in AI research significantly expands. With automation at the forefront, researchers can focus on critical analysis rather than mundane data entry, paving the way for groundbreaking discoveries and advancements.