AI Performance Metrics play a critical role in understanding the capabilities and effectiveness of artificial intelligence systems. As organizations increasingly leverage AI for strategic decisions, grasping these metrics becomes essential. A well-defined metric offers insights into performance, helping executives make informed choices that drive business outcomes.
In this introduction, we will explore the significance of AI Performance Metrics and how they can enhance reporting for executives. From assessing accuracy to analyzing throughput, understanding these metrics enables leaders to understand how AI technologies impact their operations. This foundation allows for improved decision-making and a clearer view of AI's contributions to business goals.
Analyze qualitative data. At Scale.
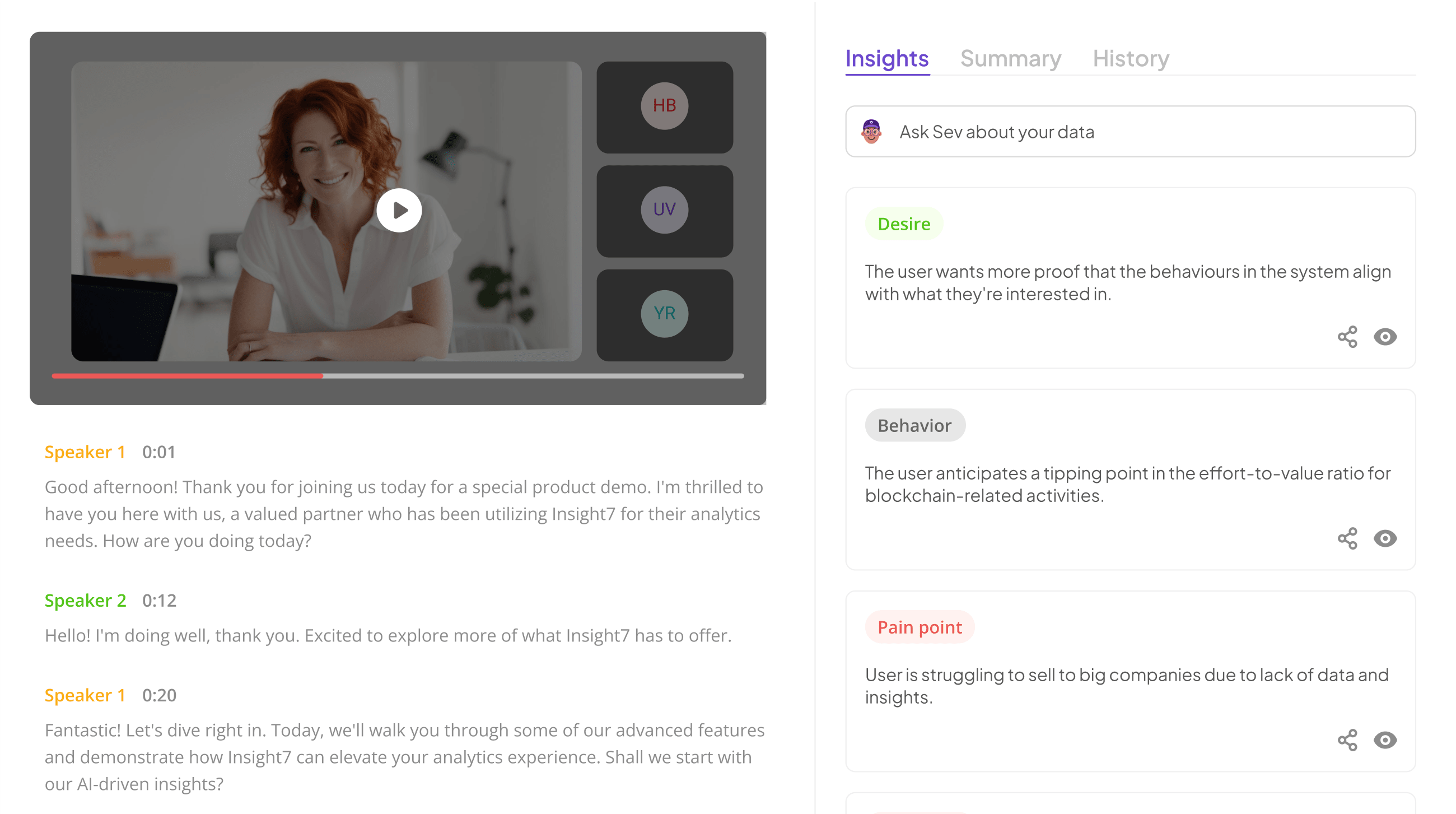
AI Performance Metrics serve as vital tools that enhance executive performance reports. These metrics help leaders gauge how well AI systems are functioning and impacting overall business outcomes. They provide a structured way to analyze performance, ensuring decisions are informed by data rather than intuition.
Focusing on key performance indicators is essential. For instance, metrics like accuracy, precision, and recall quantify how effectively an AI system performs its intended tasks. Additionally, understanding latency and throughput gives insights into system efficiency, highlighting areas for necessary improvement. By utilizing these metrics, executives can identify strengths and weaknesses within their AI initiatives, fostering a culture of continuous improvement. As AI technologies evolve, the need for precise metrics becomes increasingly relevant in driving strategic decisions. Ultimately, incorporating AI Performance Metrics in executive reporting leads to better decision-making and enhanced organizational performance.
The Importance of AI Performance Metrics in Executive Reports
AI Performance Metrics play a vital role in enhancing the effectiveness of executive reports. By accurately measuring how AI systems perform, organizations can make informed decisions that drive business growth. For executives, understanding these metrics is essential to identify strengths, weaknesses, and potential areas for improvement.
Furthermore, clear metrics provide a baseline for comparing different AI solutions, enabling leaders to allocate resources more effectively. This understanding empowers decision-makers to tailor strategies that align with organizational goals. Incorporating AI Performance Metrics helps create a transparent dialogue between technical teams and executive leadership, fostering collaboration and driving innovation.
In summary, the importance of AI Performance Metrics in executive reports cannot be overstated. These metrics provide a framework for evaluating AI's business impact and aligning technology with strategic objectives, ultimately leading to better outcomes for the organization.
Improving Decision-Making Through AI Performance Metrics
Decisions made by executives significantly impact the direction of a company. Improving decision-making through AI performance metrics can greatly enhance this process. These metrics provide valuable insights into the effectiveness and efficiency of AI systems, enabling leaders to make informed choices. By evaluating specific performance indicators, executives can assess risks and opportunities, reducing uncertainty and improving outcomes.
Understanding AI performance metrics helps in developing clear standards for accountability. By establishing these benchmarks, organizations can systematically measure performance, identify areas for improvement, and encourage smarter decision-making. These metrics not only facilitate enhanced accountability but also empower teams to adapt and innovate. Ultimately, robust AI performance metrics are crucial for driving business performance and ensuring strategic alignment with organizational goals.
Gauging Business Impact with AI Performance Metrics
To effectively gauge business impact with AI performance metrics, organizations must first clearly define the metrics that matter most to their objectives. These metrics provide critical insights into the effectiveness of AI systems, illuminating trends that influence decision-making and strategy. By monitoring selected performance indicators, companies can evaluate how AI initiatives contribute to operational efficiency and bottom-line results.
Furthermore, itโs essential to align AI performance metrics with business goals. This ensures the chosen measures reflect the organization's priorities and challenges. For instance, understanding latency, accuracy, and customer satisfaction metrics can help executives determine the value generated by AI implementations. Ultimately, effective tracking of these metrics aids in making informed choices that drive growth and innovation while ensuring that investments in AI deliver tangible business value.
Key AI Performance Metrics for Executive Reporting
In the realm of AI, key performance metrics are crucial for informed executive reporting. AI performance metrics serve as foundational indicators that help assess an AI system's effectiveness and reliability. These metrics are not only essential for understanding how well AI models function but also play a pivotal role in guiding executive decision-making. By analyzing these metrics, organizations can pinpoint areas for improvement and optimize AI applications for better business outcomes.
Notable performance metrics include accuracy, precision, recall, latency, and throughput. Accuracy gives a percentage of correct predictions, while precision and recall provide insights into the model's relevance and completeness. Latency refers to the time taken for the system to respond, and throughput measures how many tasks the system can handle in a given timeframe. By integrating these metrics into executive reports, leaders are better equipped to evaluate the performance and impact of AI initiatives effectively.
Extract insights from interviews, calls, surveys and reviews for insights in minutes
Accuracy, Precision, and Recall: Essential AI Performance Metrics
Accuracy, precision, and recall are pivotal in evaluating AI performance metrics and understanding its capabilities. These metrics help organizations gauge how well their AI models are making decisions based on available data. Accuracy measures the model's overall correctness, which indicates the percentage of correct predictions among total predictions. High accuracy demonstrates that the AI system generally performs well in its predictions.
Precision and recall provide deeper insights into model performance. Precision reflects the proportion of true positive results in relation to all positive predictions made by the model. It helps assess the quality of positive predictions. Recall, on the other hand, measures the model's ability to identify all relevant instances, indicating how many actual positives were captured. Together, these metrics offer a comprehensive understanding of AI effectiveness, assisting leaders in making informed decisions based on robust analytical insights.
Latency and Throughput in AI Systems
In AI systems, latency and throughput are crucial metrics that directly impact performance. Latency refers to the time taken for a system to respond to a request, while throughput measures the number of requests processed over a specific time period. Understanding these aspects is essential for optimizing AI functionality and enhancing user experience.
Latency: This metric reflects how quickly an AI system can deliver results. High latency can hinder user satisfaction and operational efficiency. For instance, in customer service applications, a delay in response can lead to frustration, diminishing the overall user experience.
Throughput: This indicates how many tasks or requests an AI system can handle simultaneously. Maximizing throughput is vital for performance, especially under high-demand circumstances. Organizations need to balance between minimizing latency and maximizing throughput to achieve optimal AI performance metrics, ultimately supporting informed decision-making at the executive level.
Tools for Analyzing AI Quality Metrics
When it comes to tools for analyzing AI quality metrics, several robust options can significantly enhance performance reporting. Insight7 offers a user-friendly interface designed to simplify data analysis, enabling executives to make informed decisions based on accurate AI performance metrics. With its comprehensive analytics dashboard, users can visualize performance at a glance.
TensorBoard is another powerful tool that assists in tracking model metrics, providing detailed insights into training performance and parameters. MLflow allows teams to manage the machine learning lifecycle effectively, from experimentation to deployment. Furthermore, Neptune.ai aids in tracking experiments and visualizing results, ensuring that teams can maintain high-quality AI standards. By utilizing these tools, organizations can ensure that their AI systems not only meet quality metrics but also contribute positively to their overall performance outcomes.
insight7
Organizations today are increasingly reliant on AI performance metrics to inform decision-making and strategy. Insight7 focuses on the essential quality metrics that can elevate executive reports by translating raw data into actionable insights. These metrics enable leaders to gauge performance accurately, ensuring that they remain competitive in their respective industries.
To deepen understanding, key AI performance metrics include accuracy, which gauges the correctness of predictions; precision, which measures the exactness of positive outcomes; and recall, which assesses how well the system identifies all relevant instances. Furthermore, considering latency and throughput is vital for operational efficiency, as low latency and high throughput directly contribute to enhanced user experiences. By integrating these metrics, executives can drive significant improvements across their organizations, positioning themselves for sustained success in a data-driven world.
TensorBoard
TensorBoard is a powerful visualization tool designed to help monitor and analyze AI performance metrics effectively. By providing insights into the training process of machine learning models, it allows users to track their modelsโ performance over time. The intuitive interface enables users to visualize loss functions, accuracy, and other relevant metrics, which are crucial for making informed decisions in AI development.
Within TensorBoard, data is organized to showcase various aspects of model performance. It clearly displays graphs and charts that depict how models learn, including overfitting or underfitting trends. By consistently monitoring these AI performance metrics, stakeholders can identify areas needing improvement, ensuring models meet the desired benchmarks. Ultimately, TensorBoard empowers teams to enhance model quality, driving better outcomes for executive performance reports and overall business objectives.
MLflow
MLflow is a pivotal tool in the landscape of AI performance metrics, integral for tracking and managing machine learning models. Its design enables seamless experimentation and deployment, facilitating the collection of vital data that can be transformed into actionable insights. In the realm of AI, ensuring high performance requires consistency in monitoring various metrics throughout the model's lifecycle.
One key benefit of MLflow is its capacity for collaboration among teams, allowing data scientists and engineers to share insights easily. Users can log metrics, parameters, and artifacts, which contribute to well-rounded AI performance evaluations. This holistic approach enables organizations to make informed decisions based on comprehensive data analysis, ultimately driving enhanced business outcomes. Emphasizing quality metrics in AI, MLflow supports the shift toward data-centric strategies that ensure organizations remain competitive in an evolving market.
Neptune.ai
This platform serves as a pivotal tool for collecting and analyzing AI performance metrics that can significantly influence executive performance reports. Its user-friendly interface enables team members, regardless of their technical expertise, to engage with the data effectively. By streamlining insights from various processes, organizations can make data-driven decisions swiftly.
The tool offers a comprehensive library to house project files, facilitating the analysis of customer interactions and behaviors. Users can readily extract key insights, such as pain points and customer desires, while visually interpreting data through intuitive dashboards. This functionality enhances the ability to identify actionable metrics, ultimately supporting effective performance reporting for executives. By democratizing access to vital insights, it fosters a culture of informed decision-making, leading to improved organizational outcomes and strategic alignment in artificial intelligence initiatives.
Conclusion: Elevating Executive Reports with AI Performance Metrics
The integration of AI performance metrics into executive reports can substantially boost the quality and relevance of insights provided to leadership teams. By harnessing these metrics, decision-makers gain a clearer, data-driven view of operational effectiveness and AI capabilities. This empowerment allows organizations to align strategic initiatives with measurable outcomes, ultimately enhancing performance and fostering growth.
Moreover, utilizing well-defined AI performance metrics cultivates a culture of accountability and transparency. This approach not only facilitates informed decision-making but also enhances stakeholders' trust in AI systems. In conclusion, prioritizing these metrics in executive reporting is essential for transforming data into actionable insights, paving the way for improved business results.