AI-Driven Research Databases represent a significant evolution in how researchers manage qualitative data. These innovative platforms harness the power of artificial intelligence to streamline data collection, organization, and analysis. By automating repetitive tasks, they enable researchers to focus on deriving insights that can drive impactful decisions.
In this section, we will explore how AI-Driven Research Databases enhance the research process. Researchers can leverage advanced filtering, sorting, and reporting capabilities to isolate specific themes relevant to their studies. This targeted approach not only saves time but also ensures a higher quality of insights through precise data extraction and interpretation.
Analyze & Evaluate Calls. At Scale.
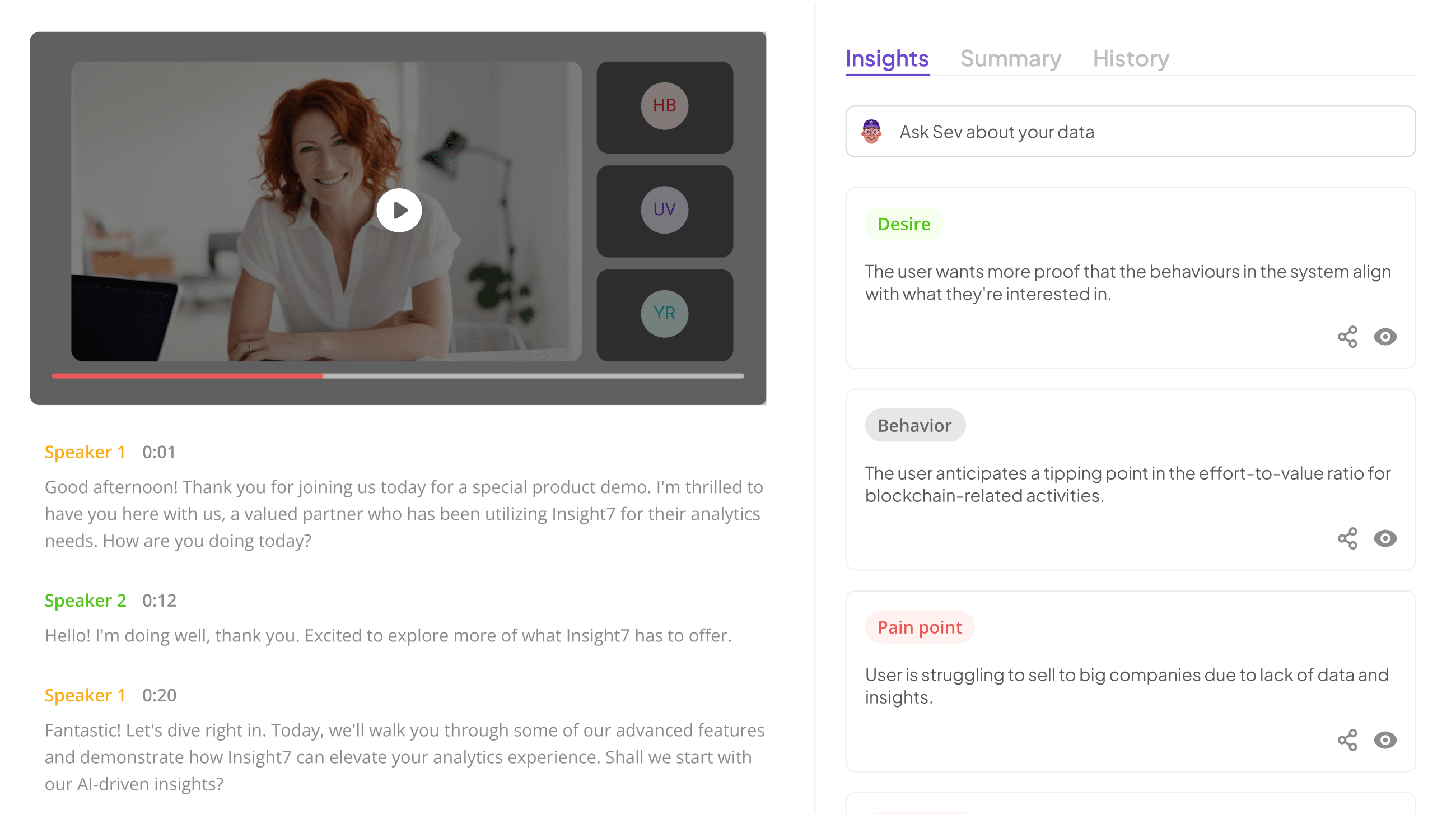
Understanding AI-Driven Qualitative Research Databases
AI-driven qualitative research databases are transforming the way researchers analyze and interpret data. By integrating advanced algorithms and machine learning capabilities, these databases streamline the management of qualitative data, making it easier to derive meaningful insights from interviews and open-ended survey responses. This integration helps address common issues such as bias, inconsistency, and time-consuming manual analysis, allowing researchers to focus on generating value from their findings.
Utilizing AI-driven research databases enables teams to consolidate various qualitative inputs, ensuring that diverse perspectives are captured without losing accuracy. Additionally, researchers can rapidly identify trends and themes in data, which leads to quicker reporting and enhanced client satisfaction. This dynamic approach not only enhances the overall quality of research but also empowers teams to maintain a competitive edge in an increasingly fast-paced environment. Adopting such innovative tools will ultimately redefine how qualitative research is conducted, fostering a more efficient and insightful research process.
The Role of AI in Enhancing Research Databases
AI-Driven Research Databases are revolutionizing how researchers access, analyze, and interpret data. By harnessing the capabilities of artificial intelligence, these databases enhance user experience, allowing efficient data management. One significant advantage is the ability to quickly ingest vast amounts of information from various sources. This capability accelerates the research lifecycle and enables users to derive insights almost instantaneously.
Furthermore, AI enhances data analysis through advanced algorithms that identify patterns and extract relevant themes from qualitative data. This means researchers can focus on interpreting results rather than spending excessive time on manual data processing. Enhanced visualization tools also facilitate better comprehension of complex data sets, making the research findings more accessible. By streamlining these processes, AI-Driven Research Databases optimize research productivity, fostering a more effective research environment overall. Through these advancements, researchers are poised to leverage AI's full potential, transforming how qualitative research is conducted and displayed.
Challenges Addressed by AI-Powered Tools
AI-powered tools address several persistent challenges in building qualitative research databases. First, researchers often struggle with data organization and analysis due to vast amounts of unstructured information. Traditional methods can be time-consuming, leading to missed insights. AI-Driven Research Databases streamline data categorization, making it easier to organize and retrieve critical information.
Additionally, human biases can unintentionally distort qualitative research results. AI-powered analytics can help reduce these biases by analyzing data objectively and presenting findings based on patterns rather than preconceived notions. Another significant challenge is the collaboration among team members, as team members often work in silos. AI tools foster seamless collaboration by allowing for real-time data sharing and updates, ensuring that everyone is on the same page. These advancements ultimately enhance the quality, speed, and reliability of qualitative research.
Extract insights from interviews, calls, surveys and reviews for insights in minutes
Exploring Top AI Tools for Building Research Databases
AI-Driven Research Databases have transformed the way researchers manage and analyze qualitative data. A myriad of AI tools is now available, each offering unique capabilities designed to enhance the efficiency and effectiveness of research processes. Understanding these tools is essential for researchers committed to expanding their databases while maintaining rigor and accuracy in their findings.
Several key tools stand out in the field of AI-powered research database management. NVivo simplifies data analysis through coding and organization, making it easier for researchers to draw meaningful insights. Atlas.ti facilitates collaborative efforts by enabling teams to engage in real-time data analysis. Similarly, MAXQDA supports diverse data sources with advanced analysis features, streamlining complex research tasks. By incorporating these tools, researchers can navigate through massive datasets with improved precision and clarity, ultimately enhancing the quality of their research outcomes. Embracing AI technology not only aids in data management but also promotes innovation in qualitative research methodologies.
insight7: Leading the Charge in AI-Driven Research Databases
AI-Driven Research Databases are transforming the way qualitative research is conducted and analyzed. These databases utilize advanced algorithms to process vast amounts of data, extracting meaningful insights that would take significant time and effort to uncover manually. By automating data ingestion and analysis, researchers can focus on interpreting results rather than getting bogged down by the data collection process.
The key features of these AI-powered solutions include rapid transcription capabilities, multilingual support, and the ability to generate visualizations from aggregated data. They also reduce the risk of bias in research findings by offering an objective view of the data. Applications of AI in research databases are far-reaching, from refining interview transcriptions to uncovering patterns across multiple studies. As demand for faster, more accurate research insights continues to grow, AI-Driven Research Databases stand at the forefront of qualitative research innovation.
Key Features and Benefits
AI-Driven Research Databases come packed with several key features that significantly enhance qualitative research processes. First, they offer intuitive data organization, allowing researchers to easily categorize and access insights through a user-friendly interface. This feature streamlines the analysis, enabling users to focus on deriving meaningful conclusions rather than getting lost in data complexity.
Next, these tools provide advanced analytical capabilities. They incorporate AI algorithms to identify patterns and themes within qualitative data, simplifying the extraction of actionable insights. This not only saves time but also increases the accuracy and reliability of findings. Furthermore, the ability to generate structured reports quickly facilitates effective communication of results, helping stakeholders make informed decisions.
Overall, the adoption of AI-driven research methods boosts productivity while ensuring that researchers can maintain a sharp focus on their project goals, ultimately leading to richer insights and more impactful outcomes.
Use Cases and Applications
AI-Driven Research Databases are transforming how qualitative research is conducted, enabling researchers to harness data in impactful ways. These databases facilitate applications across various fields, enhancing both workflow efficiency and insight extraction. For example, marketing research teams can utilize AI tools to analyze consumer sentiments and preferences, generating actionable insights that influence product development and positioning.
Additionally, academic researchers can use AI-powered solutions to streamline the coding and thematic analysis of qualitative data. This allows for better categorization of insights, ensuring that findings are relevant to specific research questions. As these applications evolve, the potential of AI-Driven Research Databases continues to expand, opening new avenues for collaboration and knowledge sharing that can enhance overall research productivity and effectiveness. Such capabilities not only save time but also elevate the quality of insights derived from qualitative data, making them indispensable in contemporary research methodologies.
Other AI Tools for Research Database Management
AI-driven research databases are transforming the way we manage qualitative data, offering innovative solutions beyond conventional methods. Several noteworthy tools enhance research database management, each catering to different needs and preferences.
NVivo: This software allows researchers to analyze unstructured data through powerful qualitative analysis features. It supports complex queries and visualizations that help in extracting key themes from data sources, making it easier to manage and interpret qualitative insights.
Atlas.ti: Known for its intuitive interface, Atlas.ti specializes in organizing and analyzing textual and multimedia data. The tool enables qualitative researchers to build comprehensive databases, linking various data pieces for deeper insights.
MAXQDA: MAXQDA combines qualitative and mixed methods research tools. It provides features for effective coding, visual data analysis, and team collaboration. This flexibility helps researchers to tailor databases according to specific project requirements.
These AI-powered tools streamline the process of managing qualitative research data, allowing researchers to focus on deriving meaningful insights rather than grappling with data logistics.
NVivo
NVivo is a powerful tool designed to facilitate qualitative data analysis, making it an essential component in the realm of AI-driven research databases. This software allows researchers to explore vast amounts of text and multimedia data intuitively. By utilizing coding and data visualization features, NVivo streamlines the organization and interpretation of qualitative findings.
With NVivo, researchers can easily categorize data, uncover patterns, and develop themes from complex qualitative data sets. This tool also integrates various data sources, enabling a comprehensive analysis strategy that combines interviews, surveys, and social media content. Its AI capabilities enhance the efficiency of qualitative research, transforming raw data into actionable insights, ultimately aiding researchers in making informed decisions based on empirical evidence.
Atlas.ti
Atlas.ti represents a formidable tool in the realm of AI-driven research databases. This platform is designed to assist researchers in organizing and analyzing qualitative data effectively. By integrating AI capabilities, it empowers researchers to identify patterns and insights that may otherwise remain hidden. The user-friendly interface allows for seamless interaction with complex datasets, facilitating deeper understanding.
The platform supports various research types, such as interviews, focus groups, and open-ended survey responses. Its advanced coding functions enable researchers to categorize data swiftly, making the synthesis of findings more efficient. Additionally, Atlas.ti provides visualization tools, helping researchers present their insights clearly. As the demand for qualitative research grows, utilizing AI-driven capabilities can enhance data analysis, ultimately leading to more informed conclusions and better decision-making in organizations. This makes it an essential consideration for any researcher looking to streamline their qualitative research processes.
MAXQDA
MAXQDA serves as a versatile tool for qualitative research, focusing on streamlining data analysis and enhancing user experience. This AI-powered software allows researchers to easily manage, evaluate, and visualize qualitative data, which is crucial for building efficient AI-driven research databases. One of the key advantages of using MAXQDA lies in its capability to handle various data types, including interviews, focus groups, and survey results, making it adaptable to different research needs.
Additionally, MAXQDA incorporates features such as coding, retrieval of quotations, and interactive visualizations, which help researchers conduct comprehensive analyses without becoming overwhelmed by data volume. By automating repetitive tasks, the software empowers users to concentrate on deriving meaningful insights. Overall, MAXQDA plays a significant role in shaping AI-powered research methodologies, allowing researchers to uncover deeper insights while managing their databases efficiently.
Conclusion: Revolutionizing Research with AI-Driven Databases
In the evolving landscape of research, AI-Driven Research Databases are transforming how researchers collect, analyze, and interpret qualitative data. By integrating advanced algorithms and data management techniques, these databases streamline the research process, ensuring swift access to invaluable insights. As researchers face increasing demands for rapid results, AI tools facilitate faster data ingestion and analysis, enabling teams to focus on strategic decision-making.
The future of qualitative research lies in these AI systems, which not only enhance efficiency but also improve the accuracy of insights. With AI handling data categorization and trend identification, researchers can uncover patterns that would otherwise remain hidden. Ultimately, embracing AI-Driven Research Databases is essential for staying competitive and responsive in today’s fast-paced research environment.