Creating a structured markdown outline for a blog post on AI-powered methods to analyze customer complaints at scale sets the foundation for informed discussion. The first section introduces the concept of automated complaint analysis, emphasizing its importance in today's business landscape. Understanding how to effectively gather and assess customer feedback allows organizations to identify patterns, thereby improving service and addressing concerns swiftly.
Next, we delve into the mechanics of AI in automated complaint analysis. This includes a thorough exploration of why businesses must adopt these technologies and how AI effectively enhances the overall analysis process. To implement these AI-powered methods successfully, it’s essential to outline clear steps. These will include data collection and preprocessing, model selection, training, deployment, and monitoring. Furthermore, we will review top tools like Insight7 and others that aid in ensuring an efficient complaint management system. This outline not only emphasizes key tactics but also paves the way for deeper insights into implementing automated complaint analysis effectively.
Introduction to Automated Complaint Analysis
In today's fast-paced business environment, customer complaints can significantly impact a company's reputation and bottom line. Automated Complaint Analysis emerges as an essential tool, enabling businesses to manage and interpret large volumes of feedback with efficiency and accuracy. By employing AI-powered methods, organizations can swiftly identify recurring issues, prioritize urgent concerns, and ultimately enhance the customer experience.
Automated Complaint Analysis streamlines the process of understanding customer sentiments and pain points. Through sophisticated algorithms and data processing techniques, businesses can effectively translate raw feedback into actionable insights. This not only helps in mitigating potential risks but also fosters a culture of continuous improvement, where the voice of the customer informs strategic decision-making and service enhancements.
Analyze qualitative data. At Scale.
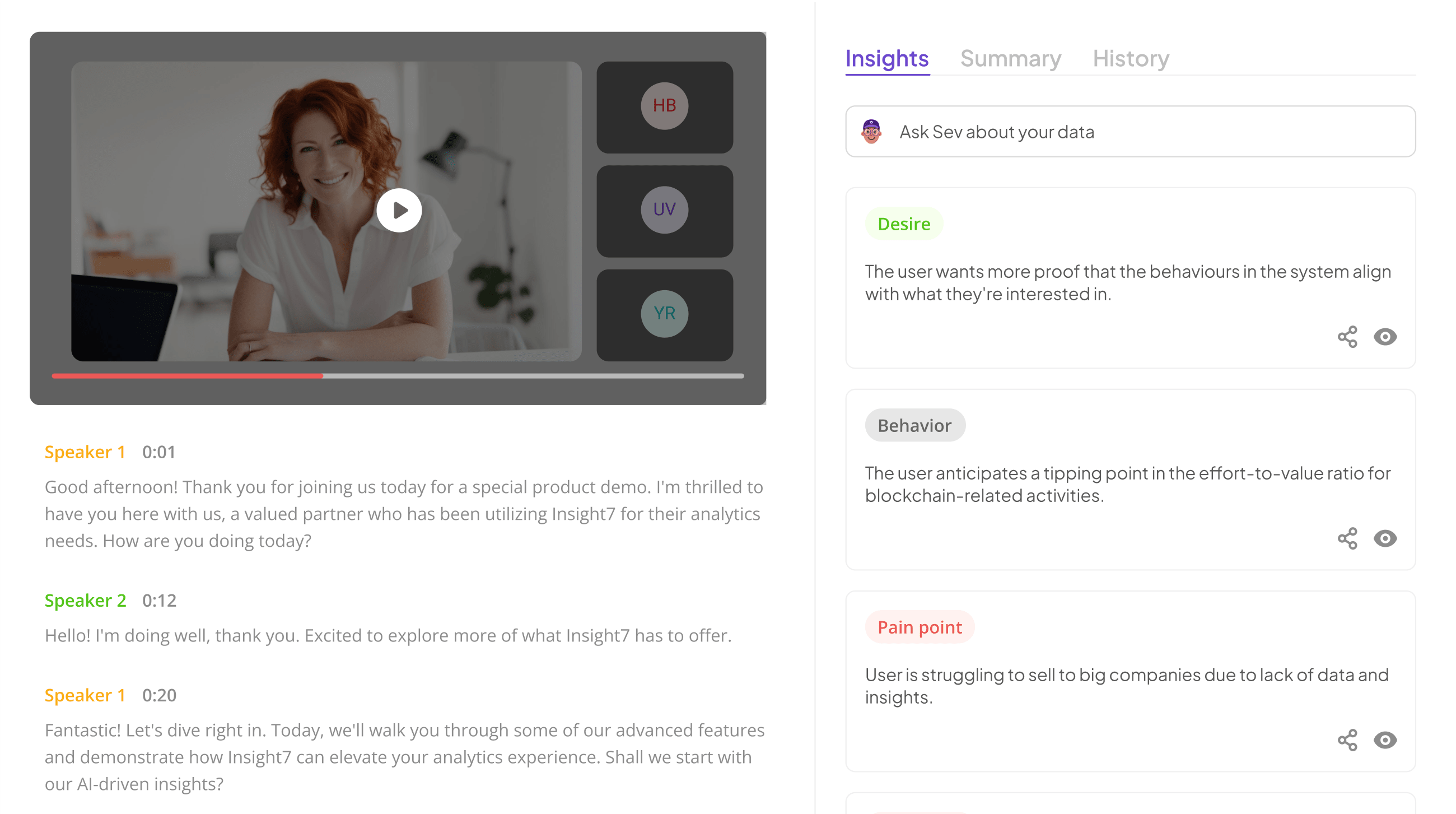
Exploring AI in Automated Complaint Analysis
Artificial intelligence plays a transformative role in automated complaint analysis, revolutionizing how businesses understand customer feedback. By leveraging machine learning algorithms, organizations can efficiently analyze vast amounts of complaint data, identifying patterns and sentiments that human analysts may overlook. This technology enhances responsiveness by prioritizing issues based on urgency and relevance, ultimately leading to improved customer satisfaction.
The journey towards effective automated complaint analysis begins with robust data collection and preprocessing. Accurate models must then be selected and trained to differentiate between varied types of complaints. Once the system is deployed, continuous monitoring ensures its performance remains optimal. These methods facilitate proactive complaint management and allow businesses to glean actionable insights, guiding future improvements. With tools like Insight7 and others, organizations are better equipped to tackle customer concerns at scale, making them more resilient and customer-focused.
The Need for Automated Complaint Analysis
Automated Complaint Analysis has become essential in today's business landscape. As organizations grow and customer bases expand, the volume of complaints can overwhelm traditional response mechanisms. Automating this analysis provides a streamlined approach, enabling companies to swiftly identify trends, recurring issues, and areas for improvement. By leveraging automated systems, businesses can process thousands of complaints in real-time, providing timely responses and better customer service.
Moreover, automated complaint analysis minimizes human error, ensuring that insights drawn from data are precise and actionable. The need for such analytical tools arises from the increasing complexity of customer expectations. Businesses that harness automated systems not only enhance operational efficiency but also cultivate deeper customer relationships. Embracing automated complaint analysis can lead to improved customer satisfaction and loyalty, making it a crucial component in any customer-centric strategy.
How AI Enhances Automated Complaint Analysis
AI enhances automated complaint analysis by transforming how organizations process and interpret customer feedback. Through natural language processing and machine learning, AI can sift through vast amounts of data, extracting key patterns and sentiments. This technology enables businesses to identify common issues, helping them prioritize which concerns to address first. By automating data interpretation, organizations significantly reduce the time taken to analyze customer complaints, allowing for quicker and more informed responses.
Moreover, AI-driven systems continually learn from new data. This adaptability ensures that the analysis remains relevant and accurate over time. Organizations can utilize various tools, such as Insight7, to complement AI's capabilities, leading to more insightful outcomes. By harnessing AI for automated complaint analysis, businesses can not only enhance their response strategies but also improve overall customer satisfaction. Ultimately, this tech-driven approach empowers organizations to create a more proactive and customer-centric service model.
Implementing AI-Powered Methods for Scalable Complaint Management
To implement AI-powered methods for scalable complaint management, organizations should first focus on automating complaint analysis. This approach enables businesses to handle large volumes of customer feedback efficiently. By employing machine learning models, businesses can quickly extract insights from complaints, identify recurring issues, and categorize them for further action. This reduces response times and improves customer satisfaction.
Next, effective complaint management systems should include robust data collection techniques. Utilizing tools that automate data gathering ensures a seamless flow of information. Similarly, applying advanced text analysis helps in gauging sentiment and urgency in customer messages. Continuous monitoring and model updates will further enhance the effectiveness of these systems. By harnessing AI's capabilities, organizations can transform customer complaints into actionable insights, driving meaningful improvements in service delivery and customer experience.
Extract insights from interviews, calls, surveys and reviews for insights in minutes
Steps to Deploy an AI Complaint Analysis System
To deploy an effective AI Complaint Analysis System, start by focusing on Data Collection and Preprocessing. Gather diverse datasets from various customer feedback channels, ensuring you have a rich variety of complaints and sentiments. Standardize the format of this data to prepare it for analysis. This initial step is crucial; the quality of your data directly affects the effectiveness of the automated complaint analysis.
Next, move on to Model Selection and Training. Choose the right machine learning models suited for analyzing text, such as Natural Language Processing (NLP) algorithms. Train the models using the collected data, ensuring they learn both the nuances of customer complaints and the context behind them. Once trained, proceed with Deployment and Monitoring, where the models are integrated into your existing systems. Continuously monitor their performance to make necessary adjustments, ensuring they remain effective over time. This cycle of refinement is essential for maintaining a robust automated complaint analysis process.
Step 1: Data Collection and Preprocessing
In the journey toward effective automated complaint analysis, the initial phase, focusing on data collection and preprocessing, is crucial. Collecting comprehensive customer feedback can provide invaluable insights into their experiences and expectations. This typically involves gathering data from various sources such as online reviews, social media platforms, and direct communication channels. Proper tools can assist in streamlining this process, ensuring that diverse data points are effectively captured.
Once the data is collected, preprocessing is essential to ensure its quality and relevance. This step involves cleaning the data by removing duplicates, irrelevant entries, or any inconsistencies. Techniques such as text normalization, tokenization, and stopword removal are commonly employed. Additionally, sentiment analysis may be integrated at this stage to categorize complaints based on emotional tone. By achieving clean and structured data, businesses pave the way for accurate analysis, ultimately enhancing the overall effectiveness of automated complaint analysis initiatives.
Step 2: Model Selection and Training
Model selection and training are crucial components in the process of automated complaint analysis. Once the data is collected and preprocessed, the next step is to identify the right model that can effectively analyze customer complaints. Selecting the suitable model requires understanding various algorithms, including decision trees, random forests, and deep learning methods. Each model has unique strengths that cater to different analytical needs.
Once a model is selected, training involves feeding it the prepared data to help it learn patterns and trends in customer complaints. This training process helps improve the model's accuracy and efficiency over time. It's important to validate the model periodically to ensure it continues to perform well as new data comes in. Ultimately, effective model selection and training play a vital role in producing insights that can enhance customer service and satisfaction.
Step 3: Deployment and Monitoring
To effectively execute Automated Complaint Analysis, deployment and monitoring are crucial stages that follow model selection and training. In this phase, the developed AI system is integrated into the existing infrastructure, ensuring it can process incoming customer complaints in real-time. Clear protocols must be established during this process to facilitate a seamless user experience and maintain system reliability.
Monitoring is equally important, as it provides ongoing insights into the AI’s performance. Regular evaluation helps identify areas for improvement, ensuring the system adapts over time. Key performance indicators (KPIs) should include accuracy rates, response times, and user satisfaction scores. These metrics guide adjustments and enhance the system's response to evolving customer needs. Furthermore, transparent communication with customers about how their complaints are managed fosters trust and satisfaction, paving the way for improved service delivery.
Top Tools for AI Complaint Analysis
When exploring the top tools for AI complaint analysis, it’s essential to consider their unique capabilities and use cases. Insight7 stands out as a robust tool designed for quantitative analysis and data transcription, making it invaluable for transforming raw feedback into actionable insights. Its ability to process large datasets quickly enables companies to pinpoint trends and recurring issues efficiently.
Other noteworthy tools include MonkeyLearn, which specializes in text analysis and sentiment detection. This tool allows businesses to categorize customer complaints effectively, providing deeper insights into customer sentiments. IBM Watson Natural Language Understanding enhances language processing through its advanced AI algorithms, ensuring nuanced comprehension of customer feedback. Meanwhile, platforms like Clarabridge bring together various feedback sources, helping organizations paint a comprehensive picture of customer sentiment. Utilizing these tools, companies can streamline their automated complaint analysis processes and enhance customer satisfaction at scale.
insight7
Automated Complaint Analysis plays a pivotal role in understanding customer grievances more efficiently. By harnessing advanced algorithms, businesses can sift through vast volumes of complaints to identify trends and patterns. This capability allows organizations to prioritize issues that significantly impact customer satisfaction, enabling a more responsive approach.
Moreover, employing AI-powered tools like Insight7 can enhance this process by offering insightful quantitative analysis. The synergy between machine learning models and data interpretation tools allows for accurate identification of recurring complaints. Consequently, organizations can focus on improving customer experiences rather than merely addressing individual issues. Ultimately, this automated approach not only fosters customer loyalty but also streamlines operational efficiency, making it an essential strategy for modern businesses seeking to thrive in a competitive landscape.
MonkeyLearn
MonkeyLearn offers innovative solutions tailored for automated complaint analysis, enabling businesses to efficiently process vast amounts of customer feedback. By leveraging natural language processing, this tool facilitates the understanding of customer sentiments and themes within complaints. Companies can analyze trends and common issues without the need for extensive manual review.
The platform's features allow organizations to categorize complaints effectively and draw actionable insights from them. This automated complaint analysis not only saves time but also enhances the quality of customer service by identifying areas needing attention. Ultimately, utilizing such AI-powered tools can transform how businesses respond to customer dissatisfaction, paving the way for improved satisfaction and loyalty in the long run.
IBM Watson Natural Language Understanding
IBM Watson Natural Language Understanding is a powerful tool designed to streamline the process of analyzing customer complaints. By employing advanced AI techniques, it enables organizations to extract meaningful insights from vast amounts of unstructured text data. This capability is essential for effectively handling customer grievances at scale. Automated Complaint Analysis is enhanced by its ability to identify sentiment, key themes, and relevant concepts within customer feedback.
The functionality encompasses several critical aspects. Firstly, it processes language with precision, delivering accurate sentiment analysis and keyword extraction. Secondly, it categorizes complaints based on predefined metrics, helping teams prioritize and address urgent issues. Lastly, the integration of this tool within customer service workflows enhances operational efficiency, allowing teams to focus on resolving issues rather than sifting through data. Such insights not only improve customer satisfaction but also inform product and service enhancements that align with customer expectations.
Sentiment Analyzer
In the realm of automated complaint analysis, the Sentiment Analyzer plays a crucial role in interpreting customer emotions effectively. This tool leverages advanced natural language processing algorithms to assess the sentiments expressed in customer feedback. By classifying statements as positive, negative, or neutral, businesses can quickly gauge customer satisfaction levels and identify areas needing improvement.
Utilizing a Sentiment Analyzer not only streamlines the process of analyzing large volumes of feedback but also enables companies to respond proactively to customer issues. The accuracy of these tools ensures that even subtle nuances in customer language are recognized, highlighting critical feedback that may otherwise be overlooked. By applying such AI-driven methods, organizations can foster a deeper connection with their customers and enhance their overall service experience, ultimately leading to improved customer loyalty and business growth.
Clarabridge
In the realm of automated complaint analysis, a particular tool stands out for its robust capabilities in processing customer feedback. This tool specializes in extracting insights from vast amounts of customer complaints, using advanced algorithms to identify trends and issues that may otherwise go unnoticed. By focusing on natural language processing and machine learning, it enables organizations to automate the tedious task of analyzing customer sentiment, thus enhancing the decision-making process.
Furthermore, it provides a comprehensive platform where businesses can not only gather complaints but also categorize and prioritize them effectively. This functionality streamlines how companies respond to customer issues, ensuring that significant complaints are addressed promptly. The automation of these processes is crucial, as it leads to better customer satisfaction and ultimately, loyalty. Embracing such a sophisticated tool can profoundly impact an organization’s approach to customer interactions, making automated complaint analysis a game-changer in modern business practices.
Conclusion on Automated Complaint Analysis
Automated Complaint Analysis offers businesses a powerful solution to manage customer feedback efficiently. By harnessing AI technology, organizations can effectively analyze large volumes of complaints, uncovering trends and root causes that may otherwise go unnoticed. This systematic approach not only enhances understanding but also fosters a proactive stance in addressing customer issues.
Furthermore, successful implementation of automated systems enables businesses to allocate resources more effectively, prioritize urgent matters, and tailor responses to individual customer needs. As the landscape of customer service continues to evolve, embracing AI-driven solutions will remain essential for maintaining customer satisfaction and loyalty in a competitive market.