In an era where customer expectations are higher than ever, organizations find themselves seeking innovative solutions to enhance interactions. Sentiment Analysis Automation has emerged as a pivotal tool that enables call centers to gauge customer emotions in real-time. By analyzing customer conversations systematically, businesses can better understand sentiments and responses, leading to more effective engagement strategies.
This technology streamlines the process of gaining insights from customer interactions, facilitating a proactive approach to service. With accurate sentiment detection, call centers can tailor responses to improve satisfaction and loyalty. As organizations harness this power, the potential for transforming customer experience in real-time becomes not just a possibility but a necessity in today's competitive landscape.
Analyze qualitative data. At Scale.
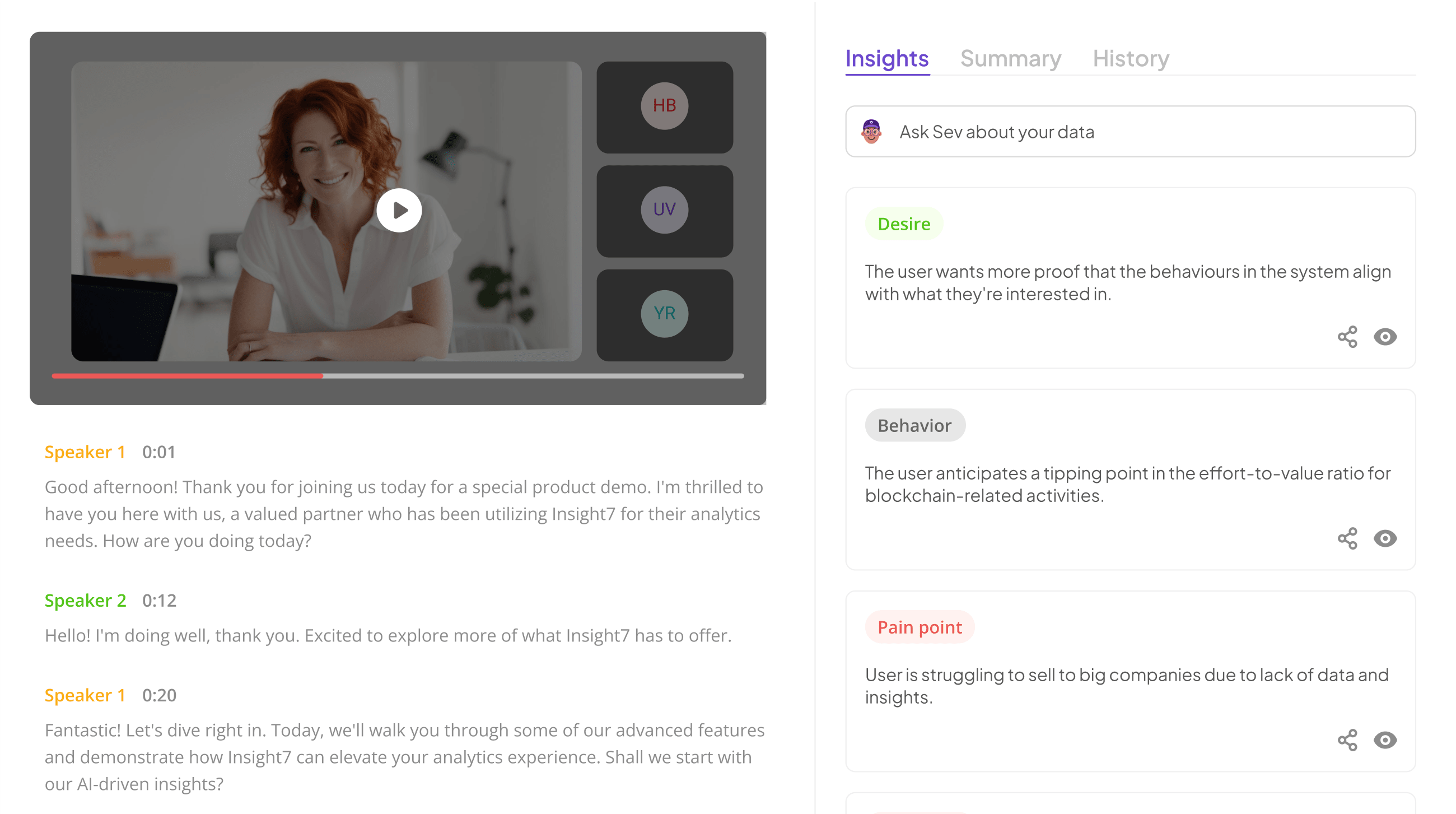
Importance of Sentiment Analysis Automation for Real-Time Customer Interaction
Sentiment analysis automation plays a crucial role in enhancing real-time customer interaction. By swiftly analyzing customer sentiments during calls, this technology enables organizations to understand customer emotions and responses instantly. The ability to interpret customer feelings enhances engagement, allowing representatives to address concerns effectively and improve service quality. When agents are equipped with real-time insights into customer moods, they can tailor their approach, leading to a more personalized interaction.
Moreover, sentiment analysis automation streamlines the process of gathering and reviewing feedback. It eliminates delays associated with traditional manual analysis, allowing businesses to respond proactively to emerging trends. With prompt detection of negative sentiments, organizations can mitigate issues before they escalate, ensuring higher customer satisfaction. As the landscape of customer support continues to evolve, investing in sentiment analysis technologies is vital for maintaining competitive advantage and fostering customer loyalty.
Enhancing Customer Experience with Automation
Automation is revolutionizing customer interactions by streamlining processes and enhancing the overall experience. With sentiment analysis automation, businesses can better understand customer emotions in real time, allowing for immediate responses to their needs. This proactive approach not only fosters customer satisfaction but also builds long-term loyalty through personalized interactions.
By implementing sentiment analysis automation, call centers can transform how they manage inquiries and feedback. Detecting key emotional cues enables agents to tailor their responses, addressing concerns effectively. This capability leads to improved communication, faster resolution times, and an overall elevated customer experience. Ultimately, embracing automation enhances operational efficiency, making every interaction more meaningful and productive.
Key Metrics for Sentiment Analysis in Call Centers
Key metrics play a crucial role in evaluating the success of sentiment analysis automation within call centers. Understanding these metrics allows organizations to monitor customer interactions effectively and adjust their strategies accordingly. One essential metric is the sentiment score, which quantifies the emotional tone of customer interactions. A high sentiment score typically indicates satisfied customers, while a low score can signal underlying issues that need to be addressed.
Another vital metric is the call resolution rate. This measures the effectiveness of agents in resolving customer inquiries during the first contact. Monitoring this metric can highlight training needs and process improvements. Additionally, tracking customer feedback trends can provide insights into recurring questions or concerns, allowing organizations to refine their training programs and enhance overall service quality. By focusing on these key metrics, call centers can harness the power of sentiment analysis automation to improve both customer satisfaction and operational efficiency.
Implementing AI-Powered Sentiment Detection Systems
Integrating AI-Powered Sentiment Detection Systems involves several key steps that streamline the process of understanding customer emotions during interactions. First, identifying call center needs is essential; this helps to determine what insights are necessary for effective sentiment analysis automation. Evaluate specific challenges, such as call volume and customer demographics, to tailor the approach.
Next, selecting the right AI tools plays a critical role in successfully implementing the system. Various options are available, but choosing a tool that aligns with your unique requirements ensures optimal performance. Following this, training and deployment are crucial phases where staff are educated on the new system, ensuring an easier transition and better utilization of the tool.
Once the system is in place, it's vital to ensure accuracy and reliability. Continuous monitoring helps to refine the sentiment analysis automation and adjust parameters, allowing for improved interpretations of customer feedback over time. This iterative process creates a robust framework for understanding customer interactions in real-time.
Extract insights from interviews, calls, surveys and reviews for insights in minutes
Steps to Integrate Sentiment Analysis Automation
Integrating sentiment analysis automation into call centers involves several vital steps to ensure successful implementation. First, it is essential to identify the specific needs of the call center, such as understanding what types of customer interactions are most critical. Tailoring the sentiment analysis tools to these unique business requirements will enhance the insights gathered from customer interactions.
Next, selecting the right AI tools is crucial for positive outcomes. Research various options and choose platforms like AI-powered text analytics or voice sentiment analysis tools that align with your objectives. Once you have selected the tools, it's important to focus on training and deployment, ensuring staff is adequately trained to use the technology effectively. This holistic approach allows for the accurate gathering of sentiment data, which is crucial for improving customer interactions and overall satisfaction. By following these steps, call centers can seamlessly integrate sentiment analysis automation and enhance their service delivery.
- Identifying Call Center Needs
Understanding the unique requirements of a call center is essential for effective sentiment analysis automation. To start, assessing the current challenges faced by customer service representatives (CSRs) can reveal opportunities for improvement. Identifying the specific types of interactions needing attention allows for targeted enhancements in training and support processes. For example, analyzing patterns in customer inquiries can help identify common topics, enabling CSRs to prepare more effectively.
Next, evaluating available data can uncover how sentiment analysis automation can streamline operations. This approach helps reduce the time spent manually grading calls while providing valuable insights into customer interactions. By focusing on the key metrics that matter most, organizations can better align their training programs and improve overall service quality. The journey of successfully integrating sentiment analysis automation begins with these foundational steps, ensuring that the technology implemented meets the true needs of the call center environment.
- Selecting the Right AI Tools
When considering Sentiment Analysis Automation for your call center, selecting the right AI tools is crucial to achieving effective customer interactions. Begin by assessing your specific needs based on the nuances of your customer base and the volume of calls you manage. Different AI tools offer varied functionalities; therefore, understanding what emotions or sentiments are most relevant to your conversations will guide your selection process.
Next, evaluate the capabilities and features of various AI tools available on the market. For instance, some solutions excel in real-time sentiment detection, while others may provide comprehensive analytics. Choose a tool that integrates seamlessly with your existing systems and allows for easy customization to suit your operational goals. Additionally, consider the scalability of the AI solutions, as your needs may evolve over time. Ensuring that your selected tools align with your long-term strategy will enable your call center to harness the full potential of sentiment analysis automation.
- Training and Deployment
Training AI models to perform sentiment analysis automation involves several key steps to ensure seamless deployment in call centers. Initially, data collection is essential; large sets of labeled conversations can significantly enhance model accuracy. These transcripts provide a diverse range of interactions, allowing the AI to learn different sentiment expressions effectively.
Once data is collected, the next step involves training the model using advanced algorithms. Techniques such as supervised and unsupervised learning can be employed to fine-tune algorithm accuracy. Post-training, deploying the AI requires integrating it into existing call center systems. This ensures real-time sentiment detection alongside regular customer interactions, allowing representatives to respond more effectively to customer moods and concerns. Regular updates and maintenance are crucial for continued accuracy as customer language and preferences evolve. By following these guidelines, sentiment analysis automation can transform customer service dynamics, leading to improved experiences and insights.
Ensuring Accuracy and Reliability with Sentiment Analysis Automation
Ensuring accuracy and reliability with sentiment analysis automation involves multiple key elements that enhance customer engagement. The first step is implementing advanced algorithms that analyze a customer's tone, word choice, and emotional cues during interactions. Through continuous training and fine-tuning of these algorithms, organizations can ensure that they respond appropriately to customer sentiments, leading to improved satisfaction rates.
Moreover, real-time feedback mechanisms are crucial for maintaining the effectiveness of sentiment analysis automation. By utilizing customer interactions as learning opportunities, AI systems can adapt and refine their responses based on actual feedback. This iterative process fosters greater trust and reliability, allowing customer service representatives to engage more effectively with clients. Ultimately, successful sentiment analysis embodies a commitment to accuracy, as organizations strive to translate nuanced emotions into actionable insights.
Top AI Tools for Sentiment Detection in Call Centers
In the realm of customer service, leveraging top AI tools for sentiment detection can significantly enhance call center operations. These tools utilize advanced algorithms to analyze spoken language, allowing businesses to gauge customer emotions in real-time. Effective sentiment analysis automation enables agents to respond appropriately, fostering a more personalized and positive customer experience.
Several leading AI tools stand out for their capabilities in sentiment detection. The IBM Watson Tone Analyzer excels in identifying emotional tones during conversations. Meanwhile, the Google Cloud Natural Language API offers comprehensive sentiment scoring, giving a clearer picture of customer satisfaction levels. Microsoft Text Analytics further enriches this suite with its robust text analysis features, making them invaluable resources for modern call centers. By integrating these technologies, businesses can transform customer interactions into insightful experiences, ultimately driving brand loyalty and client retention.
insight7
In the realm of customer service, optimizing interaction through Sentiment Analysis Automation can profoundly enhance engagement. The importance of understanding customer sentiments lies not just in reaction, but in proactive communication. Implementing systems that can analyze customer conversations in real-time enables representatives to pick up on emotions and adjust their responses accordingly. This capability fosters a more personalized customer experience, as understanding the underlying emotions can lead to more meaningful interactions.
There are several steps to effectively implement Sentiment Analysis Automation within call centers. First, identifying the specific needs of the call center is crucial for tailoring the solution. Next, selecting appropriate AI tools ensures the necessary capabilities are available for accurate analysis. Finally, effective training and deployment of these systems solidify their reliability and enhance operational efficiency. By incorporating these elements, businesses can not only keep pace with customer expectations but also strive to exceed them, creating loyal clientele.
IBM Watson Tone Analyzer
In the context of AI-Powered Call Center Sentiment Detection, the use of advanced tone analysis technology has significant implications for real-time customer interaction. By integrating an effective tone analysis tool, businesses can gain deeper insights into customer emotions and sentiments expressed during calls. This technology automates sentiment analysis, enabling call centers to quickly assess how customers feel about products or services.
The tone analyzer evaluates verbal cues and linguistic patterns, providing actionable insights that help businesses tailor their responses. By identifying emotional states such as happiness, frustration, or confusion, call centers can adjust their strategies and improve customer experiences. This proactive approach not only enhances customer satisfaction but also fosters better communication, ultimately leading to higher retention rates. The ability to respond appropriately and promptly to customer needs makes this technology invaluable for modern call center operations.
Google Cloud Natural Language API
The Google Cloud Natural Language API provides powerful tools for sentiment analysis automation, enabling call centers to understand customer emotions in real time. By integrating this API, businesses can process vast amounts of text data from customer interactions, allowing them to categorize sentiments as positive, negative, or neutral effectively. This capability enhances not only monitoring efforts but also optimizes customer engagement strategies.
To fully utilize sentiment analysis automation through this API, follow these essential steps:
- Data Input: Feed in transcriptions from customer calls for analysis.
- Sentiment Scoring: The API automatically assigns sentiment scores, highlighting areas that need attention.
- Actionable Insights: Utilize generated data to inform training and coaching strategies in your call center.
By implementing these steps, call centers can enhance their responsiveness and improve overall customer satisfaction, ensuring a more personalized interaction approach.
Microsoft Text Analytics
Microsoft Text Analytics provides essential tools for sentiment analysis automation, enriching real-time customer interactions in call centers. This technology leverages natural language processing to evaluate the emotions and sentiments expressed in customer conversations. By automating sentiment detection, organizations can swiftly gauge customer feelings, driving more informed and responsive service initiatives.
Understanding patterns and trends in customer feedback is crucial. With robust project frameworks, users can easily compile and analyze data from numerous calls. The ability to visualize insights allows companies to detect issues swiftly, enabling proactive measures to enhance customer satisfaction. By automating sentiment analysis, teams can focus on quality interactions rather than manual data processing. As businesses continue to embrace AI, Microsoft Text Analytics stands out, ensuring that companies can maintain a clear understanding of their customers' sentiments in real time.
Conclusion: The Future of Sentiment Analysis Automation in Real-Time Interactions
As we look ahead, the future of sentiment analysis automation in real-time interactions promises significant advancements. Companies are increasingly recognizing the value of immediate insights, which can transform customer engagement. By automating sentiment detection, businesses can quickly understand customer emotions, allowing them to respond more effectively during interactions.
Moreover, the ongoing development in AI technologies will enhance the accuracy and efficiency of sentiment analysis automation. As these tools evolve, organizations will have the opportunity to refine their communication strategies, thereby creating more personalized customer experiences. Investing in such innovations will be crucial for staying competitive in a rapidly changing market.
Analyze qualitative data. At Scale.
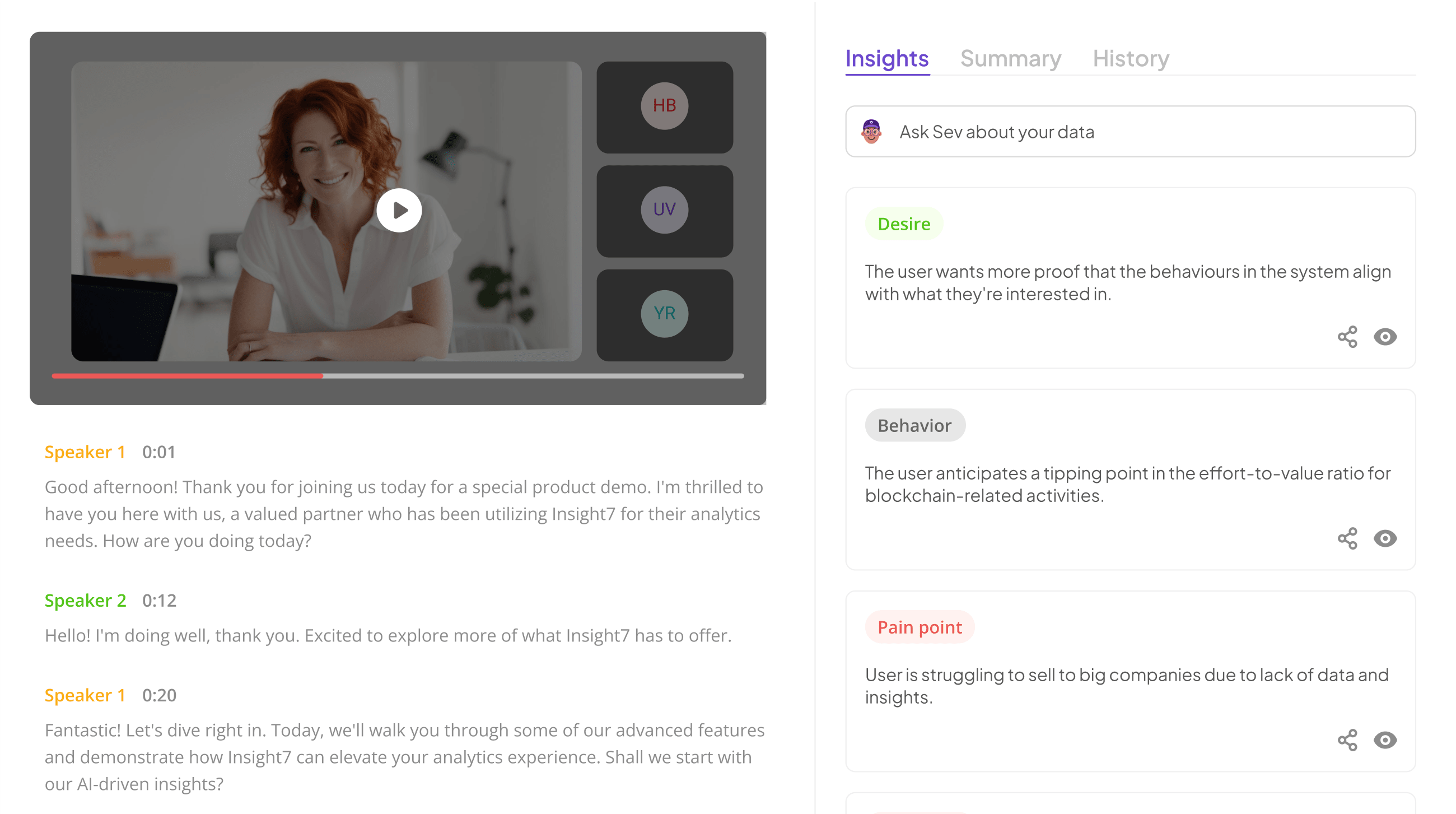