AI Internal And External Validity Assessment Tools
-
Bella Williams
- 10 min read
AI Validity Tools are essential for anyone looking to evaluate the reliability and effectiveness of artificial intelligence systems. In a rapidly evolving technological landscape, understanding these tools is crucial to ensure AIโs internal and external validity. This section will explore the importance of such tools in assessing the soundness of AI models and their capacity to perform in diverse real-world environments.
Effective AI Validity Tools provide a framework for examining how well AI systems deliver reliable outcomes. By deploying these tools, organizations can ensure that their AI solutions are not only accurate but also adaptable to changing conditions. This exploration offers valuable insights into the importance of maintaining integrity in AI applications while navigating both internal assessments and external validations.
Analyze & Evaluate Calls. At Scale.
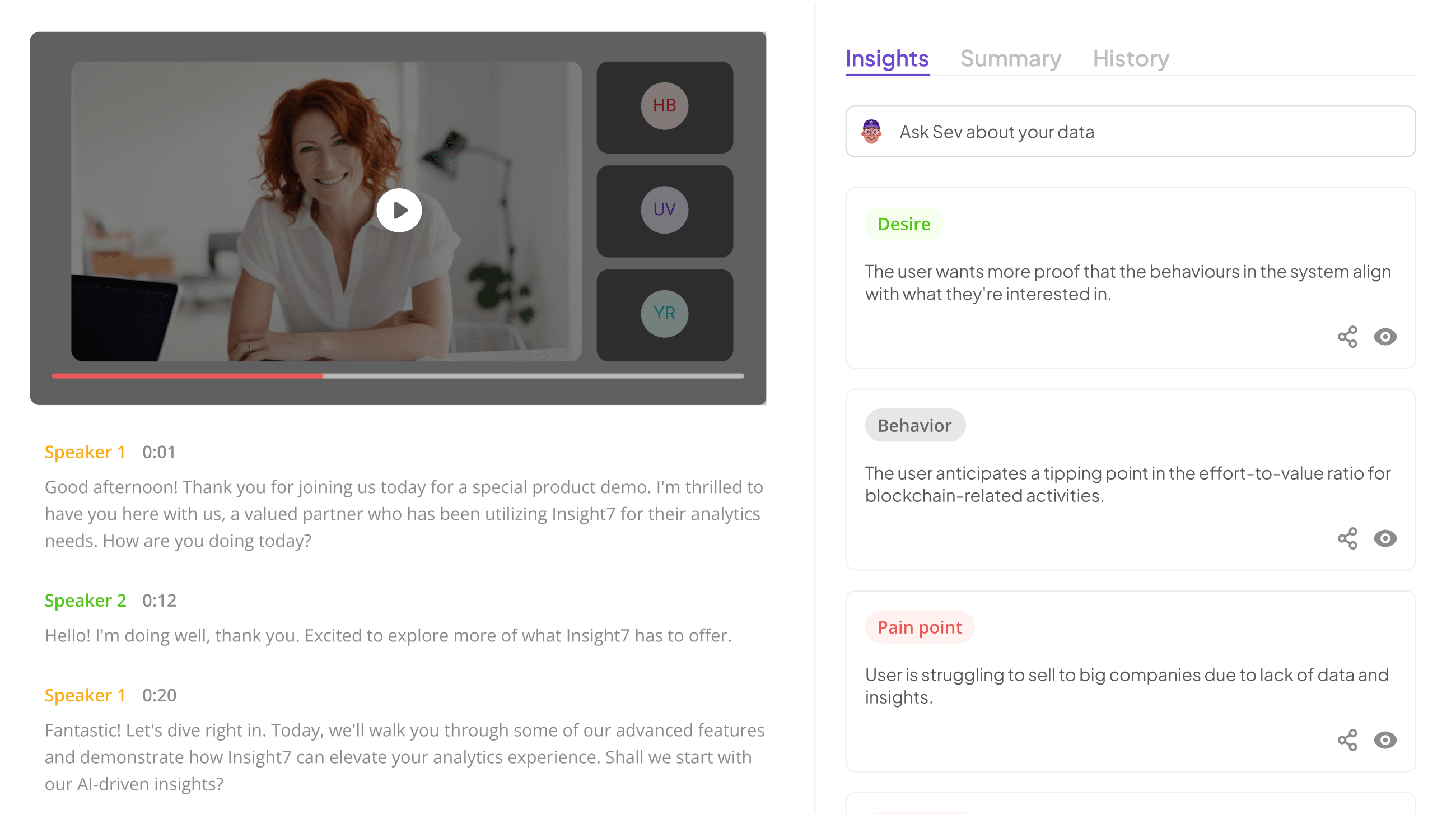
Understanding the nuances of AI systems requires effective assessment tools. Among the most crucial are AI Validity Tools that ensure both internal coherence and external applicability. In this blog post, we will delve into these tools and highlight their significance in the AI landscape.
Understanding the nuances of AI systems requires effective assessment tools. Among these, AI Validity Tools are essential for evaluating both internal coherence and external applicability. These tools serve a vital function by ensuring that AI models remain accurate and reliable across various scenarios. A comprehensive assessment can help identify any biases or inconsistencies, thus giving stakeholders confidence in the outputs they generate.
The significance of these tools extends beyond immediate functionality. By utilizing appropriate AI Validity Tools, organizations can ensure that their models are not only performing well under controlled conditions but also thriving in real-world applications. This balance of internal consistency and external adaptability is crucial in today's AI-driven society, enabling developers to build intelligent systems that meet the diverse needs of users. Ultimately, understanding and implementing these tools will lead to more trustworthy and effective AI solutions.
Exploring AI Internal Validity Tools
Internal validity is crucial for ensuring that AI models deliver consistent results under specific conditions. By utilizing AI Validity Tools, we can maintain the integrity of assessments and foster a trustworthy model evaluation process. These tools help pinpoint biases and errors, enabling developers to create more reliable outcomes. A key aspect of internal validity lies in the thorough examination of the data and algorithms used in AI systems, ensuring that results aren't affected by extraneous variables.
To implement AI Validity Tools effectively, it is essential to follow systematic steps. First, recognize potential internal threats that may compromise data accuracy or algorithm performance. Next, it is vital to employ these tools for rigorous testing of datasets and algorithms to evaluate their reliability. By concentrating on these core areas, organizations can enhance their model's robustness, leading to more reliable outputs in AI applications. This focused approach to internal validity is critical for building trust and ensuring impactful AI solutions.
Internal validity focuses on ensuring that ai models produce reliable outcomes under consistent conditions. Here, AI Validity Tools play a pivotal role in maintaining the integrity of model assessments.
Internal validity is essential for ensuring that AI models achieve consistent and reliable outcomes. It emphasizes the importance of testing models under identical conditions to establish trust in their performance. AI Validity Tools are instrumental in this process, as they help maintain the integrity of model assessments. By systematically evaluating the models, these tools identify potential flaws that could compromise results, ensuring that the technology operates as intended.
Utilizing AI Validity Tools involves examining critical elements like bias detection, data safety, and model efficiency. The first step is to identify internal threats that might influence outcomes negatively. Following this, the tools conduct precise tests to evaluate the performance of datasets and algorithms accurately. By ensuring thorough testing and validation, developers can enhance not only the reliability of models but also instill confidence in their use across various applications. This structured approach is crucial for building robust AI systems that stakeholders can depend on.
Importance of AI Validity Tools in Model Integrity
AI Validity Tools play a pivotal role in ensuring model integrity, significantly impacting the reliability and effectiveness of AI systems. These tools help detect biases within models, ensuring they function consistently across different scenarios. By implementing AI Validity Tools, organizations can maintain focus on crucial aspects such as data safety and output accuracy, which ultimately influences decision-making processes.
Understanding the importance of AI Validity Tools is paramount for organizations seeking to enhance their AI implementations. They ensure the robustness of models by conducting thorough assessments of both inputs and outputs, thereby reinforcing user trust. Furthermore, these tools provide a structured approach to recognize and rectify potential weaknesses within AI models. Consequently, integrating AI Validity Tools not only improves model performance but also fosters a culture of accountability and transparency in AI development.
Exploring how validity tools help in maintaining model consistency by checking for biases, data safety, and overall efficiency in AI outputs.
AI Validity Tools play an essential role in ensuring the consistency of AI models by systematically checking for potential biases, maintaining data safety, and assessing overall efficiency in outputs. To achieve model integrity, these tools enable practitioners to evaluate the reliability of AI systems. Incorporating such assessments helps identify discrepancies and improve accountability, crucial for stakeholders relying on AI-driven insights.
Bias detection is a vital aspect of these tools. Ensuring that models do not propagate existing biases helps create fairer outcomes. Additionally, data safety measures safeguard sensitive information, protecting users from breaches. Furthermore, evaluating the overall efficiency of AI outputs ensures that models deliver not only accurate results but also do so in an optimized manner. Together, these elements contribute to a robust framework for maintaining model consistency in the evolving landscape of artificial intelligence.
Steps for Implementing AI Validity Tools
To implement AI Validity Tools effectively, the process begins with clearly identifying internal threats. This involves recognizing potential errors within datasets or algorithms that could distort outcomes. These might include issues such as biases, inconsistencies, or data integrity problems that could compromise the model's reliability.
Next, utilizing AI Validity Tools for testing is paramount. Leverage these tools to conduct thorough evaluations on datasets and algorithms, focusing on their performance under consistent conditions. This testing phase helps ensure the tools thoroughly assess model reliability and uphold the integrity of outputs. Regularly validating processes will enhance the overall applicability of AI systems, allowing them to adapt to real-world scenarios effectively while minimizing errors. By following these steps, you can foster a robust framework that ensures the reliability and accountability of AI implementations.
- Identify Internal Threats
To effectively identify internal threats within AI systems, a structured approach is essential. Internal threats often arise from errors in data or algorithms, which can significantly skew results. First, scrutinizing data inputs for accuracy is crucial, as flawed data can lead to incorrect conclusions. Secondly, examining the algorithms themselves will reveal biases that might compromise internal validity.
Utilizing AI Validity Tools enhances this process by providing comprehensive assessments that spot inconsistencies early on. Regular testing of these tools against current data sets ensures the reliability of the AI models. By being proactive, organizations can mitigate risks associated with internal threats, thus improving overall performance. Adopting this methodology fosters an environment of continual improvement and strengthens the integrity of AI systems.
Recognize potential errors within the data or algorithm that may skew results.
Errors in data or algorithms can significantly impact the reliability of AI results. Identifying these potential errors is critical for upholding the integrity of AI findings. Common issues include biases within the dataset, which can arise from non-representative sample selection or flawed data collection methods. Additionally, algorithms may include inherent biases due to their training processes, leading to skewed outputs. Recognizing and addressing these factors is essential for ensuring that the AI system generates valid and applicable results.
Furthermore, external factors such as changing environments or evolving datasets can also introduce errors. When utilizing AI Validity Tools, it is vital to conduct thorough assessments that identify these discrepancies. By staying vigilant and employing effective evaluation measures, engineers can mitigate risks associated with biased data and flawed algorithms, enabling more reliable AI outcomes. A comprehensive approach to recognizing potential errors enhances the overall validity of AI systems, ensuring they perform accurately across varied contexts.
- Utilize AI Validity Tools for Testing
Testing the efficacy of AI systems is crucial for achieving reliable outcomes. Utilizing AI Validity Tools for testing not only ensures that algorithms function consistently but also verifies that they are applicable to real-world scenarios. These tools allow teams to conduct systematic evaluations of models, identifying any discrepancies that may arise during operations.
To effectively utilize these tools, it's important to follow a structured approach. Start by identifying internal threats, such as biases in the dataset or flaws in the algorithm. Next, apply AI Validity Tools to perform rigorous tests on these datasets and algorithms, measuring their reliability and performance. This methodology can significantly enhance the trustworthiness of AI outputs. Employing this framework creates a more robust evaluation process, thereby solidifying the foundational integrity of AI systems in diverse applications. By prioritizing these steps, organizations can achieve a deeper understanding of their AI models and ensure more accurate, dependable results.
Leverage tools to conduct tests on data sets and algorithms to evaluate reliability.
To evaluate reliability, it is essential to conduct systematic tests on data sets and algorithms. AI Validity Tools enable organizations to assess the effectiveness of machine learning models, ensuring they operate as intended. By utilizing these tools, teams can effectively analyze data accuracy, identify biases, and ensure consistency across models. When assessments are properly performed, organizations gain confidence in their AI systems, paving the way for improved decision-making.
There are several key actions to take when employing AI Validity Tools. First, initiate thorough testing on the data sets to uncover any inconsistencies or errors that could impact outcomes. Next, conduct algorithm evaluations against predefined metrics that reflect performance reliability. Finally, incorporate feedback loops to continuously enhance models based on test results. Such structured approaches help organizations maintain the integrity and usability of their AI innovations.
[hfe_template id=22694]Navigating AI External Validity Tools
Navigating AI External Validity Tools is essential for ensuring that AI systems are effective in diverse real-world applications. These tools assess how well AI models transfer knowledge beyond their training environments. Understanding their functionality helps organizations adapt AI technologies to new contexts, ultimately enhancing their relevance and performance.
One of the primary objectives of AI External Validity Tools is to evaluate how models respond to varying datasets and conditions. Some noteworthy tools in this arena include insight7, known for its comprehensive validation capabilities; Open AI Gym, which assesses model performance across simulated environments; and TensorFlow Extended, providing end-to-end validation mechanisms. By utilizing these tools, organizations can confidently implement AI solutions tailored to specific real-world challenges, ensuring that their investments yield meaningful results across different applications.
External validity ensures that AI systems can perform well in varied, real-world situations. AI Validity Tools designed for this purpose are essential for understanding and predicting broader applicability.
AI systems must demonstrate external validity to succeed in diverse real-world contexts. This capability ensures that the models not only operate well in controlled environments but also adapt effectively to varying conditions and challenges. AI Validity Tools designed for external assessment are pivotal for measuring this adaptability. They provide insights into how well AI systems can transform learned knowledge into practical solutions, aligning with real-world expectations and user needs.
One key aspect of these tools is their ability to evaluate different external datasets to predict broader applicability. When used effectively, AI Validity Tools can identify potential weaknesses or biases that may emerge when models interact with unfamiliar data. Additionally, they help practitioners understand how contextual factors can impact model performance, ultimately influencing deployment strategies across various sectors. Ensuring that AI systems are robust in varied scenarios reinforces their reliability and utility in real-world applications.
The Role of AI Validity Tools in Real-World Applications
AI Validity Tools play a crucial role in real-world applications by bridging the gap between theoretical modeling and practical functionality. These tools evaluate how well AI systems perform in diverse environments, ensuring reliability when applied to external datasets. They enable businesses to understand the adaptability of their models, which is essential for achieving desired outcomes in unpredictable conditions.
Moreover, AI Validity Tools facilitate the identification of limitations and biases that may arise when models are deployed. This not only enhances the overall integrity of AI applications but also bolsters user trust. By implementing these tools, organizations can continuously monitor and assess the performance of their AI systems, allowing for iterative improvement. In this way, AI Validity Tools serve as indispensable assets in navigating the complexities of real-world scenarios, ultimately leading to more effective and trustworthy AI solutions.
Detailed insight into how these tools assess the adaptability of AI models to different environments and external datasets.
AI Validity Tools play a critical role in evaluating the adaptability of AI models as they operate across various environments and interact with external datasets. These tools assess how well an AI model can generalize beyond its training data, ensuring that it maintains accuracy and relevance in real-world applications. By scrutinizing data shifts and contextual differences, these tools help identify performance variances, enabling developers to fine-tune their models accordingly.
To effectively assess adaptability, AI Validity Tools typically employ several methods. First, they analyze the model's performance metrics in diverse settings, which can highlight its strengths and weaknesses. Next, they perform stress tests using varied datasets to simulate different scenarios. Finally, these tools monitor feedback loops, continuously refining the model based on real-time data inputs. This ongoing analysis empowers AI developers to create robust models that can thrive in unpredictable environments and deliver reliable outcomes.
Notable AI Validity Tools for External Assessment
Notable AI Validity Tools for External Assessment enable a deeper understanding of how AI models perform in real-world scenarios. These tools are essential for evaluating the adaptability and generalizability of AI systems across diverse environments. By utilizing such tools, stakeholders can gain valuable insights into the reliability and effectiveness of their AI applications in practical settings.
Some of the notable AI validity tools include insight7, which excels in validating AI processes for numerous applications. Open AI Gym offers a simulated environment to assess model performance under varying conditions, allowing users to identify strengths and weaknesses. TensorFlow Extended provides a robust framework to validate AI model workflows, ensuring they can operate effectively despite dynamic changes. Engaging with these AI validity tools enhances confidence in AI outputs and supports informed decision-making for stakeholders aiming for excellence in their AI initiatives.
- insight7
Understanding the significance of insight7 in relation to AI Validity Tools is essential for anyone invested in the advancement of AI technologies. This platform serves as a comprehensive solution for assessing both internal and external validity in AI systems. By providing a user-friendly interface, it allows organizations to analyze customer interactions seamlessly, turning complex data into actionable insights.
Insight7 specifically addresses the challenges seen in traditional data analysis methods, which often fail to keep pace with the enormous volume of customer signals generated today. It streamlines the process of translating insights into practical business actions, thus enhancing collaboration and decision-making across teams. Furthermore, it focuses on problem-solving by facilitating dialogues that drive engagement, rather than merely responding to inquiries. This robust approach ensures that organizations can harness the full potential of their data, securing a competitive edge in the rapidly evolving landscape of AI technology.
Leading the market in effective validation of AI for diverse applications.
The realm of AI is expanding rapidly, making the effective validation of AI applications essential. Leading the market in effective validation of AI for diverse applications begins with understanding the role of AI Validity Tools. These tools allow developers to rigorously assess the performance of AI systems, ensuring that they provide consistent and reliable outcomes across various scenarios.
In diverse applications, AI Validity Tools help identify potential biases and inconsistencies within algorithms. By conducting thorough evaluations, these tools enable organizations to adapt and fine-tune their AI models for real-world settings. This adaptability is crucial for ensuring that AI systems can be trusted in critical sectors like healthcare, finance, and autonomous driving. The ongoing commitment to validating AI technology not only enhances model integrity but also fosters greater transparency, ultimately leading to more robust and user-friendly AI applications.
- Open AI Gym
Open AI Gym is a pivotal resource for those engaged in the development and evaluation of artificial intelligence systems. This platform provides a versatile environment for simulating various tasks, enabling users to test the efficacy of their models against a range of challenges. By offering diverse scenarios, it allows for comprehensive assessments, ensuring that AI systems perform as intended across different conditions.
The significance of Open AI Gym extends to its role in validating AI models, which is essential to understanding internal and external validity. The platform facilitates experimentation in a controlled setting, allowing researchers and developers to iterate on their designs and achieve better performance. This capacity to simulate real-world applications enhances the credibility of AI Validity Tools, ultimately leading to more robust and applicable intelligent systems.
Specializes in assessing model performance across simulated environments.
In assessing model performance across simulated environments, specialized methodologies are essential. These approaches allow for thorough evaluation of AI models in controlled settings, ensuring that they can be optimized before deployment. Utilizing simulation environments enables stakeholders to test various scenarios, which helps in identifying strengths and weaknesses in performance. With AI Validity Tools, analysts can simulate different conditions and observe how models respond, providing valuable insights into their reliability.
Moreover, this specialized assessment facilitates comparisons across various model architectures and configurations. By creating a benchmark within a simulated framework, organizations can quantitatively measure performance indicators such as accuracy, precision, and responsiveness. This iterative process ultimately improves the robustness of AI models, ensuring they are equipped to address real-world challenges post-launch. Thus, incorporating tools that specialize in these assessments empowers developers to create more effective and adaptable AI solutions.
- TensorFlow Extended
TensorFlow Extended (TFX) is a robust framework designed for managing machine learning workflows in production environments. It offers tools essential for building and validating AI models effectively. The framework not only provides capabilities that enhance the development process but also integrates essential AI validity tools for assessing model performance and adaptability.
One of the key advantages of TFX is its comprehensive suite of components that streamline model building, evaluation, and deployment. For example, TFX facilitates data validation, ensuring that datasets are consistent and free from bias, which is crucial for maintaining internal validity. Moreover, it supports model validation processes that simulates real-world scenarios, enhancing external validity. By utilizing TensorFlow Extended, organizations can ensure their AI systems are not only effective in controlled conditions but can also adapt to the complexities of real-world applications, thereby strengthening overall AI validity.
Offers end-to-end tools for validating AI model workflows in dynamic conditions.
In the realm of artificial intelligence, it is essential to ensure that model workflows are robust and adaptable, particularly in dynamic conditions. This is where end-to-end tools for validating AI model workflows become invaluable. These tools not only streamline the process of assessment but also provide comprehensive insights into how models behave under varying scenarios. By employing these solutions, teams can effectively monitor AI performance, enabling quick adjustments when needed.
Moreover, these end-to-end tools facilitate a deeper understanding of model reliability. They allow users to gain insights from complex data sets and identify potential biases that may affect outcomes. By incorporating feedback loops and iterative testing models, organizations can enhance their AI validity, ensuring that systems work accurately across different environments. With the right AI Validity Tools, businesses can confidently deploy machine learning applications, knowing that their workflows are rigorously validated against real-world challenges.
Conclusion: The Future of AI Validity Tools
As we look toward the future, the role of AI Validity Tools in machine learning development will undoubtedly become more pronounced. With the increasing integration of AI into diverse applications, the need for these tools to ensure both internal and external validity will be paramount. The ongoing advancements in technology will lead to more sophisticated tools that can effectively assess and validate AI models, enhancing their reliability and applicability across different fields.
Moreover, as organizations become more data-driven, the demand for robust AI Validity Tools will grow. Businesses will require these tools not only to ensure model accuracy but also to foster trust among stakeholders. Continuous improvement and innovation in validation techniques will empower engineers and researchers to develop AI systems that are accountable, transparent, and aligned with ethical standards.
AI Validity Tools are foundational to the progress of machine learning applications. As we continue to integrate AI into various sectors, ensuring internal and external validity will remain crucial. By leveraging these tools effectively, we can create systems that are both innovative and reliable.
AI Validity Tools are essential in establishing a solid groundwork for the advancement of machine learning applications. As we embed AI deeper into various industries, the need for both internal and external validity becomes increasingly vital. Internal validity ensures that AI models yield consistent results under controlled conditions. These tools mitigate biases and maintain data integrity, fostering confidence in the outcomes generated by these systems.
External validity assesses how well AI models perform in real-world scenarios. By utilizing AI Validity Tools, organizations can evaluate their models for adaptability and applicability across diverse environments. Integrating these assessments allows for the development of innovative systems that can withstand scrutiny in dynamic contexts. As we continue to explore AIโs potential, the focus on validity tools will guide us in shaping reliable and effective applications that serve users across various domains.