AI for Text Analysis: Tools and Techniques
-
Hello Insight
- 10 min read
Understanding Text Analysis
Text analysis, also known as text mining, involves the process of deriving meaningful information from text. It encompasses various techniques, including natural language processing (NLP), sentiment analysis, and thematic analysis. By leveraging AI, organizations can automate the extraction of insights from customer feedback, interviews, surveys, and other textual data sources, leading to improved decision-making and enhanced customer experiences.
Why Use AI for Text Analysis?
- Efficiency: AI tools can process large volumes of text quickly, saving time and resources compared to manual analysis.
- Accuracy: Advanced algorithms can identify patterns and trends in data that may be overlooked by human analysts, reducing bias and improving the quality of insights.
- Scalability: AI solutions can easily scale to accommodate growing data needs, making them suitable for organizations of all sizes.
- Actionable Insights: By automating the analysis process, businesses can derive actionable insights that inform strategy and drive growth.
Top AI Tools for Text Analysis
1. Insight7
Insight7 is a powerful interview analysis platform designed to help organizations synthesize qualitative data at scale. With its user-friendly interface and robust features, Insight7 enables users to analyze customer interviews, focus groups, and surveys efficiently. Here are some key functionalities:
- Transcription Services: Insight7 offers native transcription capabilities, allowing users to convert audio and video recordings into text with high accuracy (up to 99%). This feature supports multiple languages and can handle various accents, making it ideal for diverse research environments.
- Thematic Analysis: Users can extract themes and insights from transcripts, identifying key pain points, desires, and behaviors expressed by participants. This helps organizations understand customer needs and improve their offerings.
- Project Management: Insight7 allows users to group related files into projects, enabling aggregated analysis across multiple interviews or focus groups. This feature is particularly useful for identifying trends and patterns in customer feedback.
- Journey Mapping: The platform can generate customer journey maps, visualizing the steps customers take and the emotions they experience throughout their interactions with a brand. This helps organizations pinpoint areas for improvement and optimize the customer experience.
- Customizable Reporting: Insight7 enables users to create tailored reports based on their analysis, making it easy to share findings with stakeholders and drive informed decision-making.
To get started with Insight7, you can sign up here for a free trial and experience its capabilities firsthand.
Extract insights from interviews, calls, surveys and reviews for insights in minutes
2. NVivo
NVivo is a qualitative data analysis software that allows researchers to analyze text, audio, video, and image data. It offers a range of features, including:
- Coding and Categorization: Users can code text data to identify themes and patterns, making it easier to analyze qualitative data.
- Visualizations: NVivo provides various visualization tools, such as word clouds and charts, to help users understand their data better.
- Collaboration: The software supports team collaboration, allowing multiple users to work on the same project simultaneously.
3. MAXQDA
MAXQDA is another popular qualitative data analysis tool that supports text analysis. Key features include:
- Text Analysis Tools: MAXQDA offers various tools for coding, analyzing, and visualizing text data, making it suitable for qualitative research.
- Mixed Methods Support: The software allows users to integrate qualitative and quantitative data, providing a comprehensive view of research findings.
- User-Friendly Interface: MAXQDA is designed to be intuitive, making it accessible for researchers with varying levels of experience.
4. Atlas.ti
Atlas.ti is a qualitative data analysis software that helps researchers analyze text, audio, and visual data. Its features include:
💬 Questions about AI for Text Analysis: Tools and Techniques?
Our team typically responds within minutes
- Coding and Memoing: Users can code data and create memos to capture insights and reflections during the analysis process.
- Network Views: Atlas.ti allows users to create network views to visualize relationships between codes and themes, facilitating deeper analysis.
- Collaboration Tools: The software supports team collaboration, enabling multiple users to work on projects together.
5. Google Cloud Natural Language API
Google Cloud Natural Language API is a powerful tool for analyzing text using machine learning. Key functionalities include:
- Sentiment Analysis: The API can determine the sentiment of a given text, helping organizations gauge customer emotions and opinions.
- Entity Recognition: Users can identify entities (people, places, organizations) mentioned in the text, providing valuable context for analysis.
- Syntax Analysis: The API can analyze the syntax of text, helping users understand the structure and meaning of sentences.
6. IBM Watson Natural Language Understanding
IBM Watson Natural Language Understanding is another AI-powered tool for text analysis. Its features include:
- Emotion Analysis: The tool can analyze text for emotions, helping organizations understand customer feelings and reactions.
- Keyword Extraction: Users can extract keywords from text, making it easier to identify key themes and topics.
- Custom Models: IBM Watson allows users to create custom models tailored to their specific needs, enhancing the accuracy of analysis.
7. MonkeyLearn
MonkeyLearn is a no-code text analysis platform that enables users to analyze text data without programming skills. Key features include:
- Text Classification: Users can classify text data into predefined categories, making it easier to organize and analyze information.
- Sentiment Analysis: MonkeyLearn offers sentiment analysis capabilities, helping organizations understand customer opinions and emotions.
- Customizable Workflows: The platform allows users to create custom workflows for text analysis, streamlining the process.
8. TextRazor
TextRazor is a text analysis API that provides various natural language processing capabilities. Key functionalities include:
- Entity Extraction: The API can extract entities from text, helping organizations identify key topics and themes.
- Sentiment Analysis: TextRazor offers sentiment analysis features, allowing users to gauge customer emotions.
- Customizable Analysis: Users can customize the analysis process to suit their specific needs, enhancing the accuracy of insights.
Innovative Techniques in Text Analysis AI
Sentiment Analysis in Text Data
Topic Modeling and Classification
Conclusion on Text Analysis AI
AI tools for text analysis have revolutionized the way organizations derive insights from qualitative data. By leveraging these tools, businesses can save time, improve accuracy, and make data-driven decisions that enhance customer experiences. Insight7 stands out as a powerful platform for analyzing interviews and focus groups, offering a range of features that streamline the analysis process. Whether you’re looking to improve customer satisfaction, optimize marketing strategies, or drive product innovation, the right text analysis tool can help you achieve your goals. To explore the capabilities of Insight7, sign up here for a free trial and start transforming your qualitative data into actionable insights.
Analyze & Evaluate Calls. At Scale.
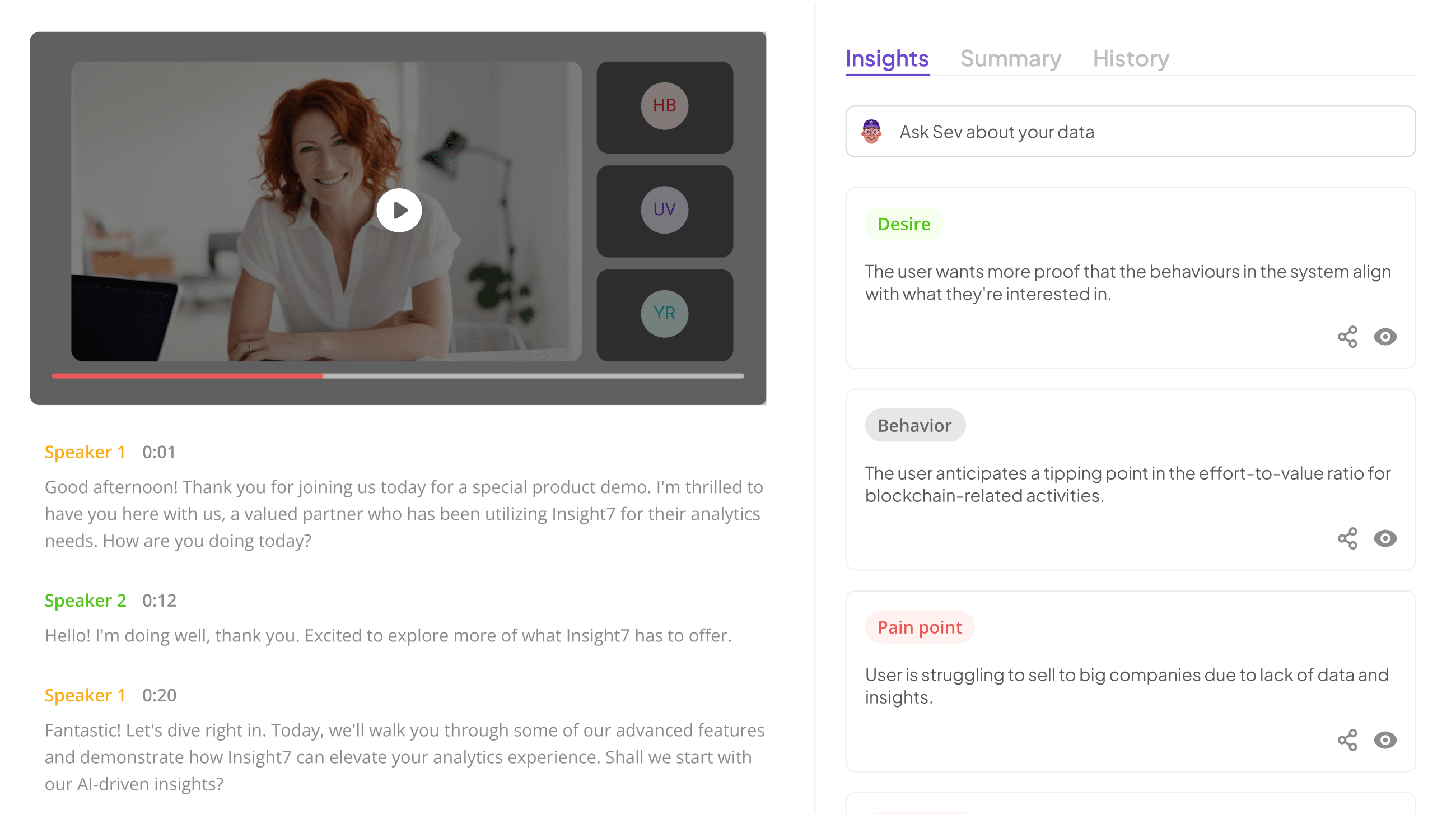
💬 Questions about AI for Text Analysis: Tools and Techniques?
Our team typically responds within minutes