AI Analytics Tools Features And Comparisons
-
Hello Insight
- 10 min read
In the rapidly evolving landscape of modern business, AI analytics comparison has become crucial for organizations seeking to harness data effectively. Business leaders increasingly rely on AI analytics tools to uncover actionable insights that drive strategic decisions and improve customer engagement. With the plethora of available options, understanding the features of these tools has become essential for making informed choices.
By comparing various AI analytics platforms, businesses can identify solutions that align with their specific needs. Factors such as data integration capabilities, real-time reporting, and user-friendly interfaces play significant roles in this evaluation. This document aims to provide a detailed analysis of the key features and functionalities of prominent AI analytics tools on the market today, assisting businesses in their quest for the ideal analytics solution.
Analyze & Evaluate Calls. At Scale.
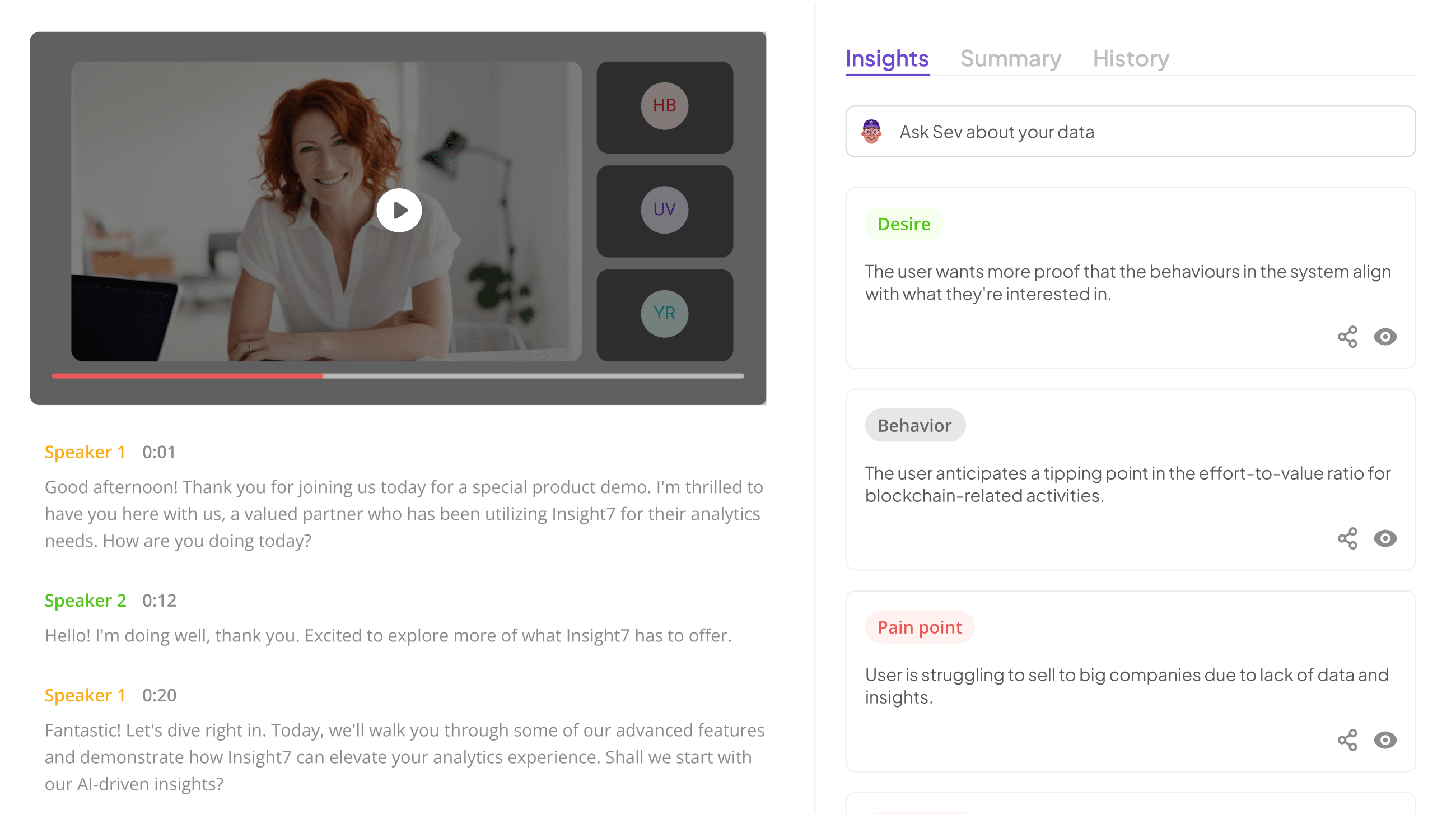
Understanding AI Analytics Tools Features
Understanding the features of AI analytics tools is crucial for businesses aiming to leverage data effectively. Each tool provides unique functionalities that can greatly enhance data analysis and decision-making processes. Key features to consider include automated data processing, real-time analytics, and customizable dashboards. Knowing what each tool offers allows organizations to select the solution that best fits their requirements.
AI analytics comparison reveals varying capabilities among tools. For instance, some platforms excel in visualization while others focus on integration with existing systems. It's essential to evaluate these differences to make informed decisions that align with business goals. Diving into reports generated by these tools provides insights into performance metrics and compliance evaluations. Ultimately, understanding these features ensures that organizations can maximize the benefits of AI analytics tools for effective data-driven strategies.
Key Features in AI Analytics Tools
AI analytics tools come equipped with several key features designed to transform data into actionable insights. Firstly, an intuitive user interface allows users to navigate the tool effortlessly, fostering quicker comprehension of complex datasets. Visualization capabilities stand out, enabling users to create interactive dashboards and charts that depict data trends clearly.
Moreover, advanced algorithms enhance predictive analytics, allowing businesses to foresee market trends and customer behaviors effectively. Real-time data processing is also essential; it ensures that decisions are based on the most current information available. Additionally, integration with various data sources streamlines workflows and facilitates comprehensive analyses.
These features play a crucial role in an AI analytics comparison, helping businesses choose the right tool for their specific needs. Each functionality contributes to delivering reliable insights that drive strategic decisions, ultimately supporting business growth and operational efficiency.
Benefits of Using AI Analytics Tools
AI Analytics Comparison showcases the transformative advantages gained through the use of AI analytics tools. One of the primary benefits is enhanced data interpretation. These tools utilize advanced algorithms to identify patterns and trends that human analysts might overlook, thus enabling businesses to derive actionable insights quickly.
Another significant advantage is increased efficiency. By automating data processing tasks, AI analytics tools free up time for teams to focus on strategy and decision-making. Additionally, they facilitate scalability, as businesses can handle larger datasets without compromising on speed or accuracy. Lastly, AI analytics improves forecasting accuracy by synthesizing historical data with predictive analytics, leading to informed strategic decisions. As businesses seek to optimize their operations, understanding the benefits of using AI analytics tools is crucial for staying ahead in competitive markets.
Extract insights from interviews, calls, surveys and reviews for insights in minutes
AI Analytics Comparison: Popular Tools in the Market
In the realm of AI analytics, comparing popular tools available in the market is essential for informed decision-making. Several tools are designed to cater to various business needs, from data visualization to comprehensive analytics solutions. This AI analytics comparison will delve into the specific features, benefits, and use cases of each tool, making it easier for users to understand how they stack up against one another.
For instance, tools like Tableau and Power BI are well-known for their user-friendly dashboards and integration capabilities. Tableau excels in visual storytelling, while Power BI is renowned for its seamless integration with Microsoft products. On the other hand, IBM Cognos offers enterprise-level insights tailored for larger organizations. By examining these tools closely, users can determine which solution aligns best with their operational goals and analytics requirements, ensuring they enhance their decision-making process effectively.
insight7: Leading the Pack
In today's fast-paced business environment, organizations must prioritize their ability to analyze data efficiently, making AI analytics tools indispensable. The focus on AI analytics comparison highlights how leading technologies can significantly enhance data interpretation and strategic decisions. Key players in the market offer innovative features, enabling businesses to make sense of vast amounts of customer insights rapidly.
The standout solution in this landscape is a platform designed for self-service and scalability. It empowers users to analyze customer conversations at scale, enabling swift translation of insights into actionable business strategies. By streamlining collaboration and making insights easily accessible, companies can foster a culture of continuous improvement. This capability allows organizations to not only keep pace with but also lead their competition, ultimately aligning with the needs and expectations of modern consumers. By understanding the features and benefits through an AI analytics comparison, stakeholders can make informed decisions that propel their businesses forward.
- Overview
In the realm of data-driven decision-making, AI analytics tools have become indispensable. These tools empower organizations to distill vast amounts of data into actionable insights rapidly. By facilitating the AI analytics comparison, decision-makers can evaluate various tool features and select the best fit for their specific needs. This section aims to provide clarity on the essential elements that define effective AI analytics tools, helping users understand their functionalities and advantages.
When assessing AI analytics tools, several key aspects emerge. First, ease of use is crucial; the tools should allow users to generate insights without requiring extensive training. Next, data visualization qualitatively matters, enabling teams to interpret complex datasets intuitively. Finally, integration capabilities with existing systems play a significant role, enhancing the overall efficiency of data analysis. By understanding these pivotal features, organizations can make more informed choices in their pursuit of AI-driven analytics.
💬 Questions about AI Analytics Tools Features And Comparisons?
Our team typically responds within minutes
- Key Features and Benefits
AI Analytics Comparison showcases a variety of powerful tools designed to transform data into actionable insights. Key features of these tools generally include user-friendly interfaces, data visualization capabilities, and advanced analytical functions. Such features democratize data access, allowing users across all levels of an organization to engage with analytics without requiring specialized training. This is particularly beneficial for streamlining business processes and making data-driven decisions quickly.
The benefits of using AI analytics tools extend beyond their features. They enhance operational efficiency by automating data processing, identifying trends, and presenting findings in intuitive formats. Organizations can leverage these insights to improve customer experiences, optimize operations, and make informed strategic decisions. Ultimately, AI Analytics Comparison reveals that the right tool can significantly contribute to a company’s success by improving overall decision-making and fostering a data-centric culture.
- Use Cases
Use cases for AI analytics tools demonstrate their practical applications across various industries. Organizations are increasingly utilizing these tools to enhance processes such as quality assurance, customer service, and sales evaluations. The capability to transcribe and analyze interactions at scale allows users to extract critical insights swiftly. This transforms raw data into actionable reports, making it easier for teams to make informed decisions.
For instance, in quality assurance, these tools can evaluate customer interactions against established compliance criteria. They assess factors like problem identification and context collection, ensuring that customer service representatives meet specific benchmarks. By employing AI analytics comparison, businesses can identify which tools best fit their evaluation needs. Whether focusing on compliance or customer engagement, understanding these use cases helps organizations optimize their operations and elevate customer experiences effectively.
Tableau: A Comprehensive Solution
Tableau stands out as a comprehensive solution in the realm of AI analytics comparison. Its intuitive interface allows users, regardless of technical expertise, to interact effectively with data. This accessibility empowers teams across the organization to derive insights quickly, promoting a data-driven culture. With varying functionalities, users can easily create visualizations, dashboards, and reports to communicate findings compellingly.
Key features include robust data connectivity, enabling integration from various sources, and customization options for tailored visualizations. As users explore their data, they can uncover trends and anomalies, fostering informed decision-making. The platform's collaborative capabilities allow teams to share insights seamlessly, enhancing organizational transparency. Additionally, its automated analytics streamlines the process of data interpretation, saving valuable time. In essence, Tableau offers a holistic approach, making it an invaluable tool in the AI analytics toolkit.
Power BI: Integrating with Business Suites
Power BI stands out for its seamless integration with various business suites, enhancing workflow and data analysis processes. By facilitating connections with tools such as Microsoft Excel and SharePoint, users can effortlessly consolidate data for deeper insights. This integration not only streamlines operation but also allows teams to share real-time insights across departments. Consequently, the ability to visualize data directly from existing business applications helps organizations make informed decisions swiftly.
Moreover, the platform supports advanced analytics features, including AI-driven insights, enabling users to uncover trends and anomalies in their data. With automated reporting and dashboards, employees can quickly access key metrics that matter to their roles. Importantly, Power BI prioritizes user accessibility by requiring minimal expertise. This democratization of analytics empowers everyone in the organization to participate in data-driven decision-making, reinforcing a data-centric culture. Integrating Power BI into business suites exemplifies its role in enhancing efficiency and driving strategic insights.
IBM Cognos: Enterprise-Level Insights
The significance of enterprise-level insights cannot be understated in the era of AI analytics. Tailored to serve large organizations, enterprise-level insights encompass a range of functional capabilities that empower decision-makers to derive actionable knowledge from data easily. Accessible interfaces allow team members at varying skill levels to generate valuable reports and analyze customer interactions in real time, ensuring everyone in the company can participate in the analytics process.
Key features include the ability to transcribe and visualize calls, enabling users to examine customer feedback comprehensively. Analytics engines can automatically extract pain points and desires, facilitating deeper understanding of customer behaviors. Furthermore, grouping multiple projects allows businesses to gain insights across different datasets, enhancing the quality of decision-making. The interactive nature of such tools helps bridge the gap between raw data and strategic initiatives, making them essential in the AI analytics comparison landscape.
Looker: Data Exploration and Insights
Data exploration and insights play a crucial role in understanding and leveraging data effectively. Users can interact with comprehensive datasets to unearth actionable insights that inform business decisions. Through advanced querying capabilities, one can ask specific questions about their data and visualize various trends. This allows for a thorough analysis of customer feedback, enabling organizations to discern unique opinions and patterns.
Moreover, the ability to compare datasets from different sources enhances the depth of analysis. For instance, comparing feedback from various locations reveals regional differences in customer sentiment. This level of data exploration empowers businesses to adapt their strategies and products more effectively. Ultimately, effective data exploration tools are essential for deriving meaningful insights, ensuring that organizations remain competitive and responsive in today's market.
Conclusion: The Ultimate Guide to AI Analytics Comparison
In the realm of AI analytics comparison, we have explored a variety of tools that offer unique features and capabilities. Understanding these differences helps businesses choose the right solution tailored to their specific needs. Tools like Tableau, Power BI, and IBM Cognos each have strengths that cater to various analytical demands, from comprehensive reporting to real-time data integration.
Ultimately, an informed AI analytics comparison enables organizations to harness data effectively for strategic decision-making. By assessing key functionalities, user-friendliness, and scalability, companies can better navigate the landscape of AI analytics tools. This guide serves as a valuable resource for those looking to make data-driven choices in their analytics journey.
💬 Questions about AI Analytics Tools Features And Comparisons?
Our team typically responds within minutes