AI Analytics Implementation is transforming the way organizations make decisions and understand their data. As businesses grapple with vast amounts of information, the need for insightful analysis becomes critical. The implementation of AI analytics offers a structured approach to extracting valuable insights, enabling informed decisions that drive success.
This section will explore the core aspects of AI Analytics Implementation, highlighting its significance in todayโs data-driven world. We will discuss the various stages of implementation and the essential components needed to ensure success. By grasping the fundamentals, organizations can begin their journey towards unlocking the full potential of AI in their analytics efforts.
Generate visualizations from your qualitative data. At Scale.
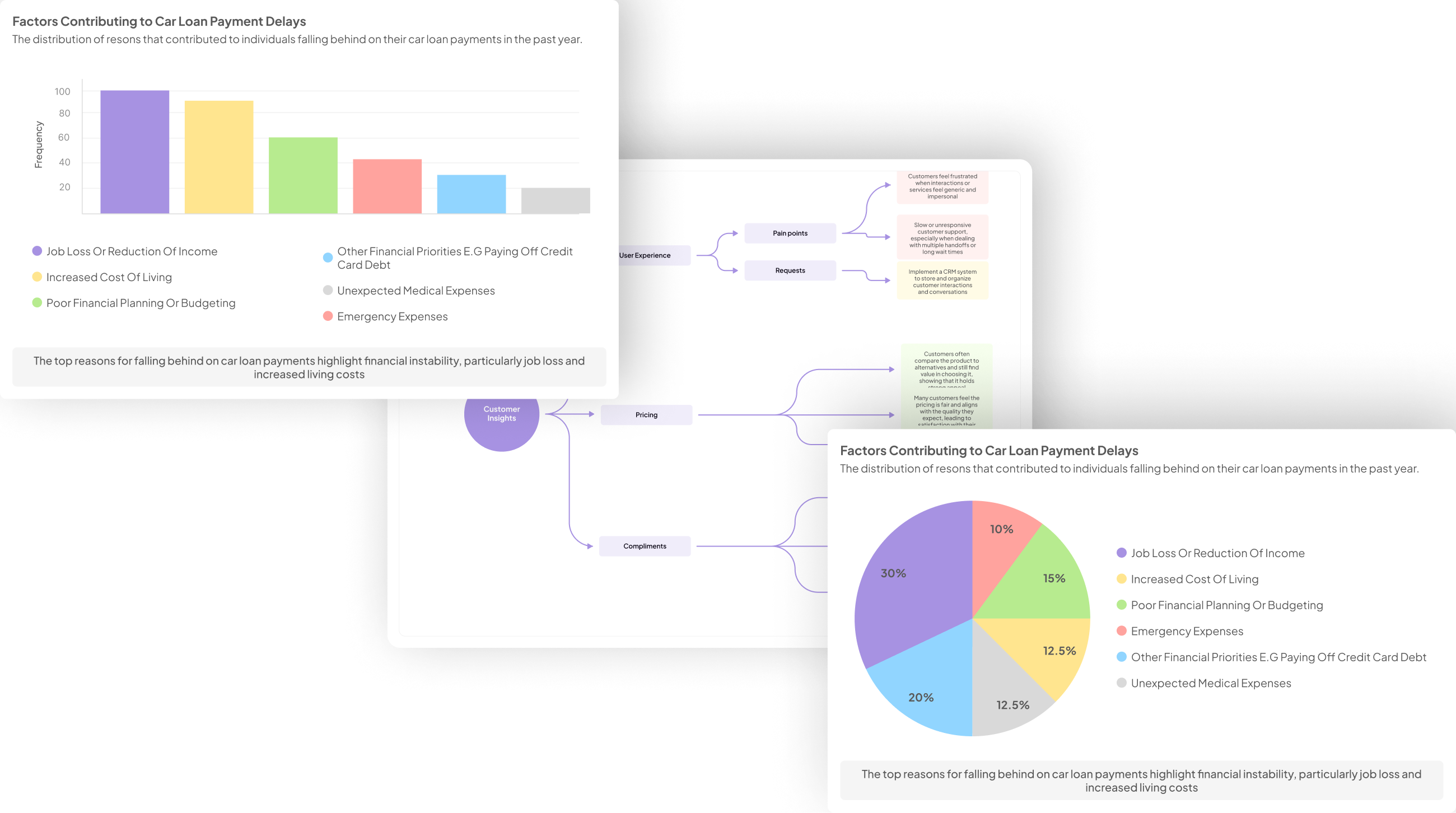
Understanding AI Analytics Implementation
Understanding AI Analytics Implementation involves grasping how artificial intelligence transforms data into actionable insights. This process begins with clarity about objectives and the specific problems AI can solve. It is essential for organizations to comprehend the data they possess and how it can be effectively analyzed using AI technologies. Knowing what questions to ask of the data ensures meaningful outcomes that align with business goals.
Moreover, successful AI Analytics Implementation combines the right tools, technologies, and an understanding of internal processes. Essential components include robust data preparation, integration capabilities, and ongoing model training. Organizations also need to encourage a culture of data-driven decision-making. By fostering collaboration among teams, organizations can enhance their analytics sophistication, leading to improved outcomes. Engaging stakeholders at each step bolsters understanding and adoption, paving the way for a successful AI journey.
The Basics of AI-Driven Insights
AI analytics implementation begins with understanding AI-driven insights, which serve as the foundation of effective decision-making. These insights transform raw data into meaningful information by revealing patterns and trends that may not be immediately apparent. Often, this process involves analyzing customer feedback, market trends, and operational metrics to generate actionable insights that guide strategy.
To successfully implement AI-driven insights, it is essential to establish a clear framework. Key elements include data collection, which ensures that relevant information is gathered; analysis, where algorithms process the data to extract insights; and visualization, which presents these insights in an easily interpretable format. Engaging all levels within an organization is vital, as it democratizes access to information, empowering employees to utilize insights in their everyday tasks. By recognizing the importance of AI-driven insights, teams can make better-informed decisions that ultimately enhance performance and innovation across the board.
Key Components of Successful Implementation
Successful AI analytics implementation hinges on several key components that can significantly affect outcomes. First, clear objectives must be established to ensure that all stakeholders are aligned on the desired results. This initial step enables teams to focus their efforts and resources on specific goals, making implementation more efficient.
Next, selecting the right tools and technologies is essential for effective data handling. These solutions should not only meet the requirements of your objectives but also integrate seamlessly with existing systems. Third, thorough data preparation and integration cannot be overlooked, as quality data is vital for accurate analysis. After securing reliable data, the model training and optimization phase helps tailor algorithms to specific needs, enhancing the overall effectiveness of the AI analytics implementation. Each of these components plays a crucial role in achieving successful results and maximizing the value of your AI initiatives.
Generate Journey maps, Mind maps, Bar charts and more from your data in Minutes
Steps to Effective AI Analytics Implementation
Effective AI analytics implementation begins with clearly defining objectives and scope. Organizations must understand what insights they seek and how these insights will influence decision-making processes. Establishing measurable goals provides a foundation for tracking progress and success throughout the implementation journey. Engaging key stakeholders early ensures that all perspectives are considered, which ultimately leads to a more comprehensive strategy.
Next, selecting appropriate tools and technologies plays a vital role in AI analytics implementation. Different tools offer unique capabilities, so itโs important to evaluate solutions that align with specific needs and objectives. Following this, data preparation and integration is crucial, as the quality of results directly relates to the data utilized. Ensuring clean, well-structured, and relevant data sets will enhance the accuracy of analytics. Finally, focus on model training and optimization, constantly refining the models to improve their predictive power. Each of these steps is critical for achieving robust AI analytics outcomes.
Step 1: Identifying Objectives and Scope
Identifying objectives and scope is a crucial first step in AI Analytics Implementation. The process begins by clearly defining what you intend to achieve with AI analytics, whether itโs optimizing operations, enhancing customer engagement, or gaining market insights. Understanding these objectives will help you remain focused and aligned throughout the entire implementation journey. It is essential to engage stakeholders early on, gathering their views and integrating their goals into your analytics strategy.
Once objectives are established, you must define the scope of your project. Identify the specific data sets you will analyze, the metrics you will use, and any technological limitations you may face. Outlining clear boundaries can prevent scope creep and ensure efficient use of resources. Establish a plan to evaluate success criteria, ensuring that your analytics efforts yield measurable outcomes aligned with your objectives. This foundational work will streamline subsequent stages of your AI analytics implementation, ultimately leading to a more effective and impactful strategy.
Step 2: Selecting Appropriate Tools and Technologies
Choosing the right tools and technologies is crucial for effective AI Analytics Implementation. The vast array of available options necessitates a thorough evaluation of your specific needs and goals. Start by assessing your organizational requirements, which could include capabilities for data storage, processing power, and user interface. Tools should be user-friendly and scalable, allowing team members at various skill levels to leverage them efficiently.
Next, prioritize technologies that align with your analytical objectives. For instance, if real-time data analysis is vital, consider platforms that facilitate rapid processing and visualization. Also, ensure that the tools you select can integrate seamlessly with your existing systems. By carefully considering these factors, you can empower your team to derive meaningful insights and drive impactful decisions. Effective tool selection lays a strong foundation for your AI Analytics journey, ensuring that resources are utilized to their fullest potential.
Step 3: Data Preparation and Integration
Data preparation and integration are pivotal in the process of AI Analytics Implementation. In this step, it's essential to gather diverse data sources, ensuring they are relevant and high-quality. This typically includes structured data from databases and unstructured data from sources like transcripts, surveys, or social media. The significance of careful data aggregation cannot be overstated, as it directly affects the insights gleaned later in the process.
To effectively prepare and integrate your data, consider the following key actions:
- Data Cleaning: Remove inaccuracies and irrelevant information to ensure data integrity.
- Data Normalization: Standardize data formats to facilitate smoother integration.
- Data Enrichment: Enhance data sets with additional information, such as demographic details, to provide deeper insights.
- Data Integration: Merge data from multiple sources for a unified view that supports comprehensive analysis.
By executing these actions thoughtfully, organizations lay the groundwork for impactful AI analytics, enabling informed decision-making and strategic planning based on reliable insights.
Step 4: Model Training and Optimization
In the context of AI Analytics Implementation, model training and optimization play a critical role in harnessing the full potential of your data. This step begins with selecting the right algorithm based on your project requirements. Factors such as data types, volume, and anticipated outcomes will guide your choice, ensuring alignment with the overall objectives.
Once the model is selected, thorough training using high-quality data becomes essential. This phase involves iterating through various parameters to optimize performance. Techniques such as cross-validation can help validate the model's accuracy, while hyperparameter tuning enhances its predictive capabilities.
Ultimately, the goal is to refine the model until it accurately reflects real-world scenarios. Continuous monitoring and adjustment maintain its relevance, adapting to new data and ensuring sustained effectiveness in decision-making. Properly executed model training and optimization are key to successful AI analytics implementation, driving meaningful insights and informed strategies.
Conclusion: Embracing AI Analytics Implementation for Success
Successfully adopting AI analytics involves a transformative journey that combines technology, strategy, and people. By embracing AI analytics implementation, organizations can turn data into actionable insights that drive informed decision-making. This collaborative effort not only enhances operational efficiency but fosters a culture of continuous improvement, ultimately positioning businesses for long-term success.
As organizations embark on this journey, they must prioritize clear objectives and stakeholder engagement. Fostering a supportive environment and employing versatile tools are essential for overcoming challenges. When effectively implemented, AI analytics solutions yield valuable insights that inform strategy and encourage growth, making the investment worthwhile in the ever-evolving business landscape.