AI Agents for Predictive Qualitative Insights in Financial Services
-
Bella Williams
- 10 min read
Predictive Financial Analytics plays a pivotal role in transforming the financial services sector. By harnessing artificial intelligence, organizations can efficiently analyze vast amounts of data to uncover trends and insights that inform strategic decision-making. This analysis empowers businesses to anticipate market shifts and better understand customer behaviors, ultimately leading to enhanced financial forecasting.
As the demand for real-time insights increases, the integration of AI techniques becomes essential. AI agents can extract qualitative information from financial data, revealing underlying themes and patterns that may not be immediately apparent. Utilizing Predictive Financial Analytics enables firms to improve their operational efficiency and remain competitive in a rapidly evolving marketplace.
Extract insights from Customer & Employee Interviews. At Scale.
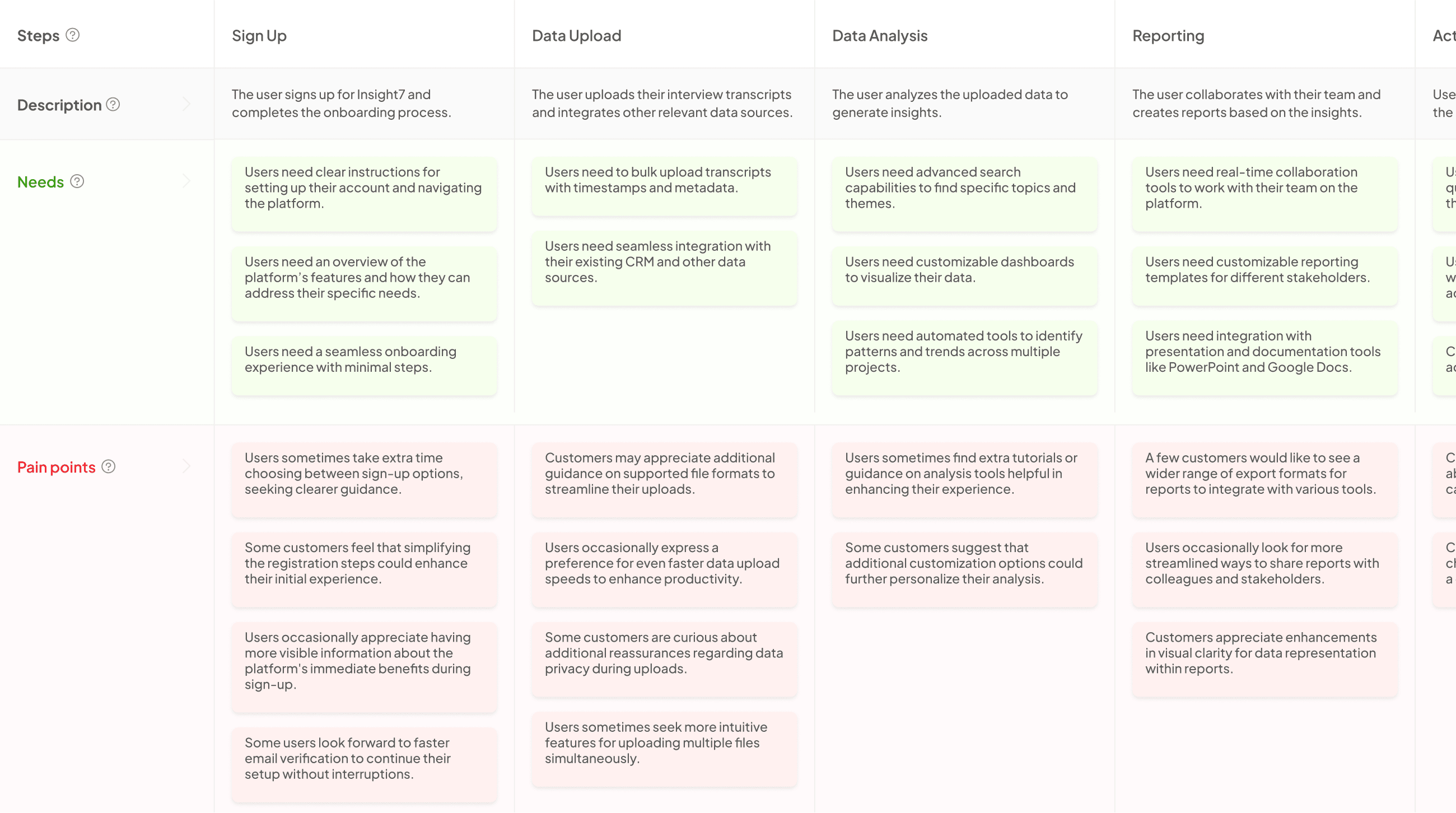
The Role of AI Agents in Predictive Financial Analytics
AI agents play a transformative role in predictive financial analytics, serving as vital tools for understanding market behaviors and trends. These intelligent systems analyze extensive datasets, extracting patterns and generating insights that human analysts may overlook. By leveraging advanced algorithms, AI agents can forecast financial outcomes with remarkable accuracy, leading organizations to make informed, proactive decisions.
The integration of AI agents enhances the efficiency of financial forecasting processes. They streamline data collection and analysis, reducing the time required for manual evaluation. Moreover, AI agents continuously learn from new information, refining their predictive capabilities over time. This adaptability not only improves the precision of predictions but also empowers financial institutions to respond swiftly to market changes. In summary, AI's role in predictive financial analytics represents a significant advancement, offering deeper qualitative insights and fostering a more proactive financial strategy.
Understanding AI-Driven Predictive Models
AI-driven predictive models use sophisticated algorithms to analyze vast datasets, identifying trends that would be invisible to the human eye. These models play a critical role in predictive financial analytics by accurately forecasting market movements and possible future scenarios. Understanding how these AI agents operate is vital for professionals aiming to harness the power of data for strategic decision-making in financial services.
The foundation of predictive financial analytics lies in the ability of AI models to process historical data and identify patterns. By assessing variables like market sentiment, economic indicators, and customer behavior, these models can generate qualitative insights to guide business strategies. As AI technology continues to evolve, the integration of these predictive models offers businesses a substantial advantage, enabling them to respond proactively to market changes and improve their overall performance. This understanding of AI-driven models not only enhances decision-making processes but also fosters a more agile approach to risk management in financial sectors.
- Defining AI agents and their relevance in financial analytics.
AI agents play a crucial role in the realm of predictive financial analytics, streamlining processes and enhancing decision-making. By automating data analysis, these agents can identify patterns that humans may overlook. In financial analytics, their ability to process vast datasets in real time allows organizations to make informed decisions faster.
Furthermore, AI agents provide qualitative insights that help businesses understand market dynamics and customer behaviors. These insights are invaluable for forecasting and strategic planning, ensuring firms remain competitive. As they continue to evolve, the relevance of AI agents in predictive financial analytics will only increase, offering deeper insights and greater operational efficiency.
In summary, AI agents are not merely tools; they are integral to refining predictive models, optimizing investment strategies, and contributing significantly to operational success in financial services. Their growing importance underscores the necessity for organizations to embrace this technology for superior financial analytics.
- How AI models predict trends and provide qualitative insights.
AI models excel in predictive financial analytics by analyzing vast datasets to identify patterns and trends. They utilize historical data to forecast potential outcomes, enabling financial institutions to make informed decisions. These models process qualitative insights, transforming subjective feedback into actionable data that can drive strategic decisions.
Additionally, AI agents evaluate sentiments within customer communications, such as call transcripts and surveys. By extracting nuanced perspectives—both positive and negative—these models help organizations understand customer needs and market dynamics. As a result, they deliver deeper insights that enhance risk assessment and the formulation of tailored services. Through continuous learning, AI models adapt to changing market conditions, ensuring that financial services remain agile and responsive to emerging trends. This interplay between quantitative predictions and qualitative insights enriches the decision-making landscape in financial services, reinforcing the importance of advanced analytics in shaping superior strategies.
Benefits of AI in Predictive Financial Analytics for Financial Services
AI has significantly transformed the realm of predictive financial analytics, bringing a multitude of benefits for financial services. Firstly, it enhances decision-making processes by providing real-time, data-driven insights. Financial institutions can analyze vast amounts of data quickly, allowing for more informed choices and strategies. AI-powered tools also create a proactive approach, enabling firms to anticipate market changes rather than merely reacting to them.
Moreover, AI improves the accuracy and efficiency of financial forecasting. Traditional methods often rely on historical data and subjective interpretations, which can lead to inaccuracies. In contrast, AI algorithms evaluate countless variables and trends, producing more reliable forecasts. With continuous learning capabilities, these systems refine their predictions over time, making them invaluable in strategic planning and risk management. The integration of AI in predictive financial analytics ultimately positions financial services to navigate complexities more adeptly and maintain a competitive edge.
- Enhancing decision-making processes.
In the realm of financial services, enhancing decision-making processes is crucial for achieving strategic objectives. AI agents are transforming traditional methodologies by providing predictive financial analytics that guide decision-makers through complex financial landscapes. By harnessing advanced algorithms, these agents analyze vast datasets, uncover insights, and identify emerging trends that would otherwise remain obscure.
Integrating AI agents into decision-making practices offers several key benefits. First, they help minimize human error, ensuring that conclusions draw from data rather than intuition alone. Second, AI can quickly analyze diverse variables, facilitating a more comprehensive evaluation of scenarios. Third, timely insights enable firms to adapt swiftly to market fluctuations, helping to maintain a competitive edge. Overall, leveraging predictive financial analytics through AI agents empowers organizations to make informed decisions that drive growth and innovation in the financial sector.
- Improving accuracy and efficiency in financial forecasting.
Effective financial forecasting is integral to driving informed decision-making. By employing Predictive Financial Analytics, firms can enhance their forecasting accuracy and efficiency significantly. This methodology leverages advanced algorithms to analyze historical data, helping identify patterns and trends that inform future outcomes. As a result, decision-makers are better equipped to anticipate market movements and align strategies accordingly.
To improve forecasting processes, organizations should focus on three key aspects: data quality, integration of AI-driven insights, and continuous model training. First, ensuring high-quality data is fundamental for reliable predictions; discrepancies can lead to misguided decisions. Second, integrating AI insights improves accuracy, as AI models continuously learn from new information. Finally, regular updates to these models ensure they adapt to changing market conditions. Committing to these practices positions financial services to achieve superior forecasting capabilities.
Generate Journey maps, Mind maps, Bar charts and more from your data in Minutes
Tools for Implementing AI Agents in Financial Services
Implementing AI agents in financial services requires carefully chosen tools that enhance predictive financial analytics. The right technology can streamline processes, enabling firms to analyze vast amounts of qualitative data swiftly. Organizations can benefit from platforms like Insight7, which focuses on harnessing customer insights for better financial predictions, and IBM Watson, known for its robust AI capabilities that facilitate comprehensive analytics.
💬 Questions about AI Agents for Predictive Qualitative Insights in Financial Services?
Our team typically responds within minutes
In addition to these, tools such as DataRobot provide automated machine learning solutions, ensuring precision in insights, while H2O.ai offers a scalable, open-source platform ideal for predictive modeling. RapidMiner stands out as a data science platform that excels in providing robust predictive analytics, and Alteryx enhances the entire analytics process with its end-to-end solutions that integrate AI capabilities. By leveraging these tools, financial institutions can significantly improve decision-making processes and enhance the accuracy of their forecasts.
Choosing the Right AI Tools for Predictive Financial Analytics
Selecting suitable AI tools for predictive financial analytics is pivotal for financial services seeking accurate insights. Understanding the specific needs of your financial organization is the first step. Each tool offers unique features tailored to various aspects of predictive analytics, such as data ingestion, trend analysis, and reporting capabilities.
Consideration of tools like IBM Watson or DataRobot can enhance your analytics framework significantly. These platforms provide advanced algorithms, automating the process of analyzing vast datasets while ensuring precise predictions. Furthermore, tools like RapidMiner and H2O.ai facilitate improved scalability and programming flexibility. Evaluating user feedback and performance metrics becomes essential in making well-informed decisions. Ultimately, the right AI tools empower organizations to convert qualitative insights into actionable strategies, leading to improved financial outcomes and customer satisfaction.
- Insight7: Harnessing qualitative insights for better financial predictions.
To enhance financial predictions, organizations must embrace qualitative insights sourced from customer interactions. Traditional data analysis often overlooks the nuanced understanding that comes from conversations, which can yield valuable context for trends and behaviors. Predictive Financial Analytics should therefore incorporate these insights to refine forecasting models, ensuring they capture the complexities of human decision-making.
By blending quantitative data with qualitative insights, businesses can develop a more holistic view of market dynamics. Qualitative feedback highlights customer sentiments, preferences, and pain points, which are vital in shaping financial strategies. Moreover, timely extraction of these insights allows organizations to respond proactively to market changes, thereby maintaining a competitive edge. Ultimately, integrating qualitative insights into financial predictions not only enhances accuracy but also fosters a responsive and customer-centric approach within the financial services sector.
- IBM Watson: Powerful AI capabilities for comprehensive analytics.
In the realm of predictive financial analytics, one transformative player is known for its robust AI capabilities that significantly enhance data interpretation. The powerful AI tools integrated within this platform are designed to offer comprehensive analytics, enabling financial institutions to process vast amounts of data efficiently. By leveraging advanced algorithms, these capabilities allow users to uncover intricate trends and patterns, leading to actionable insights that can inform strategic financial decisions.
Furthermore, the ability to conduct predictive analyses enhances comprehension of market dynamics. Financial professionals can benefit from improved forecasting accuracy, facilitating timely decision-making that aligns with evolving market conditions. This integration of comprehensive analytics not only streamlines data processing but also impacts overall operational efficiency. Ultimately, the synergy of AI technology with financial analytics empowers institutions to harness data-driven insights, addressing complex challenges and making informed choices in today's competitive landscape.
- DataRobot: Automated machine learning for precision insights.
Automated machine learning transforms the landscape of financial analytics by streamlining complex processes. This innovative approach allows financial professionals to derive precision insights effortlessly, making data more accessible to everyone in the organization. By utilizing automated techniques, it democratizes the analytics experience, enabling employees without technical expertise to engage with predictive models effectively.
The advantages of harnessing automated machine learning in predictive financial analytics are profound. First, it accelerates data processing, leading to quicker decision-making. Second, the system continuously learns from new data, enhancing its accuracy over time. Lastly, it allows organizations to focus on strategic initiatives while the machine handles routine analyses, ultimately leading to a more agile financial operation. This shift not only drives efficiency but enhances the overall quality of insights drawn from financial data, empowering businesses to navigate the complexities of finance successfully.
- H2O.ai: Open-source platform for scalable predictive modeling.
The open-source platform for scalable predictive modeling offers an intuitive interface for users across various expertise levels. This approach fosters accessibility, allowing individuals within financial services to harness the power of predictive analytics efficiently. Users can input various datasets—such as calls and surveys—to uncover meaningful patterns and insights, which aid in decision-making and strategy formulation.
Integrating this platform into daily business operations facilitates the analysis of large data clusters, enabling financial analysts to identify key trends quickly. The system automatically extracts insights related to customer behavior, preferences, and pain points, thus revealing crucial qualitative data. These capabilities significantly enhance predictive financial analytics, empowering organizations to make informed decisions based on quantitative data and qualitative insights comprehensively. The result is a more streamlined process, leading to better alignment with customer expectations and market demands.
- RapidMiner: Data science platform for robust predictive analytics.
RapidMiner stands out as a powerful data science platform specifically designed for robust predictive analytics. This user-friendly tool democratizes access to data insights, enabling users across various skill levels to harness the power of predictive financial analytics without needing advanced expertise. It streamlines the process of analyzing data, making it accessible for seamless integration into business workflows.
Key features include an intuitive interface for visualizing conversations and extracting valuable insights. Users can easily aggregate data from multiple calls or sources, discovering patterns that drive customer behavior and enhance decision-making. Furthermore, RapidMiner facilitates the identification of pain points, desires, and actionable insights which can significantly elevate performance in the financial sector. By leveraging this platform, financial services can cultivate a competitive edge through informed forecasting and strategic planning, ultimately leading to better customer experiences and optimized resource allocation.
- Alteryx: End-to-end data analytics platform with AI capabilities.
In today’s rapidly evolving financial landscape, an end-to-end data analytics platform equipped with AI capabilities is essential for achieving predictive financial analytics. Such a platform enables organizations to seamlessly gather, analyze, and visualize vast amounts of data to draw meaningful insights. By integrating AI functionalities, it empowers users to uncover trends, pain points, and customer behaviors without needing extensive technical expertise.
One key feature of this platform is its intuitive interface, which allows users to initiate tasks directly from the homepage, such as creating reports or analyzing customer experiences. This democratization of data enables teams to engage more deeply with customer insights, generate actionable reports, and understand underlying issues. Whether summarizing data from numerous calls for a cohesive project or drilling down into specific pain points, the platform is designed to streamline the process, making predictive financial analytics accessible to all levels of an organization.
Conclusion: The Future of Predictive Financial Analytics with AI
As we look ahead, the future of predictive financial analytics with AI appears promising and transformative. The integration of AI agents enables financial services to not only process vast amounts of data but also derive meaningful qualitative insights. This evolution is set to enhance decision-making, making it more informed and timely.
Moreover, advancements in predictive financial analytics foster increased accuracy and efficiency, helping organizations anticipate trends and mitigate risks effectively. As AI technologies continue to mature, we can expect a more data-driven approach in financial services, ultimately leading to unprecedented levels of innovation and competitiveness in the market. Embracing this shift will empower financial institutions to thrive in an increasingly complex landscape.
💬 Questions about AI Agents for Predictive Qualitative Insights in Financial Services?
Our team typically responds within minutes