AI Agents for Predictive Insights in Business Intelligence in Banking
-
Bella Williams
- 10 min read
Predictive Banking Intelligence is transforming the way financial institutions operate in today's complex marketplace. Increasingly, banks are turning to AI agents to unlock valuable insights that drive strategic decision-making. These advanced tools enable organizations to forecast trends, assess risks, and enhance customer experiences, ultimately fostering a competitive edge in the industry.
As predictive capabilities evolve, the integration of AI becomes essential. By harnessing big data and sophisticated algorithms, banks can anticipate customer needs and market shifts. This alignment not only streamlines operations but also improves overall financial performance, making the adoption of Predictive Banking Intelligence a crucial step for businesses aiming to thrive in the evolving landscape of finance.
Extract insights from Customer & Employee Interviews. At Scale.
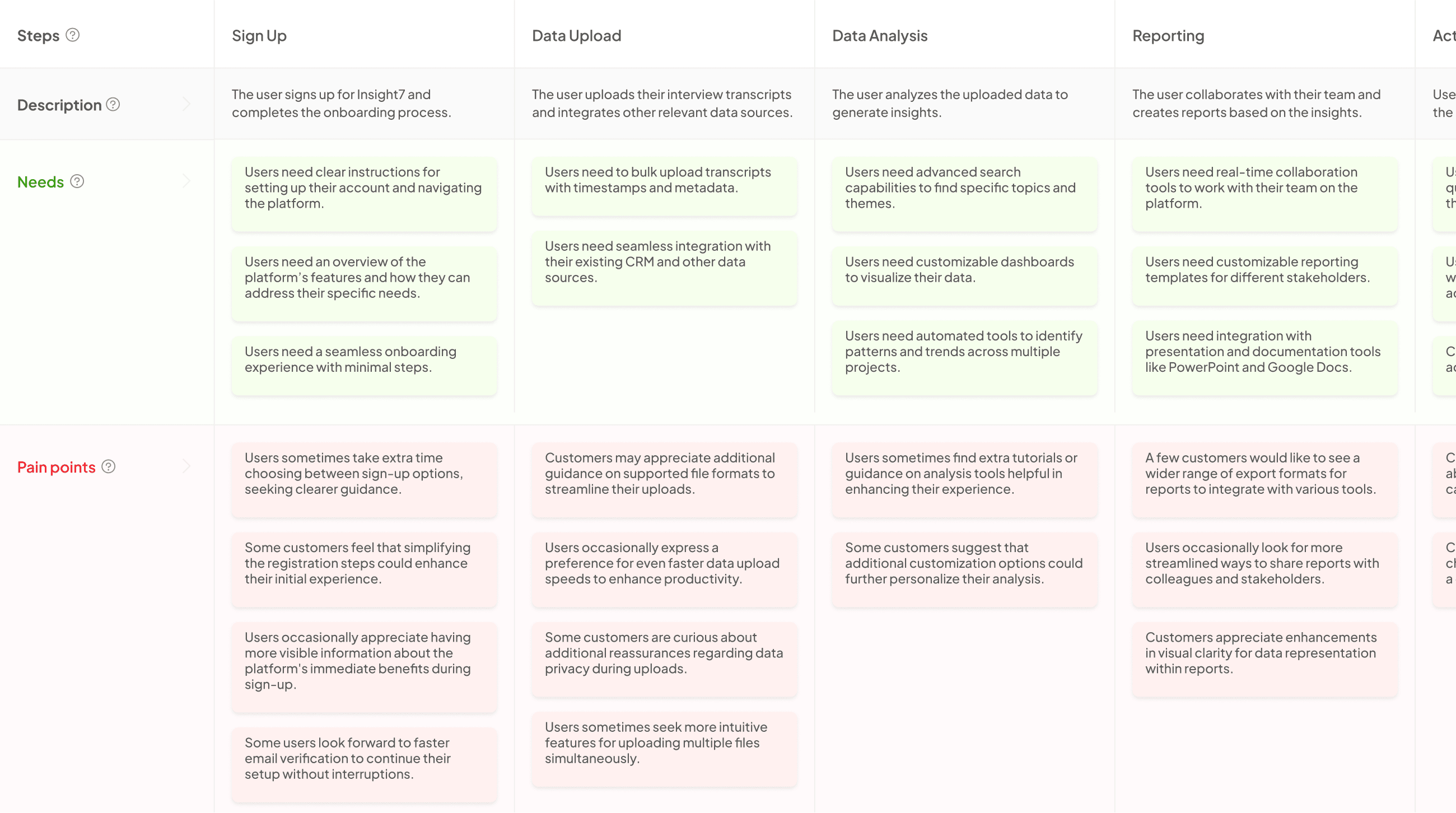
The Rise of AI Agents in Predictive Banking Intelligence
The emergence of AI agents is reshaping the landscape of Predictive Banking Intelligence, enabling institutions to harness advanced data analytics and predictive modeling. These intelligent systems can process vast amounts of transaction data swiftly, allowing banks to identify trends and behaviors in real-time. By utilizing AI, banks can enhance customer satisfaction, predict future financial needs, and mitigate risks effectively.
These AI agents act as digital assistants, analyzing patterns to provide actionable insights. They inform decision-making processes, helping institutions preempt client demands and optimize their services. As AI technology continues to evolve, its role in Predictive Banking Intelligence is becoming increasingly critical. This transition not only boosts operational efficiency but also fosters a more personalized banking experience for customers. The future promises even deeper integration of AI capabilities, fundamentally altering how banks operate and engage with their clients.
Understanding Predictive Banking Intelligence
Predictive Banking Intelligence represents a transformative approach within the banking sector, focusing on foresight and data-driven decision-making. By harnessing advanced algorithms and machine learning, banks can analyze vast amounts of data to identify trends, forecast customer behavior, and personalize services. This capability not only enhances operational efficiency but also enhances customer experiences by anticipating needs and mitigating risks.
To fully leverage Predictive Banking Intelligence, banking institutions must embrace a holistic strategy involving key components. Firstly, data quality is critical; accurate insights stem from clean and reliable data sources. Secondly, cross-functional collaboration among departments is essential to create a unified understanding of customer behaviors. This collaboration can be complemented by AI agents that automate data analysis, enabling teams to focus on strategic actions. Lastly, continuous assessment and adaptation of these intelligent systems are vital to ensure they adapt to changing customer expectations and market conditions. Emphasizing these components will empower banks to fully unlock the potential of Predictive Banking Intelligence.
Key Benefits of AI-Driven Insights in Banking
AI-driven insights in banking significantly enhance decision-making processes and operational efficiency. By harnessing predictive banking intelligence, institutions can analyze vast amounts of customer data, identify patterns, and anticipate future behaviors. This proactive approach not only optimizes customer relationship management but also minimizes risks associated with lending and investments.
One of the key benefits lies in personalized customer experiences. With precise insights, banks can tailor their services to meet individual needs, thereby improving customer satisfaction and retention. Furthermore, AI-driven analytics streamline risk assessment by providing real-time evaluations of potential threats. This capability allows banks to respond promptly to market changes, ensuring they remain competitive. Ultimately, the integration of predictive banking intelligence fosters greater trust and transparency between banks and their clients, positioning them to navigate future challenges more effectively.
Generate Journey maps, Mind maps, Bar charts and more from your data in Minutes
Leveraging AI Tools for Predictive Banking Intelligence
To harness the power of AI tools for predictive banking intelligence, institutions must begin by identifying their specific needs. Predictive banking intelligence enables banks to analyze customer behavior, anticipate market trends, and improve decision-making processes. By utilizing advanced AI agents, organizations can streamline data processing, revealing actionable insights that enhance operational efficiency.
Several key tools are transforming the landscape of predictive banking intelligence. These include platforms that analyze customer interactions, assess risks, and forecast market fluctuations. AI-driven solutions such as DataRobot and IBM Watson facilitate a deeper understanding of customer needs while enhancing reporting capabilities. Integrating these tools into existing banking systems is crucial, allowing organizations to capitalize on customer insights and drive strategic initiatives effectively. As banks begin to implement AI solutions, continuous monitoring and evaluation will ensure they harness predictive intelligence to meet both current challenges and future opportunities.
Top AI Tools Transforming Banking Insights
In the realm of predictive banking intelligence, various advanced AI tools are reshaping how banks derive insights from their data. These tools empower financial institutions to analyze customer behavior, predict trends, and enhance decision-making processes. By leveraging machine learning algorithms, these solutions can process vast amounts of data quickly, offering insights that were previously unattainable.
Five significant AI tools are transforming banking insights. First, Insight7 stands out for its user-friendly interface and powerful analytics capabilities, allowing users to generate comprehensive reports effortlessly. DataRobot offers a robust platform for automating machine learning workflows, driving accuracy in predictive modeling. H2O.ai excels in real-time data processing, providing banks with immediate insights that improve operational efficiency. With IBM Watson, financial institutions can harness natural language processing to analyze customer interactions and sentiment. Lastly, Microsoft Azure AI offers scalable solutions suitable for diverse banking operations, ensuring insights can be tailored to specific business needs. Collectively, these tools contribute to a new standard of predictive banking intelligence that is data-driven and customer-focused.
- insight7
In the context of AI agents for Predictive Banking Intelligence, insight7 stands out as a pivotal tool for gathering actionable insights. This self-service platform allows banks to efficiently analyze customer data, conversations, and interviews to derive valuable signals that inform strategic decision-making. As banks witness an explosion of customer interactions, traditional data analysis methods struggle to keep pace, leading to untapped opportunities.
To harness the true potential of Predictive Banking Intelligence, banks must focus on three critical areas. First, understanding customer needs ensures that data translation into actionable insights is both timely and relevant. Second, enhancing collaboration among departments can streamline the process of turning insights into strategies. Finally, investing in advanced analytical tools can dramatically improve data analysis efficiency, thereby placing banks ahead of competitors who rely on outdated approaches. In todayโs fast-paced environment, leveraging insight7 can be the key to unlocking superior predictive capabilities tailored to the banking sector.
- DataRobot
In the realm of predictive banking intelligence, the capability to harness data effectively can significantly transform decision-making. With advanced AI tools at hand, institutions can not only generate insights but also customize their analytical approaches. This adaptability empowers banks to refine customer interactions and optimize operational efficiency, paving the way for proactive service delivery.
To gain an edge in predictive banking intelligence, consider the following focal points:
User-Friendly Interfaces: A well-designed interface enables easier interaction with data, improving usability for various teams.
Automated Reporting: Regular and automated reports on key metrics provide timely insights, minimizing the need for manual data analysis.
Customizable Insights: Tailoring the analytical outputs to specific needs can enhance relevance, ensuring deeper customer understanding.
Integration Capabilities: A seamless connection with existing systems ensures that data flows without disruption, enhancing overall operational cohesion.
By addressing these aspects, banks will not only improve their predictive banking intelligence but also foster a culture of data-informed decision-making across all business units.
- H2O.ai
In the evolving landscape of banking, advanced tools play a significant role in enhancing predictive banking intelligence. These tools facilitate insightful decision-making, allowing financial institutions to harness the full potential of their data. By employing sophisticated algorithms, banks can create accurate forecasts and uncover patterns that drive better financial services. Understanding how these tools operate is crucial for aligning strategies with specific business needs.
AI agents serve as essential components in this process, transforming data into actionable intelligence. They enable banks to analyse customer behavior, assess risks, and optimize operations with unprecedented accuracy. When integrated effectively, these solutions not only streamline workflows but also enhance customer satisfaction through personalized experiences. Implementing such tools can be pivotal in remaining competitive, fostering innovation, and supporting strategic growth in today's dynamic banking environment.
The sector can greatly benefit from embracing this technology, ensuring that predictive banking intelligence becomes an integral part of their business intelligence strategy.
- IBM Watson
IBM Watson exemplifies innovation in AI-driven technologies, particularly in the realm of predictive banking intelligence. This advanced AI platform empowers financial institutions to analyze vast amounts of data and derive actionable insights. By utilizing natural language processing and machine learning, it enables banks to process information quickly and efficiently, enhancing decision-making processes.
In an industry where every second counts, Watsonโs ability to predict trends and customer needs proves invaluable. For instance, it can identify patterns in customer behavior, helping banks tailor services to individual preferences. Furthermore, implementing such technology allows institutions to proactively address potential risks and optimize operational efficiencies. By harnessing the predictive capabilities offered by this AI, banking professionals can advance toward a future where data-driven insights pave the way for improved customer satisfaction and competitive advantage. In an ever-evolving financial landscape, embracing these innovations is no longer optional; it has become essential for survival.
- Microsoft Azure AI
Microsoft Azure AI plays a pivotal role in advancing predictive banking intelligence within the financial sector. Its robust suite of tools enables banks to harness vast amounts of data effectively, turning raw information into actionable insights. By leveraging powerful machine learning algorithms and pre-built AI models, financial institutions can anticipate customer needs and enhance decision-making processes.
Moreover, Microsoft Azure AI's scalability allows businesses to customize solutions according to their unique requirements. This flexibility is key in a fast-evolving banking environment, where timely responsiveness to market dynamics is crucial. By integrating these AI capabilities, banks can aid in risk management, customer engagement, and operational efficiency, driving continuous improvement. Thus, Microsoft Azure AI stands as a cornerstone in the transformation journey toward predictive banking intelligence, ensuring that organizations remain competitive and customer-focused.
Steps to Implement AI Agents in Banking
To implement AI agents effectively in banking, it is essential to follow a structured approach that aligns with the goals of predictive banking intelligence. First, define your predictive goals by identifying the specific insights you aim to achieve. By clearly outlining these objectives, the application of AI agents will be more focused and relevant to your banking operations.
Next, select suitable AI solutions that fit your needs. Evaluate different AI tools that can provide the necessary data analytics and insights required for decision-making. Once selected, integrate the AI agents with your existing systems to ensure seamless functionality. This integration is crucial for maximizing the efficacy of predictive insights and ensuring that your agents can access and utilize real-time data. Finally, monitor and evaluate the performance of your AI agents regularly. Continuous assessment not only helps optimize their functionality but also ensures that they remain aligned with your evolving predictive banking intelligence objectives.
Step 1: Define Predictive Goals for Banking
Defining predictive goals is the first crucial step in harnessing Predictive Banking Intelligence effectively. This initial stage involves identifying specific objectives that align with your bank's strategic direction. Start by assessing various areas where predictive insights can add value, such as customer retention, loan default prediction, or fraud detection. These goals must be measurable so your institution can evaluate success with data-driven insights.
Once you've outlined these goals, itโs vital to communicate them across your organization. Engaging stakeholders helps ensure alignment of interests and resources. Each goal should reflect the unique challenges and opportunities within your banking context. By clearly defining what you aim to achieve, you pave the way for selecting appropriate AI tools and methodologies, setting the stage for a successful implementation of AI agents in banking.
Step 2: Select Suitable AI Solutions
Selecting suitable AI solutions is crucial for maximizing predictive banking intelligence. Start by assessing your specific business needs and identifying areas where predictive insights can be valuable. Different AI tools offer unique features tailored for various banking operations, such as customer service automation or risk assessment. Understanding these capabilities allows for a more informed choice.
Next, consider factors like scalability and integration with existing systems. Ensure that the AI solution can evolve with your business requirements and align with your overall strategy. Look for solutions that provide accurate and timely insights, as these will drive better decision-making. By evaluating these criteria, you can choose AI agents that not only enhance predictive capabilities but also foster a stronger customer relationship, ultimately paving the way for improved operational efficiency and profitability in banking.
Step 3: Integrate AI with Existing Systems
Integrating AI with existing systems is a crucial step in harnessing Predictive Banking Intelligence. This phase begins with assessing current infrastructure to determine compatibility with AI tools. Businesses must ensure that their technology stack can support the specific AI applications chosen, maintaining data integrity and security throughout the process. Effective integration enables seamless interactions between AI agents and existing databases, providing richer, more accurate insights.
Next, organizations should prioritize staff training. Empowering teams with the skills to utilize AI-driven systems enhances productivity and improves overall adaptability. Frequent evaluations during this phase guarantee that AI solutions evolve according to organizational needs, capturing real-time feedback for refinement. As banking continues to embrace technological advancements, integrating AI with existing systems positions institutions to leverage predictive insights effectively for strategic decision-making.
Step 4: Monitor and Evaluate AI Performance
Monitoring and evaluating AI performance is critical for successful implementation in predictive banking intelligence. Once AI agents are integrated, it's crucial to establish metrics that measure their effectiveness. Performance indicators should focus on accuracy, response times, and the quality of insights generated. Regular assessments of these metrics help identify areas for improvement and ensure alignment with organizational objectives.
Additionally, feedback loops play a vital role in AI evaluation. Engaging stakeholders, such as data analysts and compliance teams, provides insights into the AI's functionality in real-world scenarios. This collaborative approach fosters continuous refinement, enhancing predictive models over time. Documenting results and adjustments will also ensure compliance with regulatory standards. Through vigilant monitoring, organizations can harness predictive banking intelligence effectively, leading to more informed business decisions and optimized customer engagement. Remember, consistent evaluation can dramatically impact the overall success of AI initiatives in banking.
Conclusion: The Future of Predictive Banking Intelligence
The evolution of Predictive Banking Intelligence signifies a transformative shift in how financial institutions operate. By integrating AI agents into business intelligence processes, banks can anticipate customer needs and enhance decision-making. This forward-thinking approach not only improves efficiency but also fosters a deeper understanding of consumer behavior and preferences.
As we look to the future, the potential for predictive insights in banking is expansive. Financial institutions that harness the power of AI can not only streamline operations but also create personalized experiences for their clients. Ultimately, the future of predictive banking lies in continuous innovation, where technology and customer insights converge to redefine the banking experience.