AI Agents for Predictive Financial Intelligence in Banking
-
Bella Williams
- 10 min read
Predictive Banking Intelligence is transforming the financial landscape by enabling banks to anticipate customer needs and preferences. As institutions harness sophisticated AI agents, they unlock insights that were previously unattainable. This technology not only enhances operational efficiency but also fosters deeper customer relationships, ultimately leading to improved satisfaction and loyalty.
In todayโs rapidly evolving financial services sector, understanding the importance of Predictive Banking Intelligence is paramount. The ability to perceive trends and make data-informed decisions empowers banks to stay ahead of market dynamics. By integrating these intelligent systems, financial institutions can deliver tailored solutions, ensuring they meet the ever-changing demands of their clients effectively.
Extract insights from Customer & Employee Interviews. At Scale.
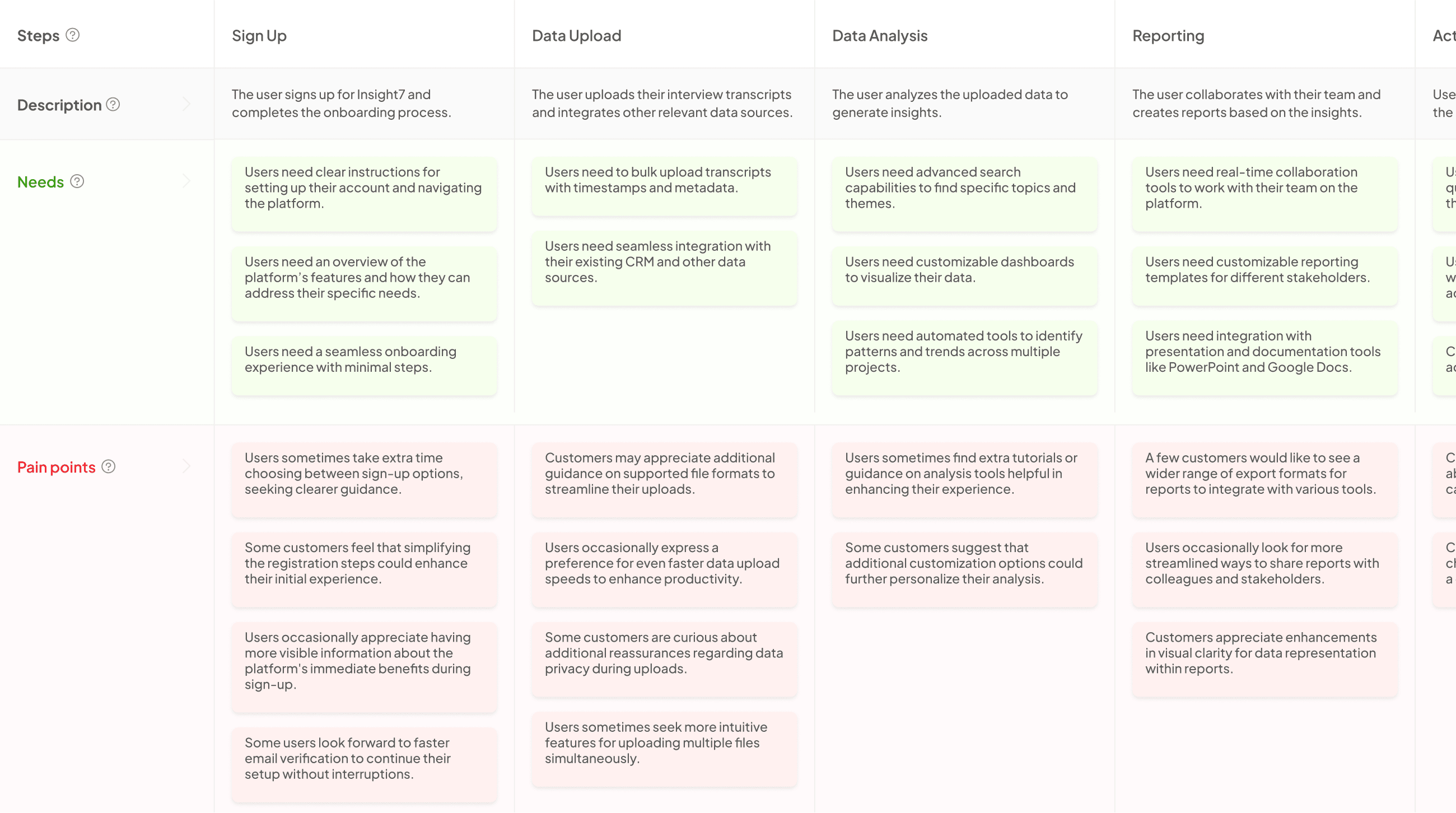
Understanding AI Agents in Predictive Banking Intelligence
Understanding AI agents within the context of predictive banking intelligence is crucial for grasping their transformative role in the banking sector. These agents harness advanced algorithms and machine learning techniques to analyze vast datasets, enabling banks to make informed decisions. By predicting customer behaviors and market trends, AI agents facilitate tailored financial services that enhance customer experiences and operational efficiency.
The integration of AI agents empowers banks to offer personalized solutions while mitigating risks associated with fraud and compliance. Data collection and preprocessing are fundamental steps in implementing these agents effectively. Following this, model training and validation ensure the accuracy of predictions, while continuous monitoring optimizes their performance. Adopting AI agents in predictive banking intelligence not only streamlines processes but also drives innovation, helping banks to stay competitive in an increasingly digital landscape.
The Role of AI in Transforming Banking
AI is playing an increasingly pivotal role in shaping the future of banking through Predictive Banking Intelligence. By harnessing vast amounts of data, AI systems can analyze customer behavior and market trends, helping banks to make informed decisions. This technology empowers financial institutions to anticipate customer needs, refine their service offerings, and enhance customer satisfaction effectively.
Moreover, AI-driven insights can streamline operations by automating routine tasks and enabling real-time risk assessments. As banks strive to stay ahead in a competitive environment, predictive analytics becomes a cornerstone for innovative strategies and substantial cost savings. By transforming raw data into actionable intelligence, AI not only prepares banks to meet current market demands but also positions them well for future challenges. Embracing this transformation will ultimately lead to improved financial stability and a more personalized banking experience for customers.
Key Components of Predictive Banking Intelligence
Predictive Banking Intelligence relies on several key components that contribute significantly to enhancing financial decision-making. Firstly, data collection and analysis form the foundation of this intelligence. Gathering data from diverse sources allows predictive systems to identify patterns and trends that inform future decisions. Furthermore, integrating AI-driven analytics enhances the capability to process vast datasets with efficiency and speed.
Next, model training and validation are essential. By employing robust algorithms, these models can accurately predict outcomes based on historical data. Continuous monitoring ensures models remain relevant as market conditions change, adapting to new information and behaviors. Finally, user-friendly interfaces facilitate access to insights, allowing stakeholders at all levels to leverage predictive banking intelligence without specialized training. Together, these components create a powerful ecosystem that empowers banks to anticipate customer needs and navigate market complexities effectively.
Implementation Steps for AI Agents in Predictive Banking Intelligence
Implementing AI agents in Predictive Banking Intelligence involves a structured approach to ensure seamless integration and optimal performance. The first step is data collection and preprocessing. Banks must gather both structured and unstructured data from various sources, ensuring that the data is cleaned and formatted appropriately. This foundational step enables the accurate feeding of quality data into AI models.
The next phase consists of model training and validation. Here, the AI algorithms learn from the provided data, identifying patterns that predict customer behavior and financial trends. It is important to periodically validate these models against real-world scenarios to ensure accuracy. Subsequently, integration with banking systems must occur. This step is critical for enabling the AI agents to access real-time data and interact with existing workflows effectively. Lastly, continuous monitoring and optimization of the models are necessary to adapt to changing market conditions. By following these steps, banking institutions can harness the full potential of Predictive Banking Intelligence.
Generate Journey maps, Mind maps, Bar charts and more from your data in Minutes
Step 1: Data Collection and Preprocessing
Effective predictive banking intelligence begins with thorough data collection and preprocessing. This initial step is crucial as it sets the foundation for developing AI agents that can analyze financial trends and customer behavior. Start by identifying diverse data sources such as transaction records, customer interactions, and market conditions. Gathering comprehensive and high-quality data ensures that the predictive models will be accurate and reliable.
Once data is collected, preprocessing becomes essential. This involves cleaning the dataset by removing duplicates and missing values to enhance data quality. Additionally, standardizing variables can facilitate better model performance. Transforming and categorizing data allows for more meaningful interpretations, which are vital for drawing insights. By prioritizing data collection and preprocessing, banks can effectively harness predictive banking intelligence to anticipate customer needs and improve decision-making processes.
Step 2: Model Training and Validation
In this crucial step, Model Training and Validation, the objective is to refine AI algorithms using historical data for enhancing Predictive Banking Intelligence. Initially, data sets are split into training and validation subsets. The training set helps the model learn patterns, while the validation set evaluates its readiness to predict unseen outcomes.
During the training phase, various algorithms are tested, optimizing parameters to improve accuracy. Techniques such as cross-validation can help ensure that the model is robust and generalizes well across different scenarios. Following training, validation kicks inโwhere the model's performance is critically assessed against predefined metrics. This stage ensures that predictions maintain a high level of reliability when applied to real-world banking scenarios, ultimately enhancing decision-making processes. By effectively training and validating the model, institutions can better harness AI for financial forecasting and risk management, driving informed strategic initiatives.
Step 3: Integration with Banking Systems
Integrating AI agents with banking systems is a crucial step in enhancing predictive banking intelligence. This integration ensures that the AI systems align seamlessly with existing banking infrastructure, allowing for the timely processing of customer data and improving service delivery. By incorporating AI-driven insights into core banking operations, institutions can forecast financial trends, detect anomalies, and better understand customer behaviors.
The integration process typically involves several key steps. First, banks must assess their current systems to identify where AI can provide the most benefit. Next, data from diverse sources must be aggregated and formatted to create a comprehensive input for the AI models. Following this, APIs should be deployed to facilitate communication between banking platforms and AI tools. Lastly, continuous feedback loops are essential to refine the predictive models continuously. This structured approach not only boosts operational efficiency but also significantly enhances the overall customer experience.
๐ฌ Questions about AI Agents for Predictive Financial Intelligence in Banking?
Our team typically responds within minutes
Step 4: Continuous Monitoring and Optimization
Continuous monitoring and optimization are vital to maintaining effective Predictive Banking Intelligence. This process ensures that AI agents remain responsive to evolving market conditions and customer needs. Regular analysis of performance data allows banks to identify potential areas for improvement, fostering agility in their decision-making. By refining algorithms based on real-time data, organizations can enhance accuracy in predictions, thus driving better financial outcomes.
Moreover, ongoing optimization promotes a culture of continuous improvement within banking institutions. As insights are gathered, teams can swiftly adapt strategies and services to align more closely with customer expectations. The interplay between monitoring and optimization creates a dynamic system that not only reacts to customer feedback but also anticipates future demands. Emphasizing these practices strengthens the bank's position in a competitive marketplace, ensuring robust financial intelligence solutions are in place.
Top Tools for Predictive Banking Intelligence
Predictive Banking Intelligence is greatly enhanced by various tools that empower financial institutions to harness data effectively. These tools play a key role in analyzing customer behavior, market trends, and operational efficiencies. Among the top options, insight7 stands out for its user-friendly interface, allowing teams to easily access and analyze call transcripts, generate reports, and identify customer pain points in one platform.
Another notable tool is IBM Watson, which excels in natural language processing, providing deep insights into customer interactions. Microsoft Azure Machine Learning offers robust capabilities for model training and deployment, ensuring that banking institutions can effectively predict outcomes and adapt strategies. Google Cloud AI delivers powerful analytics tools to uncover hidden trends, while DataRobot simplifies the process of building and deploying machine learning models. Each of these tools contributes significantly to achieving predictive banking intelligence, ultimately optimizing customer experiences and driving informed decision-making.
insight7
Integrating AI Agents into banks is reshaping the landscape of Predictive Banking Intelligence, paving the way for a new era of data-driven decisions. These intelligent systems are designed to analyze vast amounts of customer data, enabling banks to predict trends and behaviors with remarkable accuracy. By employing advanced algorithms, banks can uncover actionable insights from what were once overwhelming datasets.
The key to successful implementation lies in understanding the core challenges faced in the financial industry. Firstly, efficient data collection must be prioritized to ensure a solid foundation for predictive models. Following this, rigorous model training and validation is essential to enhance accuracy and reliability. Lastly, the continuous monitoring and optimization of these AI systems will ensure that they adapt to changing market conditions and customer expectations. Through these integrated steps, banks can harness Predictive Banking Intelligence, transform their operations, and enhance customer experiences.
Tool 2: IBM Watson
IBM Watson serves as a powerful tool in the realm of predictive banking intelligence, offering insights that empower financial institutions to make informed decisions. This technology leverages advanced artificial intelligence to analyze vast quantities of financial data rapidly. By doing so, it not only identifies trends but also predicts future market movements, enhancing the bankโs strategic capabilities.
Implementing IBM Watson can significantly streamline operational processes. With its natural language processing abilities, it effectively interprets customer inquiries and feedback, turning them into actionable insights. This capability allows banks to respond proactively to customer needs and market changes. Additionally, the tool supports risk assessment measures by analyzing historical data and identifying potential financial threats. By harnessing these AI-driven insights, banking institutions can achieve a competitive edge, leading to enhanced customer satisfaction and improved financial outcomes.
Tool 3: Microsoft Azure Machine Learning
Microsoft Azure Machine Learning serves as a powerful tool designed to enhance predictive banking intelligence. By providing robust data analytics and machine learning capabilities, it enables financial institutions to harness vast amounts of data to derive actionable insights. Users can efficiently build, train, and deploy machine learning models tailored to their specific banking needs.
For effective predictive banking intelligence, Azure Machine Learning offers several key features. First, it allows for seamless integration with existing banking systems, ensuring easy access to relevant data sources. Next, users can utilize automated machine learning tools to expedite model development, thus reducing the time from conception to deployment. Finally, the platform supports continuous monitoring and improvement of models, which is essential for adapting to market changes and evolving data patterns. This approach empowers banks to proactively respond to customer needs and enhance decision-making, ultimately leading to improved financial services.
Tool 4: Google Cloud AI
Google Cloud AI provides a robust framework for advancing Predictive Banking Intelligence in the finance sector. Its versatile tools enable banks to harness vast amounts of data, transforming it into actionable insights. By using advanced machine learning algorithms, financial institutions can forecast trends, identify potential risks, and enhance customer interactions with data-driven recommendations. This adaptability fosters a more proactive and personalized banking experience, tailored to individual client needs.
Moreover, Google Cloud AI emphasizes ease of integration with existing banking systems, ensuring a smooth transition for organizations. Continuous monitoring and optimization powered by real-time analytics help maintain accuracy in predictions. The powerful capabilities of this tool not only support risk management but also boost operational efficiencies. As banks explore predictive analytics, Google Cloud AI stands out as a significant enabler of smarter financial decision-making and improved customer engagement.
Tool 5: DataRobot
DataRobot serves as a powerful ally in the quest for predictive banking intelligence. This innovative platform simplifies the process of harnessing data, allowing banking professionals to generate valuable insights without requiring extensive technical expertise. As users interact with the platform, they can quickly transform raw data into insightful reports, enhancing decision-making processes across the organization.
One of the key benefits of DataRobot is its user-friendly interface, which democratizes access to predictive analytics. Individuals from various departments can utilize the tool to identify customer pain points and understand desires through automated insights. By categorizing data effectively, DataRobot not only streamlines analysis but also accelerates the time-to-insight cycle, enabling banks to stay ahead in an ever-evolving market. As a result, decision-makers can leverage actionable insights to refine strategies, optimize customer experiences, and ultimately drive better financial outcomes.
Conclusion: The Future of Predictive Banking Intelligence in Banking
The future of predictive banking intelligence presents a transformative opportunity for financial institutions. As AI agents evolve, their capabilities will integrate seamlessly with banking operations, enhancing decision-making processes. These advancements will lead to more personalized customer experiences and improved risk management strategies, ultimately fostering greater trust between banks and their clients.
In this dynamic landscape, continuous data analysis will be essential. Predictive banking intelligence will empower banks to anticipate customer needs and identify market trends, enabling proactive responses. As this technology becomes standard, institutions that embrace these innovations will not only stay competitive but also redefine the banking experience for a new era.
๐ฌ Questions about AI Agents for Predictive Financial Intelligence in Banking?
Our team typically responds within minutes