AI Agents for Predictive Customer Feedback in E-commerce
-
Bella Williams
- 10 min read
In the realm of predictive e-commerce feedback, understanding how AI agents function is pivotal. These agents analyze customer data to forecast future behaviors and sentiments. By processing vast amounts of feedback, they can identify patterns and trends, enabling businesses to tailor their strategies accordingly. This proactive approach ensures that customer preferences are anticipated, paving the way for enhanced customer satisfaction.
AI agents play a crucial role in collecting this predictive feedback. They can sift through various sources, such as social media, reviews, and surveys, to glean insights that may otherwise go unnoticed. By leveraging natural language processing, these agents can decode sentiments, highlighting both positive and negative aspects of customer interactions. This deep analysis allows e-commerce businesses to refine their offerings and engage with customers in a more meaningful way, ultimately driving sales and loyalty.
Introduction to Predictive E-commerce Feedback in AI Agents
In the evolving realm of e-commerce, understanding customer preferences has become paramount. Predictive e-commerce feedback offers a transformative approach by leveraging artificial intelligence to analyze customer sentiments and behaviors. This method not only enhances the shopping experience but also empowers businesses to adapt swiftly to consumer needs.
AI agents play a critical role in this ecosystem by efficiently gathering and processing data. They sift through vast amounts of customer interactions, pinpointing emerging trends and providing actionable insights. By interpreting this feedback, businesses can tailor their offerings, ultimately creating a more personalized and engaging environment for shoppers. Through this approach, companies can stay ahead in a competitive marketplace.
Extract insights from Customer & Employee Interviews. At Scale.
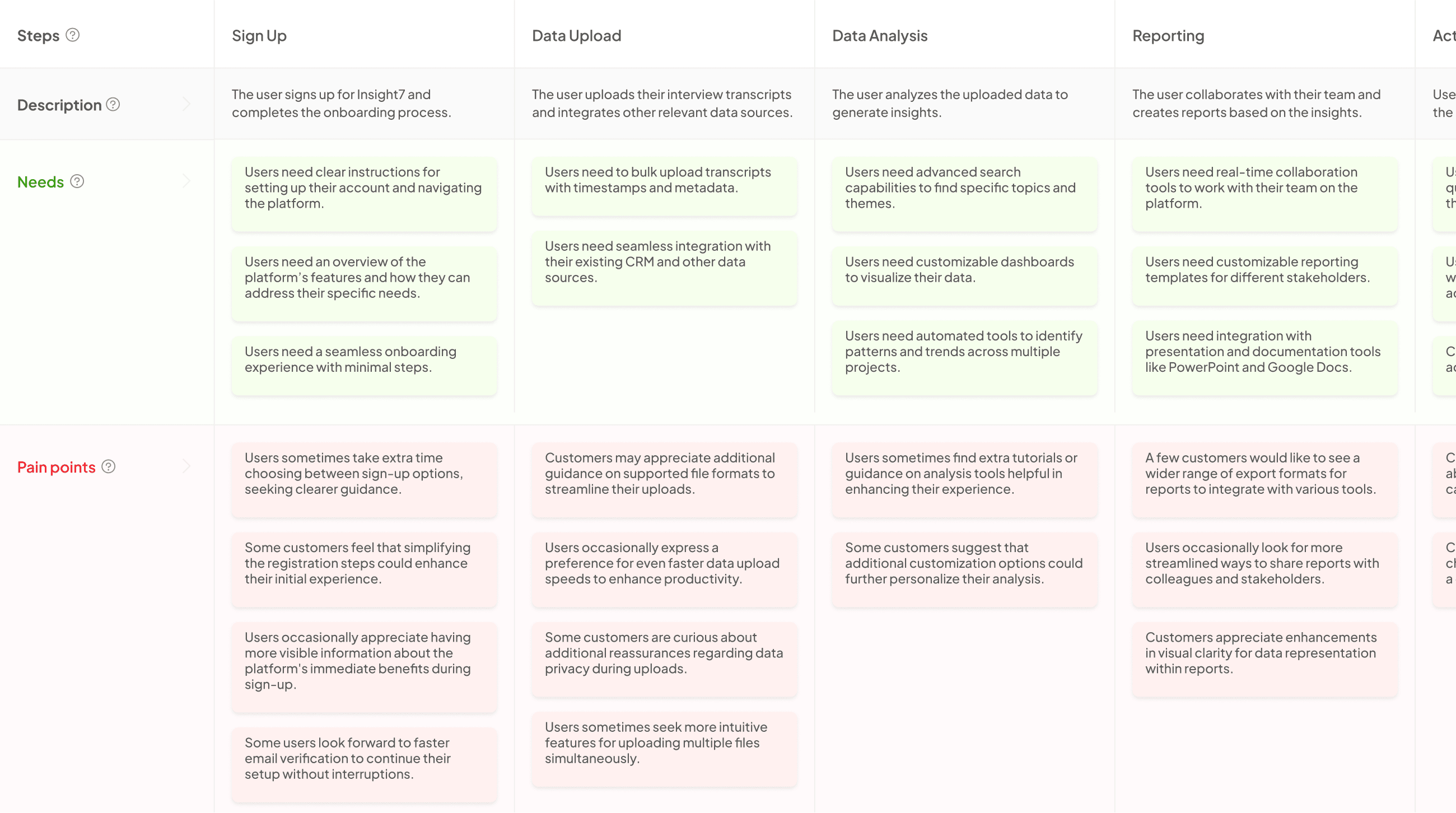
Understanding Predictive E-commerce Feedback
Predictive E-commerce Feedback serves as a vital tool for businesses aiming to understand customer behavior and preferences. The insights garnered through predictive analytics can enhance product offerings, leading to tailored experiences for consumers. By analyzing data from various touchpoints, companies can identify trends, preferences, and potential areas for improvement. This proactive approach allows e-commerce platforms to anticipate customer needs before they become apparent.
Understanding this feedback mechanism involves recognizing how data collection and analysis processes work. Through advanced algorithms, AI agents sift through vast amounts of customer interactions, generating actionable insights. These insights can include identifying common queries, analyzing sentiment from reviews, and recognizing product feedback. Ultimately, harnessing predictive E-commerce Feedback empowers businesses to make informed decisions that enhance customer satisfaction and loyalty, ensuring a competitive edge in the fast-paced online marketplace.
How Predictive E-commerce Feedback Works
Predictive e-commerce feedback operates through sophisticated algorithms that analyze customer interactions, preferences, and feedback. These AI-driven tools collect vast amounts of data, enabling businesses to anticipate customer needs and derive actionable insights. By identifying patterns and trends in consumer behavior, companies can refine their offerings and enhance the shopping experience.
To effectively utilize predictive e-commerce feedback, businesses often follow specific steps. First, they gather relevant customer data, such as previous purchases, browsing behavior, and feedback from surveys. Next, AI algorithms analyze this data to uncover insights and predictions about future customer preferences. This allows e-commerce platforms to tailor marketing strategies and optimize inventory, thereby improving customer satisfaction and driving sales.
Through this process, companies can not only enhance their operations but also foster stronger customer relationships by proactively addressing their needs.
The Role of AI Agents in Collecting Predictive E-commerce Feedback
AI agents play a pivotal role in gathering predictive e-commerce feedback, enhancing the shopping experience and informing business strategies. These intelligent systems meticulously analyze customer interactions, preferences, and behaviors, deriving insights that can forecast future trends. Through automated surveys and real-time engagement, AI agents efficiently collect feedback from numerous customers simultaneously, ensuring a broad data spectrum that strengthens predictions.
Moreover, the precision of these AI systems allows them to identify specific customer needs with remarkable accuracy. By utilizing advanced machine learning techniques, they discern patterns and anomalies within customer feedback. This information is vital for e-commerce businesses to adapt swiftly to shifting consumer sentiments. Ultimately, the role of AI agents is not merely about data collection; it is about transforming feedback into actionable insights that can drive strategic decisions, enhancing overall customer satisfaction and loyalty.
Generate Journey maps, Mind maps, Bar charts and more from your data in Minutes
Implementing AI Agents for Enhanced Predictive Customer Feedback
To implement AI agents effectively for enhanced predictive customer feedback, businesses must first focus on identifying key customer data points. This involves gathering essential information from various touchpoints, including purchase behavior, customer interactions, and feedback surveys. Understanding which data is most pertinent allows the AI agents to analyze actionable insights more efficiently. By honing in on user preferences and behaviors, organizations can craft tailored approaches that resonate with their customer bases.
Following data identification, employing sophisticated AI algorithms for feedback analysis is crucial. These algorithms can sift through vast amounts of information to unearth patterns and trends that human analysis might overlook. With the capacity to provide real-time analysis, AI agents facilitate a proactive approach to feedback, allowing organizations to adjust strategies promptly. Ultimately, this seamless integration of technology engenders a responsive system, ensuring businesses remain at the forefront of customer satisfaction and engagement.
Step 1: Identifying Key Customer Data Points
To effectively harness predictive e-commerce feedback, the first step involves keenly identifying key customer data points. Start by analyzing purchasing behavior, including frequency and volume of transactions, as well as customer demographics. Understanding these aspects allows businesses to tailor offerings to meet customer needs closely.
Next, prioritize customer interactions such as feedback, inquiries, and complaints. These types of data highlight pain points and preferences, paving the way for creating a personalized shopping experience. Additionally, segmenting customers based on various attributes, such as purchase history or engagement level, allows for more precise targeting. This structured approach ultimately forms a robust foundation for predictive analytics, enabling e-commerce platforms to not only understand their customers but also anticipate their future behaviors and preferences effectively.
Step 2: Employing AI Algorithms for Feedback Analysis
Employing AI algorithms for feedback analysis marks a significant advance in understanding customer sentiments in e-commerce. This phase involves utilizing machine learning models to sift through large datasets of customer feedback. By analyzing patterns in this feedback, businesses can gain insights into customer preferences, pain points, and overall satisfaction. This analysis is crucial as it enables companies to make data-driven decisions, enhancing customer experiences and driving sales.
💬 Questions about AI Agents for Predictive Customer Feedback in E-commerce?
Our team typically responds within minutes
To effectively implement AI algorithms for feedback analysis, consider the following key steps:
- Data Collection: Gather feedback from various sources, including reviews, surveys, and social media.
- Text Processing: Clean and preprocess the collected data to remove noise and irrelevant information.
- Sentiment Analysis: Apply sentiment analysis algorithms to determine the positive or negative tone of the feedback.
- Pattern Recognition: Use clustering techniques to identify common themes and trends in the feedback.
- Actionable Insights: Transform the analyzed data into actionable strategies that address customer concerns and leverage positive feedback.
By following these steps, businesses can refine their approach and enhance predictive e-commerce feedback, leading to improved customer satisfaction and loyalty.
Top Tools for Predictive E-commerce Feedback
In the realm of predictive e-commerce feedback, selecting the right tools is critical for enhancing customer insights. Several platforms excel in harnessing AI technology to extract actionable data from user interactions. Tools like insight7 offer comprehensive feedback analysis, enabling businesses to sift through vast amounts of customer data to identify trends and sentiments. Similarly, Sysomos combines social media analytics with customer feedback, allowing for a holistic view of consumer behavior.
Qualaroo stands out by providing targeted surveys that capture user thoughts in real-time, ensuring that insights stay relevant. Meanwhile, MonkeyLearn specializes in text analysis, helping businesses understand complex customer feedback through natural language processing. Lastly, Revuze offers automated insights, transforming raw data into understandable metrics that support strategic decision-making. Each of these tools plays a vital role in shaping effective predictive e-commerce feedback strategies. By leveraging these resources, businesses can closely align their offerings with customer preferences and expectations.
insight7
Predictive E-commerce Feedback plays a crucial role in shaping customer experiences in today's digital landscape. As e-commerce businesses strive to meet ever-evolving customer expectations, understanding feedback becomes paramount. The integration of AI agents accelerates this process by efficiently gathering and analyzing vast amounts of customer data. By doing so, they identify trends and preferences, enabling businesses to adapt quickly and drive improvements.
AI agents excel in converting raw customer interactions into actionable insights. By analyzing sentiment and behavioral patterns, these agents uncover valuable feedback that can inform product development, customer service strategies, and marketing initiatives. Moreover, this dynamic approach reduces the time spent on manual data analysis, ultimately enhancing collaboration and decision-making. As AI agents continue to evolve, businesses that embrace these technologies will gain a competitive edge in their pursuit of predictive e-commerce feedback.
Sysomos
In the realm of predictive e-commerce feedback, certain platforms stand out for their ability to aggregate and analyze customer insights effectively. These sophisticated tools ensure that e-commerce businesses can harness customer feedback at scale, translating data into actionable strategies. They excel in identifying patterns and trends within large volumes of data, making it easier for companies to respond to consumer needs swiftly.
Additionally, these systems support improved collaboration by centralizing insights, allowing teams across departments to access valuable customer signals without the chaos of scattered information. This streamlining is essential, as timely access to data can significantly enhance decision-making processes, putting businesses ahead of their competitors. Ultimately, leveraging such advanced tools for predictive e-commerce feedback empowers businesses to connect more meaningfully with their customers, fostering loyalty and driving sales growth.
Qualaroo
In the realm of Predictive E-commerce Feedback, a notable tool offers a focused approach to understanding customer experiences. It facilitates real-time feedback collection, allowing businesses to gather insights directly from users as they interact with their websites. Such timely information is crucial in identifying areas for improvement, ensuring that businesses can swiftly adapt to customer needs.
This tool employs targeted surveys that engage customers without disrupting their online shopping experience. By gathering specific opinions and suggestions, e-commerce platforms can refine their offerings and enhance customer satisfaction. Furthermore, these insights can support strategic decision-making, influencing everything from product development to marketing efforts. Ultimately, utilizing this resource empowers businesses to stay attuned to customer preferences, driving growth in today’s competitive e-commerce landscape.
MonkeyLearn
In the realm of predictive e-commerce feedback, advanced tools play a vital role in harnessing valuable customer insights. One such tool empowers businesses to easily access and analyze customer interactions without needing extensive training or expertise. This democratization of data ensures that employees across various functions can delve into the rich feedback available from customer communications, enhancing overall understanding.
Beyond basic analytics, this platform excels in extracting nuanced insights, such as pain points and customer desires. It visualizes conversations and captures evidence-backed feedback, allowing companies to identify key themes and issues swiftly. As a result, organizations can adapt based on real-time input, fostering a more responsive customer experience. By utilizing this powerful tool, e-commerce businesses can significantly enhance their predictive feedback processes, leading to improved decision-making and customer satisfaction.
Revuze
In the realm of predictive e-commerce feedback, the innovative use of AI tools has transformed the way businesses gather and analyze customer insights. Revuze specializes in automating this process, allowing e-commerce companies to efficiently tap into vast amounts of customer feedback data. The platform uses advanced algorithms to identify trends and sentiments within customer reviews, making it easier for businesses to understand their audience's needs and preferences.
Through its capabilities, Revuze enables e-commerce companies to anticipate customer behavior and make data-driven decisions. By leveraging AI to analyze feedback patterns, businesses can enhance their products and services, ultimately leading to improved customer satisfaction. This focus on predictive analytics in e-commerce is essential for staying competitive in a fast-paced digital landscape, where customer expectations continue to evolve.
Conclusion on the Future of Predictive E-commerce Feedback with AI Agents
As we look to the future of predictive e-commerce feedback, AI agents are set to revolutionize how businesses understand customer preferences. With advancements in machine learning and natural language processing, these agents will more accurately interpret feedback patterns and provide real-time insights, enhancing the overall consumer experience. This technology fosters a deeper connection between brands and buyers, allowing for tailored solutions that anticipate needs rather than react to them.
In this evolving landscape, the importance of specificity will become paramount. AI agents need precise parameters to generate meaningful insights, ensuring their recommendations align with brand goals. As organizations commit to refining these systems, the potential for predictive e-commerce feedback will grow, leading to more informed decision-making and improved customer satisfaction. Ultimately, embracing these AI-driven innovations holds the key to unlocking unprecedented growth in the e-commerce sector.
Predictive e-commerce feedback plays a crucial role in understanding customer sentiments and expectations. By leveraging advanced AI agents, businesses can analyze customer interactions to gain actionable insights. These insights enable companies to refine their products and services based on real-time feedback, ultimately enhancing customer satisfaction.
The process involves several stages to ensure effective feedback collection. Firstly, it's important to identify key customer data points, including both positive and negative feedback. Secondly, advanced AI algorithms can analyze this feedback to uncover trends and patterns. This systematic approach ensures that businesses are not only responding to current customer needs but are also able to predict future demands. Understanding this workflow makes predictive e-commerce feedback pivotal in driving customer-centric strategies within e-commerce platforms.
💬 Questions about AI Agents for Predictive Customer Feedback in E-commerce?
Our team typically responds within minutes