AI Agents for Predictive Customer Feedback Analysis
-
Bella Williams
- 10 min read
Predictive Feedback Analytics is revolutionizing the way organizations understand customer sentiments and preferences. By harnessing advanced AI algorithms, these analytics tools can sift through vast amounts of feedback data, uncovering patterns and insights that were once hidden in plain sight. This innovative approach not only enhances the ability to respond to customer needs but also fosters stronger connections between businesses and their clients.
AI agents play a crucial role in this transformational process, serving as intelligent intermediaries that analyze feedback in real-time. They facilitate the extraction of actionable insights, enabling organizations to make informed decisions that align with customer expectations. As businesses increasingly rely on data-driven strategies, understanding the intricacies of predictive feedback analytics will become essential for fostering customer loyalty and driving growth.
Extract insights from Customer & Employee Interviews. At Scale.
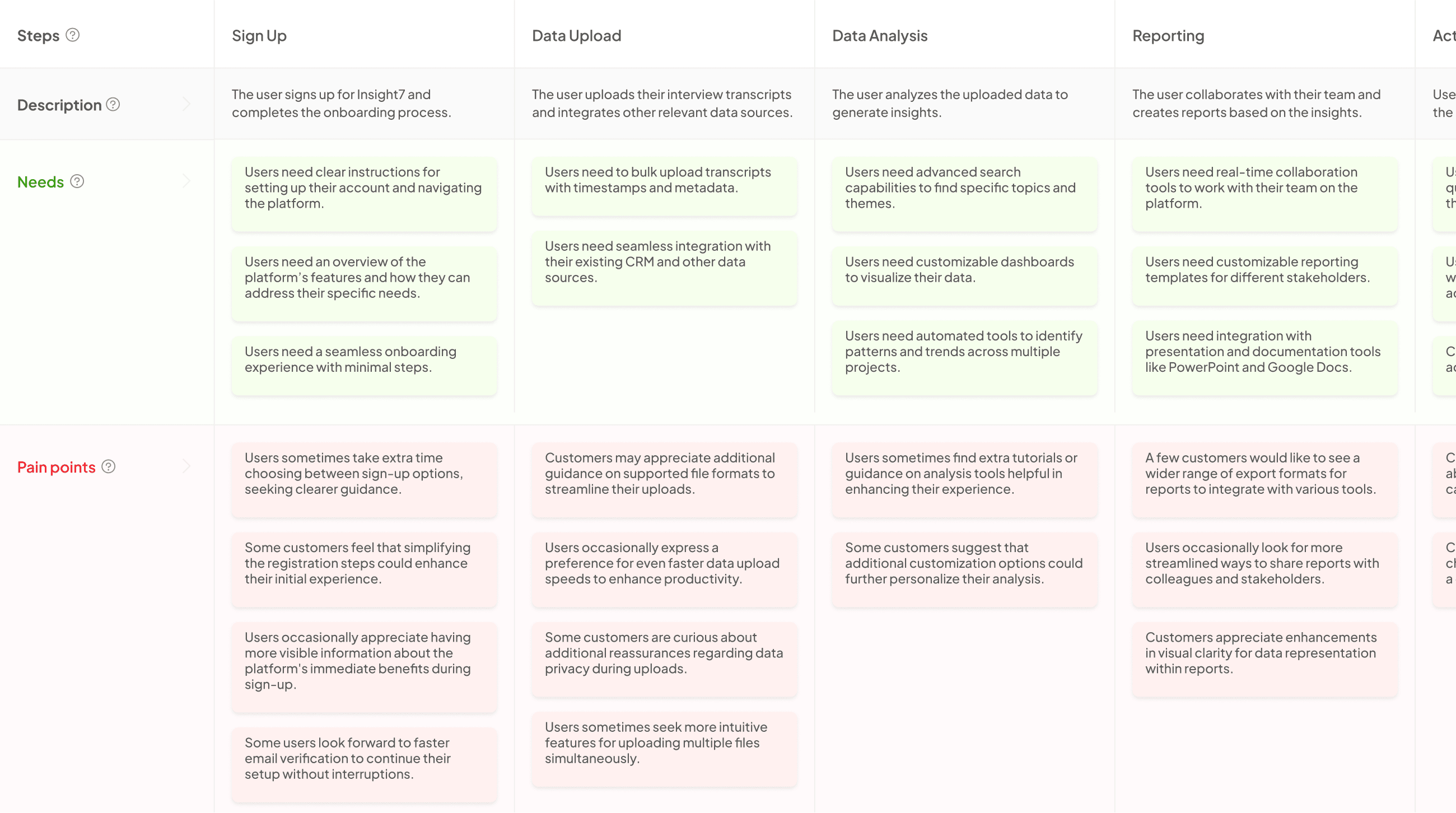
The Role of AI in Predictive Feedback Analytics
AI plays a pivotal role in enhancing predictive feedback analytics, enabling organizations to anticipate customer sentiments and behaviors. By analyzing vast amounts of data, AI agents can identify patterns and trends that would otherwise remain unnoticed. This capability empowers businesses to make informed decisions and tailor their strategies to meet customer needs effectively.
One primary advantage of AI in predictive feedback analytics is its ability to deliver real-time insights. With machine learning algorithms, AI agents can process feedback as it comes in, allowing companies to adjust their offerings or approach swiftly. Moreover, the precision of AI minimizes human error, ensuring that strategies are grounded in accurate data. By integrating AI agents into feedback analysis, organizations can transform raw data into actionable insights, enhancing their overall customer engagement and satisfaction.
Understanding Predictive Feedback Analytics
Predictive Feedback Analytics harnesses the power of data to anticipate customer needs and preferences. By analyzing feedback trends, organizations can decode sentiments, distinguishing between positive and negative comments efficiently. This level of insight helps businesses tailor their strategies, ultimately enhancing customer satisfaction. Understanding these analytics is crucial for businesses looking to adapt and thrive in competitive markets.
The process entails various methods, including data visualization and querying. First, visual tools allow stakeholders to see the feedback landscape clearly, making it easier to identify significant patterns. Next, querying capabilities facilitate targeted analysis on particular datasets, revealing insights that can inform product development decisions. Both these features are integral in transforming raw feedback into strategic actions, ensuring organizations remain aligned with customer expectations. By grasping the fundamentals of predictive feedback analytics, companies can drive meaningful changes to their offerings and increase customer loyalty.
How AI Agents Transform Feedback into Actionable Insights
AI agents play a pivotal role in transforming collected feedback into actionable insights. By analyzing vast amounts of customer interactions, these intelligent systems identify prevalent trends, preferences, and potential issues. This transformation revolves around several crucial steps that enhance predictive feedback analytics. First, AI compiles data from various customer touchpoints, ensuring a holistic view of feedback. Next, it employs natural language processing to extract meaningful patterns from unstructured data, such as comments and reviews.
Once patterns are recognized, AI agents can prioritize issues based on urgency and potential impact. This prioritization enables companies to respond swiftly to customer needs, ultimately improving satisfaction and loyalty. Finally, actionable insights derived from this process inform targeted strategies and initiatives, aligning products or services with customer expectations. The ability to convert simple feedback into strategic responses marks a significant advancement in customer experience management, showcasing the power of AI in contemporary business practices.
Generate Journey maps, Mind maps, Bar charts and more from your data in Minutes
Top Tools for Predictive Customer Feedback Analysis
In the realm of predictive customer feedback analysis, selecting the right tools is critical for transforming insights into impactful actions. A variety of innovative applications can enhance your understanding of customer sentiment and behavior. These tools leverage advanced algorithms to analyze large volumes of feedback, enabling businesses to react promptly to customer needs and preferences.
1. IBM Watson Analytics: Known for its robust natural language processing capabilities, this tool interprets customer feedback with precision. It helps businesses uncover trends and sentiments, pushing efficiency in decision-making processes.
2. MonkeyLearn: This platform focuses on text analysis, allowing users to extract actionable insights from customer comments or reviews quickly. Its user-friendly interface aids in the seamless categorization and sentiment analysis of feedback.
3. Qualtrics XM: Renowned for its extensive features, it offers a comprehensive solution for feedback collection and analytics. Qualtrics XM helps businesses measure sentiment through detailed survey analytics.
4. RapidMiner: As a powerful data mining tool, RapidMiner enables users to create predictive models that inform business strategies based on customer feedback trends. Its visual interface simplifies the analysis process.
By incorporating these tools, businesses can harness predictive feedback analytics to stay ahead of their competition and effectively address customer needs.
๐ฌ Questions about AI Agents for Predictive Customer Feedback Analysis?
Our team typically responds within minutes
Insight7: Leading the Charge in Predictive Feedback Analytics
In todayโs fast-paced environment, companies generate vast amounts of customer feedback, presenting an unprecedented opportunity for analysis. Insight7 is at the forefront of predictive feedback analytics, pioneering methods to harness this data effectively. By employing sophisticated AI agents, organizations can analyze customer interactions to derive actionable insights that drive business strategies. The need for real-time analysis is crucial, as timely insights allow companies to stay ahead of their competition.
Moreover, many businesses face challenges when relying on traditional methods for analyzing data. These methods often fall short, leaving companies unable to respond swiftly to customer needs. By embracing predictive feedback analytics, organizations can transcend these limitations, enabling seamless collaboration and efficient decision-making. This transformational approach not only improves the accuracy of insights but also elevates overall customer experience, ensuring a more proactive engagement. As AI agents continue to lead this charge, the landscape of customer feedback analysis will evolve, promising exciting possibilities for businesses willing to adapt.
Other Notable Tools for Feedback Analysis
In the realm of predictive feedback analytics, several tools complement the capabilities of AI agents by streamlining data analysis and enhancing insights. Understanding these additional resources can significantly improve how businesses interpret and respond to customer feedback. IBM Watson Analytics stands out as a powerhouse for its robust data visualization and predictive modeling features. With its machine learning capabilities, businesses can derive deeper insights from customer interactions.
Another valuable tool is MonkeyLearn, which excels in text analysis, allowing users to extract sentiments and themes from open-ended customer responses effectively. Qualtrics XM also plays a crucial role by providing an all-in-one platform for experience management, guiding companies to improve customer satisfaction based on real-time feedback. Lastly, RapidMiner offers an open-source environment for advanced data analytics, suitable for users looking to conduct complex analyses and predictive modeling. Each of these tools plays a unique role in enhancing predictive feedback analytics and can empower businesses to make informed decisions based on customer sentiments.
Tool 1: IBM Watson Analytics
IBM Watson Analytics serves as a powerful tool for organizations aiming to harness predictive feedback analytics effectively. By enabling users to analyze customer feedback through advanced data visualization techniques, it transforms raw information into actionable insights. The ability to categorize comments, whether positive or negative, allows businesses to gauge customer sentiment with precision. This sets the stage for informed decision-making about product improvements or new offerings.
Moreover, the platform supports complex queries across large datasets, facilitating a deeper understanding of customer behaviors. Users can compare feedback from different locations, providing clarity on geographic sentiment variations. This feature enables businesses to uncover patterns that might otherwise remain hidden. As organizations look to optimize their feedback systems, utilizing IBM Watson Analytics can significantly enhance their ability to derive useful predictions from customer feedback. This ultimately fosters a more customer-centric approach, driving business success through data-driven decisions.
Tool 2: MonkeyLearn
MonkeyLearn stands out as a user-friendly tool that simplifies the process of managing customer feedback. Its intuitive interface allows anyone in your organization to access valuable insights without prior training. As businesses strive for more transparent customer engagement, this tool enables teams to analyze and categorize large volumes of feedback rapidly, unlocking patterns and key trends.
With MonkeyLearn, users can upload call transcriptions and analyze them for pain points and customer desires. The tool distills data into digestible insights, making it easier to identify areas for improvement. Whether working on specific projects or scanning multiple datasets, its capabilities support the goals of predictive feedback analytics. By streamlining data gathering and assessment, every member of the organization can contribute to the collective understanding of customer needs, ultimately enhancing overall satisfaction and business outcomes.
Tool 3: Qualtrics XM
Qualtrics XM stands out as a potent resource in the realm of predictive customer feedback analysis. With its user-friendly interface, businesses can easily gather insights from qualitative and quantitative data. The platform empowers organizations to analyze customer interactions and feedback systematically, ultimately leading to enhanced decision-making processes. Companies can effortlessly import transcripts and apply real-time analytics to evaluate performance metrics and customer sentiment.
The significance of Qualtrics XM lies in its robust evaluation capabilities. Users can customize evaluation templates tailored to their specific business needs. This flexibility allows for precise analysis of customer service interactions, enabling organizations to identify problem areas and strengths in their engagement strategies. By harnessing predictive feedback analytics, businesses can transform customer insights into actionable strategies, enhancing customer satisfaction and loyalty. As a result, Qualtrics XM is essential for any organization seeking to advance its feedback analysis efforts effectively.
Tool 4: RapidMiner
RapidMiner is a powerful tool that supports predictive feedback analytics through its versatile data analysis capabilities. By helping users extract, process, and visualize feedback data, this platform allows businesses to uncover valuable insights from customer interactions. With its user-friendly interface, RapidMiner provides an accessible entry point for teams looking to harness data effectively.
One of the standout features of RapidMiner is its ability to conduct queries across extensive datasets. Users can compare feedback from different locations or product lines, identifying patterns and trends that inform future strategies. Additionally, the platform enables deep dives into specific feedback categories, allowing businesses to address both positive and negative comments comprehensively. Ultimately, RapidMiner empowers organizations to transform raw customer feedback into actionable strategies, enhancing customer satisfaction and driving informed decision-making.
Conclusion: The Future of Predictive Feedback Analytics with AI
In envisioning the future of predictive feedback analytics with AI, we see a landscape enriched by innovative capabilities. As AI agents evolve, their ability to analyze customer data will lead to deeper insights, enabling organizations to anticipate customer needs more effectively. This transition from reactive to proactive feedback management enhances responsiveness and creates opportunities for personalized experiences.
The future promises even greater integration of AI technologies, driving efficiency in data analysis and interpretation. With ongoing advancements, businesses can harness predictive feedback analytics to become more adaptive, meeting ever-changing customer expectations. Ultimately, the synergy of AI and feedback analytics will empower companies to foster stronger relationships, outperform competitors, and achieve long-term success.
๐ฌ Questions about AI Agents for Predictive Customer Feedback Analysis?
Our team typically responds within minutes