AI Agents for Predictive Customer Behavior Analysis in E-commerce
-
Bella Williams
- 10 min read
Predictive Shopping AI is reshaping the e-commerce landscape by offering unique insights into customer behavior. As shoppers increasingly seek personalized experiences, businesses must adapt to forecast these preferences effectively. The advent of AI enables companies to analyze vast amounts of consumer data, identifying trends and anticipating future purchasing decisions. This transformation is not merely about technology; it's about understanding what drives consumers to choose specific products at particular times.
In this section, we will explore how Predictive Shopping AI empowers retailers to harness actionable insights. By implementing advanced algorithms and machine learning techniques, businesses can refine their marketing strategies and enhance customer engagement. Ultimately, this intelligent approach fosters more meaningful interactions between brands and consumers, paving the way for a more dynamic e-commerce environment.
Extract insights from Customer & Employee Interviews. At Scale.
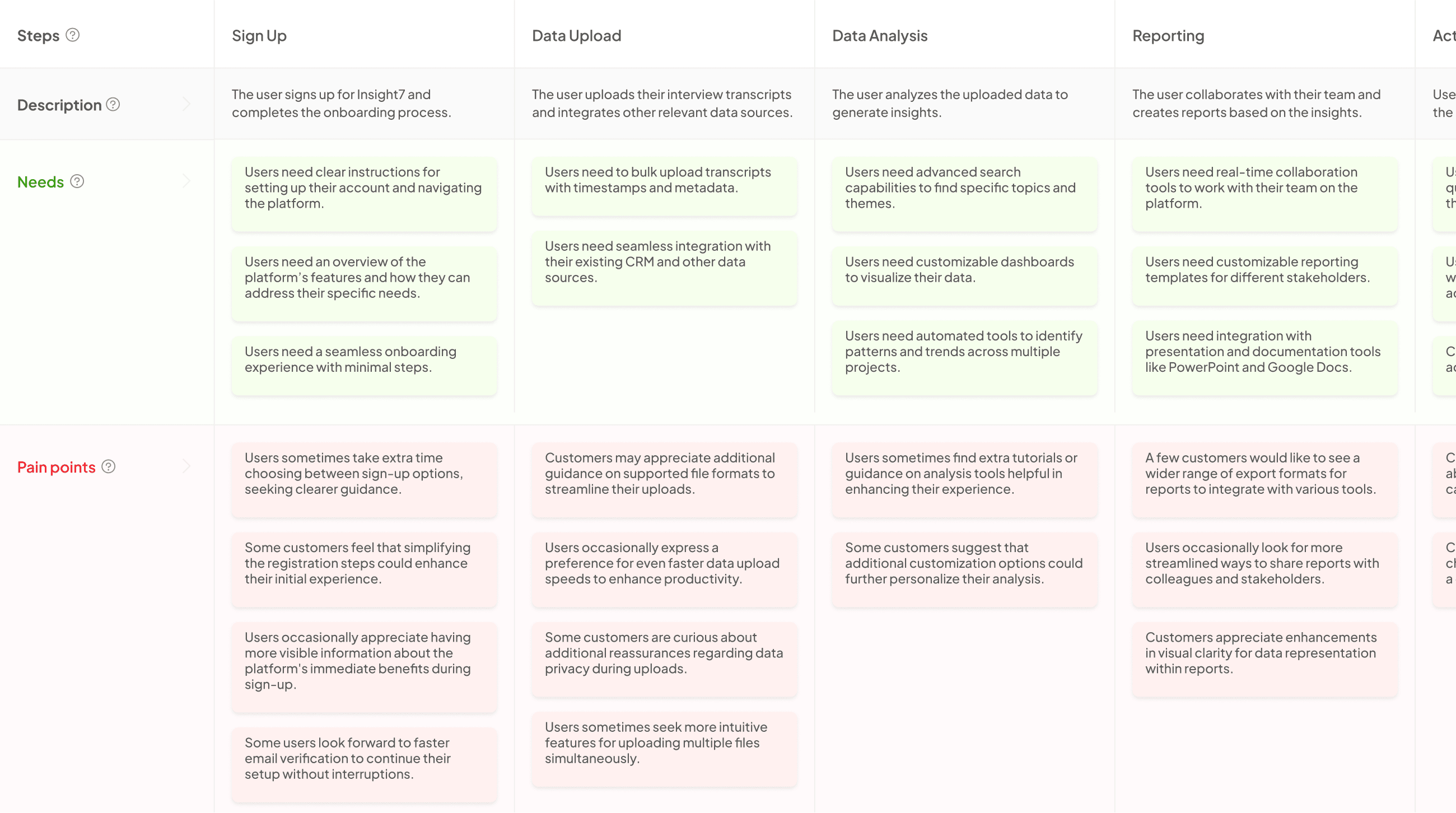
How Predictive Shopping AI Transforms Customer Insights
Predictive Shopping AI significantly transforms how businesses understand customer insights, shifting from static analysis to dynamic, actionable information. By analyzing vast amounts of consumer data, this technology identifies preferences and behaviors that were previously elusive. Rather than relying on generic customer profiles, businesses can leverage specific insights to tailor marketing strategies and product offerings, ultimately enhancing user experiences.
Furthermore, predictive algorithms anticipate purchase patterns, enabling e-commerce platforms to offer personalized recommendations that resonate with individual shoppers. This proactive approach not only increases conversion rates but also builds customer loyalty. Businesses equipped with robust predictive shopping AI can quickly adapt to evolving market trends and actively engage consumers. As a result, they gain a competitive edge, translating data into strategic actions that align with customer expectations, driving sustained growth in the ever-evolving retail landscape.
Understanding Customer Preferences with Predictive Shopping AI
Predictive Shopping AI plays a crucial role in shaping customer preferences by analyzing vast amounts of shopping data. By understanding the nuances of customer behavior, businesses can tailor their offerings to meet specific needs. This technology enables retailers to segment customers based on preferences, past purchases, and browsing habits, allowing for more personalized marketing strategies. As AI algorithms process customer interactions, they can identify trends that reveal what products are likely to appeal to different demographics.
Moreover, the integration of Predictive Shopping AI facilitates proactive engagement with customers. Retailers can predict future buying behaviors, which informs inventory management and promotional strategies. For instance, by analyzing patterns in data, businesses can anticipate demand surges for specific items, ensuring they are prepared with adequate stock. This level of insight enhances customer satisfaction and drives loyalty, ultimately leading to a more profitable e-commerce environment. Understanding customer preferences through this advanced technology creates a seamless shopping experience, paving the way for successful business strategies.
Anticipating Purchase Patterns through AI
Anticipating purchase patterns through AI involves analyzing vast amounts of customer data to identify trends and forecast future shopping behavior. Predictive Shopping AI employs advanced algorithms to interpret purchase history, browsing habits, and customer demographics, providing valuable insights into what products customers are likely to buy next. By examining this data, e-commerce platforms can create more personalized shopping experiences that enhance customer satisfaction and loyalty.
When utilizing Predictive Shopping AI, businesses can follow essential steps to refine their approach.
- Data Collection: Gather comprehensive customer data, including past purchases and interaction histories.
- Behavior Analysis: Utilize machine learning to uncover hidden patterns in purchasing behavior.
- Personalization: Tailor marketing strategies based on insights gathered, promoting relevant products to the right audience.
These strategies enable e-commerce businesses to stay ahead of customer needs, driving sales and enhancing overall shopping experiences. By harnessing the power of AI, retailers can maintain a competitive edge in an ever-evolving market.
Generate Journey maps, Mind maps, Bar charts and more from your data in Minutes
Key Components of Predictive Shopping AI Systems
Predictive Shopping AI systems are built on several key components that allow e-commerce platforms to analyze customer behavior effectively. The primary element is data collection and integration, which involves gathering information from multiple sources to create comprehensive consumer profiles. By employing tools like Salesforce Einstein, businesses can uncover behavioral patterns that inform strategy and enhance customer engagement.
Machine learning techniques further refine this analysis. The first step involves data preprocessing, where raw data is cleansed and transformed for better analysis. Next, model training and evaluation occur, allowing the AI to learn from historical data and make accurate predictions about future shopping behavior. Integrating platforms like Adobe Analytics and Google Cloud AI strengthens insights, creating a dynamic system that adapts and responds to evolving customer needs. Ultimately, these components work collaboratively to provide actionable insights and drive sales within e-commerce environments.
Data Collection and Integration
Data collection and integration are critical steps in developing effective Predictive Shopping AI systems. To harness valuable consumer insights, e-commerce platforms must gather data from multiple sources. This may include transaction records, customer interactions, and social media engagement. Each data point adds depth to understanding customer preferences and purchasing behaviors, which is essential for accurate predictions.
Once data is collected, integration becomes vital. Combining disparate datasets allows for a cohesive view of customer behavior. This can involve using advanced analytics tools to identify trends and patterns within the data. Moreover, continuous integration ensures that the Predictive Shopping AI remains current with changing consumer behaviors. Successfully navigating this process establishes a robust foundation for AI agents, enabling them to deliver personalized recommendations that enhance the shopping experience.
The Role of insight7
Predictive Shopping AI plays a crucial role in enhancing e-commerce by delivering valuable customer insights. By transforming raw customer data into actionable information, businesses can refine their marketing strategies and tailor offerings to individual preferences. This AI technology analyzes patterns in consumer behavior, anticipating future purchases based on historical data.
One of the significant advantages of this approach is the ability to streamline the decision-making process for businesses. Companies that successfully harness these insights can respond rapidly to customer needs, thereby gaining a competitive edge. Additionally, predictive shopping AI can identify trends that may not be immediately apparent, allowing businesses to innovate their product lines and enhance customer experiences. Effective utilization of these insights fosters deeper relationships with customers, ensuring they feel understood and valued throughout their shopping journey.
Understanding Consumer Data Profiles
Consumer data profiles play a pivotal role in shaping a brand's understanding of its audience. By gathering a multitude of data pointsโsuch as browsing history, purchasing habits, and demographic informationโretailers can create comprehensive profiles of their customers. These profiles allow brands to tailor their offerings and marketing strategies, making interactions more meaningful and relevant. Effective application of predictive shopping AI can significantly enhance these profiles by revealing patterns and behaviors that may not be immediately visible.
To truly grasp the importance of consumer data profiles, consider the following key aspects:
Data Source Integration: Gathering data from multiple sources, such as social media, past purchases, and website interactions, creates a well-rounded profile of customers.
Behavioral Insights: Analyzing purchasing behavior helps predict future trends, enabling proactive adjustments in inventory and marketing tactics.
Personalization: Tailored recommendations and offers increase customer satisfaction and loyalty by making shopping experiences unique to each individual.
Understanding these elements illustrates how predictive shopping AI can leverage consumer data effectively, resulting in a more engaging e-commerce experience.
Utilizing Salesforce Einstein for Behavior Analysis
๐ฌ Questions about AI Agents for Predictive Customer Behavior Analysis in E-commerce?
Our team typically responds within minutes
Salesforce Einstein plays a pivotal role in predictive shopping AI, providing businesses with the capability to analyze customer behavior and preferences. By employing robust machine learning algorithms, this platform can sift through vast datasets to uncover insights that traditional analysis might overlook. Businesses can anticipate customer needs, enhancing their ability to create personalized shopping experiences.
To effectively utilize Salesforce Einstein for behavior analysis, focus on three crucial elements. First, data integration allows for a comprehensive view of customer interactions across multiple touchpoints. Second, behavior tracking empowers businesses to identify patterns in shopping habits, enabling them to tailor marketing strategies effectively. Lastly, real-time analytics facilitates immediate adjustments to offerings based on customer behavior, ultimately driving higher conversion rates and customer satisfaction. This synergy between technology and consumer insight ensures that predictive shopping AI not only meets but anticipates the changing desires of customers in e-commerce.
Leveraging Adobe Analytics for Deep Insights
In today's e-commerce environment, understanding customer behavior is crucial. Utilizing advanced analytics tools can significantly enhance this understanding. Adobe Analytics plays a pivotal role in extracting valuable insights from customer data. By analyzing real-time data, businesses can identify trends, preferences, and pain points that influence customer behavior. This process informs strategies for better targeting and personalization, ultimately promoting customer satisfaction.
Moreover, Adobe Analytics integrates seamlessly with predictive shopping AI to forecast future buying patterns. This synergy enables businesses to proactively address customer needs, often before they are recognized by the consumer themselves. Employing these insights can improve the overall shopping experience by minimizing friction and enhancing engagement. Consequently, brands that effectively utilize Adobe Analytics position themselves to achieve a competitive edge in the rapidly evolving e-commerce landscape.
Harnessing the Power of Google Cloud AI
Harnessing advanced technology is crucial for e-commerce success, and Google Cloud AI plays a pivotal role in this arena. This platform offers powerful tools that provide businesses with insights into customer behavior, enabling brands to create tailored shopping experiences. With robust data processing capabilities, companies can harness vast amounts of customer interactions to understand nuanced shopping preferences.
In the realm of Predictive Shopping AI, Google Cloud AI's machine learning algorithms can forecast purchase patterns and consumer trends. Utilizing predictive models, retailers can make informed decisions on inventory management and personalized marketing strategies. Moreover, real-time data analysis allows businesses to adjust their tactics promptly, enhancing customer engagement and satisfaction. By leveraging Google Cloud AI, e-commerce companies can effectively transform raw data into actionable insights, driving better customer relationships and increased sales.
Exploring IBM Watson for Enhanced Predictions
As we explore advanced capabilities offered by cognitive computing, one standout option enhances predictions in customer behavior significantly. By integrating these intelligent systems into your e-commerce environment, you can deeply analyze past customer interactions, preferences, and trends. This process will uncover patterns that drive buying decisions, ultimately improving customer satisfaction and optimizing the shopping experience.
To elevate your predictive shopping experience, consider these key factors:
Data Mining Techniques: Effective data analysis narrows focus on specific customer segments and their behaviors. This contributes significantly to tailoring marketing efforts.
Natural Language Processing: This technology analyzes customer feedback, allowing businesses to gauge sentiment and refine product offerings by understanding emotions behind customer statements.
Predictive Models: By continuously learning from evolving data, AI can create accurate forecasts of future buying behaviors, ensuring your approach aligns with market trends.
Implementing these elements enables a comprehensive understanding of customer preferences, leading to improved decision-making and enhanced overall performance in predictive shopping.
Machine Learning Techniques in Predictive Shopping
Machine Learning Techniques in Predictive Shopping rely on sophisticated algorithms to analyze consumer behavior and forecast future purchases. These techniques allow businesses to gain insights into shopper preferences, enabling personalized experiences. A crucial first step in utilizing these techniques is data preprocessing, which involves cleaning and organizing the raw data for analysis. This stage is essential to ensure high-quality input for machine learning models.
Once the data is prepared, model training and evaluation commence. In this phase, various algorithms are applied to identify patterns in consumer behavior, such as recent purchase history or seasonal trends. Testing these models against existing data helps determine their predictive accuracy. By refining and validating these models, businesses can directly enhance their predictive shopping strategies, leading to better engagement and increased sales. In this ever-evolving e-commerce landscape, employing such machine learning techniques is vital for remaining competitive and meeting customer expectations.
Step 1: Data Preprocessing
Data preprocessing is a crucial first step in developing a Predictive Shopping AI. This phase involves cleaning and organizing the data collected from various sources, ensuring accuracy and consistency. Proper preprocessing helps to minimize noise and remove irrelevant information that could disrupt the AI model's performance. By standardizing data formats and filling in missing values, you create a reliable dataset that will significantly improve the quality of insights generated later.
Key processes in data preprocessing include data cleaning, transformation, and reduction. Data cleaning focuses on identifying and correcting errors within the dataset, such as duplicates or inconsistencies. Transformation involves normalizing data to a common scale, making it easier for the model to understand patterns. Finally, reduction techniques streamline the dataset, ensuring it is manageable and relevant for training the AI. By meticulously preparing your data, you set the foundation for effective predictive modeling in e-commerce.
Step 2: Model Training and Evaluation
In Step 2: Model Training and Evaluation, the process of refining predictive models begins. Here, we focus on creating a robust predictive shopping AI that effectively analyzes customer behavior. The first stage involves selecting appropriate algorithms based on the data collected. Models such as regression analysis, decision trees, and neural networks are commonly utilized, each offering unique strengths in analyzing consumer patterns.
Following the selection of algorithms, training the model on historical data is crucial. This training phase helps the AI learn from past customer interactions, enabling it to make informed predictions. Once trained, the model undergoes rigorous evaluation using various metrics like accuracy and recall. This assessment ensures that the predictive shopping AI provides reliable insights, aiding retailers in anticipating customer needs and optimizing their sales strategies. Implementing thorough training and evaluation processes secures the AI's effectiveness in driving e-commerce success.
Conclusion: The Future of E-commerce with Predictive Shopping AI
The future of e-commerce is increasingly intertwined with the capabilities of Predictive Shopping AI. As technology advances, businesses will harness AIโs potential to deeply understand and anticipate customer behavior. This shift will empower retailers to offer personalized shopping experiences that are responsive to individual preferences and trends.
In embracing Predictive Shopping AI, e-commerce platforms can look forward to optimized inventory management and improved customer satisfaction. By forecasting demand more accurately, retailers will not only enhance operational efficiency but also cultivate stronger customer relationships, ensuring they remain competitive in a rapidly evolving market.
๐ฌ Questions about AI Agents for Predictive Customer Behavior Analysis in E-commerce?
Our team typically responds within minutes