AI Agent for Predictive Behavioral Insights
-
Bella Williams
- 10 min read
Predictive Behavior Analytics in AI Agents reveals an exciting intersection of technology and human behavior, enabling businesses to gain insights into user actions. With the power of AI, organizations can analyze vast amounts of data, making sense of trends and patterns that typically remain hidden. This analytical capability allows companies to anticipate consumer needs, ultimately leading to improved decision-making processes.
Understanding the principles behind predictive behavior analytics is essential for those looking to harness its benefits. By leveraging advanced algorithms and machine learning, AI agents can provide a glimpse into future behaviors, equipping businesses with the tools necessary to stay competitive in fast-evolving markets. As organizations adopt these technologies, they unlock new possibilities for enhancing user engagement and tailoring experiences that resonate authentically with their audience.
Analyze & Evaluate Calls. At Scale.
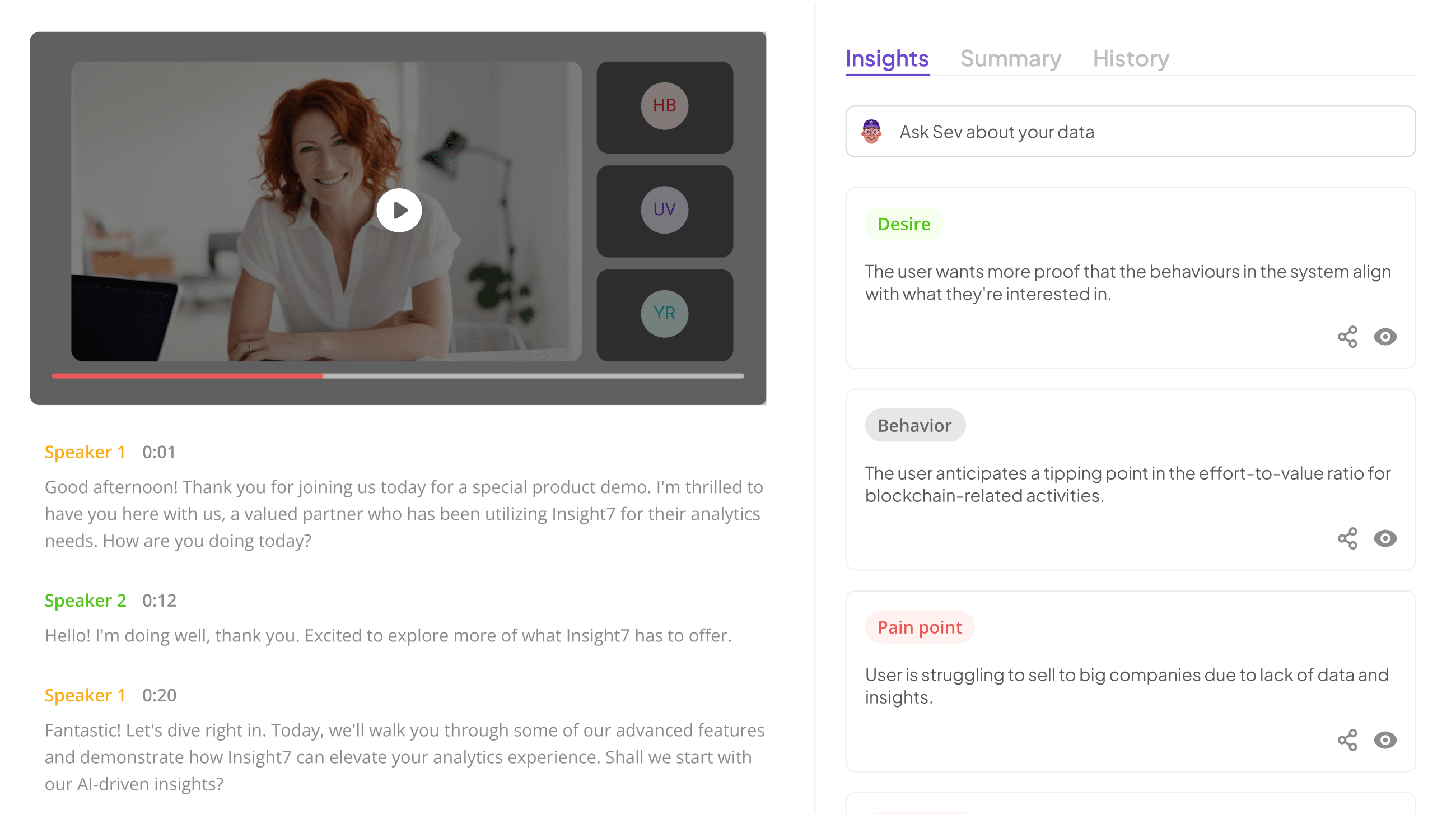
The Science Behind Predictive Behavior Analytics
Predictive Behavior Analytics combines data science, AI, and behavioral theories to forecast future actions based on past behaviors. At its core, this analytics approach focuses on identifying patterns in large datasets, allowing organizations to better understand customer motivations and predict their next moves. By employing algorithms that analyze historical data, businesses can uncover trends and insights that guide strategic decisions.
AI plays a crucial role in enhancing predictive insights by automating the data analysis process. Traditional methods often struggle to keep pace with the volume and complexity of modern data, whereas AI-driven tools rapidly analyze consumer signals. Organizations leveraging predictive behavior analytics not only gain a competitive advantage but also ensure timely and effective responses to changing market dynamics. Ultimately, the fusion of behavioral science and artificial intelligence empowers businesses to tap into valuable insights that drive informed decision-making.
Understanding the Core Concepts of Predictive Behavior Analytics
Predictive Behavior Analytics is a powerful tool that aims to understand and forecast human actions based on historical data. At its core, this approach leverages patterns derived from past behavior to predict future actions, making it invaluable across various sectors. By examining this data, organizations can anticipate user needs, enhance decision-making processes, and tailor their strategies accordingly.
Central to Predictive Behavior Analytics are several foundational concepts. First is data collection, where diverse datasets provide the raw material for analysis. Next is data analysis, transforming this information into actionable insights through various algorithms and models. Lastly, the application phase utilizes these insights to inform marketing strategies, customer engagement, and product development. Understanding these elements equips businesses to harness predictive analytics effectively, ultimately driving growth and improving user experiences.
The Role of AI in Enhancing Predictive Insights
Artificial Intelligence (AI) plays a pivotal role in enhancing predictive insights through the analysis of complex behavioral patterns. By utilizing Predictive Behavior Analytics, AI can dissect vast amounts of data to identify trends and forecast future behaviors. This capability allows organizations to make informed decisions based on reliable predictions about customer actions, thereby improving overall strategic planning.
The AI systems process data from various sources, which enriches the insights gained. This leads to better engagement strategies, optimized marketing efforts, and improved customer experiences. Moreover, the continual evolution of machine learning algorithms ensures that these predictive models become increasingly accurate over time. Consequently, organizations can remain proactive rather than reactive in their operations, adapting quickly to changing consumer needs while fostering stronger relationships with their audiences. AI's ability to translate data into actionable insights positions it as an invaluable tool for businesses aiming for growth and sustainability.
Extract insights from interviews, calls, surveys and reviews for insights in minutes
Applications of Predictive Behavior Analytics in AI
Predictive Behavior Analytics plays a vital role in artificial intelligence applications, enabling companies to anticipate future behaviors based on historical data. By analyzing patterns and trends, businesses can tailor their strategies to meet customer needs more effectively. This powerful approach enhances decision-making across various sectors, from marketing to product development.
Several key applications of Predictive Behavior Analytics stand out. Firstly, it aids in customer segmentation, allowing organizations to identify distinct groups based on behavior and preferences. Secondly, predictive analytics can forecast sales trends, helping companies optimize inventory and staffing. Additionally, it is instrumental in improving customer service by anticipating issues before they arise, thereby increasing customer satisfaction. Lastly, this analytics approach supports risk management by identifying potential areas of concern early on. Through these applications, businesses can unlock valuable insights that drive strategic initiatives and improve overall performance.
Real-World Case Studies of Predictive Behavior Analytics
Real-world case studies of predictive behavior analytics showcase its practical applications across various sectors. One prominent example emerges in the retail industry, where businesses utilize this technology to forecast customer purchasing patterns. By analyzing previous consumer behaviors, companies can tailor their marketing strategies effectively, enhancing customer engagement and driving sales. This approach not only helps in meeting customer needs but also significantly boosts operational efficiency.
Another compelling case is within the healthcare sector, where predictive behavior analytics is leveraged to improve patient care. By examining patient data, healthcare providers can identify trends and potential health risks, allowing for proactive interventions. This capability not only improves patient outcomes but also streamlines resource allocation, ultimately fostering a more responsive healthcare system. These cases illustrate the transformative potential of predictive behavior analytics, underscoring its value in optimizing decision-making across diverse disciplines.
Steps to Implementing Predictive Behavior Analytics in Your Systems
Implementing predictive behavior analytics in your systems is a structured process that begins with data collection and preparation. Start by identifying the datasets relevant to your business goals, ensuring they are clean and formatted for analysis. This foundational step is crucial, as the quality of your data directly impacts the insights derived from predictive analytics. With robust data in hand, proceed to select the appropriate AI models. Consider the unique needs of your organization and the questions you seek to answer through these models.
Once you have chosen your models, the next step involves integrating these into your existing systems. Ensure that predictive insights are easily accessible to stakeholders, facilitating informed decision-making. Regular monitoring of these insights will help maintain accuracy and relevance over time. By following these steps diligently, you can effectively leverage predictive behavior analytics to enhance your operational capabilities and drive strategic initiatives.
Step 1: Data Collection and Preparation
Data collection and preparation are foundational steps in developing an effective AI agent for predictive behavior analytics. Initially, it is crucial to gather diverse data sources that accurately represent the behaviors and preferences of your target audience. This data can include customer interactions, surveys, transaction histories, and social media engagement. By ensuring a rich dataset, you lay the groundwork for impactful predictive modeling.
Next, the organization and cleaning of this data are essential for proper analysis. Removing duplicates, addressing missing values, and standardizing formats will enhance data quality. Following this, segmenting the data into relevant categories can facilitate more targeted insights. This ensures the AI can identify patterns and correlations among different behaviors effectively. Ultimately, thorough data collection and meticulous preparation significantly boost the accuracy and reliability of the insights generated, leading to informed decision-making based on predictive behavior analytics.
Step 2: Choosing the Right AI Models
Choosing the right AI models is crucial for effective predictive behavior analytics. It involves analyzing your specific needs and determining the characteristics of the data you have. Different models excel at distinct tasks, so identifying whether you require classification, regression, or clustering models is essential. For instance, classification models can help segment customer behaviors, while regression might better suit forecasting trends.
When selecting AI models, considering the complexity of your dataset is vital. Simple models may suffice for straightforward problems, while complex scenarios may necessitate advanced algorithms like neural networks or ensemble methods. Each model has its strengths and weaknesses, so it's beneficial to test several options to discover which one yields the most accurate predictions. By aligning the choice of AI models with your objectives, you set a foundation for generating meaningful insights from your predictive behavior analytics efforts.
Step 3: Integrating and Monitoring Predictive Insights
Integrating and monitoring predictive insights is essential for leveraging responsive and actionable data. Once predictive behavior analytics are generated, the next step is to embed these insights into existing business practices effectively. This integration requires careful alignment with organizational workflows and decision-making processes. By making insights accessible to all stakeholders, businesses can foster a culture of data-driven decision-making that enhances strategy formulation.
Regular monitoring of these predictive insights is equally crucial. It involves continually evaluating the accuracy and relevance of the insights gained. Adapting to new data or shifts in customer behavior ensures that predictive models remain effective and insightful. Organizations should set up performance metrics to assess the utility of the predictions actively. This cyclical process of integration and monitoring solidifies the foundation for ongoing improvement and innovation in behavior analytics, ensuring that businesses not only respond to trends but also anticipate them effectively.
Top Tools for Predictive Behavior Analytics
To effectively utilize predictive behavior analytics, understanding available tools is essential. Each tool offers distinct features that cater to varied analytical needs, ensuring businesses can derive actionable insights efficiently. Highlighting a selection of the top tools aids organizations in making informed decisions regarding the implementation of predictive analytics within their operations.
Insight7 – This platform streamlines data processing and visualization, making insights easily accessible without needing technical expertise. Users can analyze customer interactions seamlessly and gather essential feedback.
💬 Questions about AI Agent for Predictive Behavioral Insights?
Our team typically responds within minutes
IBM Watson Analytics – Known for its advanced machine learning capabilities, this tool provides powerful predictive insights by analyzing large datasets, helping businesses anticipate customer behaviors and trends.
Salesforce Einstein Analytics – This user-friendly tool integrates deeply with Salesforce, enabling companies to leverage customer data for in-depth behavior analysis and prediction.
RapidMiner – Featuring robust data science capabilities, RapidMiner allows businesses to build predictive models rapidly and visualize outcomes, facilitating data-driven decision-making.
SAS Predictive Analytics – A comprehensive solution offering sophisticated analytics capabilities, SAS helps organizations explore complex relationships between variables to predict future behavior effectively.
Leveraging these tools strengthens predictive behavior analytics efforts, empowering organizations to optimize operations and enhance customer experiences.
insight7
In this section, we delve into the intricate workings of predictive behavior analytics, focusing on its pivotal role in informing decision-making processes. Predictive behavior analytics utilizes sophisticated algorithms to forecast user actions, thus enabling businesses to anticipate needs and adjust accordingly. By analyzing patterns within customer data, organizations can unlock valuable insights that influence product development, marketing strategies, and customer engagement efforts.
To harness predictive behavior analytics effectively, follow these key steps:
Data Collection and Preparation: Begin by gathering relevant data while ensuring its accuracy and consistency. Clean, organized datasets lay the foundation for meaningful predictions.
Choosing the Right AI Models: Select AI models best suited for your predictive analytics goals, considering factors such as complexity, scalability, and the specific insights sought.
Integrating and Monitoring Results: Implement the chosen models within your systems, ensuring continuous monitoring to refine predictions and improve decision-making over time.
By adopting these steps, businesses can put themselves ahead of the competition, enhancing their ability to respond proactively to customer behaviors.
IBM Watson Analytics
IBM Watson Analytics offers significant capabilities for understanding and interpreting complex datasets. It provides users with intuitive visualizations and tools to uncover insights through predictive behavior analytics. By transforming raw data into actionable insights, organizations can make informed decisions that shape future strategies.
Through the platform, users can explore various data dimensions, enabling the identification of trends and patterns in customer behavior. Features like natural language processing allow for conversational queries, making data exploration more accessible. Additionally, by analyzing positive and negative customer feedback, businesses can better align their offerings with consumer expectations. This empowers teams to proactively respond to market demands and improve their products or services. Integrating IBM Watson Analytics into your workflow can elevate your organization’s predictive analysis capabilities, ultimately driving growth and innovation.
Salesforce Einstein Analytics
Salesforce Einstein Analytics offers powerful capabilities in the realm of Predictive Behavior Analytics. Designed to empower users, this tool leverages AI-driven insights to analyze past behaviors and project future trends. Essentially, it transforms complex data into intuitive visualizations that facilitate understanding of behavioral patterns.
With Einstein Analytics, businesses can delve into customer interactions and preferences, allowing for informed decision-making. The platform's robust data integration capabilities enable seamless connections to existing systems, enriching the analysis process. Users can customize dashboards to highlight key metrics, ensuring that the focus remains on actionable insights. This dynamic approach enhances the ability to forecast customer behavior, which is crucial for crafting effective strategies.
Incorporating Salesforce Einstein Analytics can optimize engagement and improve customer satisfaction by anticipating needs and preferences. By understanding behavioral patterns, organizations can tailor their offerings, ultimately driving growth and fostering loyalty.
RapidMiner
RapidMiner serves as a powerful tool in the realm of predictive behavior analytics. This platform offers a user-friendly interface that allows analysts to visualize and explore complex datasets effectively. Analysts can seamlessly manipulate the data, conducting exploratory analyses that reveal hidden patterns and trends among various customer behaviors.
Moreover, RapidMiner enables the integration of advanced machine learning models, enhancing the accuracy of predictive insights. Through its capabilities, users can dive deep into customer interactions across different segments—understanding sentiments from feedback, identifying positive and negative patterns, and predicting future behaviors. This rigorous analysis can significantly inform product development, ensuring that insights derived from data guide strategic business decisions comprehensively. As organizations aim to unlock valuable customer insights, RapidMiner stands out as a critical asset in transforming data into actionable intelligence.
SAS Predictive Analytics
SAS Predictive Analytics offers powerful tools that bring clarity to complex data through predictive behavior analytics. By utilizing historical data, businesses can identify patterns and trends crucial for decision-making. This analytic capability empowers organizations to forecast future behaviors, enabling them to tailor their strategies effectively.
To effectively implement SAS Predictive Analytics, organizations should consider three essential steps. First, the process begins with data collection and preparation, which ensures that the data used is clean and relevant. Second, selecting the right AI models is vital, as this choice impacts the accuracy of predictions. Finally, integrating and monitoring these insights will allow businesses to adapt their strategies and respond proactively to changing dynamics. By embracing SAS Predictive Analytics, organizations can unlock valuable behavioral insights that drive informed decision-making and enhance overall performance.
Conclusion: Harnessing AI for Enhanced Predictive Behavioral Insights
The integration of AI in predictive behavior analytics marks a significant advancement in understanding human actions and decisions. By harnessing vast data and sophisticated algorithms, businesses can gain deeper insights into customer preferences and trends, enhancing their decision-making processes. This technology allows for the identification of patterns that were previously undetectable, empowering organizations to anticipate needs and personalize experiences more effectively.
Moreover, the democratization of access to predictive behavior analytics tools means that insights are no longer confined to data experts. Employees across various departments can now engage with these insights, fostering a culture of data-driven decision-making. As organizations adopt AI-enhanced predictive analytics, the potential to refine strategies and better meet customer expectations continues to grow. Embracing these capabilities will ultimately lead to more informed business practices and improved outcomes.
Analyze & Evaluate Calls. At Scale.
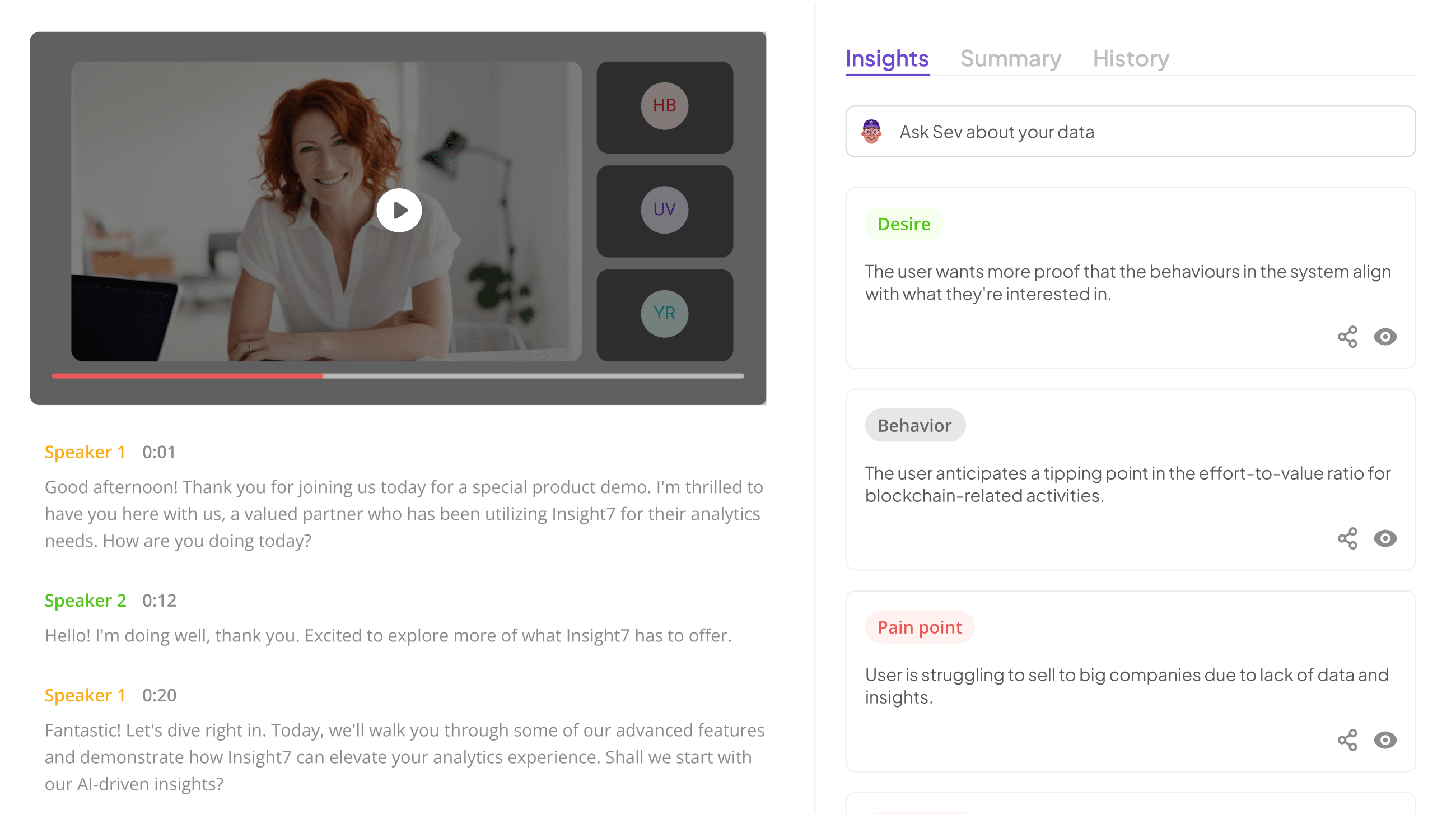
💬 Questions about AI Agent for Predictive Behavioral Insights?
Our team typically responds within minutes