AI Academic Research Report Generator: How it Works
-
Bella Williams
- 10 min read
Automated Research Synthesis is transforming the way researchers compile and analyze vast amounts of academic literature. In an age where information overload is common, these AI systems work diligently to extract relevant insights, ensuring a more streamlined research process. By leveraging advanced algorithms, they automate the synthesis of research findings, freeing scholars to focus on interpretation and innovation rather than data gathering.
The significance of Automated Research Synthesis lies not only in efficiency but also in accuracy. It aids scholars in comprehensively reviewing existing studies while identifying gaps for future exploration. As researchers increasingly rely on these tools, understanding their mechanisms and capabilities will enhance the academic landscape, driving insightful and impactful research outcomes.
Transcribe & extract insights from interviews. At Scale.
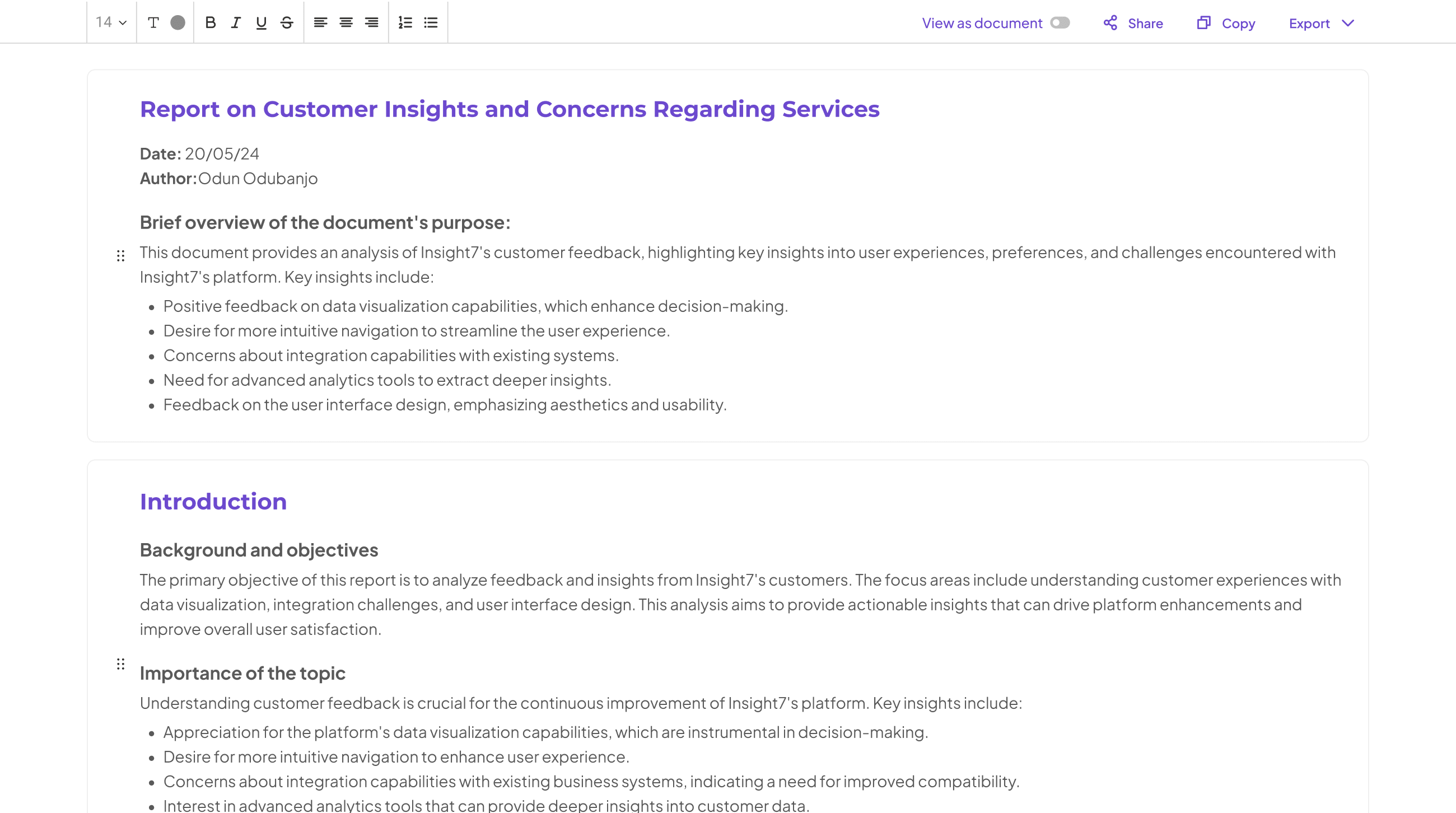
The Mechanics of Automated Research Synthesis
Automated Research Synthesis plays a crucial role in enhancing the efficiency and accuracy of academic research report generation. It acts as a bridge between vast pools of existing literature and the final, polished report. The process begins with the collection of diverse data sources, ensuring a comprehensive perspective. This data is subsequently refined through advanced techniques to ensure clarity and relevance.
Once the data is gathered, Natural Language Processing (NLP) techniques analyze the existing literature. By comprehending various contexts and semantics, AI can identify key themes and patterns. The synthesis of this information unfolds through machine learning algorithms that further clarify insights. This systematic approach not only accelerates research but also enhances the quality of findings, resulting in informative reports that meet specific academic standards.
The journey of Automated Research Synthesis ultimately transforms raw data into valuable insights, streamlining the research process from start to finish.
How AI Analyzes Existing Literature
AI's ability to analyze existing literature relies heavily on advanced techniques to provide insights into academic research. By utilizing Natural Language Processing (NLP), AI can comprehend and interpret large volumes of text, summarizing key findings and extracting relevant information. This process involves identifying patterns and trends in the literature, facilitating a comprehensive understanding of the topic at hand. The application of these algorithms enables AI to synthesize different research perspectives seamlessly.
Incorporating machine learning further enhances this capability, as the algorithms continuously improve their accuracy over time. They learn from the data they process, refining their interpretations and recommendations. As a result, Automated Research Synthesis becomes an efficient method for generating academic reports. Users benefit from a streamlined approach, allowing them to focus on critical insights rather than manual data analysis. This transformation empowers researchers to stay updated with the latest advancements and to produce reports that are both reliable and informed.
- Understanding Natural Language Processing (NLP) Techniques
Natural Language Processing (NLP) techniques play a crucial role in Automated Research Synthesis, enabling AI systems to comprehend and interpret human language effectively. By utilizing NLP, AI can analyze vast amounts of literature quickly, identifying key themes, concepts, and relationships that inform academic research. This process not only enhances data retrieval but also aids in distilling complex information into clear insights, making research synthesizing significantly more efficient.
NLP encompasses various methods, including tokenization, sentiment analysis, and named entity recognition. Tokenization breaks text into manageable parts, facilitating easier analysis. Sentiment analysis gauges the emotional tone behind the text, while named entity recognition identifies important subjects within the research. By leveraging these techniques, AI can transform bulky datasets into structured knowledge formats, significantly enriching the quality of research reports generated. Understanding these NLP techniques empowers researchers to effectively employ AI in their academic endeavors.
- The Role of Machine Learning Algorithms in Synthesizing Research
Machine learning algorithms play a crucial role in automated research synthesis by efficiently analyzing large volumes of academic literature. These algorithms can identify patterns, extract relevant findings, and summarize complex information, transforming extensive research data into coherent summaries. This automation streamlines the process, allowing researchers to quickly gather insights without wading through numerous articles.
The integration of machine learning in synthesizing research significantly enhances the quality and speed of literature reviews. For instance, algorithms can prioritize studies based on relevance, ensuring that the most pertinent information is highlighted. Additionally, they can detect potential biases and inconsistencies within the literature, offering a more accurate synthesis. Ultimately, machine learning algorithms facilitate a comprehensive understanding of existing research, empowering academics to build upon established knowledge and drive future innovations.
Step-by-Step Process of Generating a Report
Generating a report through automated research synthesis involves a systematic approach that ensures consistency and quality. This process begins with the collection of data and relevant literature, which serves as the groundwork for the report. Researchers utilize various databases and sources to gather information pertinent to their topic, ensuring a comprehensive foundation for analysis.
Next, NLP-based analysis and interpretation take center stage. This involves employing advanced algorithms designed to process and understand human language. As the analysis unfolds, an initial draft of the report is drafted. This draft is then subject to a review and refinement process, allowing for adjustments and enhancements based on feedback and additional insights. Ultimately, this step-by-step approach ensures that the final report is not only accurate but also insightful, fulfilling the objectives of automated research synthesis.
- Collection of Data and Relevant Literature
When embarking on the collection of data and relevant literature, the focus centers on gathering comprehensive evidence to support effective research synthesis. This initial phase involves identifying credible sources and extracting pertinent information that aligns with the research objectives. Ensuring a diverse range of literature enhances the depth and validity of the analysis, allowing researchers to draw meaningful insights.
The collection process encompasses various methods such as keyword searches, database inquiries, and reviewing existing publications. Once the data is gathered, it serves as a foundation for Automated Research Synthesis, enabling efficient analysis and interpretation. This systematic approach not only streamlines the research workflow but also fosters a more thorough understanding of the subject matter. In essence, a well-executed collection phase lays the groundwork for insightful and impactful academic research reports.
- NLP-Based Analysis and Interpretation
In the realm of automated research synthesis, NLP-based analysis and interpretation play a crucial role. This section delineates how advanced natural language processing techniques transform vast amounts of literature into coherent insights. By systematically analyzing texts, NLP algorithms can identify patterns and trends that may not be immediately evident to human researchers. As a result, researchers can quickly understand the existing body of work in their field, facilitating a more informed synthesis of knowledge.
The process involves several key steps. First, NLP techniques extract essential information from diverse academic sources, consolidating data into a manageable form. Second, the interpretation of these findings enables researchers to pinpoint gaps in existing research, guiding future inquiries. Efficiently translating raw data into meaningful conclusions, NLP removes barriers traditionally associated with manual analysis. Consequently, automated research synthesis fosters enhanced collaboration and drives actionable insights, ultimately refining research strategies in a competitive landscape.
- Drafting the Initial Report
Creating the initial report is a crucial phase in the automated research synthesis process. It involves assembling insights derived from literature and multiple data sources into a cohesive document. The AI system begins by identifying key themes and findings, ensuring that all essential information is accurately captured. By turning complex data into a structured format, the initial report acts as a foundation for further analysis and refinement.
Once the raw content is drafted, the emphasis shifts to clarity and coherence. This step often requires multiple revisions to enhance readability and ensure that the data supports logical conclusions. Feedback loops may be established to incorporate user insights, allowing for continuous improvement of the report structure. Overall, drafting the initial report harnesses technology to streamline research processes, ensuring that scholars can focus more on interpretation and application rather than manual data compilation.
- Review and Refinement Process
The review and refinement process is essential for ensuring the quality and accuracy of the AI-generated academic research reports. In this stage, the initial drafts undergo thorough scrutiny to identify any inaccuracies or areas needing improvement. Human oversight is crucial here, as it allows experts to enhance the context and reliability of the findings presented.
The process typically involves several important steps. First, reviewers analyze the synthesized data for coherence and relevance. Next, specific feedback is gathered to address identified gaps or deficiencies. Finally, the report is refined based on this feedback, ensuring that the final product meets academic standards. This approach guarantees that automated research synthesis not only generates relevant content but also maintains a high level of trustworthiness and credibility in academic discourse.
Generate Detailed Reports from Your Qualitative Data in Minutes.
Top Tools for Automated Research Synthesis in AI
Automated Research Synthesis is transforming how researchers approach their work. There are several powerful tools available that streamline the synthesis of academic literature, making data gathering and analysis more efficient and effective. These tools utilize advanced technologies like natural language processing and machine learning, allowing users to analyze vast amounts of research quickly.
๐ฌ Questions about AI Academic Research Report Generator: How it Works?
Our team typically responds within minutes
Among the top tools, we find a variety of functionalities tailored for different academic needs. Each tool excels in unique areas, such as enhanced data visualization or comprehensive literature reviews. For example, some may offer automated summarizing features that condense lengthy studies into concise insights. Others might excel at identifying research gaps or cross-referencing citations.
In choosing the right tool, researchers should consider their specific requirements and objectives. By leveraging these advanced capabilities, they can significantly improve the quality and accuracy of their research reports. Automated Research Synthesis tools not only save time but also empower researchers with richer insights.
insight7
Automated Research Synthesis significantly transforms the landscape of academic research by streamlining how researchers gather and analyze data. This process begins with the robust collection of data and relevant academic literature, which forms the backbone of insightful reports. Once the data compilation is complete, Natural Language Processing (NLP) techniques come into play, allowing the system to interpret and engage with text as a human would. This leads to interpretations that accurately reflect the underlying meanings within the literature, paving the way for comprehensive synthesis.
Following analysis, the generated reports undergo a drafting and review phase, ensuring clarity and coherence. This method allows researchers to save time and focus on more strategic tasks rather than getting bogged down by endless data interpretations. In essence, Automated Research Synthesis facilitates a more efficient workflow, optimizing the way insights are translated into impactful academic contributions. As users continue to adapt to these innovations, the pace of knowledge creation across various fields is likely to further accelerate.
- Unique Features and Capabilities
The automated research synthesis process boasts numerous features that empower researchers in their academic endeavors. One of its standout capabilities is the ability to analyze extensive literature rapidly. This feature allows researchers to pinpoint relevant studies and extract key insights, streamlining the overall research process. Moreover, it employs advanced natural language processing techniques to understand complex texts, ensuring that the data synthesized is both accurate and meaningful.
Additionally, the automated reports generated can be customized based on specific research needs. This flexibility ensures that the findings are tailored to particular academic requirements, enhancing the reporting's relevance and effectiveness. With ongoing improvements in machine learning algorithms, researchers can expect even more advanced synthesis capabilities in the future. Overall, these unique features position automated research synthesis as an essential tool for academic research, helping to enhance productivity and accuracy significantly.
- Best Use Cases for Academic Research
Automated Research Synthesis is particularly valuable in the realm of academic research, where efficiency and accuracy are paramount. Scholars often face daunting amounts of data, requiring a streamlined method to analyze and synthesize findings. AI-powered tools facilitate this process by evaluating vast bodies of literature, saving researchers hours of manual work. By automating repetitive tasks, these tools enable researchers to focus on critical analysis and interpretation, enhancing the depth and quality of their work.
Several use cases illustrate the effectiveness of this technology. First, it aids systematic reviews, allowing researchers to efficiently gather and synthesize relevant studies. Second, it supports literature mapping, helping identify trends and gaps in research fields. Additionally, AI-driven tools can produce concise summaries, making it easier for academics to extract key insights quickly. Overall, integrating Automated Research Synthesis into academic workflows can significantly enhance productivity and research outcomes.
Other Prominent AI Tools
Numerous AI tools complement the functionality of automated research synthesis, each bringing unique strengths to academic research. Tool 1 utilizes advanced natural language processing to streamline data analysis, significantly reducing the time spent on literature reviews. This tool is particularly effective for researchers looking to quickly identify trends and gaps in existing studies.
Tool 2 offers an intuitive interface that simplifies the drafting process. Researchers can leverage its features to create structured reports with easily identifiable sections. Meanwhile, Tool 3 specializes in collaborative features, allowing teams to work together and edit reports in real-time, enhancing overall productivity. Lastly, Tool 4 focuses on integrating databases, enabling users to seamlessly pull in relevant studies and references, thus enriching their manuscripts.
By employing these prominent AI tools, researchers can enhance their workflow and improve their outcomes in automated research synthesis, ultimately refining the quality of their academic reports.
- Tool 1: Overview and Features
Automated Research Synthesis is an innovative approach redefining how academic reports are generated. This tool streamlines the entire process, allowing users to effortlessly compile relevant literature and insights. Its intuitive interface ensures that anyone, regardless of their technical expertise, can navigate and access valuable resources. This democratization of data usage empowers more individuals to engage in in-depth research and analysis.
Key features of this tool include advanced content organization, efficient data analysis, and customizable report outputs. Users can quickly input large volumes of information for synthesis, transforming complex data into understandable insights. Furthermore, the tool's ability to extract key themes and evidence from conversations enhances the research quality, making it easier to identify customer pain points or desires. Overall, this tool is indispensable for anyone looking to enhance their academic research with minimal hassle.
- Tool 2: Overview and Features
The automated research synthesis tool offers an intuitive interface designed for ease of use. Users can quickly upload various types of data, such as interview transcripts or call recordings, which the system organizes into a centralized library. This feature makes it simple for anyone, regardless of expertise, to access and utilize the information efficiently. Additionally, the platform automatically extracts key insights like pain points and customer desires from the uploaded content, allowing for a sophisticated understanding of user needs without requiring extensive training.
Another noteworthy feature of this tool is its ability to analyze multiple pieces of content collectively. By grouping files into projects, users can synthesize findings from hundreds of calls simultaneously. This enhances the depth and breadth of analysis, providing rich, actionable insights that can drive decision-making. Overall, this tool represents a significant advancement in automated research synthesis, enabling streamlined academic research operations while democratizing valuable insights across teams.
- Tool 3: Overview and Features
In the domain of Automated Research Synthesis, Tool 3 offers unparalleled features designed to streamline academic report generation. This tool simplifies the process, allowing users from various backgrounds to engage with research data effortlessly. Within its user-friendly interface, researchers can easily input raw data, facilitating swift analysis and synthesis of key findings.
One of the standout features is its ability to analyze multiple datasets simultaneously, pulling together insights from various sources. Users can also visualize key trends, pain points, and significant themes through intuitive dashboards. This functionality not only saves time but enhances the quality of insights garnered. By ensuring that valuable research is accessible to anyone, Tool 3 plays a crucial role in democratizing academic research synthesis and empowering users to obtain actionable insights with minimal effort.
- Tool 4: Overview and Features
Automated Research Synthesis streamlines the process of combining existing research literature into coherent reports. This tool is designed to aid researchers by simplifying the synthesis of vast amounts of information. By harnessing advanced technologies, it allows users to transform interviews and calls into insightful data effortlessly. The ability to analyze multiple sources at once enhances productivity significantly.
One key feature of this tool is its intuitive interface, which does not require prior expertise. Users can upload calls or interviews, and the system will extract pertinent insights like pain points and themes. Furthermore, each insight is backed by evidence, such as direct quotes from participants, ensuring reliability. The capability to summarize findings and keywords from grouped projects makes navigating through large datasets straightforward. This tool empowers researchers to easily access valuable academic insights and foster informed decision-making.
Conclusion on the Role of Automated Research Synthesis in AI Academic Research Generators
Automated Research Synthesis is pivotal in enhancing the efficiency of AI Academic Research Generators. By systematically analyzing vast amounts of existing literature, these tools minimize the time researchers spend on data collection and synthesis. This efficiency allows scholars to focus more on interpretation and application of findings, rather than merely gathering information, thereby streamlining the research process.
Furthermore, Automated Research Synthesis fosters consistency and accuracy in reports. By employing advanced techniques such as natural language processing and machine learning, these systems can produce comprehensive analyses that remain free from human bias. Ultimately, the role of Automated Research Synthesis is not just about automation; it is about providing a reliable foundation for innovative academic research.
๐ฌ Questions about AI Academic Research Report Generator: How it Works?
Our team typically responds within minutes