Text Analysis Innovations are reshaping how researchers interpret qualitative survey data. In a world where customer conversations generate vast amounts of information, traditional analysis methods often fall short. These innovations enable organizations to transform raw data into actionable insights more swiftly and effectively, improving their decision-making processes.
By harnessing tools like Natural Language Processing (NLP) and sentiment analysis, researchers can unlock deeper understanding from qualitative surveys. With efficient data handling, researchers not only enhance collaboration but also ensure that vital insights are readily available. This introduction sets the stage for exploring advanced techniques that can bridge the gap between data collection and meaningful outcomes in qualitative research.
Analyze qualitative data. At Scale.
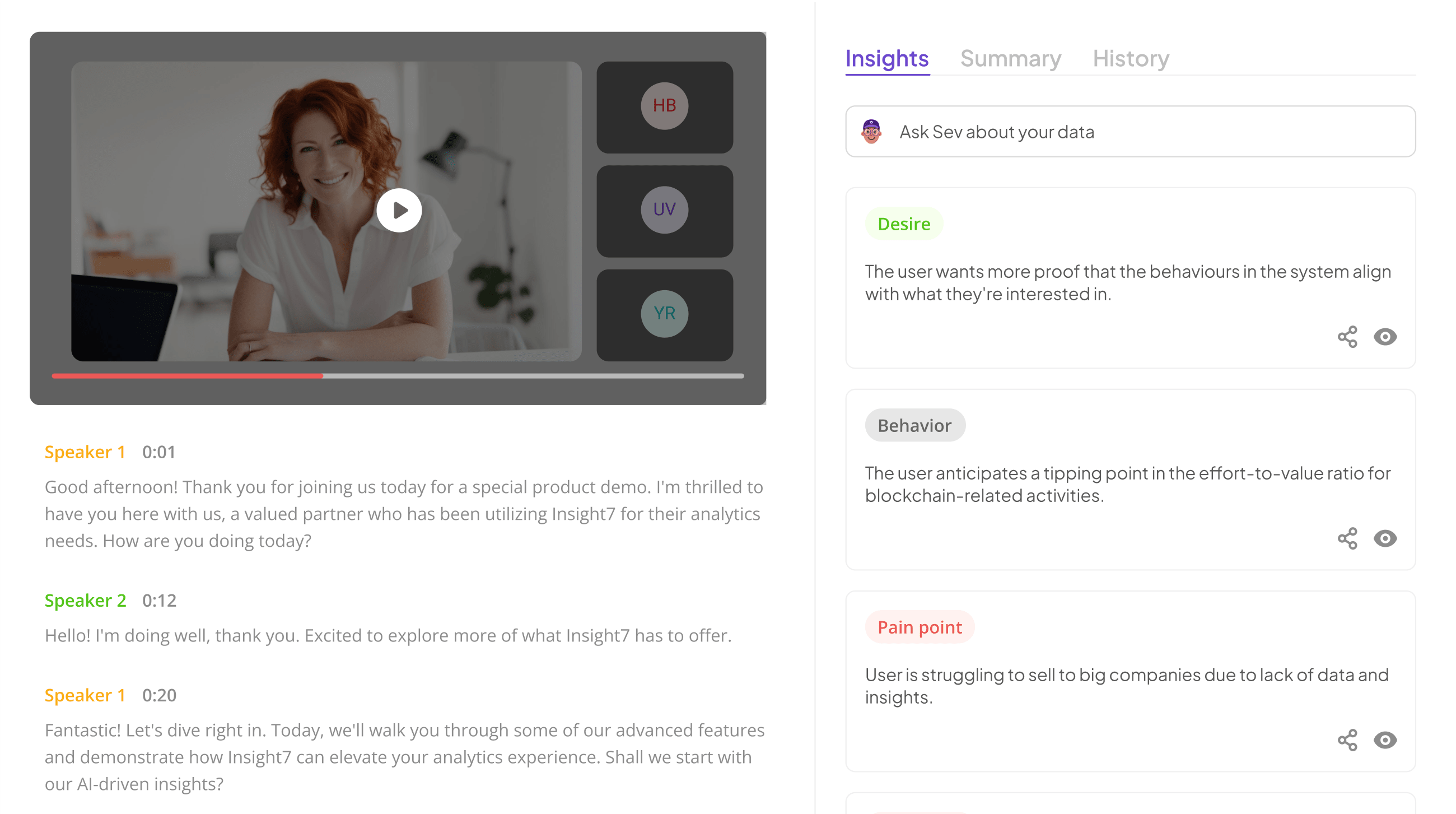
Understanding Text Analysis Innovations
Text Analysis Innovations represent a significant shift in how researchers process qualitative survey data. Traditional methods often fall short in addressing the vast amounts of data generated through customer interactions. As businesses capture increasing volumes of conversational insights, innovative text analysis techniques help streamline the process, allowing companies to draw meaningful conclusions quickly. The need to translate complex insights into strategic actions has never been more pressing.
One of the critical advancements in this field is the integration of technology with qualitative analysis. Techniques such as Natural Language Processing (NLP) play a pivotal role in preprocessing data. They enable efficient entity recognition and topic modeling, thus revealing underlying themes within customer responses. Additionally, by employing sentiment analysis and visualization tools, researchers can interpret emotional tones and visualize data trends more effectively. As these methodologies evolve, organizations that embrace these text analysis innovations will significantly enhance their competitive edge.
The Evolution of Text Analysis Techniques
Text analysis has undergone significant transformation over the years, largely driven by advancements in technology and methodology. Initially, analysts relied on manual techniques, applying basic statistical methods to extract insights from qualitative data. However, as data volumes increased, the limitations of traditional approaches became evident. Analysts struggled to process vast amounts of information swiftly and accurately, leading to a demand for more sophisticated techniques.
In response, numerous innovations emerged in text analysis tools and frameworks. These advancements include the development of Natural Language Processing (NLP) and machine learning algorithms, which allow for deeper analysis and the ability to identify patterns in text data more efficiently. As a result, businesses are now equipped to convert customer insights into actionable strategies swiftly, maintaining their competitive edge in a fast-paced market. These text analysis innovations not only enhance the quality of insights but also streamline the entire analysis process, ultimately shaping the future of qualitative research.
Modern Innovations in Text Analysis
Text analysis innovations have transformed how researchers approach qualitative data, making analysis faster and more insightful. Advances in Natural Language Processing (NLP) allow for the automatic identification of themes and sentiments within textual responses. This technology facilitates quick data preprocessing and enhances the accuracy of identifying significant insights that can directly influence business strategies.
Additionally, machine learning algorithms are now capable of analyzing vast amounts of textual data, providing nuanced interpretations that were previously unattainable. Techniques like topic modeling help researchers uncover trends and patterns, ensuring meaningful insights emerge from the data. Visual tools further enrich this process by presenting complex findings in accessible formats. These innovations not only streamline the analysis of qualitative surveys but also empower companies to act on customer insights more efficiently, staying ahead of the competition. Overall, understanding these modern approaches is essential for leveraging the full potential of qualitative research in todayโs data-driven landscape.
Techniques for Enhanced Qualitative Survey Analysis
Techniques for Enhanced Qualitative Survey Analysis combine innovative methodologies to extract meaningful insights from textual data. One key method is the application of Natural Language Processing (NLP), which enhances the understanding and classification of survey responses. Through the stages of data preprocessing and cleaning, NLP ensures that the data is accurate and reliable. It goes a step further with entity recognition and topic modeling, pinpointing critical themes that emerge from survey responses.
Sentiment analysis, another influential technique, plays a vital role in interpreting the emotional tone of text data. It allows researchers to gauge public opinion and sentiment towards specific topics effectively. Coupled with visualization tools, this approach transforms complex data into easily interpretable charts, making it simpler to communicate insights. By employing these advanced techniques, organizations can secure a competitive advantage, swiftly translating insights into actionable strategies.
Extract insights from interviews, calls, surveys and reviews for insights in minutes
Using Natural Language Processing (NLP)
Natural Language Processing (NLP) serves as a pivotal innovation in the domain of text analysis, particularly in qualitative surveys. This advanced technique facilitates the systematic analysis of large volumes of unstructured text data, helping researchers derive meaningful insights. By employing NLP, teams can swiftly preprocess and clean data, ensuring the quality and relevance necessary for accurate analysis. This step sets the foundation for the subsequent phases of the research process, making data more manageable and interpretable.
Moving forward, NLP excels in entity recognition and topic modeling. These methodologies enable researchers to identify key themes and trends within the data, creating a structured overview of customer sentiments and perspectives. As a result, organizations gain timely insights, empowering them to translate findings into actionable business strategies. With the constant evolution of text analysis innovations, harnessing NLP tools is essential for staying competitive in today's data-driven landscape.
- Step 1: Data Preprocessing and Cleaning
Data preprocessing and cleaning serve as critical initial steps in effectively harnessing Text Analysis Innovations for qualitative surveys. This process begins by gathering raw text data, typically in the form of transcripts from interviews or open-ended survey responses. The objective during this stage is to prepare this data for accurate analysis. This involves various techniques, such as removing irrelevant information, correcting typos, and standardizing text formats. By doing so, researchers can reduce noise in the data, allowing for clearer insights.
Moreover, data cleaning often includes handling missing values and filtering out any inconsistencies. Researchers might employ techniques like tokenization, where text is broken down into manageable parts, facilitating analysis. This foundational step ensures that subsequent analysis methods, such as natural language processing or sentiment analysis, yield reliable and actionable insights. Ultimately, effective data preprocessing sets the stage for the innovative exploration of qualitative data, leading to more informed decisions and deeper understanding.
- Step 2: Entity Recognition and Topic Modeling
In the process of advanced text analysis innovations, Step 2 focuses on Entity Recognition and Topic Modeling. This crucial stage enhances the ability to extract meaningful insights from qualitative survey data. By employing Natural Language Processing (NLP) techniques, researchers can automatically identify and classify key entities such as names, dates, and events. This identification is essential for understanding context and relevance, allowing for a deeper analysis of responses.
Moreover, topic modeling plays a vital role in uncovering hidden patterns within the data. Through algorithms that cluster similar themes, researchers can quickly recognize prevalent topics among respondents. This not only saves time but also highlights significant areas of interest, which might not be immediately apparent. Together, entity recognition and topic modeling empower researchers to reveal complex insights, paving the way for more informed decision-making and targeted strategies in qualitative survey analysis.
Sentiment Analysis and Visualization Tools
Sentiment analysis is an essential tool in understanding emotional responses shared in qualitative surveys. By utilizing natural language processing, analysts can gain insights into the sentiments conveyed by respondents. This process involves categorizing responses as positive, negative, or neutral, thereby providing a clearer picture of public opinion regarding a product or service. This method helps organizations identify key themes in customer feedback, enabling more informed decision-making.
Visualization tools enhance this analysis by presenting sentiment data in an easily digestible format. Graphs, charts, and word clouds allow stakeholders to interpret and interact with data dynamically. This visual clarity ensures that insights derived from sentiment analysis can influence strategic directions effectively. Using these tools, professionals can quickly compare different datasets, reveal trends, and uncover unique patterns that traditional analysis might overlook. Overall, integrating sentiment analysis and visualization tools into text analysis innovations empowers organizations to harness qualitative data for meaningful insights.
- Step 1: Analyzing Sentiment with NLP
Analyzing sentiment with Natural Language Processing (NLP) serves as a crucial first step in the realm of text analysis innovations, particularly in qualitative surveys. By employing machine learning algorithms, NLP techniques can systematically assess emotions expressed in textual data. This allows researchers to gain deeper insights into participant attitudes, whether they are positive, negative, or neutral.
To begin, sentiment analysis can be divided into three key components. First, data cleaning involves removing irrelevant information, ensuring that the analysis focuses on meaningful content. Next, feature extraction enables the identification of words or phrases that carry emotional weight, forming the basis for sentiment classification. Finally, sentiment classification categorizes identified sentiments, offering a quantitative measure of public perception.
Through these steps, organizations can effectively transform raw qualitative data into actionable insights, enhancing decision-making processes and fostering greater understanding of customer mindsets. Employing these advanced techniques allows for a more nuanced approach to qualitative survey analysis, paving the way for informed strategies and outcomes.
- Step 2: Visualizing Data Insights for Better Interpretation
Visualizing data insights is critical for understanding qualitative survey results effectively. By presenting information in engaging formats, stakeholders can easily grasp complex patterns and themes that emerge from responses. Tools such as word clouds, bar graphs, and sentiment heatmaps enhance interpretation of data trends. These visualizations allow researchers to highlight positive and negative sentiments, making it clear which aspects resonate with audiences or require attention.
Moreover, advanced visualization techniques support comparative analysis, enabling researchers to juxtapose different datasets, such as feedback from varied demographic groups. This approach uncovers hidden insights within diverse responses, fostering a deeper understanding of user experiences. A vital part of these text analysis innovations is the ability to interactively query and manipulate data visuals, allowing researchers to dig deeper into specific themes and sentiments. Properly harnessing these visual tools will significantly enhance decision-making processes while ensuring that insights from text analysis are actionable and grounded in evident user sentiments.
Tools and Technologies Transforming Text Analysis
Text Analysis Innovations are reshaping how researchers handle qualitative data, enhancing efficiency and accuracy. Modern applications are transforming traditional methodologies, allowing for more in-depth insights into textual information. The integration of advanced technologies, such as machine learning and natural language processing, enables researchers to dissect large volumes of data swiftly.
Key tools in this arena are optimizing text analysis processes. For instance, software like NVivo and ATLAS.ti provides advanced data coding and visualization options. Similarly, MAXQDA and Dedoose offer comprehensive mixed-methods analysis, helping researchers synthesize qualitative data effectively. Additionally, tools like QDA Miner specialize in text mining, making it easier to extract meaningful content from extensive datasets.
These innovations not only enhance the accuracy of insights but also empower researchers to visualize results, thereby supporting better decision-making and strategic planning in qualitative research.
Top Tools for Text Analysis Innovations
Emerging tools for text analysis innovations are transforming how researchers engage with qualitative survey data. These technologies are designed to simplify the complex process of data interpretation, making it more efficient and accessible. Each tool offers unique features that enable researchers to analyze extensive customer feedback, interviews, and open-ended survey responses at scale. With the right tools, insights can be extracted quickly and accurately, allowing for timely strategic decisions.
Among the top tools in this arena are NVivo and ATLAS.ti, which excel in qualitative data analysis and coding. MAXQDA also stands out with its capabilities for mixed methods research, while Dedoose provides a web-based approach for qualitative and quantitative analysis. QDA Miner enhances text mining and content analysis, making it easier for researchers to visualize trends and sentiments. By leveraging these advanced tools, researchers can drive actionable insights that advance their objectives and respond effectively to customer needs.
- Insight7
Text analysis innovations are reshaping how we approach qualitative surveys. One notable advancement is the integration of natural language processing (NLP) techniques that streamline data preprocessing and enhance engagement with customer feedback. By analyzing large volumes of text data quickly, organizations can derive meaningful insights that would otherwise be overlooked.
Focusing on innovations, we explore critical techniques for enhanced qualitative survey analysis. Initially, effective data cleaning sets the stage for precise entity recognition and topic modeling. Following this, sentiment analysis tools allow researchers to gauge emotional responses, offering a deeper understanding of customer perceptions. With these insights readily visualized, interpreting and translating this data into actionable strategies becomes more coherent and efficient. Utilizing these advanced techniques ensures organizations stay competitive by effectively responding to emerging customer needs and preferences.
- NVivo: Robust Qualitative Data Analysis
NVivo provides researchers with a powerful platform for robust qualitative data analysis. It allows users to efficiently organize, analyze, and visualize data from various sources, such as interviews and surveys. When integrating this tool into text analysis innovations, it simplifies the process of extracting insights from large qualitative datasets.
One of the key features of NVivo is its project management capabilities. Users can create projects to collate and manage their qualitative data systematically. By utilizing advanced coding techniques and queries, researchers can identify patterns and trends that inform their analyses. Additionally, features like sentiment analysis and data visualization enable a deeper understanding of respondents' perspectives, further enhancing the insights generated from qualitative surveys. Hence, NVivo plays an essential role in driving text analysis innovations in qualitative research, supporting researchers in uncovering valuable insights.
- ATLAS.ti: Advanced Data Coding and Visualization
Advanced data coding and visualization play crucial roles in enhancing text analysis innovations, especially in qualitative surveys. By streamlining the process of organizing and interpreting qualitative data, they provide researchers with powerful tools to unlock insights quickly. With features designed for efficient data management, you can create projects that gather diverse information sources. Each project functions as a comprehensive exploration hub where various documents can be uploaded, allowing for thorough analysis.
Within this framework, advanced visualization techniques help in revealing patterns and trends that might not be immediately apparent. For instance, visualizing historical queries can provide immediate context for your analysis, making it easier to identify key themes from customer feedback. As you analyze sentiments, the ability to create matrix analyses enables you to summarize findings succinctly, showcasing comparative insights. Ultimately, these methodologies enhance the interpretative power of qualitative surveys, ensuring that you derive clear conclusions from complex data sources.
- MAXQDA: Comprehensive Mixed Methods Data Analysis
In the realm of qualitative research, MAXQDA offers a vital platform for comprehensive mixed methods data analysis. This tool empowers researchers to synthesize disparate data types, seamlessly merging qualitative insights with quantitative metrics. Such versatility is essential for creating a rounded understanding of complex datasets. It allows for efficient project setup, where you can easily import various formats like transcripts and audio files, aligning data for thorough examination.
Text analysis innovations shine through MAXQDA's functionalities, enabling users to visualize trends and extract meaningful themes. With features like matrix analysis, researchers can pose targeted questions, derive insights, and identify patterns across substantial datasets. The ability to summarize findings in intuitive formats enhances the interpretation process. Thus, the commitment to advanced text analysis techniques positions this tool as an invaluable resource for researchers seeking to delve deep into qualitative surveys.
- Dedoose: Web-based Qualitative and Mixed-Methods Research
For qualitative researchers seeking an effective platform for analysis, the web-based option offers several advantages. It supports both qualitative and mixed-methods research, streamlining the process of gathering and analyzing data. By allowing users to upload various file types and manage projects efficiently, it creates a seamless workflow for analyzing large volumes of text.
One of the standout features is the ability to visualize data through tables and matrices, which simplify identifying trends and patterns across participantsโ feedback. This aids researchers in deriving actionable insights quickly, ultimately enhancing the overall research quality. Furthermore, by integrating various data sources, researchers can ensure that no critical data goes unnoticed, which is essential for comprehensive text analysis innovations. As such, this platform not only enhances the qualitative research experience but also significantly enriches the quality of data insights produced.
- QDA Miner: Innovative Text Mining and Content Analysis
QDA Miner stands out as a leading tool in text analysis innovations for qualitative research. Its unique capabilities allow users to conduct in-depth content analysis quickly and effectively. Researchers can easily organize and categorize various text sources, extracting meaningful insights from qualitative data. This process is essential for generating reliable results in qualitative surveys, ensuring every detail is accounted for in their findings.
With features like project management, audio file transcription, and data visualization, QDA Miner enhances user experience. Researchers can effortlessly compile various data sources, enabling them to analyze patterns and trends across different conversations. This functionality not only streamlines the qualitative analysis process but also empowers teams to derive actionable insights rapidly. In such an evolving research environment, utilizing tools that embody text analysis innovations is crucial for gaining a competitive edge in understanding qualitative data.
Conclusion: The Future of Text Analysis Innovations in Qualitative Research
The future of text analysis innovations in qualitative research promises significant advancements that will reshape how researchers gather and interpret data. As tools and techniques evolve, researchers will increasingly harness AI and machine learning, allowing for deeper insights and more nuanced understanding of qualitative data. The potential for improved natural language processing will facilitate more accurate sentiment analysis and topic modeling, enhancing the richness of qualitative stories extracted from survey responses.
Moreover, the integration of visualization tools will aid researchers in presenting complex data in intuitive formats, making insights more accessible. These innovations not only streamline data analysis but also empower researchers to extract actionable insights rapidly. Ultimately, embracing these developments will help advance the field, providing more robust frameworks for qualitative research.